-
PDF
- Split View
-
Views
-
Cite
Cite
Jinzhao Chen, Gaoyu Wu, Andrew Michelson, Zachary Vesoulis, Jennifer Bogner, John D Corrigan, Philip R O Payne, Fuhai Li, Mining reported adverse events induced by potential opioid-drug interactions, JAMIA Open, Volume 3, Issue 1, April 2020, Pages 104–112, https://doi.org/10.1093/jamiaopen/ooz073
- Share Icon Share
Abstract
Opioid-based analgesia is routinely used in clinical practice for the management of pain and alleviation of suffering at the end of life. It is well-known that opioid-based medications can be highly addictive, promoting not only abuse but also life-threatening overdoses. The scope of opioid-related adverse events (AEs) beyond these well-known effects remains poorly described. This exploratory analysis investigates potential AEs from drug-drug interactions between opioid and nonopioid medications (ODIs).
In this study, we conduct an initial exploration of the association between ODIs and severe AEs using millions of AE reports available in FDA Adverse Event Reporting System (FAERS). The odds ratio (OR)-based analysis and visualization are proposed for single drugs and pairwise ODIs to identify associations between AEs and ODIs of interest. Moreover, the multilabel (multi-AE) learning models are employed to evaluate the feasibility of AE prediction of polypharmacy.
The top 12 most prescribed opioids in the FAERS are identified. The OR-based analysis identifies a diverse set of AEs associated with individual opioids. Moreover, the results indicate many ODIs can increase the risk of severe AEs dramatically. The area under the curve values of multilabel learning models of ODIs for oxycodone varied between 0.81 and 0.88 for 5 severe AEs.
The proposed data analysis and visualization are useful for mining FAERS data to identify novel polypharmacy associated AEs, as shown for ODIs. This approach was successful in recapitulating known drug interactions and also identified new opioid-specific AEs that could impact prescribing practices.
INTRODUCTION
Adverse events (AEs) are unexpected and potentially injurious side effects and harms which occur during typical usage of a medication.1 In the United States, approximately 3–7% of hospitalizations are caused by AEs, 10–20% of hospitalizations include AEs, and 10–20% of these AEs are severe.2 Opioids is a generic term for a class of medications which activate central and peripheral opioid receptors, producing analgesia and other physiological effects, such as decreased heart and respiratory rates,3,4 which also can promote addiction leading to potential overdose and death. In 2016, there were approximately 42 000 deaths involving opioids in the United States, which represents a 21.4% increase from 2015.5 Additional well-known AEs associated with opioids include nausea, vomiting, dizziness, and constipation.6
In practice, opioids are often coprescribed for patients with a complicated medical history who are concurrently taking a wide array of additional medications.7 Beyond the aforementioned well-described AEs; however, little is known about the potential for drug-drug interactions between opioid and nonopioid medications. As a result, opioids are often coprescribed with limited information on opioid-drug interactions (ODIs).
The Food and Drug Administration's (FDA) Adverse Event Reporting System (FAERS)8 is a database that contains reported AEs and medication errors involving FDA-approved drugs. The AE reports in FAERS previously have been mined previously to identify potential AEs for specific drugs.9–11 Using FAERS data, some common severe AEs, such cardiovascular incidents, abuse, death, and overdose have been associated with pain-killers, such as nonsteroidal anti-inflammatory drugs (NSAIDs), acetaminophen, and opioids.12
Despite widespread use of opioids and the significant burden of opioid-related adverse outcomes, to the best of our knowledge; however, the linkage of AEs and ODIs have not been well investigated. It is common for opioids to be prescribed to patients taking other medications (polypharmacy), often in the setting of complex medical conditions (eg, traumatic brain injury7) but medical providers lack general safety parameters to guide decision making when combining opioids with other medications. In this preliminary study, we explore the association between ODIs and severe AEs using AE reports in the FAERS database. Using the odds ratio (OR) analysis of single and pairwise ODIs, we identify a set of opioid-specific ODIs that are highly associated with the risk for a set of severe AEs. We also implement a novel visualization method to show the ODIs associated with increased risk of individual AEs. Lastly, we applied multilabel machine learning models to evaluate the feasibility of predicting the association of a specific AE for the use of multiple medicine including opioids to improve the safety of medical treatment. The rest of the paper is organized as follows. First, we identify the mostly commonly reported opioids in the FAERS database along with their reporting frequency. We then introduce the approaches for data preprocessing and AE identification for the most commonly prescribed opioids, as well as the methods employed for the OR analysis of both single-drug and pairwise ODIs, as well as the evaluation of multilabel learning models to predict the AEs of polypharmacy. In the Results section, we provide the top 20 associated AEs for the top 5 reported opioids, the list and visualization of top-ranked ODIs are associated with each of 18 selected AEs for oxycodone and hydrocodone, as well as AE prediction evaluation using 3 multilabel learning models. The article concludes with the Discussion and Conclusion sections.
MATERIALS AND METHODS
Opioids
In this preliminary study, we conducted our analysis on the top 12 opioids reported in the FAERS database, which is an expanded list of the most commonly prescribed opioids according to the National Institute on Drug Abuse (Table 1).6,7,13
. | Opioid . | Frequency . | % . |
---|---|---|---|
1 | Oxycodone | 91 073 | 2.25 |
2 | Hydrocodone | 80 163 | 1.98 |
3 | Morphine | 78 320 | 1.93 |
4 | Fentanyl | 75 844 | 1.87 |
5 | Codeine | 51 308 | 1.27 |
6 | Methadone | 27 236 | 0.67 |
7 | Hydromorphone | 15 971 | 0.39 |
8 | Buprenorphine | 8024 | 0.20 |
9 | Heroin | 5084 | 0.13 |
10 | Dihydrocodeine | 3755 | 0.09 |
11 | Tapentadol | 1287 | 0.03 |
12 | Alfentanil | 663 | 0.02 |
. | Opioid . | Frequency . | % . |
---|---|---|---|
1 | Oxycodone | 91 073 | 2.25 |
2 | Hydrocodone | 80 163 | 1.98 |
3 | Morphine | 78 320 | 1.93 |
4 | Fentanyl | 75 844 | 1.87 |
5 | Codeine | 51 308 | 1.27 |
6 | Methadone | 27 236 | 0.67 |
7 | Hydromorphone | 15 971 | 0.39 |
8 | Buprenorphine | 8024 | 0.20 |
9 | Heroin | 5084 | 0.13 |
10 | Dihydrocodeine | 3755 | 0.09 |
11 | Tapentadol | 1287 | 0.03 |
12 | Alfentanil | 663 | 0.02 |
Abbreviations: FAERS: FDA Adverse Event Reporting System.
