-
PDF
- Split View
-
Views
-
Cite
Cite
Tammy Toscos, Michelle Drouin, Jessica A Pater, Mindy Flanagan, Shauna Wagner, Amanda Coupe, Ryan Ahmed, Michael J Mirro, Medication adherence for atrial fibrillation patients: triangulating measures from a smart pill bottle, e-prescribing software, and patient communication through the electronic health record, JAMIA Open, Volume 3, Issue 2, July 2020, Pages 233–242, https://doi.org/10.1093/jamiaopen/ooaa007
- Share Icon Share
Abstract
Our primary objectives were to examine adherence rates across two technologies (e-prescribing software and smart pill bottle) with cross-validation from alert-triggered messaging within the patient electronic health record (EHR) portal and to explore the benefits and challenges faced by atrial fibrillation (AF) patients in using a smart pill bottle.
We triangulated the rate of oral anticoagulant medication adherence among 160 AF patients over 6 months using an EHR in combination with data from the AdhereTech© Wireless Smart Pill Bottle and Surescripts©. In addition, we collected qualitative feedback on patients’ Smart Pill Bottle usage through structured interviews with 153 participants.
Patients maintained an average adherence rate of 90.0% according to the smart pill bottle; however, when dose misses were calibrated based on patient or provider feedback, the adjusted adherence was 93.6%. Surescripts adherence rates for refills were 92.2%. Participants generally found the bottle easy to operate but suggested that its size and functionality did not fit seamlessly into their existing routine, as many used weekly pill organizers to manage multiple medications.
Though each method of tracking adherence has positive and negative attributes, combining them and seeking patient feedback may help capture a more accurate adherence rate than any single technological intervention. Technologies may have different design considerations for research and consumer use.
Overall, these technologies provide useful but imperfect adherence data for research purposes, and smart pill bottles could be improved with patient-centered design.
Medication adherence is very important for those with chronic health issues. For those with heart disease, medication adherence not only offers opportunities for improving quality of life, but it also can be life-saving. Nonetheless, many patients with heart disease, including those with atrial fibrillation (the target group for this study) do not take their medications regularly. As technologies advance, there is unprecedented opportunity to track patients’ medication adherence through various methods, which might provide motivation and information to patients as they make daily choices about medication use. In this study, we cross-referenced the results of two of these measures over 6 months—a smart pill bottle, which we used to track pill bottle openings, and e-prescribing software, which we used to track medication refills. We also supplemented these measures with nurse-patient communication via the EHR messaging portal to record exceptions (eg, travel and medication changes) and interviewed patients about their medication use during the 6-month trial. Overall, the tracking technologies worked relatively well to track patient (n = 160) medication behavior; however, they did not capture exceptions. Hence, triangulating data from different sources, with a patient feedback loop, appears critical for gathering accurate data on medication adherence.
INTRODUCTION
Background and significance
Atrial fibrillation (AF) is the most common heart arrhythmia,1 predicted to affect 6–12 million Americans by 2050.2 Patients with AF are typically prescribed oral anticoagulant (OAC) medication to reduce the risk of clot-related complications related to the arrhythmia; however, nonadherence to these medications can have severe and even fatal effects.3,4 AF contributes to least 1 in 6 strokes,5,6 which tend to be more severe than strokes due to other causes7 and cost over $8 billion per year,8 rendering OAC adherence in AF patients both an individual and public health issue. The AF patient population generally consists of adults age 65+,9 and AF-related knowledge among these patients if sometimes lacking.10
Medication adherence is a multifaceted process involving factors like motivation, patient information, and learned behaviors.11 Research on technologies that support medication adherence, including adherence among those with cardiovascular conditions, has flourished in recent years.12–19 These technologies track and/or support adherence through a variety of mechanisms. One such technology is electronic pill bottles, the newest versions of which can not only track pill bottle openings, but also alert patients to missed doses and remotely transmit signals so that clinicians can be alerted of such misses.16,18,20,21 As their prices have become more affordable and research has demonstrated their relative accuracy in tracking medication adherence, smart pill bottles are gaining popularity as a mechanism for medication behavior change.22 Another innovation is e-prescribing software, like Surescripts, which collects data on patients’ medication histories and refills, which can be shared with doctors, clinics, and pharmacists. Currently, Surescripts collects medication history information from approximately 93% of insured patients and 79% of the general US population.23 Through partnerships with EHR vendors, Surescripts feeds information directly into many electronic health records (EHRs), which has expanded the opportunity for communication of medical histories across and within platforms.24
These innovations were designed to help identify lapses in adherence by reporting information back to the patient either directly, or indirectly through clinicians, in order to prompt behavior change.25 Although some studies reported marked increases of adherence with the use of technology,13,15,26 others showed no significant effects.19 The reasons for these inconsistencies may be design issues,27 lack of long-term engagement,19 and limitations in study methodologies.28 Even though the use of adherence technologies is generally considered more reliable than self-reports,16 they are often not nuanced enough to capture when the use of the technology does not align with the intended use (eg, when multiple doses are removed and placed into an alternative pill sorter). Moreover, behavior change might be limited when smart pill bottle remote transmissions or e-prescribing medical history data are not sufficiently integrated into clinical practice or shared back with the patient in a meaningful way.
