-
PDF
- Split View
-
Views
-
Cite
Cite
Sushanta Deb, Jhinuk Basu, Megha Choudhary, An overview of next generation sequencing strategies and genomics tools used for tuberculosis research, Journal of Applied Microbiology, Volume 135, Issue 7, July 2024, lxae174, https://doi.org/10.1093/jambio/lxae174
- Share Icon Share
Abstract
Tuberculosis (TB) is a grave public health concern and is considered the foremost contributor to human mortality resulting from infectious disease. Due to the stringent clonality and extremely restricted genomic diversity, conventional methods prove inefficient for in-depth exploration of minor genomic variations and the evolutionary dynamics operating in Mycobacterium tuberculosis (M.tb) populations. Until now, the majority of reviews have primarily focused on delineating the application of whole-genome sequencing (WGS) in predicting antibiotic resistant genes, surveillance of drug resistance strains, and M.tb lineage classifications. Despite the growing use of next generation sequencing (NGS) and WGS analysis in TB research, there are limited studies that provide a comprehensive summary of there role in studying macroevolution, minor genetic variations, assessing mixed TB infections, and tracking transmission networks at an individual level. This highlights the need for systematic effort to fully explore the potential of WGS and its associated tools in advancing our understanding of TB epidemiology and disease transmission. We delve into the recent bioinformatics pipelines and NGS strategies that leverage various genetic features and simultaneous exploration of host-pathogen protein expression profile to decipher the genetic heterogeneity and host-pathogen interaction dynamics of the M.tb infections. This review highlights the potential benefits and limitations of NGS and bioinformatics tools and discusses their role in TB detection and epidemiology. Overall, this review could be a valuable resource for researchers and clinicians interested in NGS-based approaches in TB research.
This study highlights how recent advancements in whole-genome sequencing (WGS) and Next Generation Sequencing (NGS) technologies have transformed tuberculosis (TB) research. NGS strategies have accelerated patient-level investigations and the discovery of drug resistance determinants. The integration of tailored bioinformatics pipelines has further enhanced the application of WGS and NGS approaches in TB research, offering valuable insights into genetic heterogeneity, microevolution, and disease transmission events.
Introduction
The advent of whole-genome sequencing (WGS) has facilitated the comprehensive examination of Mycobacterium tuberculosis (M.tb) strains, offered enhanced genetic resolution, and thereby enabled a deeper understanding of resistance mutations, co-infections, patterns of disease dissemination, and the identification of genetic variants. This method is a pivotal tool in tuberculosis (TB) research for understanding the evolution and pathogenicity of TB strains (Meehan et al. 2019). In TB research, the widespread adoption of the WGS approach by scientific community has led to extensive collections of M.tb strains representing various lineages and sublineages of the M.tb complex. High TB burden countries are encountering a shortage of experienced bioinformaticians for routine diagnosis, typing, and resistance monitoring of M.tb strains in healthcare settings (Rivière et al. 2021). This shortage highlights the importance of developing training programmes and freely accessible knowledge hubs focused on WGS and bioinformatics skills for low-income countries with a high burden TB infection (Karikari et al. 2015, Helmy et al. 2016). Recent developments in WGS allow tracing microevolutionary features and heteroresistance in mixed TB populations and provide super-resolution to the heterogeneity of genomic regions (Liang et al. 2020). However, the availability of WGS data varies among nations, with developing countries producing less genomic data than developed nations (Helmy et al. 2016, Rivière et al. 2021). This bias leads to an inadequate representation of M.tb strains from various geographical regions, resulting in inconsistencies in datasets used for epidemiological investigations. The emergence of state-of-the-art next generation sequencing (NGS) technology has substantially reduced the expenses associated with genome sequencing, thus enabling widespread access to M.tb genomes on a global scale. This breakthrough has opened avenues for exploring the distribution of M.tb lineages and monitoring drug-resistant strains across diverse geographical regions (Freschi et al. 2021). Cutting-edge NGS technologies, coupled with sophisticated bioinformatics tools and methodologies, have become an essential for conducting comprehensive analyses of M.tb infections (Meehan et al. 2019). The robustness of the bioinformatics method utilized in clinically relevant prediction tools is crucial, as variations in bioinformatics analyses can potentially impact clinical decisions, such as the selection of a drug regimen for treatment.
These factors highlight the need for development of robust genomic pipeline or algorithm to achieve standardized WGS results when comparing M.tb isolate genomes submitted from diverse global sources. This review aims to explore recent developments in NGS and WGS analysis employed in the investigation of TB infection. It provides a summary of available software and bioinformatics pipelines specifically designed to analyse the Mycobacterium genome. Special attention has been given to the evolving NGS approach and its optimal application in TB research and highlights recently developed NGS tools and techniques specifically designed to analyse mixed TB infections, minor genomic variations, and transmission networks.
RNA sequencing (RNA-Seq)
To date, various NGS strategies have emerged to study infectious diseases, offering comprehensive insights into the biology of host-pathogen systems (Fig. 1). RNA-Seq, an NGS sequencing technique, is employed to quantify M.tb RNA in clinical samples and explore infection mechanisms, offering cost-effective high-throughput data and exhibiting superior specificity and sensitivity compared to alternative gene expression analysis techniques. However, there are technological restrictions when employing this tactic against intracellular pathogens like M.tb. In recent studies, the RNA-seq approach has been utilized to investigate the adaptation of intracellular Mycobacteria (Rienksma et al. 2015), to identify crucial genes involved in the infection mechanism (Cornejo-Granados et al. 2021), and to comprehend the transcriptional regulatory network’s response to environmental factors (Yoo et al. 2022).
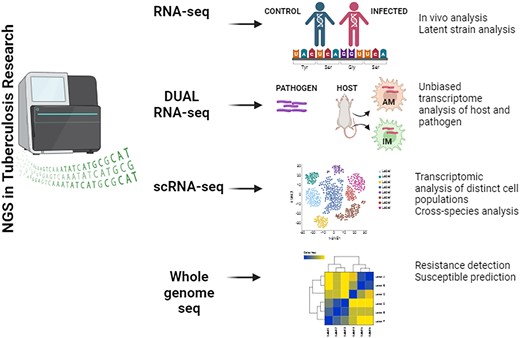
Schematic representation of various NGS method employed to infer M.tb infections.
Cornejo et al. (2021) conducted an RNA-seq study to establish an experimental protocol for testing the gene expression of M.tb and its host during in vivo infection (Cornejo-Granados et al. 2021). This approach is unique as it does not require specialized equipment to examine host–pathogen interactions in TB and can also be applied to explore other similar intracellular infections (Cornejo-Granados et al. 2021). In another study, Estévez et al. (2020) conducted an RNA-seq based investigation in latent TB infected (LTBI) individuals from Spain and Mozambique and identified a gene expression signature associated with TB progression (Estévez et al. 2020). Machine learning revealed heterogeneity within LTBI, with some individuals showing TB-like disease features. They proposed a gene panel for distinguishing between LTBI subgroups, aiding in targeted preventive treatment for those at higher risk of TB progression. This method boosts infection-specific gene detection from 13 to 702, including encoded proteins such as PE-PGRS, lppN, and LpqH lipoproteins and three non-coding RNAs. The study of the M.tb transcriptome can be challenging due to the low amount of Mycobacterial RNA in comparison to the RNA of the host cell. This low quantity of RNA could be attributed to the paucibacillary nature of M.tb infections within host cells relative to other pathogenic bacteria (Repasy et al. 2013).
Dual RNA sequencing (du RNA-seq)
Dual RNA-seq enables simultaneous tracking of gene expression alterations in both microbial and eukaryotic cells within defined conditions, such as pathogen-host interaction (Westermann et al. 2012). In recent times, the popular dual RNA-seq approach has been implemented to elucidate host–pathogen interactions, particularly in studying the response against drug-resistant M.tb infection (López-Agudelo et al. 2022). Pisu et al. 2020aisolated M.tb infected alveolar macrophages (AM) and interstitial macrophages (IM) host cell populations directly from mice lungs and used them for dual RNA-seq where they found enhanced upregulation of iron and fatty acid access in AM compared to IM, which limits M.tb growth by iron sequestration and higher nitric oxide levels (Pisu et al. 2021). An improved RNA extraction procedure and data analysis pipeline have been designed for samples with shallow sequencing depth (Pisu and Russell 2023). However, it has limitations due to distinct RNA features in prokaryotes and eukaryotes (Pisu et al. 2020a). Pisu et al. (2020a) developed a methodology to run dual RNA-seq on populations of M.tb-infected in vivo-derived macrophages where they provided a useful, step-by-step tutorial for carrying out the procedure on M.tb-infected cells from an infection model in mice (Pisu et al. 2020b). The reported methodology may also be simply applied to multiple types of M.tb-infected host cells as well as other in vitro-derived infected cells (Pisu et al. 2020b). In a subsequent study, infected human splenic macrophages were used to study the mRNA profiles of two closely related clinical strains of the Latin American and Mediterranean family of M.tb using dual RNA-seq (López-Agudelo et al. 2022). They further analysed the data using a genome-scale host–pathogen metabolic reconstruction and showed that the host and pathogen’s metabolic responses are also determined by the M.tb strain that is causing the infection. This highlights the significant role of macrophage ontogeny and M.tb genetic programs in shaping host–pathogen interactions. The expression of M.tb proteins, which are targeted by vaccines and drugs, in the lung is crucial for their effectiveness; however, the pulmonary expression of most M.tb genes and their proteins remains inadequately understood. To bridge this gap, an extensive transcriptomic analysis has been performed from M.tb-infected humans and TB prone C3H/FeJ and TB resistant C57BL/6 J mice, comparing with in vitro M.tb gene expression (Lai et al. 2021). Results reveal distinct host responses despite genetic similarity between the pathogens, indicating that the infecting M.tb strain influences both host and pathogen metabolic responses. This highlights the significant role of macrophage ontogeny and M.tb genetic programs in shaping host–pathogen interactions. Modelling the critical transmission phase of TB, which depends on infectious sputum, presents significant challenges. Dual RNA-seq on sputum of TB-infected patients reveals heightened transcriptional activation of inflammatory responses, particularly an interferon-driven proinflammatory reaction, alongside a metabolic transition towards glycolysis in the host (Lai et al. 2021). Mycobacterium tuberculosis constituted ∼1.5% of sputum bacterial sequences, and its presence led to a reduction in commensal bacterial abundance.