. | Opioid . | Frequency . | % . |
---|---|---|---|
1 | Oxycodone | 91 073 | 2.25 |
2 | Hydrocodone | 80 163 | 1.98 |
3 | Morphine | 78 320 | 1.93 |
4 | Fentanyl | 75 844 | 1.87 |
5 | Codeine | 51 308 | 1.27 |
6 | Methadone | 27 236 | 0.67 |
7 | Hydromorphone | 15 971 | 0.39 |
8 | Buprenorphine | 8024 | 0.20 |
9 | Heroin | 5084 | 0.13 |
10 | Dihydrocodeine | 3755 | 0.09 |
11 | Tapentadol | 1287 | 0.03 |
12 | Alfentanil | 663 | 0.02 |
. | Opioid . | Frequency . | % . |
---|---|---|---|
1 | Oxycodone | 91 073 | 2.25 |
2 | Hydrocodone | 80 163 | 1.98 |
3 | Morphine | 78 320 | 1.93 |
4 | Fentanyl | 75 844 | 1.87 |
5 | Codeine | 51 308 | 1.27 |
6 | Methadone | 27 236 | 0.67 |
7 | Hydromorphone | 15 971 | 0.39 |
8 | Buprenorphine | 8024 | 0.20 |
9 | Heroin | 5084 | 0.13 |
10 | Dihydrocodeine | 3755 | 0.09 |
11 | Tapentadol | 1287 | 0.03 |
12 | Alfentanil | 663 | 0.02 |
Abbreviations: FAERS: FDA Adverse Event Reporting System.
AE reports in the FAERS database and preprocessing
To study the AEs of opioids induced by ODIs, we collected AE reports from the FAERS database reported over the past 14 years, from October 1, 2003 through September 30, 2017. AEs are defined as the Medical Dictionary for Regulatory Activities (MedDRA).14 MedDRA is a medical terminology dictionary and is the dictionary of AE classification. In total, there were 9 805 596 case records reported with at least one of AE by this criterion. In this study, we limited our scope to FDA-approved small molecular drugs. A list of 2521 FDA-approved small molecule opioids was retrieved from DrugBank (version 5.1.0).15 After comparing drug names in the FAERS database with FDA-approved drugs, we removed 5 756 511 AEs (58.7%) that did not contain any drug in the FDA-approved list. In addition, we limited our interest to drugs reported at least 1000 times with an AE proportion of no less than 0.5% in the FAERS database. In the end, we collected 4 094 084 AE reports associated with 774 drugs and 151 AEs. The procedure of data preprocessing is demonstrated in Figure 1.

Data preprocessing scheme. Data were extracted from the FDA Adverse Event Reporting System (FAERS) from reports dated October 1, 2003 through September 31, 2017. The list of FDA-approved drugs was retrieved from DrugBank database on May 22, 2018 (version 5.1.0; released on 2018-04-02). N denotes the number of reports in FAERS, Q indicates the number of adverse effects (AEs), and P represents the number of drugs co-prescribed with opioids.
OR analysis to identify and visualize associations between ODIs and increased risk of severe AEs
OR is a technique to quantify the risk of an event with data presented in a 2-by-2 contingency table (see Figure 2). In the contingency table, both the column variable (AE of interest) and the row variable (drug of interest) are binary: present (+) or absent (−). The co-occurrence of any drug-AE pair is categorized into 4 conditions: “–,” “−+,” “+−,” and “++”. Then, we convert the FAERS data into a series of 2-by-2 contingency tables with populated numbers of counts: , , , and . The OR is calculated as .
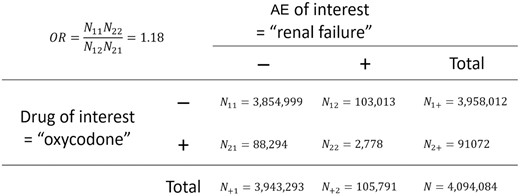
Example of 2-by-2 contingency table for computing the odds ratio of a drug (i.e., oxycodone) for inducing an adverse effect (AE) (e.g., renal failure).
For each AE, we calculated all the ORs of all individual drugs, denoted as Drug.OR for nonopioid drugs and Opioid.OR for individual opioids, and calculated the ORs of all pairwise ODIs (with one opioid drug colisted with another nonopioid drug), denoted as Pair.OR. For example, if oxycodone is colisted with 3 other nonopioid drugs in an AE report, 3 different Pair.OR’s would be calculated, one for each opioid-nonopioid combination. Then, the Fisher’s exact test was performed to confirm the ORs of specific AEs of given ODIs. In order to control the experiment-wise false alarm rate at 0.05, a Bonferroni correction was applied.
To facilitate the visualization of ODIs with increased risk of specific AEs, we implemented a new type of visualization plot (see Figures 3–5). As can be seen in the upper panels of Figures 3–5, the x-axis and y-axis are the relative OR for both ODIs, Pair.OR/Opioid.OR, and relative OR of drugs, Drug.OR/Opioid.OR. Thus, opioid-specific ODIs (eg, oxycodone [opioid]-anagrelide[drug] interaction, represented by “anagrelide”) that are above the solid-bold line are associated with an increased risk of severe AEs (eg, renal failure). ODIs with more than a 1.5-fold Opioid.OR and a P value < .05, ODIs with an Opioid.OR ≥ 0.75 and ≤ 1.5 and a P value ≥ .05, and ODIs with an Opioid.OR < 0.75 and a P value < .05 are labeled as “+,” “o,” and “Δ” symbols, respectively. Moreover, the OR density plots (lower panel) indicate the odd ratios of the specified AEs are increased by interacting with the opioid. In other words, the Pair.OR (ORs of DOIs) has a heavier tailed distribution compared with Drug.OR (ORs of single drugs). The OR of the opioid is also plotted. In addition, names of the top 10 ranked drugs interacting with the given opioid are displayed. The ODI plots can be updated conveniently by changing specific parameters.
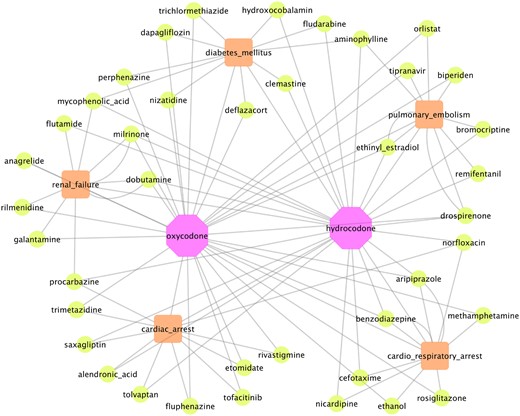
Visualization of top 5 ODIs (green nodes) for oxycodone and hydrocodone (purple nodes), respectively, causing increased risk of 5 selected example adverse events (orange nodes) (cardiorespiratory arrest, cardiac arrest, renal failure, diabetes mellitus, and pulmonary embolism). Abbreviation: ODIs: opioid-drug interactions.