Recent research has attempted to explore the accuracy of these technologies, showing that medication adherence rates vary depending on the way adherence is measured. In one study, although smart pill bottle readings and pill counting were strongly and significantly related, smart pill bottle readings were not strongly concordant with plasma levels, and patients’ self-reports tended to overestimate their adherence as compared to smart pill bottle readings.29 More recently, Mehta et al22 showed that for myocardial infarction patients, higher rates of statin adherence were recorded when using medication refill information (72%) as compared to remote pill bottle records (68%). However, notably, neither of these studies noted any feedback loop between the clinician (or researcher) and the patients, so it is unknown whether these adherence levels accurately reflect true missed dosages. Moreover, no known studies have explored the extent to which this feedback loop could prompt patient response, which could potentially provide impetus for more sustained medication adherence tracking.
Objective
The remote monitoring feature of pill bottles paired with e-prescribing software offers an unprecedented opportunity to: (1) compare adherence measures across these technologies and (2) examine the extent to which patients are receptive and responsive to clinical feedback based on data from these technologies (ie, the feedback loop). Building on past work on engaging cardiology patients with their health data30–33 and taking into consideration the known issues associated with technology-driven medication adherence approaches, we triangulated these adherence measures (smart pill bottle, medication refill information, and patient messages about missed doses). We also created a feedback loop whereby nurses would provide information to patients when they missed doses. We also solicited AF patient feedback via interviews regarding use of the smart pill bottle. The associations between these three adherence measures are displayed in Figure 1.
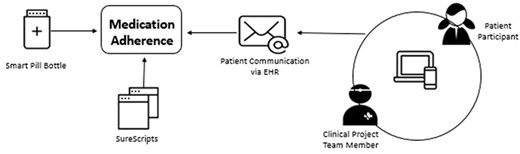
METHODS
Adult AF patients receiving outpatient care within a mid-size Midwestern hospital system were recruited by research team members, using referrals from cardiology clinic staff and chart review (n = 160). All participants had been prescribed a daily direct oral anticoagulant (DOAC) (Eliquis (apixaban) n = 52; Xarelto (rivaroxaban) n = 28) or warfarin (Coumadin n = 80). (Pradaxa (dabigatran) patients were excluded because Pradaxa (dabigatran) tablets should be stored only in their original pill bottles to preserve the tablets.) As part of a larger study evaluating the impact of tailored education on medication adherence, patients were randomized into two groups (see Figure 2). Data from both groups were analyzed for the current study.
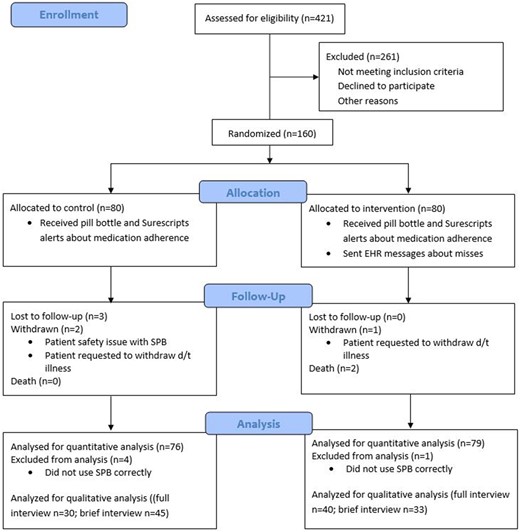
Medication adherence
Medication adherence was tracked via three methods, as described below.
Smart pill bottle
Upon enrollment, daily dosage information, including usual medication schedule, was entered by research staff into the pill bottle software application, which was accessible only to the study team. Participants were provided with the study personnel’s telephone number and email address to communicate any medication or schedule change occurring throughout the study, and this was updated immediately in the participant’s dashboard. All participants received a Wireless Smart Pill Bottle by AdhereTech (Figure 3A) with alert and reminder functionality (ie, lights/sounds for dosages due or missed) disabled. The bottle’s sensors recorded doses as missed, late, early, or on time, based on the date and time the participant removed and replaced the bottle cap, and this was displayed in a dashboard, inspected by study staff (see Figure 4). As many AF patients take multiple medications, participants were instructed to use the bottle for their OAC medication only. If the participant used a weekly pill organizer, they were given Bingo chips to use as a visual placeholder for their OAC in their own organizers but were still instructed to keep their OAC medication in the smart pill bottle.
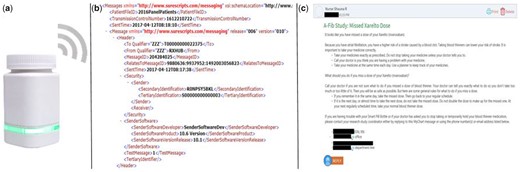
(A) Smart pill bottle; (B) Surescripts.xml data feed; (C) sample reminder message sent by study staff based on alert.