Single-cell RNA sequencing (scRNA-seq)
scRNA-seq technology enables the comparison of transcriptomes among distinct cell types in biological systems, overcoming cellular heterogeneity in clinical samples, and facilitating the study of individual M.tb strains from infection subpopulations during drug treatment. Recent studies have explained functional heterogeneity and the role of type I interferons in TB-infected lungs and intestines, highlighting the potential of this technique. Recently, an innovative approach was reported to concurrently obtain the host transcriptome, surface marker expression, and bacterial phenotype for each infected cell using scRNA-seq and fluorescent reporter strains of bacteria. This method facilitates easier analysis of the functional heterogeneity of IMs and AMs infected with M.tb in vivo (Pisu et al. 2021). Along with three distinct populations of IMs with diverse bacterial characteristics, they additionally identified clusters of pro-inflammatory AMs linked to stressed bacteria. Ultimately, they showed that the predominant lung macrophage populations exhibit epigenetically regulated responses to infection, whereas cross-species analysis indicates that the majority of AM subsets are shared by humans and mice. This theoretical framework can be easily applied to other infectious disease agents, perhaps leading to a deeper comprehension of the functions that various host cell types perform during the course of an infection (Pisu et al. 2021). In a follow-up investigation, Pisu and Russell (2023) developed a method for conducting multi-modal scRNA-seq on in vivo M.tb-infected lung macrophages (Pisu and Russell 2023). This approach aimed to elucidate the diverse roles of immune cells in either combating or exacerbating M.tb infection. This method captures cell transcriptome, surface markers, and bacterial phenotype simultaneously, using methanol fixation method, scRNA-seq library preparation, cell sorting, CITE-seq, and antibody labelling. It is applicable to diverse tissues from humans, nonhuman primates, and mice. Akter and Khader (2023) outline a protocol for robustly analysing scRNA-seq data from lung lymphocyte populations, addressing challenges posed by cellular diversity and biological variability (Akter and Khader 2023). Focused on lymphocyte populations from healthy and M.tb-infected mice, the protocol cover downloading processed data, integration of samples, and conducting cluster analysis. Furthermore, it elaborates on identifying lymphoid cell subtypes, performing differential analysis, and enriching pathways, offering a comprehensive approach to studying lung immune responses. In a single-cell transcriptomic study, lung tissues from patients with pulmonary TB, comparing the region with high 18F-labeled fluorodeoxyglucose-avidity to adjacent uninvolved areas and healthy donor tissues identified immune cell types and their transcriptional states associated with TB and inflammation (Pan et al. 2023b). scRNA-seq enables a comprehensive understanding of TB-associated inflammation and potential therapeutic targets for disease management (Wang et al. 2023). In yet another study, scRNA-seq was employed to analyse CD4+ and CD8-T cells from healthy individuals and TB patients, identifying distinct subsets and elucidating transcriptomic changes (Pan et al. 2023b). It revealed signature genes and pathways associated with T-cell exhaustion post-TB infection, highlighting potential exhaustion marker genes and an exhaustion-specific CD8-T cell subcluster, offering insights into TB-related T-cell signatures. Analysis of the local T cell-mediated immunity landscape in human based on TB pleural effusion reveals distinct T cell subsets and highlights the involvement of granzyme K-expressing CD8 T cells in disease pathogenesis (Cai et al. 2022). An scRNA-seq analysis framework identified immune response to M.tb infection and revealed a heterogeneous macrophage dynamics and suppressed host signalling (Gómez-González et al. 2021). Despite the limited tumor necrosis factor (TNF) production in resting macrophages during infection, the study revealed that the strength of inflammatory signals does not correspond with M.tb growth control, highlighting the importance of developing pathogen-specific signalling models.
Selection of NGS sequencing platforms for M.tb genome analysis
The M.tb genome exhibits high clonality and is marked by a combination of elevated guanine–cytosine (GC) content and repetitive structure, rendering WGS a challenging task (Phelan et al. 2016). The Illumina sequencing platform, renowned for its paired short reads and low error rates, has made it possible to successfully analyse almost the entire genome, including drug-resistance loci (Gómez-González et al. 2021). WGS demonstrates enhanced sensitivity and specificity in detecting resistance to first-line anti-TB drugs compared to second-line drugs (Wang et al. 2022). Comparative analysis of sequencing data reveals that long-read sequencing demonstrates a reduced efficiency in predicting resistance to first-line drug relative to short-read data (Peker et al. 2021). Conversely, both long-read and short-read sequencing data exhibit equivalent predictive capabilities for resistance against second-line drug (Peker et al. 2021). Additionally, TB genomes assembled from short-read data exhibit higher accuracy compared to those obtained through long-read assembly (Peker et al. 2021). Observations have indicated that Illumina short-read sequencing often encounters difficulties in amplifying repeat regions (Galagan 2014), resulting in coverage bias and, consequently, the exclusion of some or all of the pe and ppe multigene families in M.tb genomes (Mikheecheva et al. 2017, Meehan et al. 2019). GC bias in the M.tb genome is a frequently overlooked factor contributing to varying depth of coverage during WGS, a crucial aspect that often escapes the attention of researchers. Certain researchers mistakenly interpret coverage bias in Illumina WGS data as genuine deletions (Advani et al. 2019), while others recognize and acknowledge this bias (Zakham et al. 2019). In TB research, inference based on WGS primarily relies on the detection of single nucleotide polymorphisms (SNPs), the identification of complete gene deletions or insertions, and the acknowledgment of gene loss as a substantial contributor to variability within mycobacterial populations (Kato-Maeda et al. 2001, Brosch et al. 2002, Tsolaki et al. 2004). Identifying variations in repetitive regions, gene duplications, chromosomal rearrangements, and changes in the number of tandem repeats using NGS techniques like Illumina poses significant challenges and can produce biased results, leading to significant biological consequences.
The division within the M.tb WGS community regarding the management of Illumina bias highlights the importance of establishing precise exclusion criteria based on empirical evidence. To initiate progress in this direction, Tyler et al. (2016) research on the accuracy of Illumina-sequenced M.tb genomes emphasized the differing coverage bias in genomes prepared using Nextera and TruSeq library preps (Tyler et al. 2016). Their findings indicated that TruSeq-prepared samples exhibited a more consolidated genome structure than Nextera-prepared samples. Additionally, the study highlighted the difficulty in resolving specific regions, particularly those with high GC content, using either library preparation method. Modlin et al. (2021) developed tailored catalogs of genomic blind spots for various sequencing instruments and library preparations (Modlin et al. 2021). These catalogs are designed to optimize coverage for specific regions of interest, enabling the establishment of exclusion criteria based on empirical data from previous studies, thereby moving away from heuristic approaches. Recent studies have showcased the promising capabilities of long-read sequencing, particularly Oxford Nanopore Technologies, which has exhibited strong performance in variant calling and has shown improved coverage in repetitive regions, despite a relatively higher error rate (Gómez-González et al. 2022). In a subsequent study, a comparison between Illumina (short read) and Nanopore (long read) sequencing of M.tb isolates suggested that both technologies can be used independently or together by health laboratories involved in M.tb drug susceptibility testing (DST) genotypic and outbreak analysis (Hall et al. 2023). The selection of the sequencing platform depends on considerations such as costs, which may vary by country, as well as batching and turnaround time (Hall et al. 2023).
