-
PDF
- Split View
-
Views
-
Cite
Cite
George Davey Smith, Andrew N Phillips, Correlation without a cause: an epidemiological odyssey, International Journal of Epidemiology, Volume 49, Issue 1, February 2020, Pages 4–14, https://doi.org/10.1093/ije/dyaa016
- Share Icon Share
Background
In the 1980s debate intensified over whether there was a protective effect of high-density lipoprotein cholesterol (HDL-C) or an adverse effect of triglycerides on coronary heart disease (CHD) risk. In a 1991 paper reprinted in the IJE we suggested that the high degree of correlation between the two, together with plausible levels of measurement error, made it unlikely that conventional epidemiological approaches could contribute to causal understanding. The consensus that HDL-C was protective, popularly reified in the notion of ‘good cholesterol’, strengthened over subsequent years. Reviewing the biostatistical and epidemiological literature from before and after 1991 we suggest that within the observational epidemiology pantheon only Mendelian randomization studies—that began to appear at the same time as the initial negative randomized controlled trials—made a meaningful contribution. It is sobering to realize that many issues that appear suitable targets for epidemiological investigation are simply refractory to conventional approaches. The discipline should surely revisit this and other high-profile cases of consequential epidemiological failure—such as that with respect to vitamin E supplementation and CHD risk—rather than pass them over in silence.
Epidemiology in Thatcher’s Britain
In the mid 1980s we were both working on epidemiological studies of cardiovascular disease. A.N.P. was with the British Regional Heart Study (BRHS)1 and G.D.S. had moved, after spells with two Welsh studies,2 to the nascent Whitehall II study.3 Cardiovascular disease epidemiology was focused on identifying new causal factors that could serve as targets for intervention to reduce disease risk. The BRHS was set up to investigate the effects of water hardness on coronary heart disease4 (CHD), the Caerphilly and Speedwell Studies to test the hypothesis that high-density lipoprotein cholesterol (HDL-C) was protective against CHD,5 and the Whitehall II study the role of ‘stress’ (in its broadest sense) in generating social differences in CHD (with the potential mediating role of fibrinogen in this regard being of particular interest6).
The enterprise of identifying novel risk factors for CHD yielded an embarrassment of riches; a 1981 review article documented 246 such factors with supportive evidence.7 The central issue was the meaningfulness of such claims. The introduction of discriminant analysis, logistic regression and Cox proportional hazards models had made multivariable analysis routine,8 and therefore for many of the novel risk factors it was claimed that they were ‘independent’ of confounders, with the implication being that they were likely causal.
HDL-C was at the top of anyone’s list of the most promising novel CHD risk factors in the 1980s. A 1975 paper sparked interest through combining mechanistic evidence with largely ecological epidemiological data to hypothesize a protective effect of HDL-C.9 The foundational epidemiological investigation from 1977 combined data from five studies (including the iconic Framingham investigation) and demonstrated univariable positive associations of low-density lipoprotein cholesterol (LDL-C) and circulating triglyceride with CHD, and an inverse association for HDL-C.10 The authors then performed a multivariable discriminant analysis that led to little change in the LDL-C and HDL-C associations with CHD, but considerable attenuation (and ‘non-significance’11) of the triglyceride association. They concluded:
‘The independence of the association between HDL cholesterol and CHD has been a point of concern because there is a moderate inverse association between HDL cholesterol and triglyceride. In the studies reported here, co-variance among the various lipid factors was controlled both by analysis of cross-tabulations and by discriminant analyses. Both analytic approaches leave no doubt that the inverse association between HDL cholesterol and CHD largely persists even when other lipid factors are considered; that is, knowledge of HDL cholesterol appears to provide risk information beyond that available from the usual lipid risk factors’.10
This logic was reiterated in an influential 1980 New England Journal of Medicine paper that down-graded the role of triglycerides and advocated against their routine measurement.12 That the assessment shifted little over the 30+ years following the 1977 study is shown by a 2009 collaborative meta-analysis, now of much greater size, that presented the data summarized in Figure 1.13 These show the associations of HDL-C and triglyceride with CHD adjusted for age and sex, and then adjusted for additional risk factors, including each other. The considerably more robust nature of the HDL-C–CHD association is made clear in these figures, and echoes the 1977 findings summarized above. The authors concluded that
‘The current findings suggest that therapy directed at HDL-C as well as non-HDL-C may generate substantial additional benefit’.13
Figure 1Hazard ratios for CHD according to level of triglyceride and HDL-C, adjusted just for age and sex. (○) or additionally adjusted for cardiovascular risk factors, including the other lipid (▪). Reprinted from.13 Reproduced with permission from the American Medical Association, USA.