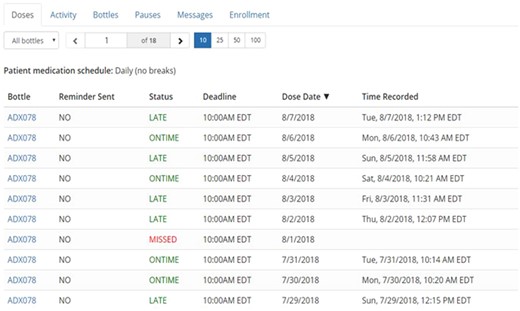
Smart pill bottle dashboard utilized by research staff to record missed doses.
E-prescribing software
Pharmacy refill records were obtained directly from a Surescripts data feed outside of the EHR (MyChart). This data feed (see Figure 3B) was in .xml format, so was translated by business informatics staff into a simple status update (late or on time). These prescription refill data do have the ability to be pulled directly into an EHR, but that was not done in this study. Instead, study staff screened translated records weekly for alerts that prescription refills for OACs or warfarin were overdue.
Patient messages through EHR
Reconciliation of missed pills and refills was attempted via messaging to patients within the EHR (Figure 3C). At enrollment, participants received assistance in setting up their account if they were new to MyChart, training on the general use of the EHR, and an interactive demonstration to show them how to access these messages on a test account and view a sample message. Remote pill bottle transmissions were monitored daily (Monday–Friday) by the study team, and one member of the study team alerted patients within 24 h following a patient missing a full day of their DOAC or two consecutive doses of warfarin. Patients received a second and third message (both unique messages) via the EHR if they missed 2 d of OAC or 4 d of warfarin. In response to prescription refill alerts, study staff sent messages to patients when their prescription refill was overdue by at least a week. Patients who received messages from the study team, triggered by smart pill bottle or Surescripts alerts, were reminded of the importance of medication adherence and asked to reply with explanations for missed doses and/or notify the study team of upcoming doses that would be missed (ie, doctor ordered a medication hold; patient leaving for vacation and bringing only their weekly pill organizer). (Separate from these reminder messages, intervention group participants received weekly educational messages as part of a separate hypothesis about the effect of educational interventions on medication adherence. Detailed descriptions of these messages and the results of this intervention are described in other work; the present study focuses on the methodology and feasibility of capturing adherence via this multifaceted approach.) Patients were also encouraged at enrollment to message study staff during the course of the study if there were any changes or interruptions to their medication schedule.
Patient experiences with pill bottle
We conducted 148 semi-structured face-to-face exit interviews with participants (full interview n = 70, abbreviated interview n = 78); 12 participants did not complete exit interviews due to withdrawal, death, or inability to contact. During the interview, patients were asked details about their missed doses and any communication (or lack of communication) with study staff. To aid their memory, research personnel showed patients logs of missed doses, Surescripts data, and missed refill messages, if applicable. In addition, they were asked about their experiences with the smart pill bottle. Interviews, lasted approximately 20 min to 1 h, were held in a private room in the research center, and were audio-recorded and transcribed for analysis.
Data analysis
Quantitative
Of the 160 enrolled patients, 155 were included in quantitative analyses. Those that were excluded had missing or unreliable (due to noncompliance with instructions) adherence data. We were interested in two main quantitative metrics: (1) adherence rates across the two technologies (both raw, and, for the smart pill bottle, adjusted, supplemented by patient feedback and other notes within EHR), and (2) messaging information (ie, messages sent to and from patient via EHR) for medication adherence reconciliation. For the smart pill bottles, the raw adherence rate was the ratio of doses that were marked as taken (late, early, or on-time) relative to the scheduled doses the participant was ordered to take during the study period. We also calculated an “adjusted” score for pill bottle adherence. For each dose marked by the pill bottle as missed, a clinical nurse researcher inspected the EHR to determine if there were documented, acceptable explanations as communicated from the patient via MyChart messages. These explanations were cross-referenced and supplemented with clinician notes from the EHR, or records from the Anticoagulation Therapy Unit (ATU) Clinic. If there was an acceptable reason noted for any of the doses marked as missed by the smart pill bottle, this was documented as an “allowed missed dose.” The allowed missed doses were subtracted from total missed doses and the adherence percentage was recalculated.
Qualitative
An inductive thematic analysis was conducted with all interview data. In this type of analysis, codes, or brief labels, are used to represent concepts identified in the qualitative data. The analysis team members independently reviewed the same transcript and identified concepts related to patients’ use of the smart pill bottle. For each concept, a provisional code was created. The team members met to compare codes, discuss discrepancies, and establish a final code set. This iterative process continued until the set of codes was stable, demonstrating conceptual saturation. Then, each transcript was coded independently by two team members, who would meet to compare codes and reach consensus. Team members were paired with a new partner each week to prevent divergence in how codes were applied. Data were coded using nVivo10 software. Once coding was complete, all data were aggregated and abstracted into summaries for each code.