WGS to investigate genetic determinants in M.tb strains
Current research is predominantly centered on the identification and characterization of chromosomal mutations that confer resistance, along with an exploration of their association with the fitness of M.tb species (Björkman et al. 2000, Gagneux et al. 2006, Caminero 2010). A notable frequency of M.tb strains with high fitness-cost conferring mutations can be observed where compensatory mutations trade-off to restore reduced fitness capability (Gygli et al. 2017). The development of drug resistance, increased transmissibility, and heightened virulence is significantly influenced by genomic heterogeneity or microevolution in M.tb strains (Gygli et al. 2017). The adaptive capability of drug-resistant M.tb strains is associated with factors such as strain genetic background, epistatic interaction of compensatory mutations, and availability of multiple resistance mutations (Fenner et al. 2012). The genetic background of a strain may influence the impact of drug resistance mutation on fitness, as demonstrated by the variable fitness observed among different clinical isolates with the same amino acid substitution. This suggests that the genetic background plays a crucial role in overcoming the fitness cost imposed by resistance-conferring mutations. Furthermore, the extent of drug resistance resulting from a specific mutation may vary based on the genetic background of the strain (Fenner et al. 2012). Conventional molecular methods and targeted sequencing techniques, such as PCR, tend to be time-consuming and have limited access to the genomic regions of the M.tb strain. Routine molecular methods, including restriction fragment length polymorphism (RFLP) typing (Kamerbeek et al. 1997), spoligotyping (van Embden et al. 1993), and Mycobacterial Interspersed Repetitive Unit-Variable-Number Tandem Repeat (MIRU-VNTR) typing (Supply et al. 1997, 2000), are limited in their ability to resolve genetic heterogeneity across the entire genome, as they operate exclusively on specific regions of the M.tb genome. The application of sanger sequencing (Sanger et al. 1977) for targeting drug resistance-associated genes revealed hetero resistance (concurrent presence of drug-resistant and drug-sensitive strains) (Streicher et al. 2012); however, the identification of heterogeneity was constrained to a small portion of the M.tb genome. Prior investigations have indicated that the identification of genetic diversity in M.tb through WGS may be influenced by the approach to sample collection (Goig et al. 2020). Specifically, the direct sequencing of strains from sputum samples has been shown to be more efficient in capturing within-sample genetic diversity compared to isolating M.tb strains from MGIT (mycobacterium growth indicator tube) culture (Walker et al. 2013). The advent of WGS has enabled the examination of the complete genomes, facilitating a more comprehensive analysis of evolutionary relationships and providing a higher resolution to the genetic background of M.tb lineages (Castro et al. 2020). As the number of TB cases continues to rise worldwide, the trend of utilizing WGS analysis and comparisons is on the rise. These NGS approaches are instrumental in delineating genetic variations, resistant mutations, and other significant genetic changes occurring in M.tb strains.
Advantage of WGS in predicting drug susceptibility of M.tb
The MGIT approach currently serves as the reference standard for DST of M.tb isolates. Nevertheless, the use of WGS is growing in many developed countries for resistance detection and susceptibility prediction. The preceding studies examined the reliability of WGS and its disparities with the MGIT method in predicting drug susceptibility for first-line drugs (Quan et al. 2018, van Beek et al. 2019). WGS outperforms line probe assay (LPA)-based susceptibility testing in detecting low-level resistance to rifampicin and ethambutol (Genestet et al. 2020). The LPA proves to be less accurate than WGS in predicting susceptibility to anti-TB drugs (Genestet et al. 2020). Consequently, WGS has the potential to substitute for phenotypic DST, especially in countries with a low prevalence of drug-resistant M.tb strain. Still, refinement is possible as additional data accumulate, especially concerning the characterization of the impacts of novel mutations, which may confer resistance, on DST predictions (Quan et al. 2018). Phenotypic DST, which relies on critical concentration testing methods like the MGIT method, may produce inaccurate results (Ruesen et al. 2018). WGS-predicted drug resistance mutations with minimum inhibitory concentrations demonstrates specific mutations correlate with different levels of resistance (Ruesen et al. 2018). This insight not only facilitates decision-making but also enhances drug monitoring protocols to improve treatment outcomes.
Recent advances in tools and databases in WGS analysis of M.tb
Creating novel software tools for the analysis of extensive biological datasets is a crucial element in the progression of contemporary biomedical research. The extensive amount of M.tb WGS data highlights the imperative for the development of customized tools and databases for bioinformatics analysis (Fig. 2). This development is essential for the effective and efficient handling of data analysis and visualization of results. Until now, the majority of databases and software tools have primarily focused on predicting resistant M.tb strains and identifying drug targets (Table 1). Here, we provide a compilation of freely available bioinformatics software tools and databases designed for the genomic analysis of M.tb strains (Table 1). Additionally, drug resistance mutations in M.tb are critical for identifying and managing drug-resistant TB strains, which can be detected using genomic analysis tools. Public databases such as TB-Lineage and TB DEPOT provide comprehensive resources for researchers to access and analyse mutation data specific to TB, facilitating advancements in diagnosis. Table 2 summarizes list of public databases designed to identify drug resistance mutations in M.tb. This resource facilitates the swift determination of antibiotic resistance profiles in clinical isolates, aiding in the development of effective treatment strategies.
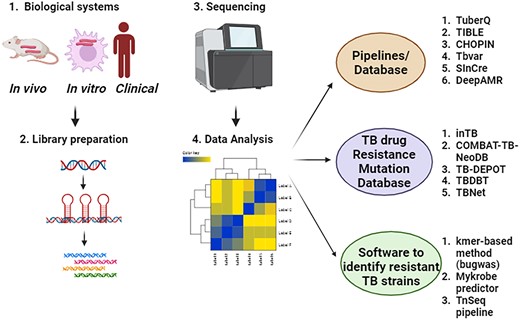
NGS sequencing leading to development of bioinformatics tools and database in TB research.
Tools and pipelines for identifying drug-resistant M.tb strains and analysing TB infections.
Name . | Category . | Application . | Access link . | References . |
---|---|---|---|---|
kmer-based method (Bugwas) | Software/Database | Uses a linear mixed model method to identify genetic variants causing drug resistance at lineage-level, focusing on differences in genomic regions in bacterial pathogens causing TB infection. | https://github.com/sgearle/bugwas | Earle et al. (2016), Jaillard et al. (2018) |
Mykrobe predictor | Software/Database | The Mykrobe predictor software package efficiently analyses raw read sequence data to produce user-friendly reports on drug-resistant M.tb strains | https://github.com/Mykrobe-tools/mykrobe | Hunt et al. (2019) |
TnSeq pipeline | Software/Database | TnSeq data analysis maps reads from transposon-junction to the mutant strain’s genome, allowing for strain-specific traits investigation | https://gitlab.com/tbgenomicsunit/tnseq-pipeline | Carey et al. (2018) |
TB-DROP | Software/Database | A tailored deep learning model to predict MTB drug resistance using genome mutations | https://github.com/nottwy/TB-DROP | Wang et al. (2024) |
Protein druggability database (TuberQ) | Pipeline/Database | It uses 3982 Open Reading Frames from the H37Rv strain for HMMer analysis, microarray expression data, structural homology-modeling, and drug pockets prediction to predict druggable M.tb proteins | http://tuberq.proteinq.com.ar/ | Radusky et al. (2014) |
SpolLineages | Pipeline/Database | This tool is a Java-based program that mainly relies on components from RuleTB, SITVIT2 database, decision tree, and evolutionary computations. Used to identify M.tb complex through various typing patterns | https://github.com/dcouvin/SpolLineages | Couvin et al. (2020) |
CHOPIN | Database | Predicts the structural effect from mutations conferring drug resistance to the M.tb complex | http://structure.bioc.cam.ac.uk/chopin | Ochoa-Montaño et al. (2015) |
Tbvar | Database | Annotates and identifies novel variants using the WGS technique | http://genome.igib.res.in/tbvar/ | Joshi et al. (2014) |
SInCre | Database | Analyse the M. tb proteome, enabling functional domain, homology, binding pockets, and structural annotation | http://proline.biochem.iisc.ernet.in | Metri et al. (2015) |
TIBLE | Pipeline/Database | TIBLE is a user-friendly online resource that offers convenient access to information on the minimal inhibitory concentrations of small molecules against various mycobacterial species. Additionally, it provides predictions on target binding and off-target effects for M.tb | http://www-cryst.bioc.cam.ac.uk/tible/ | Malhotra et al. (2017) |
AntiTbPdb | Database | The AntiTbPdb serves as a repository for experimentally validated peptides with anti-tubercular or anti-mycobacterial properties. It furnishes comprehensive details for each peptide, including sequence, modifications, origin, strain-specific mycobacterium species, inhibition concentration, specific immune response, and more. Additionally, the database incorporates predicted structures for these anti-tubercular peptides | http://webs.iiitd.edu.in/raghava/antitbpdb/ | Usmani et al. (2018) |
HGV&TB Database | Database | It contains information on 98 TB genes from 307 variants | genome.igig.res.in/hgvtb/index.html | Sahajpal et al. (2014) |