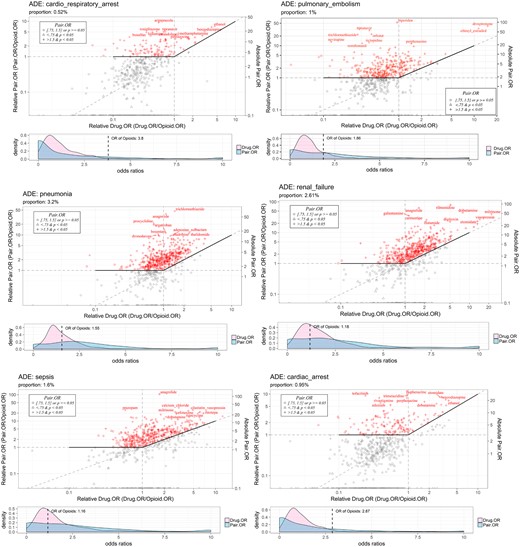
Visualization of top-ranked oxycodone-drug interactions causing increased risk of adverse events. Abbreviations: ADE: •••; OR: odds ratio.
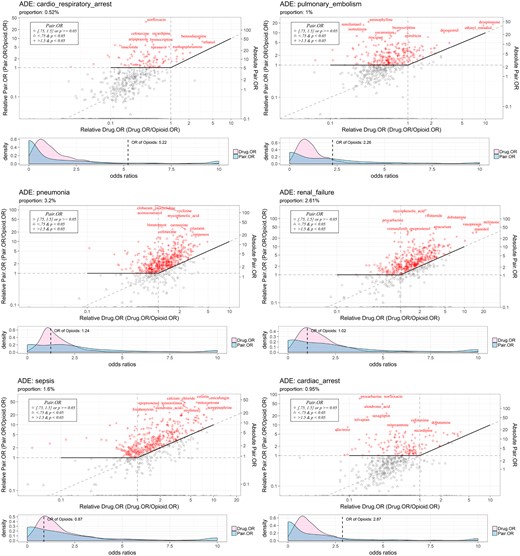
Visualization of top-ranked hydrocodone-drug interactions causing increased risk of adverse events. Abbreviations: ADE: •••; OR: odds ratio.
AE prediction of polypharmacy using multilabel learning models
In addition to the identification of pairwise ODIs using the OR analysis and visualization, we further evaluated the feasibility of predicting ODI AEs containing multiple drugs and oxycodone (the most reported opioid in FAERS).
Problem formulation
Let be the number of AE reports (n = 91 073), be the number of total drugs involved in all reports (P = 774), and represent types of AE. For example, in this preliminary study, 5 type of AEs are selected, that is, renal failure, pulmonary embolism, cardiac arrest, cardiorespiratory arrest, and pneumonia. Mathematically, let be the vector of individual reports, where and , indicate if a drug included in a report (1) or not (0). Let be the AEs, where and , , which indicates if a report is associated with a given AE (1) or not (0). Then the matrix, will be used as the input and will be used as the output (AEs labels) of the multi-AE prediction problem.
Three multilabel prediction models
In this study, 3 supervised learning models are employed, that is, the binary relevance method using logistic regression as the base learner (BR.lr),16 the classifier chains method using regression as the base learner (CC.lr),17,18 and the multivariate classification and regression random forest model (RFSRC).19 The “mlr”20 R package was used to call the BR.lr and CC.lr models, and the “randomforestSRC”21 R package was used to call the RFSRC model. We evaluate the prediction models by dividing the dataset using 5-fold cross-validation, that is, the dataset is randomly divided into 5 folds, and 4 folds (80% of the data) are used as training data, and the rest 20% are used as the testing data. The average of the following metrics is used to evaluate the performance of the models.
Prediction evaluation metrics
For interpretation purposes, hamming loss is defined as the fraction of the wrongly predicted AE labels (0 or 1) to the total number of AE labels (a report might be associated with multiple AEs). Subset 0/1 loss is defined as the fraction of reports that have at least one AE label predicted wrongly. Accuracy is defined as the fraction of correctly predicted AE labels to the total number of AE labels. Precision is defined as the fraction of true positive and also predicted positive AE labels to the predicted positive AE labels. F1 score is defined as an average of the fraction of true positive and also predicted positive AE labels to the predicted positive AE labels, and the fraction of true positive and also predicted positive AE labels to the true positive AE labels.
RESULTS
Top 20 AEs for the 5 most commonly reported opioids
Table 2 shows the top 20 AEs associated with the top 5 reported opioid drugs in FAERS. Not surprisingly, the top 20 AEs for the individual opioid drugs are different but encompass many of the well-known, opioid AEs, such as abuse, overdose, and vomiting. Aside from the expected AEs associated with opioids, each medication has a unique subset of additional AEs, for instance, epistaxis was more commonly reported with codeine than the other opioids. It suggests that opioid-specific ODIs and associated AEs should be investigated separately.
Rank . | Oxycodone . | Hydrocodone . | Morphine . | Fentanyl . | Codeine . |
---|---|---|---|---|---|
1 | Drug abuse | Completed suicide | Drug hypersensitivity | Drug abuse | Drug hypersensitivity |
2 | Cardiorespiratory arrest | Drug abuse | Hypersensitivity | Product quality issue | Drug abuse |
3 | Cardiac arrest | Cardiorespiratory arrest | Lethargy | Toxicity to various agents | Hypersensitivity |
4 | Constipation | Back pain | Toxicity to various agents | Emotional distress | Overdose |
5 | Completed suicide | Gastroesophageal reflux disease | Drug abuse | Drug effect decreased | Toxicity to various agents |
6 | Sinusitis | Anxiety | Cardiac arrest | Cardiac arrest | Hallucination |
7 | Toxicity to various agents | Emotional distress | Somnolence | Overdose | Emotional distress |
8 | Back pain | Sinusitis | Overdose | Hyperhidrosis | Bronchitis |
9 | Dehydration | Cardiac arrest | Respiratory failure | Back pain | Completed suicide |
10 | Neuropathy peripheral | Pulmonary embolism | Hallucination | Depression | Deep vein thrombosis |
11 | Disease progression | Amnesia | General physical health deterioration | Drug ineffective | Epistaxis |
12 | Pulmonary embolism | Depression | Back pain | Constipation | Joint swelling |
13 | Injury | Diabetes mellitus | Pulmonary embolism | Respiratory failure | Sinusitis |
14 | Decreased appetite | Cardiac failure congestive | Death | Amnesia | Dysphagia |
15 | Pleural effusion | Arthralgia | Completed suicide | Injury | Drug interaction |
16 | Abdominal pain | Deep vein thrombosis | Disease progression | Renal impairment | Rheumatoid arthritis |
17 | Depression | Neuropathy peripheral | Constipation | Renal failure | Hyperhidrosis |
18 | Hypokalemia | Hypoesthesia | Depression | Loss of consciousness | Cerebrovascular accident |
19 | Emotional distress | Weight increased | Vomiting | Cardiorespiratory arrest | Thrombosis |
20 | Memory impairment | Gait disturbance | Loss of consciousness | Urinary tract infection | Cardiorespiratory arrest |
Rank . | Oxycodone . | Hydrocodone . | Morphine . | Fentanyl . | Codeine . |
---|---|---|---|---|---|
1 | Drug abuse | Completed suicide | Drug hypersensitivity | Drug abuse | Drug hypersensitivity |
2 | Cardiorespiratory arrest | Drug abuse | Hypersensitivity | Product quality issue | Drug abuse |
3 | Cardiac arrest | Cardiorespiratory arrest | Lethargy | Toxicity to various agents | Hypersensitivity |
4 | Constipation | Back pain | Toxicity to various agents | Emotional distress | Overdose |
5 | Completed suicide | Gastroesophageal reflux disease | Drug abuse | Drug effect decreased | Toxicity to various agents |
6 | Sinusitis | Anxiety | Cardiac arrest | Cardiac arrest | Hallucination |
7 | Toxicity to various agents | Emotional distress | Somnolence | Overdose | Emotional distress |
8 | Back pain | Sinusitis | Overdose | Hyperhidrosis | Bronchitis |
9 | Dehydration | Cardiac arrest | Respiratory failure | Back pain | Completed suicide |
10 | Neuropathy peripheral | Pulmonary embolism | Hallucination | Depression | Deep vein thrombosis |
11 | Disease progression | Amnesia | General physical health deterioration | Drug ineffective | Epistaxis |
12 | Pulmonary embolism | Depression | Back pain | Constipation | Joint swelling |
13 | Injury | Diabetes mellitus | Pulmonary embolism | Respiratory failure | Sinusitis |
14 | Decreased appetite | Cardiac failure congestive | Death | Amnesia | Dysphagia |
15 | Pleural effusion | Arthralgia | Completed suicide | Injury | Drug interaction |
16 | Abdominal pain | Deep vein thrombosis | Disease progression | Renal impairment | Rheumatoid arthritis |
17 | Depression | Neuropathy peripheral | Constipation | Renal failure | Hyperhidrosis |
18 | Hypokalemia | Hypoesthesia | Depression | Loss of consciousness | Cerebrovascular accident |
19 | Emotional distress | Weight increased | Vomiting | Cardiorespiratory arrest | Thrombosis |
20 | Memory impairment | Gait disturbance | Loss of consciousness | Urinary tract infection | Cardiorespiratory arrest |
Abbreviations: AE: adverse events.