RESULTS
Participants were 37.5% (60/160) female and 98.1% (153/156) white with an average age of 71 years. Most had completed at least some college (75.3%), were retired (73.0%), and lived with a spouse or partner (77.9%). See Table 1 for complete participant demographics.
Characteristic . | Percent . | Count . |
---|---|---|
Gender | ||
Female | 37.5 | 60/160 |
Male | 62.5 | 100/160 |
Age | ||
41–50 | 1.3 | 2/160 |
51–60 | 11.3 | 18/160 |
61–70 | 31.3 | 50/160 |
71–80 | 46.3 | 74/160 |
81–90 | 9.4 | 15/160 |
91–100 | 0.6 | 1/160 |
Race | ||
White | 98.1 | 153/156 |
Asian | 0.6 | 1/156 |
Other | 1.3 | 2/156 |
Employment status | ||
Employed full time | 13.8 | 22/159 |
Employed part time | 5.0 | 8/159 |
Unemployed | 1.9 | 3/159 |
Disabled/unable to work | 5.7 | 9/159 |
Retired | 73.0 | 116/159 |
Education level | ||
Did not graduate high school | 3.3 | 5/154 |
High school graduate/GED | 21.4 | 33/154 |
Trade/some college | 29.9 | 46/154 |
College graduate | 25.3 | 39/154 |
Postgraduate degree | 20.1 | 31/154 |
Annual income | ||
Less than $20,000 | 5.4 | 8/149 |
$20,000–40,000 | 22.8 | 34/149 |
$40,001–60,000 | 24.2 | 36/149 |
$60,001–80,000 | 24.8 | 37/149 |
$80,001–100,000 | 9.4 | 14/149 |
Over $100,000 | 13.4 | 20/149 |
Household occupants | ||
Alone | 16.5 | 26/158 |
With a spouse or partner | 77.9 | 123/158 |
With a family member | 5.1 | 8/158 |
With a friend | 0.6 | 1/158 |
Characteristic . | Percent . | Count . |
---|---|---|
Gender | ||
Female | 37.5 | 60/160 |
Male | 62.5 | 100/160 |
Age | ||
41–50 | 1.3 | 2/160 |
51–60 | 11.3 | 18/160 |
61–70 | 31.3 | 50/160 |
71–80 | 46.3 | 74/160 |
81–90 | 9.4 | 15/160 |
91–100 | 0.6 | 1/160 |
Race | ||
White | 98.1 | 153/156 |
Asian | 0.6 | 1/156 |
Other | 1.3 | 2/156 |
Employment status | ||
Employed full time | 13.8 | 22/159 |
Employed part time | 5.0 | 8/159 |
Unemployed | 1.9 | 3/159 |
Disabled/unable to work | 5.7 | 9/159 |
Retired | 73.0 | 116/159 |
Education level | ||
Did not graduate high school | 3.3 | 5/154 |
High school graduate/GED | 21.4 | 33/154 |
Trade/some college | 29.9 | 46/154 |
College graduate | 25.3 | 39/154 |
Postgraduate degree | 20.1 | 31/154 |
Annual income | ||
Less than $20,000 | 5.4 | 8/149 |
$20,000–40,000 | 22.8 | 34/149 |
$40,001–60,000 | 24.2 | 36/149 |
$60,001–80,000 | 24.8 | 37/149 |
$80,001–100,000 | 9.4 | 14/149 |
Over $100,000 | 13.4 | 20/149 |
Household occupants | ||
Alone | 16.5 | 26/158 |
With a spouse or partner | 77.9 | 123/158 |
With a family member | 5.1 | 8/158 |
With a friend | 0.6 | 1/158 |
Characteristic . | Percent . | Count . |
---|---|---|
Gender | ||
Female | 37.5 | 60/160 |
Male | 62.5 | 100/160 |
Age | ||
41–50 | 1.3 | 2/160 |
51–60 | 11.3 | 18/160 |
61–70 | 31.3 | 50/160 |
71–80 | 46.3 | 74/160 |
81–90 | 9.4 | 15/160 |
91–100 | 0.6 | 1/160 |
Race | ||
White | 98.1 | 153/156 |
Asian | 0.6 | 1/156 |
Other | 1.3 | 2/156 |
Employment status | ||
Employed full time | 13.8 | 22/159 |
Employed part time | 5.0 | 8/159 |
Unemployed | 1.9 | 3/159 |
Disabled/unable to work | 5.7 | 9/159 |
Retired | 73.0 | 116/159 |
Education level | ||
Did not graduate high school | 3.3 | 5/154 |
High school graduate/GED | 21.4 | 33/154 |
Trade/some college | 29.9 | 46/154 |
College graduate | 25.3 | 39/154 |
Postgraduate degree | 20.1 | 31/154 |
Annual income | ||
Less than $20,000 | 5.4 | 8/149 |
$20,000–40,000 | 22.8 | 34/149 |
$40,001–60,000 | 24.2 | 36/149 |
$60,001–80,000 | 24.8 | 37/149 |
$80,001–100,000 | 9.4 | 14/149 |
Over $100,000 | 13.4 | 20/149 |
Household occupants | ||
Alone | 16.5 | 26/158 |
With a spouse or partner | 77.9 | 123/158 |
With a family member | 5.1 | 8/158 |
With a friend | 0.6 | 1/158 |
Characteristic . | Percent . | Count . |
---|---|---|
Gender | ||
Female | 37.5 | 60/160 |
Male | 62.5 | 100/160 |
Age | ||
41–50 | 1.3 | 2/160 |
51–60 | 11.3 | 18/160 |
61–70 | 31.3 | 50/160 |
71–80 | 46.3 | 74/160 |
81–90 | 9.4 | 15/160 |
91–100 | 0.6 | 1/160 |
Race | ||
White | 98.1 | 153/156 |
Asian | 0.6 | 1/156 |
Other | 1.3 | 2/156 |
Employment status | ||