SpolPred | Software | Identifies the spoligotype in M.tb from NGS raw read sequences | www.pathogenseq.org/spolpred | Coll et al. (2012) |
MycPermCheck | Online prediction tool | A web tool for analysing small molecule permeability in M.tb cells, predicting based on logistic regression, and target molecule physico-chemical features | http://www.mycpermcheck.aksotriffer.pharmazie.uni-wuerzburg.de | Merget et al. (2013) |
DeepAMR | Online prediction tool | This tool uses genome sequence data to classify drug-resistance labels with reduced dimensionality, achieving high sensitivity and specificity | http://www.robots.ox.ac.uk/∼davidc/code.php | Yang et al. (2019) |
MtbRegList | Database | This tool uses genome sequence data to classify drug-resistance labels with reduced dimensionality, achieving high sensitivity and specificity | http://www.USherbrooke.ca/vers/MtbRegList | Jacques et al. (2005) |
TubercuList | Database | This database utilizes up-to-date curated genomes and protein 3D structures information to reannotate previously published TB genomes, enabling accurate prediction of genes and their respective functions | http://genolist.pasteur.fr/TubercuList/ | Camus et al. (2002) |
SAM-TB | Pipeline/Database | SAM-TB integrates variant detection, genomic cluster inference, detection of mixed NTM and MTB samples, and NTM species identification. SAM-TB also offers confidence levels for resistance predictions and supports batch export of analysis results | http://samtb.szmbzx.com | Yang et al. (2022b) |
TB-Profiler | Online profiling tool | Bioinformatics webserve for trimming NGS reads, reference genome alignment, and variant calling | https://tbdr.lshtm.ac.uk/ | Phelan et al. (2019) |
Name . | Category . | Application . | Access link . | References . |
---|---|---|---|---|
kmer-based method (Bugwas) | Software/Database | Uses a linear mixed model method to identify genetic variants causing drug resistance at lineage-level, focusing on differences in genomic regions in bacterial pathogens causing TB infection. | https://github.com/sgearle/bugwas | Earle et al. (2016), Jaillard et al. (2018) |
Mykrobe predictor | Software/Database | The Mykrobe predictor software package efficiently analyses raw read sequence data to produce user-friendly reports on drug-resistant M.tb strains | https://github.com/Mykrobe-tools/mykrobe | Hunt et al. (2019) |
TnSeq pipeline | Software/Database | TnSeq data analysis maps reads from transposon-junction to the mutant strain’s genome, allowing for strain-specific traits investigation | https://gitlab.com/tbgenomicsunit/tnseq-pipeline | Carey et al. (2018) |
TB-DROP | Software/Database | A tailored deep learning model to predict MTB drug resistance using genome mutations | https://github.com/nottwy/TB-DROP | Wang et al. (2024) |
Protein druggability database (TuberQ) | Pipeline/Database | It uses 3982 Open Reading Frames from the H37Rv strain for HMMer analysis, microarray expression data, structural homology-modeling, and drug pockets prediction to predict druggable M.tb proteins | http://tuberq.proteinq.com.ar/ | Radusky et al. (2014) |
SpolLineages | Pipeline/Database | This tool is a Java-based program that mainly relies on components from RuleTB, SITVIT2 database, decision tree, and evolutionary computations. Used to identify M.tb complex through various typing patterns | https://github.com/dcouvin/SpolLineages | Couvin et al. (2020) |
CHOPIN | Database | Predicts the structural effect from mutations conferring drug resistance to the M.tb complex | http://structure.bioc.cam.ac.uk/chopin | Ochoa-Montaño et al. (2015) |
Tbvar | Database | Annotates and identifies novel variants using the WGS technique | http://genome.igib.res.in/tbvar/ | Joshi et al. (2014) |
SInCre | Database | Analyse the M. tb proteome, enabling functional domain, homology, binding pockets, and structural annotation | http://proline.biochem.iisc.ernet.in | Metri et al. (2015) |
TIBLE | Pipeline/Database | TIBLE is a user-friendly online resource that offers convenient access to information on the minimal inhibitory concentrations of small molecules against various mycobacterial species. Additionally, it provides predictions on target binding and off-target effects for M.tb | http://www-cryst.bioc.cam.ac.uk/tible/ | Malhotra et al. (2017) |
AntiTbPdb | Database | The AntiTbPdb serves as a repository for experimentally validated peptides with anti-tubercular or anti-mycobacterial properties. It furnishes comprehensive details for each peptide, including sequence, modifications, origin, strain-specific mycobacterium species, inhibition concentration, specific immune response, and more. Additionally, the database incorporates predicted structures for these anti-tubercular peptides | http://webs.iiitd.edu.in/raghava/antitbpdb/ | Usmani et al. (2018) |
HGV&TB Database | Database | It contains information on 98 TB genes from 307 variants | genome.igig.res.in/hgvtb/index.html | Sahajpal et al. (2014) |
SpolPred | Software | Identifies the spoligotype in M.tb from NGS raw read sequences | www.pathogenseq.org/spolpred | Coll et al. (2012) |
MycPermCheck | Online prediction tool | A web tool for analysing small molecule permeability in M.tb cells, predicting based on logistic regression, and target molecule physico-chemical features | http://www.mycpermcheck.aksotriffer.pharmazie.uni-wuerzburg.de | Merget et al. (2013) |
DeepAMR | Online prediction tool | This tool uses genome sequence data to classify drug-resistance labels with reduced dimensionality, achieving high sensitivity and specificity | http://www.robots.ox.ac.uk/∼davidc/code.php | Yang et al. (2019) |
MtbRegList | Database | This tool uses genome sequence data to classify drug-resistance labels with reduced dimensionality, achieving high sensitivity and specificity | http://www.USherbrooke.ca/vers/MtbRegList | Jacques et al. (2005) |
TubercuList | Database | This database utilizes up-to-date curated genomes and protein 3D structures information to reannotate previously published TB genomes, enabling accurate prediction of genes and their respective functions | http://genolist.pasteur.fr/TubercuList/ | Camus et al. (2002) |
SAM-TB | Pipeline/Database | SAM-TB integrates variant detection, genomic cluster inference, detection of mixed NTM and MTB samples, and NTM species identification. SAM-TB also offers confidence levels for resistance predictions and supports batch export of analysis results | http://samtb.szmbzx.com | Yang et al. (2022b) |
TB-Profiler | Online profiling tool | Bioinformatics webserve for trimming NGS reads, reference genome alignment, and variant calling | https://tbdr.lshtm.ac.uk/ | Phelan et al. (2019) |
Tools and pipelines for identifying drug-resistant M.tb strains and analysing TB infections.
Name . | Category . | Application . | Access link . | References . |
---|---|---|---|---|
kmer-based method (Bugwas) | Software/Database | Uses a linear mixed model method to identify genetic variants causing drug resistance at lineage-level, focusing on differences in genomic regions in bacterial pathogens causing TB infection. | https://github.com/sgearle/bugwas | Earle et al. (2016), Jaillard et al. (2018) |
Mykrobe predictor | Software/Database | The Mykrobe predictor software package efficiently analyses raw read sequence data to produce user-friendly reports on drug-resistant M.tb strains | https://github.com/Mykrobe-tools/mykrobe | Hunt et al. (2019) |
TnSeq pipeline | Software/Database | TnSeq data analysis maps reads from transposon-junction to the mutant strain’s genome, allowing for strain-specific traits investigation | https://gitlab.com/tbgenomicsunit/tnseq-pipeline | Carey et al. (2018) |
TB-DROP | Software/Database | A tailored deep learning model to predict MTB drug resistance using genome mutations | https://github.com/nottwy/TB-DROP | Wang et al. (2024) |
Protein druggability database (TuberQ) | Pipeline/Database | It uses 3982 Open Reading Frames from the H37Rv strain for HMMer analysis, microarray expression data, structural homology-modeling, and drug pockets prediction to predict druggable M.tb proteins | http://tuberq.proteinq.com.ar/ | Radusky et al. (2014) |
SpolLineages | Pipeline/Database | This tool is a Java-based program that mainly relies on components from RuleTB, SITVIT2 database, decision tree, and evolutionary computations. Used to identify M.tb complex through various typing patterns | https://github.com/dcouvin/SpolLineages | Couvin et al. (2020) |
CHOPIN | Database | Predicts the structural effect from mutations conferring drug resistance to the M.tb complex | http://structure.bioc.cam.ac.uk/chopin | Ochoa-Montaño et al. (2015) |
Tbvar | Database | Annotates and identifies novel variants using the WGS technique | http://genome.igib.res.in/tbvar/ | Joshi et al. (2014) |
SInCre | Database | Analyse the M. tb proteome, enabling functional domain, homology, binding pockets, and structural annotation | http://proline.biochem.iisc.ernet.in | Metri et al. (2015) |
TIBLE | Pipeline/Database | TIBLE is a user-friendly online resource that offers convenient access to information on the minimal inhibitory concentrations of small molecules against various mycobacterial species. Additionally, it provides predictions on target binding and off-target effects for M.tb | http://www-cryst.bioc.cam.ac.uk/tible/ | Malhotra et al. (2017) |
AntiTbPdb | Database | The AntiTbPdb serves as a repository for experimentally validated peptides with anti-tubercular or anti-mycobacterial properties. It furnishes comprehensive details for each peptide, including sequence, modifications, origin, strain-specific mycobacterium species, inhibition concentration, specific immune response, and more. Additionally, the database incorporates predicted structures for these anti-tubercular peptides | http://webs.iiitd.edu.in/raghava/antitbpdb/ | Usmani et al. (2018) |