Rank . | Oxycodone . | Hydrocodone . | Morphine . | Fentanyl . | Codeine . |
---|---|---|---|---|---|
1 | Drug abuse | Completed suicide | Drug hypersensitivity | Drug abuse | Drug hypersensitivity |
2 | Cardiorespiratory arrest | Drug abuse | Hypersensitivity | Product quality issue | Drug abuse |
3 | Cardiac arrest | Cardiorespiratory arrest | Lethargy | Toxicity to various agents | Hypersensitivity |
4 | Constipation | Back pain | Toxicity to various agents | Emotional distress | Overdose |
5 | Completed suicide | Gastroesophageal reflux disease | Drug abuse | Drug effect decreased | Toxicity to various agents |
6 | Sinusitis | Anxiety | Cardiac arrest | Cardiac arrest | Hallucination |
7 | Toxicity to various agents | Emotional distress | Somnolence | Overdose | Emotional distress |
8 | Back pain | Sinusitis | Overdose | Hyperhidrosis | Bronchitis |
9 | Dehydration | Cardiac arrest | Respiratory failure | Back pain | Completed suicide |
10 | Neuropathy peripheral | Pulmonary embolism | Hallucination | Depression | Deep vein thrombosis |
11 | Disease progression | Amnesia | General physical health deterioration | Drug ineffective | Epistaxis |
12 | Pulmonary embolism | Depression | Back pain | Constipation | Joint swelling |
13 | Injury | Diabetes mellitus | Pulmonary embolism | Respiratory failure | Sinusitis |
14 | Decreased appetite | Cardiac failure congestive | Death | Amnesia | Dysphagia |
15 | Pleural effusion | Arthralgia | Completed suicide | Injury | Drug interaction |
16 | Abdominal pain | Deep vein thrombosis | Disease progression | Renal impairment | Rheumatoid arthritis |
17 | Depression | Neuropathy peripheral | Constipation | Renal failure | Hyperhidrosis |
18 | Hypokalemia | Hypoesthesia | Depression | Loss of consciousness | Cerebrovascular accident |
19 | Emotional distress | Weight increased | Vomiting | Cardiorespiratory arrest | Thrombosis |
20 | Memory impairment | Gait disturbance | Loss of consciousness | Urinary tract infection | Cardiorespiratory arrest |
Rank . | Oxycodone . | Hydrocodone . | Morphine . | Fentanyl . | Codeine . |
---|---|---|---|---|---|
1 | Drug abuse | Completed suicide | Drug hypersensitivity | Drug abuse | Drug hypersensitivity |
2 | Cardiorespiratory arrest | Drug abuse | Hypersensitivity | Product quality issue | Drug abuse |
3 | Cardiac arrest | Cardiorespiratory arrest | Lethargy | Toxicity to various agents | Hypersensitivity |
4 | Constipation | Back pain | Toxicity to various agents | Emotional distress | Overdose |
5 | Completed suicide | Gastroesophageal reflux disease | Drug abuse | Drug effect decreased | Toxicity to various agents |
6 | Sinusitis | Anxiety | Cardiac arrest | Cardiac arrest | Hallucination |
7 | Toxicity to various agents | Emotional distress | Somnolence | Overdose | Emotional distress |
8 | Back pain | Sinusitis | Overdose | Hyperhidrosis | Bronchitis |
9 | Dehydration | Cardiac arrest | Respiratory failure | Back pain | Completed suicide |
10 | Neuropathy peripheral | Pulmonary embolism | Hallucination | Depression | Deep vein thrombosis |
11 | Disease progression | Amnesia | General physical health deterioration | Drug ineffective | Epistaxis |
12 | Pulmonary embolism | Depression | Back pain | Constipation | Joint swelling |
13 | Injury | Diabetes mellitus | Pulmonary embolism | Respiratory failure | Sinusitis |
14 | Decreased appetite | Cardiac failure congestive | Death | Amnesia | Dysphagia |
15 | Pleural effusion | Arthralgia | Completed suicide | Injury | Drug interaction |
16 | Abdominal pain | Deep vein thrombosis | Disease progression | Renal impairment | Rheumatoid arthritis |
17 | Depression | Neuropathy peripheral | Constipation | Renal failure | Hyperhidrosis |
18 | Hypokalemia | Hypoesthesia | Depression | Loss of consciousness | Cerebrovascular accident |
19 | Emotional distress | Weight increased | Vomiting | Cardiorespiratory arrest | Thrombosis |
20 | Memory impairment | Gait disturbance | Loss of consciousness | Urinary tract infection | Cardiorespiratory arrest |
Abbreviations: AE: adverse events.