Employed full time | 13.8 | 22/159 |
Employed part time | 5.0 | 8/159 |
Unemployed | 1.9 | 3/159 |
Disabled/unable to work | 5.7 | 9/159 |
Retired | 73.0 | 116/159 |
Education level | ||
Did not graduate high school | 3.3 | 5/154 |
High school graduate/GED | 21.4 | 33/154 |
Trade/some college | 29.9 | 46/154 |
College graduate | 25.3 | 39/154 |
Postgraduate degree | 20.1 | 31/154 |
Annual income | ||
Less than $20,000 | 5.4 | 8/149 |
$20,000–40,000 | 22.8 | 34/149 |
$40,001–60,000 | 24.2 | 36/149 |
$60,001–80,000 | 24.8 | 37/149 |
$80,001–100,000 | 9.4 | 14/149 |
Over $100,000 | 13.4 | 20/149 |
Household occupants | ||
Alone | 16.5 | 26/158 |
With a spouse or partner | 77.9 | 123/158 |
With a family member | 5.1 | 8/158 |
With a friend | 0.6 | 1/158 |
Medication adherence rates
Overall, 88% (141/160) participants had available Surescripts data because they used a Surescripts enabled pharmacy, and 92.2% of patients (130/141) were adherent with refilling their medication over the 6-month project. Twenty percent (39/160) participants had no Surescripts data available. Meanwhile, the raw smart pill bottle average adherence rate was 90.0% (SD = 10.8, see Table 2). In contrast, the adjusted pill bottle medication average adherence rate was 93.6% (SD = 8.2). The distribution of these adjusted pill bottle adherence rates is displayed in Figure 5. In considering the difference between these two rates for each participant, the range of discrepancies was −0.1% to 50.0%, with the raw adherence rates tending to be lower than the adjusted rates (mean difference = 3.6%; SD = 6.9). The average number of allowed missed doses was 8 (SD = 19, median = 1, range = 0–133).
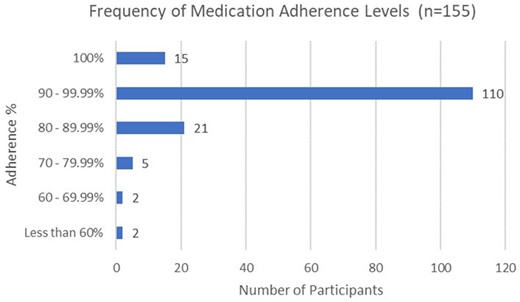
Distribution of adjusted adherence rates from smart pill bottle.
Means, minimums, and maximums for percentage of medication adherence and adjusted medication adherence (n = 155)
Summary statistic . | Pill bottle adherence . | Pill bottle adjusted adherence . |
---|---|---|
Mean adherence percentage | 90.0 | 93.6 |
Minimum | 44.6 | 51.7 |
Maximum | 100.0 | 100.0 |
Summary statistic . | Pill bottle adherence . | Pill bottle adjusted adherence . |
---|---|---|
Mean adherence percentage | 90.0 | 93.6 |
Minimum | 44.6 | 51.7 |
Maximum | 100.0 | 100.0 |
Means, minimums, and maximums for percentage of medication adherence and adjusted medication adherence (n = 155)
Summary statistic . | Pill bottle adherence . | Pill bottle adjusted adherence . |
---|---|---|
Mean adherence percentage | 90.0 | 93.6 |
Minimum | 44.6 | 51.7 |
Maximum | 100.0 | 100.0 |
Summary statistic . | Pill bottle adherence . | Pill bottle adjusted adherence . |
---|---|---|
Mean adherence percentage | 90.0 | 93.6 |
Minimum | 44.6 | 51.7 |
Maximum | 100.0 | 100.0 |
Messaging information
Of the 80 participants eligible to receive MyChart messages for medication nonadherence alerts, a total of 138 messages alerting about missed medication doses (triggered by smart pill bottle alerts) were sent to 50 participants, and 45 messages alerting about missed medication refills (triggered by Surescripts alerts) were sent to 18 participants. As some participants (n = 13) received both smart pill bottle and Surescripts triggered messages, 68.8% (55/80) participants in total received either smart pill bottle or Surescripts triggered messages (M = 3.3, SD = 2.3, range = 1–11). For both message types, the number of responses from participants addressing the reason for the missed dose was recorded. As shown in Figure 6, regardless of the number of messages sent (1, 2 or 3, or 4+), patients were more likely to respond to none of the messages than to respond to at least some messages. Overall, 65.5% (36/55) participants did not respond to any messages received. In addition, for 33.3% (16/48) of those with nonresponse or partial response, one or more MyChart messages were not read. However, 34.5% (19/55) did respond to the EHR messages with explanations, and 20.0% (11/55) sent unsolicited messages to study staff with explanations for missed doses.