HGV&TB Database | Database | It contains information on 98 TB genes from 307 variants | genome.igig.res.in/hgvtb/index.html | Sahajpal et al. (2014) |
SpolPred | Software | Identifies the spoligotype in M.tb from NGS raw read sequences | www.pathogenseq.org/spolpred | Coll et al. (2012) |
MycPermCheck | Online prediction tool | A web tool for analysing small molecule permeability in M.tb cells, predicting based on logistic regression, and target molecule physico-chemical features | http://www.mycpermcheck.aksotriffer.pharmazie.uni-wuerzburg.de | Merget et al. (2013) |
DeepAMR | Online prediction tool | This tool uses genome sequence data to classify drug-resistance labels with reduced dimensionality, achieving high sensitivity and specificity | http://www.robots.ox.ac.uk/∼davidc/code.php | Yang et al. (2019) |
MtbRegList | Database | This tool uses genome sequence data to classify drug-resistance labels with reduced dimensionality, achieving high sensitivity and specificity | http://www.USherbrooke.ca/vers/MtbRegList | Jacques et al. (2005) |
TubercuList | Database | This database utilizes up-to-date curated genomes and protein 3D structures information to reannotate previously published TB genomes, enabling accurate prediction of genes and their respective functions | http://genolist.pasteur.fr/TubercuList/ | Camus et al. (2002) |
SAM-TB | Pipeline/Database | SAM-TB integrates variant detection, genomic cluster inference, detection of mixed NTM and MTB samples, and NTM species identification. SAM-TB also offers confidence levels for resistance predictions and supports batch export of analysis results | http://samtb.szmbzx.com | Yang et al. (2022b) |
TB-Profiler | Online profiling tool | Bioinformatics webserve for trimming NGS reads, reference genome alignment, and variant calling | https://tbdr.lshtm.ac.uk/ | Phelan et al. (2019) |
Name . | Category . | Application . | Access link . | References . |
---|---|---|---|---|
kmer-based method (Bugwas) | Software/Database | Uses a linear mixed model method to identify genetic variants causing drug resistance at lineage-level, focusing on differences in genomic regions in bacterial pathogens causing TB infection. | https://github.com/sgearle/bugwas | Earle et al. (2016), Jaillard et al. (2018) |
Mykrobe predictor | Software/Database | The Mykrobe predictor software package efficiently analyses raw read sequence data to produce user-friendly reports on drug-resistant M.tb strains | https://github.com/Mykrobe-tools/mykrobe | Hunt et al. (2019) |
TnSeq pipeline | Software/Database | TnSeq data analysis maps reads from transposon-junction to the mutant strain’s genome, allowing for strain-specific traits investigation | https://gitlab.com/tbgenomicsunit/tnseq-pipeline | Carey et al. (2018) |
TB-DROP | Software/Database | A tailored deep learning model to predict MTB drug resistance using genome mutations | https://github.com/nottwy/TB-DROP | Wang et al. (2024) |
Protein druggability database (TuberQ) | Pipeline/Database | It uses 3982 Open Reading Frames from the H37Rv strain for HMMer analysis, microarray expression data, structural homology-modeling, and drug pockets prediction to predict druggable M.tb proteins | http://tuberq.proteinq.com.ar/ | Radusky et al. (2014) |
SpolLineages | Pipeline/Database | This tool is a Java-based program that mainly relies on components from RuleTB, SITVIT2 database, decision tree, and evolutionary computations. Used to identify M.tb complex through various typing patterns | https://github.com/dcouvin/SpolLineages | Couvin et al. (2020) |
CHOPIN | Database | Predicts the structural effect from mutations conferring drug resistance to the M.tb complex | http://structure.bioc.cam.ac.uk/chopin | Ochoa-Montaño et al. (2015) |
Tbvar | Database | Annotates and identifies novel variants using the WGS technique | http://genome.igib.res.in/tbvar/ | Joshi et al. (2014) |
SInCre | Database | Analyse the M. tb proteome, enabling functional domain, homology, binding pockets, and structural annotation | http://proline.biochem.iisc.ernet.in | Metri et al. (2015) |
TIBLE | Pipeline/Database | TIBLE is a user-friendly online resource that offers convenient access to information on the minimal inhibitory concentrations of small molecules against various mycobacterial species. Additionally, it provides predictions on target binding and off-target effects for M.tb | http://www-cryst.bioc.cam.ac.uk/tible/ | Malhotra et al. (2017) |
AntiTbPdb | Database | The AntiTbPdb serves as a repository for experimentally validated peptides with anti-tubercular or anti-mycobacterial properties. It furnishes comprehensive details for each peptide, including sequence, modifications, origin, strain-specific mycobacterium species, inhibition concentration, specific immune response, and more. Additionally, the database incorporates predicted structures for these anti-tubercular peptides | http://webs.iiitd.edu.in/raghava/antitbpdb/ | Usmani et al. (2018) |
HGV&TB Database | Database | It contains information on 98 TB genes from 307 variants | genome.igig.res.in/hgvtb/index.html | Sahajpal et al. (2014) |
SpolPred | Software | Identifies the spoligotype in M.tb from NGS raw read sequences | www.pathogenseq.org/spolpred | Coll et al. (2012) |
MycPermCheck | Online prediction tool | A web tool for analysing small molecule permeability in M.tb cells, predicting based on logistic regression, and target molecule physico-chemical features | http://www.mycpermcheck.aksotriffer.pharmazie.uni-wuerzburg.de | Merget et al. (2013) |
DeepAMR | Online prediction tool | This tool uses genome sequence data to classify drug-resistance labels with reduced dimensionality, achieving high sensitivity and specificity | http://www.robots.ox.ac.uk/∼davidc/code.php | Yang et al. (2019) |
MtbRegList | Database | This tool uses genome sequence data to classify drug-resistance labels with reduced dimensionality, achieving high sensitivity and specificity | http://www.USherbrooke.ca/vers/MtbRegList | Jacques et al. (2005) |
TubercuList | Database | This database utilizes up-to-date curated genomes and protein 3D structures information to reannotate previously published TB genomes, enabling accurate prediction of genes and their respective functions | http://genolist.pasteur.fr/TubercuList/ | Camus et al. (2002) |
SAM-TB | Pipeline/Database | SAM-TB integrates variant detection, genomic cluster inference, detection of mixed NTM and MTB samples, and NTM species identification. SAM-TB also offers confidence levels for resistance predictions and supports batch export of analysis results | http://samtb.szmbzx.com | Yang et al. (2022b) |
TB-Profiler | Online profiling tool | Bioinformatics webserve for trimming NGS reads, reference genome alignment, and variant calling | https://tbdr.lshtm.ac.uk/ | Phelan et al. (2019) |
Name . | Category . | Application . | Access link . | References . |
---|---|---|---|---|
TB-Lineage | Pipeline/ Online prediction tool | An online tool for classification and analysis of strains of M.tb complex | https://tbinsight.cs.rpi.edu/run_tb_lineage.html | Shabbeer et al. (2012) |
The TB Portals | Database | An open-access, web-based platform for global drug-resistant-tuberculosis data sharing and analysis | https://tbportals.niaid.nih.gov/ | Rosenthal et al. (2017) |
COMBAT-TB-NeoDB | Pipeline/Database | Fostering TB research through integrative analysis using graph database technologies | https://github.com/COMBAT-TB/combat-tb-neodb | Lose et al. (2020) |
TB DEPOT | Database | A novel public analytics platform integrating TB clinical, genomic, and radiological data for visual and statistical exploration | https://depot.tbportals.niaid.nih.gov/#/home | Gabrielian et al. (2019) |
getTBinR | Software | An R package for accessing and summarizing the World Health Organization Tuberculosis data | https://github.com/seabbs/getTBinR | Abbott (2019) |
TBDBT | Database | A TB DataBase template for collection of harmonized TB clinical research data in REDCap, facilitating data standardization for inter-study comparison and meta-analyses | https://github.com/CIDRI-Africa/TBDBT/ | Allie et al. (2021) |
TBNet | Pipeline/Database | A context-aware graph network for TB diagnosis | https://www.tbnet.eu/ | Giehl et al. (2012) |
Name . | Category . | Application . | Access link . | References . |
---|---|---|---|---|
TB-Lineage | Pipeline/ Online prediction tool | An online tool for classification and analysis of strains of M.tb complex | https://tbinsight.cs.rpi.edu/run_tb_lineage.html | Shabbeer et al. (2012) |
The TB Portals | Database | An open-access, web-based platform for global drug-resistant-tuberculosis data sharing and analysis | https://tbportals.niaid.nih.gov/ | Rosenthal et al. (2017) |
COMBAT-TB-NeoDB | Pipeline/Database | Fostering TB research through integrative analysis using graph database technologies | https://github.com/COMBAT-TB/combat-tb-neodb | Lose et al. (2020) |
TB DEPOT | Database | A novel public analytics platform integrating TB clinical, genomic, and radiological data for visual and statistical exploration | https://depot.tbportals.niaid.nih.gov/#/home | Gabrielian et al. (2019) |
getTBinR | Software | An R package for accessing and summarizing the World Health Organization Tuberculosis data | https://github.com/seabbs/getTBinR | Abbott (2019) |
TBDBT | Database | A TB DataBase template for collection of harmonized TB clinical research data in REDCap, facilitating data standardization for inter-study comparison and meta-analyses | https://github.com/CIDRI-Africa/TBDBT/ | Allie et al. (2021) |
TBNet | Pipeline/Database | A context-aware graph network for TB diagnosis | https://www.tbnet.eu/ | Giehl et al. (2012) |
Name . | Category . | Application . | Access link . | References . |
---|---|---|---|---|