Top-ranked ODIs inducing 18 selected severe AEs
In this pilot study, we empirically chose 18 common and severe AEs for further analysis: death, pneumonia, anemia, hypotension, depression, hypertension, myocardial infarction, renal failure, sepsis, overdose, completed suicide, cardiac arrest, hemorrhage, diabetes mellitus, drug abuse, cardiorespiratory arrest, pulmonary embolism, and thrombosis. Tables 3 and 4 show the top 5 ODIs, based on the pair.OR, associated with an increased risk for the 18 selected severe AEs for opioids for oxycodone and hydrocodone, respectively. As can be seen, the top-ranked ODIs are diverse and heterogenous for different AEs, given the same opioid. In addition, we graphically represent the top 5 ODIs and 5 associated AEs in a network map (see Figure 3), which can clearly and intuitively display the ODI-AE associations. As aforementioned, for given specific AEs, we implemented a new way to visualize the pair.OR of opioid-specific ODIs. In Figures 4 and 5, we show the top-ranked ODIs with 5 severe AEs (renal failure, pulmonary embolism, cardiac arrest, cardiorespiratory arrest, and pneumonia) for oxycodone and hydrocodone, respectively. As shown in the figures, diverse and distinct ODI-AE pairs emerge for each opioid, suggesting potential drug interactions that may necessitate different prescribing practices to prevent specific AEs.
Top 5 oxycodone-drug interactions associated with increased risk of 18 selected severe AEs
. | AEs . | Number of reports . | Number of drugs with pair.OR > 2 . | Top 5 drugs interacting with oxycodone associated with the AEs . |
---|---|---|---|---|
1 | Hemorrhage | 181 606 | 111 | Anagrelide, aztreonam, nizatidine, norethisterone, sulfadiazine |
2 | Pneumonia | 129 489 | 272 | Anagrelide, argatroban, sulbactam, thalidomide, trichlormethiazide |
3 | Death | 128 896 | 38 | Carmustine, cimetidine, digitoxin, methamphetamine, palonosetron |
4 | Anemia | 111 336 | 280 | Anagrelide, flutamide, mitomycin, procarbazine, thalidomide |
5 | Renal failure | 105 791 | 239 | Anagrelide, dobutamine, galantamine, milrinone, rilmenidine |
6 | Depression | 88 941 | 235 | Adenosine, ampicillin, etomidate, tetracycline, trichlormethiazide |
7 | Hypertension | 88 831 | 201 | Methyldopa, mitomycin, nicardipine, raloxifene, trichlormethiazide |
8 | Hypotension | 86 104 | 192 | Calcium chloride, clofarabine, dobutamine, dopamine, trichlormethiazide |
9 | Overdose | 83 635 | 76 | Caffeine, deflazacort, etoricoxib, flurazepam, galantamine |
10 | Thrombosis | 70 395 | 226 | Adenosine, ampicillin, drospirenone, nicardipine, tetracycline |
11 | Myocardial infarction | 70 028 | 75 | Bimatoprost, dobutamine, morniflumate, nicardipine, prasugrel |
12 | Sepsis | 64 938 | 183 | Anagrelide, calcium chloride, cefotaxime, cilastatin, vasopressin |
13 | Diabetes mellitus | 42 013 | 152 | Deflazacort, dobutamine, nizatidine, perphenazine, trichlormethiazide |
14 | Completed suicide | 41 437 | 94 | Aripiprazole, ethanol, milnacipran, phenobarbital, quetiapine |
15 | Pulmonary embolism | 40 521 | 136 | Drospirenone, ethinyl estradiol, perphenazine, tipranavir, trichlormethiazide |
16 | Cardiac arrest | 38 331 | 72 | Benzodiazepine, dobutamine, ethanol, etomidate, perphenazine |
17 | Drug abuse | 26 042 | 77 | Dextromethorphan, doxylamine, methamphetamine, nimesulide, pentazocine |
18 | Cardiorespiratory arrest | 21 056 | 70 | Aripiprazole, benzodiazepine, ethanol, methamphetamine, rosiglitazone |
. | AEs . | Number of reports . | Number of drugs with pair.OR > 2 . | Top 5 drugs interacting with oxycodone associated with the AEs . |
---|---|---|---|---|
1 | Hemorrhage | 181 606 | 111 | Anagrelide, aztreonam, nizatidine, norethisterone, sulfadiazine |
2 | Pneumonia | 129 489 | 272 | Anagrelide, argatroban, sulbactam, thalidomide, trichlormethiazide |
3 | Death | 128 896 | 38 | Carmustine, cimetidine, digitoxin, methamphetamine, palonosetron |
4 | Anemia | 111 336 | 280 | Anagrelide, flutamide, mitomycin, procarbazine, thalidomide |
5 | Renal failure | 105 791 | 239 | Anagrelide, dobutamine, galantamine, milrinone, rilmenidine |
6 | Depression | 88 941 | 235 | Adenosine, ampicillin, etomidate, tetracycline, trichlormethiazide |
7 | Hypertension | 88 831 | 201 | Methyldopa, mitomycin, nicardipine, raloxifene, trichlormethiazide |
8 | Hypotension | 86 104 | 192 | Calcium chloride, clofarabine, dobutamine, dopamine, trichlormethiazide |
9 | Overdose | 83 635 | 76 | Caffeine, deflazacort, etoricoxib, flurazepam, galantamine |
10 | Thrombosis | 70 395 | 226 | Adenosine, ampicillin, drospirenone, nicardipine, tetracycline |
11 | Myocardial infarction | 70 028 | 75 | Bimatoprost, dobutamine, morniflumate, nicardipine, prasugrel |
12 | Sepsis | 64 938 | 183 | Anagrelide, calcium chloride, cefotaxime, cilastatin, vasopressin |
13 | Diabetes mellitus | 42 013 | 152 | Deflazacort, dobutamine, nizatidine, perphenazine, trichlormethiazide |
14 | Completed suicide | 41 437 | 94 | Aripiprazole, ethanol, milnacipran, phenobarbital, quetiapine |
15 | Pulmonary embolism | 40 521 | 136 | Drospirenone, ethinyl estradiol, perphenazine, tipranavir, trichlormethiazide |
16 | Cardiac arrest | 38 331 | 72 | Benzodiazepine, dobutamine, ethanol, etomidate, perphenazine |
17 | Drug abuse | 26 042 | 77 | Dextromethorphan, doxylamine, methamphetamine, nimesulide, pentazocine |
18 | Cardiorespiratory arrest | 21 056 | 70 | Aripiprazole, benzodiazepine, ethanol, methamphetamine, rosiglitazone |
Abbreviations: AE: adverse events; OR: odds ratio.