Clearly the notion that raising HDL-C would reduce the risk of CHD remained a popular one in 2009, and its therapeutic targeting was viewed favourably.13
The logic of considering the findings from such studies as demonstrating that the triglyceride association with CHD was confounded by HDL-C, and that the HDL-C association was not due to confounding, could be seen as an application of Cornfield’s inequality,14–16 also referred to as Cornfield’s rule,17 condition18 or law.19 In the debate on whether smoking was a cause of lung cancer, in the late 1950s the biostatistician Jerome Cornfield and colleagues produced the reasoning behind what later became known as sensitivity analysis.16,20 Their analyses explored the extent to which confounding could generate a non-causal association, combining estimates of the relative risk associated with a potential confounder and the prevalence of that confounder amongst smokers and non-smokers. They concluded that ‘no … agent has been found’20 that could account for the smoking–lung cancer association, and implied that it was implausible that such a confounder could exist. Cornfield’s inequality was developed as ‘the Size Rule’ by the statistician Irwin Bross21,22 in a pair of papers that received relatively little attention at the time, although they have recently been seen to be progenitors of modern-day sensitivity analyses and bias-adjustment formulae.
For smoking and lung cancer—the motivating case for both Cornfield and Bross—the inequality rule was highly plausible. However, in less extreme situations measurement error in the assessment of the putative confounder could make it appear insufficient to generate a non-causal exposure–outcome association when it was in fact entirely responsible for it. Although literature dating back to the 1970s at least23 discussed such situations (Box 1), this was not reflected in much of the epidemiological literature at the time, which rarely applied the explicit thinking about the plausibility of confounding accounting for associations that Cornfield and Bross advanced. With some exceptions (e.g.24,25) the additional complexity of measurement error when considering confounding was little addressed. Rather, the apparently statistically ‘independent’ nature of a risk factor following multivariable adjustment was taken as evidence supporting its potential causal impact.
When we wrote our paper the earliest reference we gave with respect to measurement error in confounders was the 1977 Mosteller and Tukey book,23 and the other references we knew on this did not provide earlier sources than this. As a rule any authority identified as having priority for such insights turns out to have been preceded by others, and this is no exception. One such precursor is a paper by the psychologist Robert Thouless published in 193926 , although this was not framed in terms of utilizing estimates for causal understanding. Thouless’ views on parapsychology and his instigation of an experiment to demonstrate communication of the living with the dead (with his post-mortem self as the experimental agent) may have led to neglect of his less eccentric contributions,27 and his paper has only received 19 Google scholar citations.
In 1989 we both attended the 10 day course in Cardiovascular Epidemiology and Prevention (now in its 50th year, and organized by what is currently called the International Society of Cardiovascular Disease Epidemiology and Prevention; ISCEP), where we engaged in many discussions about problematic aspects of cardiovascular epidemiology with Juha Pekkanen. This culminated in a series of sketches the three of us performed on the last evening of the course, when the students ‘entertained’ the lecturers and tutors. The ability of CHD epidemiology to uncover a bewildering and ever-expanding list of apparently ‘independent’ putative causes of the disease was a focus of one of the sketches.
Declaring independence
The HDL-C/triglyceride issue particularly engaged us, and the idea for the paper reprinted in the IJE28 was born. We summarized our thinking (before having carried out the analyses in the paper) in a 1990 editorial29 in which we discussed the general problem of identifying apparently ‘independent’ risk factors as potential causes
‘A further example comes from the study of coronary heart disease. It is now widely believed that serum high density lipoprotein cholesterol (HDL-C), has an important inverse relationship with risk of coronary heart disease. A potentially important confounding factor for this relationship is serum triglyceride level. Triglyceride levels have a strong, positive association with risk of coronary heart disease. Furthermore there is a high inverse correlation between HDL-C and triglyceride. Evidence from prospective studies suggests that the association between HDL-C and risk of coronary heart disease is attenuated only slightly after adjustment for serum triglyceride in a multiple logistic regression model. In contrast the association between serum triglyceride and risk of coronary disease almost disappears after adjustment for HDL-C.
Serum triglyceride concentrations vary considerably during the day, from day to day, and from month to month. This means that if only one measure is taken, it poorly characterises a subject's true, or usual level. In turn, this means that the association between serum triglyceride and risk of coronary heart disease tends to be substantially underestimated. On the other hand, HDL-C is less variable, with a single measure better characterising a subject's usual level. The observed association between HDL-C and risk of coronary disease is therefore less of an underestimate. Due to this imbalance in the degree to which single measures of HDL-C and triglyceride characterise the usual exposure status, the association between HDL-C and the risk of coronary heart disease has been underadjusted for the confounding factor, triglyceride. Similarly the fact that the relationship between triglycerides and coronary disease disappears after adjustment for HDL-C may reflect the strong correlation between triglycerides and HDL-C, and the greater precision of measurement of HDL-C; thus, paradoxically, the situation arises in which measurement of triglyceride is better characterised by a subject's HDL-C level than by a one off direct measurement of triglyceride.
In this situation the disappearance of the association between triglycerides and coronary heart disease may be due to differential error in measurement of the two factors, and not due to triglycerides having no influence on coronary disease risk. Again it must be concluded that prospective studies in which these lipid factors have been measured only once cannot reliably disentangle the separate independent effects of the various lipids. HDL-C may well be an important risk factor in coronary heart disease, and triglycerides may be unimportant, but it would be dangerous to conclude this on the basis of the observational studies alone’. (Quoted from29, with a typo corrected.)