TB-Lineage | Pipeline/ Online prediction tool | An online tool for classification and analysis of strains of M.tb complex | https://tbinsight.cs.rpi.edu/run_tb_lineage.html | Shabbeer et al. (2012) |
The TB Portals | Database | An open-access, web-based platform for global drug-resistant-tuberculosis data sharing and analysis | https://tbportals.niaid.nih.gov/ | Rosenthal et al. (2017) |
COMBAT-TB-NeoDB | Pipeline/Database | Fostering TB research through integrative analysis using graph database technologies | https://github.com/COMBAT-TB/combat-tb-neodb | Lose et al. (2020) |
TB DEPOT | Database | A novel public analytics platform integrating TB clinical, genomic, and radiological data for visual and statistical exploration | https://depot.tbportals.niaid.nih.gov/#/home | Gabrielian et al. (2019) |
getTBinR | Software | An R package for accessing and summarizing the World Health Organization Tuberculosis data | https://github.com/seabbs/getTBinR | Abbott (2019) |
TBDBT | Database | A TB DataBase template for collection of harmonized TB clinical research data in REDCap, facilitating data standardization for inter-study comparison and meta-analyses | https://github.com/CIDRI-Africa/TBDBT/ | Allie et al. (2021) |
TBNet | Pipeline/Database | A context-aware graph network for TB diagnosis | https://www.tbnet.eu/ | Giehl et al. (2012) |
Name . | Category . | Application . | Access link . | References . |
---|---|---|---|---|
TB-Lineage | Pipeline/ Online prediction tool | An online tool for classification and analysis of strains of M.tb complex | https://tbinsight.cs.rpi.edu/run_tb_lineage.html | Shabbeer et al. (2012) |
The TB Portals | Database | An open-access, web-based platform for global drug-resistant-tuberculosis data sharing and analysis | https://tbportals.niaid.nih.gov/ | Rosenthal et al. (2017) |
COMBAT-TB-NeoDB | Pipeline/Database | Fostering TB research through integrative analysis using graph database technologies | https://github.com/COMBAT-TB/combat-tb-neodb | Lose et al. (2020) |
TB DEPOT | Database | A novel public analytics platform integrating TB clinical, genomic, and radiological data for visual and statistical exploration | https://depot.tbportals.niaid.nih.gov/#/home | Gabrielian et al. (2019) |
getTBinR | Software | An R package for accessing and summarizing the World Health Organization Tuberculosis data | https://github.com/seabbs/getTBinR | Abbott (2019) |
TBDBT | Database | A TB DataBase template for collection of harmonized TB clinical research data in REDCap, facilitating data standardization for inter-study comparison and meta-analyses | https://github.com/CIDRI-Africa/TBDBT/ | Allie et al. (2021) |
TBNet | Pipeline/Database | A context-aware graph network for TB diagnosis | https://www.tbnet.eu/ | Giehl et al. (2012) |
Evaluating minor genetic variation in M.tb population
The progress in molecular techniques has unveiled the capacity of M.tb to engage in polyclonal infections (Moreno-Molina et al. 2021). Mixed infections may give rise to multiple unrelated clones within a patient, or microevolution may lead to the emergence of closely related clones from a previously clonal M.tb population. It is crucial to accurately identify minor variants in M.tb population to improve our understanding of hetero-resistance within dynamic M.tb populations. Identification of minor variants in WGS data has always been challenging due to the limitations of trimming, filtering, and standard methods to differentiate low-frequency variants from sequence ambiguities (Said Mohammed et al. 2018). The recent introduction of the bioinformatics tool, BinoSNP, has simplified the process of identifying minor variants by assessing a customized collection of genomic positions through a binomial test method (Dreyer et al. 2020). Nevertheless, its capability is confined to the identification of SNPs in resistance-conferring genes. Therefore, tools like BinoSNP are unsuitable for detecting unspecified variants like de novo detection of non-resistant variation in minor population groups. Subsequent studies have reported that the LoFreq variant calling tool facilitates the identification of minor variants, including both SNPs and indels, within predetermined resistance-associated loci and previously unexplored genomic regions (Wilm et al. 2012). Goosens et al. (2022), assessed LoFreq’s performance in detecting de novo and drug resistance-associated minor variants in both simulated and clinical M.tb NGS data (Goossens et al. 2022). The results show LoFreq as a precise variant caller with high sensitivity, especially for indels. It exhibits exceptional sharpness and accuracy across the entire spectrum of coverage depths assessed, regardless of the minor variant type or frequency. It reliably detects variants with a frequency limit of detection at 0.5% for indels and 3% for SNPs. In clinical data, LoFreq successfully identified minor M.tb variants, even at low allele frequencies. This suggests its potential to reduce false positives due to sequencing errors. These findings aid in determining detection limits and guiding future M.tb variant studies. An additional limitation is small clinical sample size, which precluded the statistical validation of LoFreq’s performance metrics and underscored the need to conduct validation tests on a larger set of clinical samples, covering both SNP and indel mutations. These observations collectively emphasize the ongoing need for benchmarking whole-genome variant calling tools capable of detecting minor M.tb variants at various depths of population coverage.
Mycobacterium tuberculosis pangenome analysis
The pan-genome encompasses the entire genetic repertoire of a microbial population, comprising core orthologous genes, unique strain-specific genes, and accessory genes. Open pan-genomes incorporate novel gene families, whereas closed pan-genomes exhibit no additional extension (Bosi et al. 2015). The pan-genome approach offers insights into the distribution of virulence genes within pathogenic microbial populations, particularly in monitoring the emergence of drug-resistant genes from novel genetic variants (Muzzi et al. 2007). In recent times, pan-genome analysis has gained popularity for investigating genetic signatures related to antibiotic resistance (Kavvas et al. 2018), adaptive evolution (Yang et al. 2018), and assessing genomic distance among M.tb lineages (Jandrasits et al. 2019). PANPASCO, a computational method for pan-genome mapping, utilizes pairwise distance calculations, demonstrating high sensitivity to variations between cases, and leverages WGS for effective transmission surveillance (Jandrasits et al. 2019). Additional research on the Mycobacterium pan-genome has revealed its significance in identifying potential drug targets and understanding the diversification of the Type VII secretion system, which in turn influences the pathogenicity of M.tb strains (Dumas et al. 2016, Dar et al. 2020). However, as indicated by Kim et al. M.tb is not considered a pathogen that is ideally suited for pan-genome studies; this may be due to its high genomic homogeneity and strict clonality (Kim et al. 2020).
WGS for identification of M.tb mixed infections
Mixed infections arise from either concurrent infection by distinct strains in a patient or strain evolution within the host, resulting in two co-existing populations. Mixed M.tb infections and heteroresistance present challenges for the prognosis and treatment of TB disease. Their detection has predominantly been limited to conventional genotyping techniques, which often lack the required sensitivity and result in inaccurate estimations of population diversity in TB infections (Richardson et al. 2002, van Rie et al. 2005, Zetola et al. 2014, Zong et al. 2018, Liang et al. 2020). The GeneXpert assay has revealed that the current diagnostic methods are not very effective in identifying mixed infections that involve both M.tb and nontuberculous Mycobacteria (NTM). This highlights the urgent need to promptly adopt targeted molecular analyses that specifically capture multiple loci of mycobacterial species from specimens. Inadequate exploration of within-host M.tb diversity makes it difficult to distinguish between relapse and reinfection (Zong et al. 2018). Although WGS provides a comprehensive view of the genetic makeup of an individual strain, challenges still exist in interpreting and analysing the data to identify components of a mixed infection. There are limited established methods for identifying mixed TB infections through WGS data. New approaches, such as Bayesian framework analysis and heterozygous allele identification, have emerged to distinguish strains within M.tb population (Yang et al. 2023).
Deep WGS has proven effective in discerning M.tb strains within mixed infections through exploration of phylogenomic databases derived from single nucleotide variant (SNV) analysis (Gan et al. 2016). A recent paper by Lozano et al. (2021) proposed a novel strategy for capturing minority variants and identifying mixed infections using WGS data (Lozano et al. 2021). The researchers designed a platform named MycoCAP, comprising M.tb DNA capture probes, which enables the targeted enrichment of samples with M.tb DNA (Lozano et al. 2021). Subsequently, they conducted WGS on the captured M.tb DNA to enhance the detection of minority variants and mixed infections. To date, two bioinformatics tools have been reported, specifically designed for the classification of strains in mixed infections using WGS data. The first tool, QuantTB, was designed to quantify individual M.tb strains by comparing TB genomes with reference SNPs of each lineage (Anyansi et al. 2020). The second tool, SplitStrains, employs a rigorous statistical method and the Expectation-Maximization algorithm to separate the constituent strains in a mixed infection accurately (Gabbassov et al. 2021).