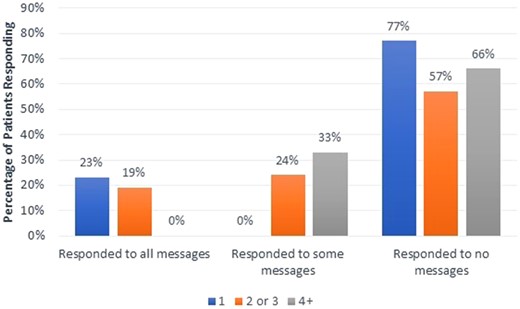
Percentage of patients (n = 80) who responded to EHR alert messages based on number of total messages sent (1, 2 or 3, or 4+).
During the exit interviews, patients reflected on the impact of the messaging with the study staff. When asked about the messages sent, participants reflected positively, that “I was delighted that I was told that I missed a dose…it put me right back on [my schedule]” (P109). Patients reported they valued the human interaction with the team via the communication feedback loop. Participants appreciated the responsiveness, indicating that “she responded to me every time, every time she answered me” (P127). They also valued how the messaging made them feel closer to the research as exemplified by P153: “I felt like I was really part of the program. Knowing I could send her a message, but not that she expected one all of the time.”
Patient experience with smart pill bottle
There were three main themes that emerged regarding patients’ experiences with the smart pill bottle technology, as described below.
Deviation from typical routine
Many participants already had a personalized medication routine, using primarily event- or location-based cues (eg, a pill organizer on their bathroom sink, taking pills with meals). Some easily integrated the smart pill bottle into this routine, whereas others had complaints, with several specifically calling it “a pain.” In the former instance, a few participants noted that the bottle itself served as a visual cue: “And it wasn’t an inconvenience for me…Because it was there and it reminded me, when I looked at it, to take my pill” (P40). Others commented that they felt the reminders from study staff or the awareness of being monitored contributed positively to their adherence. Among those who did not find the bottle to be convenient, a common complaint was that it was an extra, unnecessary step: “I had to be a little extra diligent because I um, I have two pill boxes and usually my pills are right in there with my daily pills, so it was like I had a third schedule that I had to keep track of” (P94).
Some participants modified this additional step by continuing their prior routine (eg, weekly pill organizer), but also opening the smart pill bottle lid: “When I took my Coumadin, I took the lid off…Counted to 3. Put the lid back on” (P101). Although this allowed these participants to maintain their routine while also contributing adherence data, they reported sometimes forgetting to open the lid or being away from the bottle when taking their medication. This method was also used to mitigate the inconvenience of storing half and whole tablets in the same bottle when patients’ dosing routine required both. Compared to a weekly pill organizer, patients felt it was difficult to locate and retrieve the half tablets, as well as remember which size tablet was due.
Possible misinterpretation of missed doses
Many patients expressed surprise or disbelief when initially presented with the number of missed doses. Though some eventually agreed or presented verifiable explanations, some felt adamantly the data were inaccurate, without suggestions for where the discrepancy originated. “There ain’t no way I missed that many pills. I don’t think I missed any” (P67). Others were more unsure, but still did not feel that their adherence was as poor as the data indicated: “Mmm, I don’t know, I guess I don’t think I missed that many. I might have missed one or two” (P39). Others reported technical issues with the bottle (“I know there was times that the light didn’t come on when I opened and closed it” [P12]). Some conjectured that their data were tracked incorrectly due to a change in dosage frequency: “They switched my medication and I thought I had told her that…So I’m sure lots of those days are because they thought I was supposed to take it twice a day” (P19). As was investigated in our analysis regarding discarded doses, some suggested that inaccuracies may have occurred due to technicalities regarding timing (too close together or far apart) or time of day (eg, taking an evening pill after midnight).