Top 5 oxycodone-drug interactions associated with increased risk of 18 selected severe AEs
. | AEs . | Number of reports . | Number of drugs with pair.OR > 2 . | Top 5 drugs interacting with oxycodone associated with the AEs . |
---|---|---|---|---|
1 | Hemorrhage | 181 606 | 111 | Anagrelide, aztreonam, nizatidine, norethisterone, sulfadiazine |
2 | Pneumonia | 129 489 | 272 | Anagrelide, argatroban, sulbactam, thalidomide, trichlormethiazide |
3 | Death | 128 896 | 38 | Carmustine, cimetidine, digitoxin, methamphetamine, palonosetron |
4 | Anemia | 111 336 | 280 | Anagrelide, flutamide, mitomycin, procarbazine, thalidomide |
5 | Renal failure | 105 791 | 239 | Anagrelide, dobutamine, galantamine, milrinone, rilmenidine |
6 | Depression | 88 941 | 235 | Adenosine, ampicillin, etomidate, tetracycline, trichlormethiazide |
7 | Hypertension | 88 831 | 201 | Methyldopa, mitomycin, nicardipine, raloxifene, trichlormethiazide |
8 | Hypotension | 86 104 | 192 | Calcium chloride, clofarabine, dobutamine, dopamine, trichlormethiazide |
9 | Overdose | 83 635 | 76 | Caffeine, deflazacort, etoricoxib, flurazepam, galantamine |
10 | Thrombosis | 70 395 | 226 | Adenosine, ampicillin, drospirenone, nicardipine, tetracycline |
11 | Myocardial infarction | 70 028 | 75 | Bimatoprost, dobutamine, morniflumate, nicardipine, prasugrel |
12 | Sepsis | 64 938 | 183 | Anagrelide, calcium chloride, cefotaxime, cilastatin, vasopressin |
13 | Diabetes mellitus | 42 013 | 152 | Deflazacort, dobutamine, nizatidine, perphenazine, trichlormethiazide |
14 | Completed suicide | 41 437 | 94 | Aripiprazole, ethanol, milnacipran, phenobarbital, quetiapine |
15 | Pulmonary embolism | 40 521 | 136 | Drospirenone, ethinyl estradiol, perphenazine, tipranavir, trichlormethiazide |
16 | Cardiac arrest | 38 331 | 72 | Benzodiazepine, dobutamine, ethanol, etomidate, perphenazine |
17 | Drug abuse | 26 042 | 77 | Dextromethorphan, doxylamine, methamphetamine, nimesulide, pentazocine |
18 | Cardiorespiratory arrest | 21 056 | 70 | Aripiprazole, benzodiazepine, ethanol, methamphetamine, rosiglitazone |
. | AEs . | Number of reports . | Number of drugs with pair.OR > 2 . | Top 5 drugs interacting with oxycodone associated with the AEs . |
---|---|---|---|---|
1 | Hemorrhage | 181 606 | 111 | Anagrelide, aztreonam, nizatidine, norethisterone, sulfadiazine |
2 | Pneumonia | 129 489 | 272 | Anagrelide, argatroban, sulbactam, thalidomide, trichlormethiazide |
3 | Death | 128 896 | 38 | Carmustine, cimetidine, digitoxin, methamphetamine, palonosetron |
4 | Anemia | 111 336 | 280 | Anagrelide, flutamide, mitomycin, procarbazine, thalidomide |
5 | Renal failure | 105 791 | 239 | Anagrelide, dobutamine, galantamine, milrinone, rilmenidine |
6 | Depression | 88 941 | 235 | Adenosine, ampicillin, etomidate, tetracycline, trichlormethiazide |
7 | Hypertension | 88 831 | 201 | Methyldopa, mitomycin, nicardipine, raloxifene, trichlormethiazide |
8 | Hypotension | 86 104 | 192 | Calcium chloride, clofarabine, dobutamine, dopamine, trichlormethiazide |
9 | Overdose | 83 635 | 76 | Caffeine, deflazacort, etoricoxib, flurazepam, galantamine |
10 | Thrombosis | 70 395 | 226 | Adenosine, ampicillin, drospirenone, nicardipine, tetracycline |
11 | Myocardial infarction | 70 028 | 75 | Bimatoprost, dobutamine, morniflumate, nicardipine, prasugrel |
12 | Sepsis | 64 938 | 183 | Anagrelide, calcium chloride, cefotaxime, cilastatin, vasopressin |
13 | Diabetes mellitus | 42 013 | 152 | Deflazacort, dobutamine, nizatidine, perphenazine, trichlormethiazide |
14 | Completed suicide | 41 437 | 94 | Aripiprazole, ethanol, milnacipran, phenobarbital, quetiapine |
15 | Pulmonary embolism | 40 521 | 136 | Drospirenone, ethinyl estradiol, perphenazine, tipranavir, trichlormethiazide |
16 | Cardiac arrest | 38 331 | 72 | Benzodiazepine, dobutamine, ethanol, etomidate, perphenazine |
17 | Drug abuse | 26 042 | 77 | Dextromethorphan, doxylamine, methamphetamine, nimesulide, pentazocine |
18 | Cardiorespiratory arrest | 21 056 | 70 | Aripiprazole, benzodiazepine, ethanol, methamphetamine, rosiglitazone |
Abbreviations: AE: adverse events; OR: odds ratio.
Top 5 hydrocodone-drug interactions associated with increased risk of 18 selected AEs
. | AEs . | Number of reports . | Number of drugs with Pair.OR > 2 . | Top 5 drugs interacting with hydrocodone associated with the AEs . |
---|---|---|---|---|
1 | Hemorrhage | 181 606 | 142 | Acenocoumarol, alendronic acid, clofarabine, flutamide, remifentanil |
2 | Pneumonia | 129 489 | 193 | Acenocoumarol, bimatoprost, cilastatin, cyclizine, mycophenolic acid |
3 | Death | 128 896 | 32 | Cefaclor, clozapine, doxylamine, erlotinib, meprobamate |
4 | Anemia | 111 336 | 239 | Flutamide, melphalan, mycophenolic acid, thalidomide, ticlopidine |
5 | Renal failure | 105 791 | 187 | Dobutamine, flutamide, milrinone, mycophenolic acid, vasopressin |
6 | Depression | 88 941 | 266 | Aminophylline, cyclizine, flutamide, goserelin, thrombin |
7 | Hypertension | 88 831 | 231 | Aminophylline, domperidone, flutamide, mitoxantrone, mycophenolic acid |
8 | Hypotension | 86 104 | 150 | Calcium chloride, cilastatin, dobutamine, flutamide, norepinephrine |
9 | Overdose | 83 635 | 35 | Benzodiazepine, caffeine, meprobamate, nicotine, oxazepam |
10 | Thrombosis | 70 395 | 206 | Aminophylline, argatroban, ceftazidime, dacarbazine, mycophenolic acid |
11 | Myocardial infarction | 70 028 | 128 | Acetylcysteine, aminophylline, flutamide, morniflumate, nizatidine |
12 | Sepsis | 64 938 | 117 | Calcium chloride, micafungin, mitoxantrone, norepinephrine, temsirolimus |
13 | Diabetes mellitus | 42 013 | 190 | Aminophylline, fludarabine, fosinopril, mycophenolic acid, orlistat |
14 | Completed suicide | 41 437 | 144 | Desipramine, eszopiclone, ethanol, nicardipine, valproic acid |
15 | Pulmonary embolism | 40 521 | 129 | Bromocriptine, drospirenone, ethinyl estradiol, remifentanil, vecuronium |
16 | Cardiac arrest | 38 331 | 79 | Alendronic acid, dobutamine, mitoxantrone, nicardipine, saxagliptin |
17 | Drug abuse | 26 042 | 59 | Dextromethorphan, doxylamine, methamphetamine, oxymorphone, pentazocine |
18 | Cardiorespiratory arrest | 21 056 | 80 | Aripiprazole, benzodiazepine, bromocriptine, ethanol, nicardipine |