Transmission network analysis of TB infection
Genomic sequences of M.tb strains obtained at various time points are progressively employed to deduce the initiation of specific outbreaks, the emergence and proliferation of drug-resistant clones, or the introduction of a strain into a particular geographic region (Saavedra Cervera et al. 2022, Yang et al. 2022a). To gain epidemiological insights, the consideration of a temporally calibrated phylogeny is particularly valuable for reconstructing infectious disease transmission patterns from genomic data (Didelot et al. 2021). More recently, the application of deep NGS has demonstrated considerable promise as an effective strategy for genome-based surveillance of pathogens and the establishment of transmission links among sequenced bacterial pathogens (Sobkowiak et al. 2023). A systematic effort has been devoted to the comparative analysis of publicly available transmission reconstruction models (Sobkowiak et al. 2023). The primary objective is to evaluate the accuracy of these models in predicting transmission events in both simulated and real-world outbreaks of M.tb (Sobkowiak et al. 2023). Using SNP thresholds, WGS improves precision in determining the direction and timing of individual transmission events in M.tb infection (Walker et al. 2013, Stimson et al. 2019). A more advanced approach for transmission reconstruction involves using time scale phylogenetic trees, known as phylodynamics (Didelot et al. 2014). However, challenges such as within-host evolution, latency periods, and low genomic heterogeneity complicate the application of phylodynamics in TB transmission analysis (Ypma et al. 2013, Romero-Severson et al. 2014). Various computational tools integrate genomic variation and epidemiological data to estimate the likelihood of individual-level transmission events from genomic data (Table 3). These tools predominantly employ a Bayesian Markov Chain Monte Carlo framework and robust statistical approach with rigorous computational validation of epidemiological parameters. A recent study has described online tools used for the visualization of transmission networks and evaluated their feasibility for real-time analyses of pathogen sequence data (Neher and Bedford et al. 2018).
List of available software and tools used for infection transmission analysis.
Tools . | Software applications . | Input data . | Accesses link . | Source . |
---|---|---|---|---|
TransPhylo | R and Matlab | Time-stamped phylogenetic tree | https://github.com/xavierdidelot/TransPhylo | Didelot et al. (2014) |
SCOTTI | Python | Time-stamped phylogenetic tree | https://bitbucket.org/nicofmay/scotti/src/master/ | De Maio et al. (2016) |
outbreaker2 | R and C++ | Time-stamped phylogenetic tree | http://www.repidemicsconsortium.org/outbreaker2/ | Campbell et al. (2018) |
TransFlow | R and Python | Raw reads and sample metadata | https://github.com/cvn001/transflow | Pan et al. (2023a) |
Phybreak | R | Time-stamped phylogenetic tree | https://github.com/donkeyshot/phybreak | Klinkenberg et al. (2017) |
QUENTIN | MATLAB | Aligned fasta Sequence | https://github.com/skumsp/QUENTIN | Skums et al. (2018) |
PHYLOSCANNER | Python and R | Bam files | https://github.com/BDI-pathogens/phyloscanner | Wymant et al. (2018) |
nosoi | R | User defined host parameters | https://slequime.github.io/nosoi/index.html | Lequime et al. (2020) |
TNet | Python | Pathogen phylogeny | https://github.com/sauravdhr/tnet_python | Dhar et al. (2022) |
LITT | R | SNP matrix and epidemological data | https://github.com/CDCgov/TB_molecular_epidemiology/tree/1.0; | Winglee et al. (2021) |
o2geosocial | R | Epidemiological data (do not include genetic sequences) | https://github.com/alxsrobert/o2geosocial | Robert et al. (2021) |
GraphSNP | R and Java | SNP distance | https://github.com/nalarbp/graphsnp | Permana et al. (2023) |
SOPHIE | Python and MATLAB | Phylogenetic tree and sample meta data | https://github.com/compbel/SOPHIE/ | Skums et al. (2022) |
P-DOR | Python | Assembled genome and SNP phylogeny | https://github.com/SteMIDIfactory/P-DOR | Batisti Biffignandi et al. (2023) |
StrainHub | R | phylogenetic tree and associated metadata | https://github.com/abschneider/StrainHub | de Bernardi Schneider et al. (2020) |
Time-scaled haplotypic density (THD) | R | Genetic distances and user-defined parameters | https://github.com/rasigadelab/thd | Wirth et al. (2020) |
Visualization of transmission network | ||||
Nextstrain | Web application | Nextstrain employs TreeTime to infer time-scaled phylogenies and conduct ancestral sequence inference to determine the likely geographic origins of ancestral nodes | https://nextstrain.org/ | Hadfield et al. 2018 |
Microreact | Web application | Microreact facilitates the exploration of phylogenetic trees as well as spatial and temporal data of samples. Custom datasets can be imported into the application using a Newick tree and sample metadata in tabular format | https://microreact.org/ | Argimon et al. 2016 |
Graphia | Open-source platform | Graphia is a novel visual analytics platform specifically designed for the network-based analysis of large and complex datasets, such as those generated in vast quantities by modern biological analyses | https://graphia.app/ | Freeman et al. 2022 |
Tools . | Software applications . | Input data . | Accesses link . | Source . |
---|---|---|---|---|
TransPhylo | R and Matlab | Time-stamped phylogenetic tree | https://github.com/xavierdidelot/TransPhylo | Didelot et al. (2014) |
SCOTTI | Python | Time-stamped phylogenetic tree | https://bitbucket.org/nicofmay/scotti/src/master/ | De Maio et al. (2016) |
outbreaker2 | R and C++ | Time-stamped phylogenetic tree | http://www.repidemicsconsortium.org/outbreaker2/ | Campbell et al. (2018) |
TransFlow | R and Python | Raw reads and sample metadata | https://github.com/cvn001/transflow | Pan et al. (2023a) |
Phybreak | R | Time-stamped phylogenetic tree | https://github.com/donkeyshot/phybreak | Klinkenberg et al. (2017) |
QUENTIN | MATLAB | Aligned fasta Sequence | https://github.com/skumsp/QUENTIN | Skums et al. (2018) |
PHYLOSCANNER | Python and R | Bam files | https://github.com/BDI-pathogens/phyloscanner | Wymant et al. (2018) |
nosoi | R | User defined host parameters | https://slequime.github.io/nosoi/index.html | Lequime et al. (2020) |
TNet | Python | Pathogen phylogeny | https://github.com/sauravdhr/tnet_python | Dhar et al. (2022) |
LITT | R | SNP matrix and epidemological data | https://github.com/CDCgov/TB_molecular_epidemiology/tree/1.0; | Winglee et al. (2021) |
o2geosocial | R | Epidemiological data (do not include genetic sequences) | https://github.com/alxsrobert/o2geosocial | Robert et al. (2021) |
GraphSNP | R and Java | SNP distance | https://github.com/nalarbp/graphsnp | Permana et al. (2023) |
SOPHIE | Python and MATLAB | Phylogenetic tree and sample meta data | https://github.com/compbel/SOPHIE/ | Skums et al. (2022) |
P-DOR | Python | Assembled genome and SNP phylogeny | https://github.com/SteMIDIfactory/P-DOR | Batisti Biffignandi et al. (2023) |
StrainHub | R | phylogenetic tree and associated metadata | https://github.com/abschneider/StrainHub | de Bernardi Schneider et al. (2020) |
Time-scaled haplotypic density (THD) | R | Genetic distances and user-defined parameters | https://github.com/rasigadelab/thd | Wirth et al. (2020) |
Visualization of transmission network | ||||
Nextstrain | Web application | Nextstrain employs TreeTime to infer time-scaled phylogenies and conduct ancestral sequence inference to determine the likely geographic origins of ancestral nodes | https://nextstrain.org/ | Hadfield et al. 2018 |
Microreact | Web application | Microreact facilitates the exploration of phylogenetic trees as well as spatial and temporal data of samples. Custom datasets can be imported into the application using a Newick tree and sample metadata in tabular format | https://microreact.org/ | Argimon et al. 2016 |
Graphia | Open-source platform | Graphia is a novel visual analytics platform specifically designed for the network-based analysis of large and complex datasets, such as those generated in vast quantities by modern biological analyses | https://graphia.app/ | Freeman et al. 2022 |
All software and tools are freely available for public use under the General Public License version 3.
List of available software and tools used for infection transmission analysis.