Features of the physical bottle
Some participants found the bottle easy to use and/or effective for its intended purpose—for example, P98, who “looked at the bottle as a fun project.” However, other participants frequently noted that its size and bulkiness was inconsistent with their existing routine and created issues with storage and travel. P24 stated, “The only thing I found that was inconvenient, when I was traveling or if I was gonna go out at night and I left early and I couldn’t take, you know, I took the pills out of there, you know and put them in my purse… I didn’t want to carry the big thing with me.” Many participants preferred a weekly pill box that allowed them to organize multiple pill sizes or types: “I like my pill box better…just because I take other medication. So I wouldn’t have to have two things, I could just have that” (P7). “And I’ll be honest with ya, if they ever do another study like this, try to come up with a uh, like a weekly container or something” (P27). Another issue of particular concern in an older population was the lid—multiple participants noted that it did not always screw on easily or correctly, and they worried that it would not record accurate dosing because of this: “Well, I’ve got some arthritis…. And especially in the morning it’s very noticeable and sometimes I just don’t think I got it threaded properly” (P92). Patients also offered suggestions for features the bottle has when used in the “real world” (ie, real-time text alerts), as well as features for future improvement, such as an interactive bottle that could be asked questions (like Amazon Alexa or Google Home) or programmed for travel or dosage adjustments.
DISCUSSION
These findings support the oft-cited challenges of accurately documenting medication adherence. Although new devices and technologies have provided novel methodologies by which to triangulate this important data point, each method has pros and cons. In terms of pros, both Surescripts and the smart pill bottle software reported rather high (and similar) adherence rates in our sample of AF patients (90.0% and 88.8%, respectively). These adherence rates are higher than those often reported via interview or survey among chronic illness patients (ie, 50–70%).34,35 In addition, both tools could prompt reminders or provider contact, which might influence adherence behavior. However, both of these technologies had limitations in terms of accuracy and practicability. For example, the Surescripts data were available for only 88% of patients in our sample, which limits its utility in both research in practice. Moreover, it remains a more distal and indirect measure of adherence than the smart pill bottle, which recorded patients’ daily medication activity. The smart pill bottle, however, was also an imperfect measure of adherence. Based on data from other sources, including patient messages and provider notes, the smart pill bottle underestimated patients’ medication adherence rates (90.0% raw vs 93.6% adjusted). Although the smart pill bottle is a more direct measure of medication-taking behavior than retrospective self-reporting or pharmacy refill records, it also does not account for valid “missed” doses where patients are asked to temporarily discontinue their medication or choose to transfer pills to another container. This finding is important, as it emphasizes the need for a feedback loop between patients and providers regarding medication adherence and highlights the potential for the EHR and patient portals to be sources of verification for data gathered from remote technology.
Our feedback loop via the EHR was only somewhat effective for verifying adherence, as the study team was able to reach and receive responses from only a small percentage of participants. Many participants (65.5%) did not respond to messages, a trend that persisted regardless of the number of messages sent (1 to 4+). More troubling, of those nonresponders, a sizeable minority (33.3%) did not read all messages. This could imply a lack of commitment to medication adherence or a lack of engagement with the communication medium (or a number of other motivational factors). With regard to limitations of the medium, it required participants to access messages within EHR, which although we gave training on the system, lack of familiarity with the system or lack of Internet access may have contributed to low response rates. Furthermore, our EHR-based method of alerting patients to missed doses or refills (ie, receiving the pill bottle and Surescripts data through external software and data pulls and then messaging patients) was laborious and time-lagged. This intensive surveillance monitoring from a clinical team is not practicable in the long term as it is cost- and time-intensive. Instead, an integrated platform (ie, automated messages triggered by having both types of software integrated into the EHR) or a fully functional smart pill bottle could have provided more immediate feedback. Interestingly, many patients suggested that features already present when the smart pill bottle is used as designed (eg, receiving missed dose alerts via text message) would help them see alerts within an actionable timeframe because the alert would be immediate and delivered via technology they already use daily (ie, a cell phone). Furthermore, if the patients themselves have access to these integrated dashboards, they could update the software on missed doses, medication changes, and other exceptions to medication schedules, which may help to increase personal agency and adherence rates overall. In short, as it exists currently, the patient EHR portal has shortcomings as a medication adherence feedback tool; however, there is promise for medication adherence technologies that are designed to be more accessible, transparent, and user-friendly. Moreover, as these technologies move toward integration into the EHR, it will be important for researchers and clinicians to understand that each technology might capture a slightly different view of the medication adherence picture.
In addition to accuracy concerns when using technology to measure medication adherence, the pill bottle itself posed some challenges to users. These challenges, emerging from interview data, include disruptions to existing medication routines and design shortcomings. Like many AF patients, participants in our study often took medications in addition to their OAC and used a pill organizer to manage this. Some patients found it fairly easy to integrate the smart pill bottle into this routine (ie, keeping it in the same location as their pill organizer), whereas others felt it was an unnecessary added step or that the bottle was too large or bulky to be convenient. In addition, some patients’ routines involved taking half tablets at times, and this was difficult to execute and track with just one bottle. Finally, despite the manufacturer of the smart pill bottle stating that the average user age is 70,36 some patients found the bottle difficult to use, noting that they sometimes had trouble with the physical motion of removing or replacing the lid, and expressing worry that this may affect accurate recording of their adherence. This issue could be mitigated with re-designs that consider ease of use among elderly patients or more upfront education (by clinical or study staff) on proper use. For current research and design efforts, it is critical for stakeholders to consider how personal and patient ergonomic factors might affect their adoption and use of smart medication technologies. Before technologies are dispersed to the general public, it is important to conduct pilot studies on their actual long-term use, measuring how that technology fits into patients’ current routines, how adaptable it is for exceptional circumstances (eg, travel, existing weekly pillbox, changes in medication regiment, etc.), and how practicable the device is for general usage.