. | AEs . | Number of reports . | Number of drugs with Pair.OR > 2 . | Top 5 drugs interacting with hydrocodone associated with the AEs . |
---|---|---|---|---|
1 | Hemorrhage | 181 606 | 142 | Acenocoumarol, alendronic acid, clofarabine, flutamide, remifentanil |
2 | Pneumonia | 129 489 | 193 | Acenocoumarol, bimatoprost, cilastatin, cyclizine, mycophenolic acid |
3 | Death | 128 896 | 32 | Cefaclor, clozapine, doxylamine, erlotinib, meprobamate |
4 | Anemia | 111 336 | 239 | Flutamide, melphalan, mycophenolic acid, thalidomide, ticlopidine |
5 | Renal failure | 105 791 | 187 | Dobutamine, flutamide, milrinone, mycophenolic acid, vasopressin |
6 | Depression | 88 941 | 266 | Aminophylline, cyclizine, flutamide, goserelin, thrombin |
7 | Hypertension | 88 831 | 231 | Aminophylline, domperidone, flutamide, mitoxantrone, mycophenolic acid |
8 | Hypotension | 86 104 | 150 | Calcium chloride, cilastatin, dobutamine, flutamide, norepinephrine |
9 | Overdose | 83 635 | 35 | Benzodiazepine, caffeine, meprobamate, nicotine, oxazepam |
10 | Thrombosis | 70 395 | 206 | Aminophylline, argatroban, ceftazidime, dacarbazine, mycophenolic acid |
11 | Myocardial infarction | 70 028 | 128 | Acetylcysteine, aminophylline, flutamide, morniflumate, nizatidine |
12 | Sepsis | 64 938 | 117 | Calcium chloride, micafungin, mitoxantrone, norepinephrine, temsirolimus |
13 | Diabetes mellitus | 42 013 | 190 | Aminophylline, fludarabine, fosinopril, mycophenolic acid, orlistat |
14 | Completed suicide | 41 437 | 144 | Desipramine, eszopiclone, ethanol, nicardipine, valproic acid |
15 | Pulmonary embolism | 40 521 | 129 | Bromocriptine, drospirenone, ethinyl estradiol, remifentanil, vecuronium |
16 | Cardiac arrest | 38 331 | 79 | Alendronic acid, dobutamine, mitoxantrone, nicardipine, saxagliptin |
17 | Drug abuse | 26 042 | 59 | Dextromethorphan, doxylamine, methamphetamine, oxymorphone, pentazocine |
18 | Cardiorespiratory arrest | 21 056 | 80 | Aripiprazole, benzodiazepine, bromocriptine, ethanol, nicardipine |
Abbreviations: AE: adverse events; OR: odds ratio.
Top 5 hydrocodone-drug interactions associated with increased risk of 18 selected AEs
. | AEs . | Number of reports . | Number of drugs with Pair.OR > 2 . | Top 5 drugs interacting with hydrocodone associated with the AEs . |
---|---|---|---|---|
1 | Hemorrhage | 181 606 | 142 | Acenocoumarol, alendronic acid, clofarabine, flutamide, remifentanil |
2 | Pneumonia | 129 489 | 193 | Acenocoumarol, bimatoprost, cilastatin, cyclizine, mycophenolic acid |
3 | Death | 128 896 | 32 | Cefaclor, clozapine, doxylamine, erlotinib, meprobamate |
4 | Anemia | 111 336 | 239 | Flutamide, melphalan, mycophenolic acid, thalidomide, ticlopidine |
5 | Renal failure | 105 791 | 187 | Dobutamine, flutamide, milrinone, mycophenolic acid, vasopressin |
6 | Depression | 88 941 | 266 | Aminophylline, cyclizine, flutamide, goserelin, thrombin |
7 | Hypertension | 88 831 | 231 | Aminophylline, domperidone, flutamide, mitoxantrone, mycophenolic acid |
8 | Hypotension | 86 104 | 150 | Calcium chloride, cilastatin, dobutamine, flutamide, norepinephrine |
9 | Overdose | 83 635 | 35 | Benzodiazepine, caffeine, meprobamate, nicotine, oxazepam |
10 | Thrombosis | 70 395 | 206 | Aminophylline, argatroban, ceftazidime, dacarbazine, mycophenolic acid |
11 | Myocardial infarction | 70 028 | 128 | Acetylcysteine, aminophylline, flutamide, morniflumate, nizatidine |
12 | Sepsis | 64 938 | 117 | Calcium chloride, micafungin, mitoxantrone, norepinephrine, temsirolimus |
13 | Diabetes mellitus | 42 013 | 190 | Aminophylline, fludarabine, fosinopril, mycophenolic acid, orlistat |
14 | Completed suicide | 41 437 | 144 | Desipramine, eszopiclone, ethanol, nicardipine, valproic acid |
15 | Pulmonary embolism | 40 521 | 129 | Bromocriptine, drospirenone, ethinyl estradiol, remifentanil, vecuronium |
16 | Cardiac arrest | 38 331 | 79 | Alendronic acid, dobutamine, mitoxantrone, nicardipine, saxagliptin |
17 | Drug abuse | 26 042 | 59 | Dextromethorphan, doxylamine, methamphetamine, oxymorphone, pentazocine |
18 | Cardiorespiratory arrest | 21 056 | 80 | Aripiprazole, benzodiazepine, bromocriptine, ethanol, nicardipine |
. | AEs . | Number of reports . | Number of drugs with Pair.OR > 2 . | Top 5 drugs interacting with hydrocodone associated with the AEs . |
---|---|---|---|---|
1 | Hemorrhage | 181 606 | 142 | Acenocoumarol, alendronic acid, clofarabine, flutamide, remifentanil |
2 | Pneumonia | 129 489 | 193 | Acenocoumarol, bimatoprost, cilastatin, cyclizine, mycophenolic acid |
3 | Death | 128 896 | 32 | Cefaclor, clozapine, doxylamine, erlotinib, meprobamate |
4 | Anemia | 111 336 | 239 | Flutamide, melphalan, mycophenolic acid, thalidomide, ticlopidine |
5 | Renal failure | 105 791 | 187 | Dobutamine, flutamide, milrinone, mycophenolic acid, vasopressin |
6 | Depression | 88 941 | 266 | Aminophylline, cyclizine, flutamide, goserelin, thrombin |
7 | Hypertension | 88 831 | 231 | Aminophylline, domperidone, flutamide, mitoxantrone, mycophenolic acid |
8 | Hypotension | 86 104 | 150 | Calcium chloride, cilastatin, dobutamine, flutamide, norepinephrine |
9 | Overdose | 83 635 | 35 | Benzodiazepine, caffeine, meprobamate, nicotine, oxazepam |
10 | Thrombosis | 70 395 | 206 | Aminophylline, argatroban, ceftazidime, dacarbazine, mycophenolic acid |
11 | Myocardial infarction | 70 028 | 128 | Acetylcysteine, aminophylline, flutamide, morniflumate, nizatidine |
12 | Sepsis | 64 938 | 117 | Calcium chloride, micafungin, mitoxantrone, norepinephrine, temsirolimus |
13 | Diabetes mellitus | 42 013 | 190 | Aminophylline, fludarabine, fosinopril, mycophenolic acid, orlistat |
14 | Completed suicide | 41 437 | 144 | Desipramine, eszopiclone, ethanol, nicardipine, valproic acid |
15 | Pulmonary embolism | 40 521 | 129 | Bromocriptine, drospirenone, ethinyl estradiol, remifentanil, vecuronium |
16 | Cardiac arrest | 38 331 | 79 | Alendronic acid, dobutamine, mitoxantrone, nicardipine, saxagliptin |
17 | Drug abuse | 26 042 | 59 | Dextromethorphan, doxylamine, methamphetamine, oxymorphone, pentazocine |
18 | Cardiorespiratory arrest | 21 056 | 80 | Aripiprazole, benzodiazepine, bromocriptine, ethanol, nicardipine |
Abbreviations: AE: adverse events; OR: odds ratio.