Tools . | Software applications . | Input data . | Accesses link . | Source . |
---|---|---|---|---|
TransPhylo | R and Matlab | Time-stamped phylogenetic tree | https://github.com/xavierdidelot/TransPhylo | Didelot et al. (2014) |
SCOTTI | Python | Time-stamped phylogenetic tree | https://bitbucket.org/nicofmay/scotti/src/master/ | De Maio et al. (2016) |
outbreaker2 | R and C++ | Time-stamped phylogenetic tree | http://www.repidemicsconsortium.org/outbreaker2/ | Campbell et al. (2018) |
TransFlow | R and Python | Raw reads and sample metadata | https://github.com/cvn001/transflow | Pan et al. (2023a) |
Phybreak | R | Time-stamped phylogenetic tree | https://github.com/donkeyshot/phybreak | Klinkenberg et al. (2017) |
QUENTIN | MATLAB | Aligned fasta Sequence | https://github.com/skumsp/QUENTIN | Skums et al. (2018) |
PHYLOSCANNER | Python and R | Bam files | https://github.com/BDI-pathogens/phyloscanner | Wymant et al. (2018) |
nosoi | R | User defined host parameters | https://slequime.github.io/nosoi/index.html | Lequime et al. (2020) |
TNet | Python | Pathogen phylogeny | https://github.com/sauravdhr/tnet_python | Dhar et al. (2022) |
LITT | R | SNP matrix and epidemological data | https://github.com/CDCgov/TB_molecular_epidemiology/tree/1.0; | Winglee et al. (2021) |
o2geosocial | R | Epidemiological data (do not include genetic sequences) | https://github.com/alxsrobert/o2geosocial | Robert et al. (2021) |
GraphSNP | R and Java | SNP distance | https://github.com/nalarbp/graphsnp | Permana et al. (2023) |
SOPHIE | Python and MATLAB | Phylogenetic tree and sample meta data | https://github.com/compbel/SOPHIE/ | Skums et al. (2022) |
P-DOR | Python | Assembled genome and SNP phylogeny | https://github.com/SteMIDIfactory/P-DOR | Batisti Biffignandi et al. (2023) |
StrainHub | R | phylogenetic tree and associated metadata | https://github.com/abschneider/StrainHub | de Bernardi Schneider et al. (2020) |
Time-scaled haplotypic density (THD) | R | Genetic distances and user-defined parameters | https://github.com/rasigadelab/thd | Wirth et al. (2020) |
Visualization of transmission network | ||||
Nextstrain | Web application | Nextstrain employs TreeTime to infer time-scaled phylogenies and conduct ancestral sequence inference to determine the likely geographic origins of ancestral nodes | https://nextstrain.org/ | Hadfield et al. 2018 |
Microreact | Web application | Microreact facilitates the exploration of phylogenetic trees as well as spatial and temporal data of samples. Custom datasets can be imported into the application using a Newick tree and sample metadata in tabular format | https://microreact.org/ | Argimon et al. 2016 |
Graphia | Open-source platform | Graphia is a novel visual analytics platform specifically designed for the network-based analysis of large and complex datasets, such as those generated in vast quantities by modern biological analyses | https://graphia.app/ | Freeman et al. 2022 |
Tools . | Software applications . | Input data . | Accesses link . | Source . |
---|---|---|---|---|
TransPhylo | R and Matlab | Time-stamped phylogenetic tree | https://github.com/xavierdidelot/TransPhylo | Didelot et al. (2014) |
SCOTTI | Python | Time-stamped phylogenetic tree | https://bitbucket.org/nicofmay/scotti/src/master/ | De Maio et al. (2016) |
outbreaker2 | R and C++ | Time-stamped phylogenetic tree | http://www.repidemicsconsortium.org/outbreaker2/ | Campbell et al. (2018) |
TransFlow | R and Python | Raw reads and sample metadata | https://github.com/cvn001/transflow | Pan et al. (2023a) |
Phybreak | R | Time-stamped phylogenetic tree | https://github.com/donkeyshot/phybreak | Klinkenberg et al. (2017) |
QUENTIN | MATLAB | Aligned fasta Sequence | https://github.com/skumsp/QUENTIN | Skums et al. (2018) |
PHYLOSCANNER | Python and R | Bam files | https://github.com/BDI-pathogens/phyloscanner | Wymant et al. (2018) |
nosoi | R | User defined host parameters | https://slequime.github.io/nosoi/index.html | Lequime et al. (2020) |
TNet | Python | Pathogen phylogeny | https://github.com/sauravdhr/tnet_python | Dhar et al. (2022) |
LITT | R | SNP matrix and epidemological data | https://github.com/CDCgov/TB_molecular_epidemiology/tree/1.0; | Winglee et al. (2021) |
o2geosocial | R | Epidemiological data (do not include genetic sequences) | https://github.com/alxsrobert/o2geosocial | Robert et al. (2021) |
GraphSNP | R and Java | SNP distance | https://github.com/nalarbp/graphsnp | Permana et al. (2023) |
SOPHIE | Python and MATLAB | Phylogenetic tree and sample meta data | https://github.com/compbel/SOPHIE/ | Skums et al. (2022) |
P-DOR | Python | Assembled genome and SNP phylogeny | https://github.com/SteMIDIfactory/P-DOR | Batisti Biffignandi et al. (2023) |
StrainHub | R | phylogenetic tree and associated metadata | https://github.com/abschneider/StrainHub | de Bernardi Schneider et al. (2020) |
Time-scaled haplotypic density (THD) | R | Genetic distances and user-defined parameters | https://github.com/rasigadelab/thd | Wirth et al. (2020) |
Visualization of transmission network | ||||
Nextstrain | Web application | Nextstrain employs TreeTime to infer time-scaled phylogenies and conduct ancestral sequence inference to determine the likely geographic origins of ancestral nodes | https://nextstrain.org/ | Hadfield et al. 2018 |
Microreact | Web application | Microreact facilitates the exploration of phylogenetic trees as well as spatial and temporal data of samples. Custom datasets can be imported into the application using a Newick tree and sample metadata in tabular format | https://microreact.org/ | Argimon et al. 2016 |
Graphia | Open-source platform | Graphia is a novel visual analytics platform specifically designed for the network-based analysis of large and complex datasets, such as those generated in vast quantities by modern biological analyses | https://graphia.app/ | Freeman et al. 2022 |
All software and tools are freely available for public use under the General Public License version 3.
Tools such as TransPhylo, Quentin, and Phyloscanner can be used to incorporate within-host genomic diversity of strains to infer transmission routes but have certain limitations. For example, TransPhylo uses a time-calibrated phylogeny that takes into account multiple consensus genomes from a single host. However, in the case of a new outbreak, the short timescale involved may make it difficult to generate a clear temporal signal. Quentin and Phyloscanner use different methods to establish transmission links between hosts. Quentin employs graph and network theories to reconstruct within-host phylogenies, while Phyloscanner uses subsample read mapping to generate BAM files. This allows Phyloscanner to identify sub-populations within the host. These within host network reconstruction process might cause biases potentially affecting the accurate distribution of bacterial sub-populations. A comparative analysis was conducted to evaluate the efficacy of tools used in genome-based analysis of TB transmission and proposed Phybreak, Outbreaker2, and TransPhylo are the most effective tools for identifying accurate links in the transmission network derived from TB infection data (Sobkowiak et al. 2023). These tools have demonstrated superior performance in accurately identifying the maximum number of links in the TB transmission network. Study results suggest that these tools could potentially be useful for the development of effective TB control strategies. The accuracy of transmission history inference depends on the rate and extent of genomic heterogeneity (Campbell et al. 2018). Inferring transmission trees from genetic data becomes challenging in pathogens with a high evolutionary rate, primarily due to substantial within-host diversity and genomic dissimilarity among sequenced strains (Morelli et al. 2012). As a result, methods have been developed to integrate both genomic and epidemiological data to infer potential transmission trees (Jombart et al. 2014, Goldstein et al. 2022). According to a recent study, it is crucial to conduct a comprehensive investigation to address potential biases when utilizing a statistical method that combines phylogeny and epidemiological data to analyse TB transmission incidents (Pan et al. 2023a).
Conclusion
The sequencing of the M.tb genomes has revolutionized the field of TB research and positively impacted various aspects of both research and practice. WGS has played a pivotal role in enhancing epidemiological surveillance, facilitating the monitoring of transmission within communities, and tracing the lineage of M.tb across broader geographical and temporal landscapes. The insights derived from the M.tb genome sequencing have potential to accelerate further advancements in the years ahead, as researchers delve into patient-level investigations and employ innovative whole-genome bacteriological methodologies for translational purposes. Emerging NGS strategies facilitate the simultaneous tracing of gene expression patterns while providing comprehensive coverage for studying immune responses to M.tb infections in an efficient manner. Furthermore, the expanding pool of WGS obtained from phenotypically diverse M.tb strains, coupled with advancements in genome-wide association study algorithms, is unlocking the discovery of previously elusive determinants of drug resistance. In TB research, careful selection of genomic pipelines and algorithms has become a critical factor in ensuring standardized WGS results when examining various features of M.tb isolate genomes to investigate genetic heterogeneity, microevolution, and disease transmission events. The study provides systematic information on available software and bioinformatics pipelines that are tailored for Mycobacterium genome analysis, with a focus on emerging NGS approaches and their optimal integration into TB research efforts.
Conflict of interest
The authors declare no conflicts of interest.
Funding
The authors received no specific grant from any funding agency.
Author contributions
Sushanta Deb (Conceptualization, Data curation, Formal analysis, Investigation, Methodology,Writing – original draft), Jhinuk Basu (Investigation, Methodology,Writing – original draft), and Megha Choudhary (Formal analysis, Investigation,Writing – original draft)