Overall, our study suggests that triangulating data from different sources, including a patient feedback loop, is critical for gathering accurate data on medication adherence. This is important for both research and practice, providing a method for increasing methodological rigor and effective communication between patients and providers. Meanwhile, our interview data suggest that more research into the daily integration of smart pill bottles in the routines of chronically ill patients is necessary. Specifically, future research should explore how to seamlessly integrate monitoring and alert technology into the external memory aids patients are already using in their daily lives (eg, pill sorters and mobile phones). Technologies external to these working systems may be seen as additional burdens to those already battling a chronic illness.
Limitations
Our study does have limitations that need mention. First, although we reported “adherence rates,” the Surescripts and smart pill bottle data do not necessarily reflect true adherence rates, as we cannot be sure that the patients were actually ingesting the medication that they filled or removed from the bottle. Similarly, our method of validating “allowed misses” relied on patient self-report and doctors; notes, which may not have been accurate. However, we cross-checked all provider-involved explanations with EHR documentation and/or ATU clinic records, and for explanations that involved only the patient (ie, traveling with only one pill container for all medications), we elicited additional feedback through patient portal messages and during the exit interview. This method, of course, is not foolproof, as there is no way to guarantee patients actually took their OAC during these self-reported routine changes. Conversely, to ignore these explanations of missed doses would be willfully disregarding patient feedback regarding their own medication practices. Regarding this patient feedback, we did not directly address the value of the messaging to the patients in our exit interviews. Although they provided comments on the value of the messaging, we missed an opportunity to explore why participants did not respond to messages. In addition, we did not explore in interviews how personal factors (eg, patients’ desire to limit medication consumption) or patient ergonomic factors in the home environment might influence medication adherence. As both personal and ergonomic factors might influence future designs of digital technologies, these are valuable avenues for future research. With regard to our sample, we were unable to include patients taking all available OACs, such as Pradaxa® (dabigatran), and while our sample was fairly representative of the rural and suburban geographic area from which participants were recruited, it was ethnically homogenous. Also, all patients enrolled in this study needed to have access to the computer and Internet to be enrolled in the study. This may have led to a selection bias whereby those who were most ill (and perhaps least adherent to medications) may not have been included in the study.37 These results need to be interpreted with these limitations in mind.
CONCLUSION
With increased availability of smaller and inexpensive sensor technology and the integration of e-prescribing software into the EHR, several options exist to enable remote tracking of patients’ medication adherence, which can help to prevent serious health risks and increased health care costs resulting from missed medication. However, as our study showed, remote monitoring is not a panacea for either tracking medication adherence accurately or for prompting behavior change. As medication adherence is crucial for long-term wellness and survival of those with chronic illnesses, a multipronged approach, including a patient feedback loop regarding adherence and the integration of technologies into daily lives, appears critical.
Funding
This work was supported by Janssen Pharmaceuticals protocol number [39039039AFL4007].
Author Contributions
T.T. and M.J.M. helped to conceptualize, design, and conduct this study. M.D., J.A.P., M.F., R.A., S.W., and A.C. collected, analyzed, and/or interpreted the data and wrote the manuscript with input from all authors. T.T. was in charge of overall direction and planning of the project. In addition, all authors drafted or revised this manuscript critically for important intellectual content, approved the version to be published, and agree to be accountable for all aspects of the work in ensuring that questions related to the accuracy or integrity of any part of the work are appropriately investigated and resolved.
ACKNOWLEDGMENTS
This work was funded by a grant from Janssen Scientific Affairs, LLC. We thank all the patients who participated in this research.
CONFLICT OF INTEREST STATEMENT
Dr. M.J.M. has research funding from the agency for healthcare research and quality (AHRQ) and the following financial relationships with industry to disclose: research grants from Biotronik, Inc., Medtronic, Inc., and Janssen Scientific Affairs, LLC; consulting fees/honoraria with McKesson Corporation, iRhythm Technologies, Inc., and Zoll Medical Corporation; and financial partnership with Medical Informatics Engineering, outside the submitted work. Dr. M.J.M.’s relationships with academia include serving as trustee of Indiana University and on the Indiana University Health Board. Dr. T.T. has research funding from the agency for health care research and quality (AHRQ) and the following financial relationships with industry to disclose: research grants from Biotronik, Inc., Medtronic, Inc., and Janssen Scientific Affairs, LLC; and iRhythm Technologies Inc. outside the submitted work.
REFERENCES
Surescripts. Naitonal Progress Report. Natl. Prog. Rep.