ODI AE prediction for oxycodone
In addition to the OR-based analysis to identify ODIs and associated AEs, we further demonstrated the feasibility of applying multilabel learning models to predict AEs in patients with multiple reports. We selected 5 AEs of interest (ie, renal failure, pulmonary embolism, cardiac arrest, cardiorespiratory arrest, and pneumonia) that were reported with the presence of oxycodone. Three learning methods have been evaluated (ie, BR.lr: Binary relevance with logistic regression; CC.lr: Classifier Chains with logistic regression; and RFSRC: random forest adapted for multilabel classification). We used 5-fold cross validation to evaluate the model performance based on the aforementioned metrics, that is, hamming loss, subset 0/1 loss, f1 score, accuracy, and precision. Figure 6 (upper panel) shows the average values of these metrics on the 5 selected AEs. In addition, Figure 6 (lower panel) shows the average area under the curve values of the 3 models for the AEs. The results indicate that the random forest SRC model outperformed the other models in all evaluation metrics.
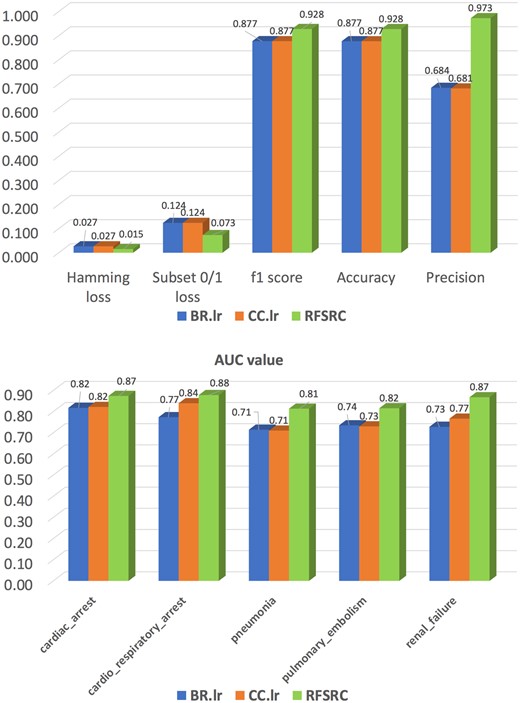
Performance comparison of 3 multilabel classification models. Abbreviations: AUC: area under the curve; RFSRC: regression random forest model.
DISCUSSION AND CONCLUSION
Opioids are a commonly prescribed class of medications and are frequently taken in combination with other medications in the management of patients with acute and chronic pain. Although there is considerable potential for AEs, the associated AEs of ODIs has not been well investigated. Herein, we conducted an initial exploration of ODI-AE associations by mining millions of AE reports in the FAERS database. Using this approach to AE identification, we were able to recapitulate the well-known AEs associated with opioids, including constipation, abuse, and cardiopulmonary arrest. Furthermore, through the pairwise analysis of opioid-associated AEs, we were also able to recreate common, other well-known medication AEs, such as estrogen-induced venous thromboembolism, immunosuppressive-therapy associated risk of pneumonia, and higher cardiopulmonary arrest with benzodiazepine use.22–24 Together, this knowledge recreation lends credence to the methodology used in this exploratory analysis.
Furthermore, the results of this study suggest individual opioids have unique AEs, potentially related to drug-specific off-target effects. For instance, fentanyl was associated with hyperhydrosis while hydrocodone was associated with gastroesophageal reflux disease. It is certainly possible that many of the identified associations are the result of underlying patient comorbidities that are being treated rather than a direct medication effect, but it is also possible that opioids potentiate the AEs reported. To further stratify these associations, we will need to consider disease-specific treatments. For instance, a strong association between hydrocodone, riociguat and pulmonary embolism emerged in our cohort. However, riociguat is a medication used to treat inoperable or persistent postsurgical chronic thromboembolic pulmonary hypertension, and as such, it is unlikely that this association represents a true ODI between hydrocodone and riociguat. By encompassing disease-level treatment bundles into this tool, we can further enrich the results produced by this pipeline.
In addition, since the FAERS database does not contain a patient cohort without the associated AE, the relative prevalence of the AEs and ODIs may be over represented in the OR calculation. By incorporating additional patient characteristics, such as age, gender, and comorbidities, the risk of specific AEs for a given ODI can be more accurately measured. Also, it is not a trivial task for the drug name comparison and normalization to make use of the reports more accurately in FEARS database. Moreover, the OR analysis depends on the properly constructed confusions matrix. It might be biased and not accurate to use all reported AEs to calculate the negative cases for OR calculation. Ideally, people who were given the drug but reported no AEs should be used as the negative. However, these data were not included in FAERS. Therefore, in addition to FAERS data, it is important to integrate more electronic health record (EHR) data and also big datasets of claims and pharmacy to identify more and unbiased negative controls to further evaluate the potential ODIs and associated AEs. In the last section of this study, we also create a process to use the FAERS database to train machine learning models to predict potential AEs for given ODIs. These models could further be enhanced using sophisticated deep learning models to integrate chemical structure features to identify ODIs associated with severe AEs. Once validated, it is our hope that this pipeline could be helpful to facilitate the identification of additional drug-drug interactions to improve safe prescribing practices.
FUNDING
This work was supported by the Institute for Informatics (I2), Department of Pediatrics startup funding, Washington University in St. Louis.
AUTHOR CONTRIBUTIONS
JB, JC, PP, and FL contributed the study idea and design. JC, GW, and FL conducted the data analysis and wrote the article. JC, GW, AM, ZV, and FL revised the manuscript.
ACKNOWLEDGMENTS
The authors would like to thank Dr. Kelley Foyil for the editing of the manuscript.
CONFLICT OF INTEREST STATEMENT
None declared.
REFERENCES
FDA Adverse Event Reporting System. https://open.fda.gov/data/faers/. Accessed June 2018.
Author notes
Jinzhao Chen and Gaoyu Wu contributed equally to this work.