For the paper reprinted in the IJE28 we conducted simulations based on the findings from the BRHS on HDL-C, triglycerides and CHD. These are summarized in Figure 2. Different indices of measurement error—the intra-class correlation between two measurements of the same factor—were modelled. The figures show that, as expected, taking measurement error into account increased the strength of the association of both lipids with CHD, but the effects of mutual adjustment were strikingly dependent on the exact level of measurement error assumed. In the left-most panel, with an intra-class correlation of 0.7 for triglyceride and 0.9 for HDL-C, HDL-C remains the dominant lipid, but reducing the assumed intra-class correlation for triglycerides to 0.6 flips this situation. Changing the intra-class correlation for HDL-C to a small degree, to 0.95, then flips it back again.
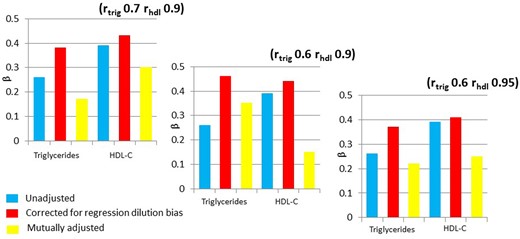
Associations of HDL-C and triglyceride with CHD, with different assumed intra-class correlations, representing the measurement characteristics of the two lipids. The HDL-C effect has been inverted, to allow comparison of the betas, which are per standard deviation of triglycerides and HDL-C. Correction for regression dilution bias takes into account the measurement error in a univariable analysis. In the mutually adjusted models the correlation between the two lipids and measurement error is taken into account. (Figures drawn from data in28).
The instability of these estimates was, to us, both striking and disturbing. The inability to separate postulated effects of correlated exposures in the HDL-C and triglyceride situation appeared little different from the foci of our work, such as on fibrinogen as a potential mediator of socio-economic influences on CHD risk, or on a potential mechanistic role of serum albumin in risk of CHD and a range of other serious outcomes.30 We generalized our initial example31 and aimed to demonstrate how misleading associations could arise through performing conventional epidemiological analyses suggesting that smoking had as large an effect on suicide as it did on cardiovascular disease (Figure 3).32 Ironically, despite the title of our paper being ‘Smoking as ‘independent’ risk factor for suicide: illustration of an artefact from observational epidemiology’, it has generally been cited as suggesting smoking does indeed cause suicide. Biological explanations could, of course, be advanced as counter-arguments to our suggestion that depression-related inability to quit smoking and other confounding factors might have generated the association. Smoking depletes brain serotonin, or affects some other then-fashionable molecule, it was suggested. Our demonstration that smoking was also associated strongly with the risk of being murdered (a ‘negative control’)33 put no dampers on such explanations, although the only straightforward causal explanation we could come up with for the latter finding was that the armed wing of health-promotion agencies had started shooting smokers as the ultimate deterrent.32 We also published on other supposedly obvious spurious ‘effects’ (e.g., more sexual activity reduced male mortality34), critical letters,35 study design suggestions,36 reviews37 and empirical studies of particular supposedly causal epidemiological associations.38 We were, it would seem, on a mission.
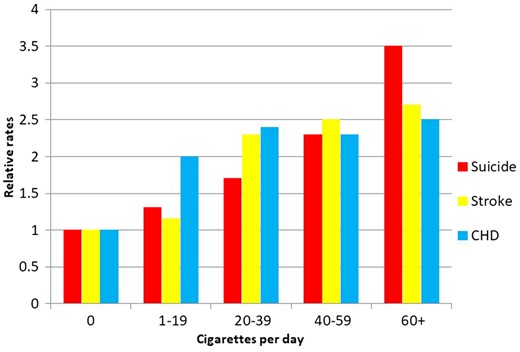
Relative rates of death from suicide, stroke and coronary heart disease (CHD) according to smoking behaviour. (Figure drawn from data in32).
Inconsequential epidemiology
Our paper had no influence whatsoever, probably deservedly so. As one senior epidemiological methodologist complained to G.D.S., it merely reiterated what he (and others) had been saying for years. Despite this, it did influence our choices of research areas on which to dedicate time over the ensuing years. Both of us moved on to work on issues where misinterpreted ‘independent effects’ were not at stake: G.D.S. worked in areas such as HIV/AIDS and sexually transmitted disease interventions in Nicaragua39 and India,40 childhood diarrhoeal disease in Nicaragua41 documenting patterns of health inequities42 and—the inevitable fall-back for one completely devoid of ideas—meta-analysis.43,44 He had embarked on a PhD on fibrinogen as a mediator of the effects of social inequality on cardiovascular disease, but since it was clear that in terms of measurement issues and confounding this was at least as problematic as HDL-C and triglycerides — and was thus probably unanswerable with current methods—he abandoned it. Uncharacteristically this was probably a good move, since it later became clear that fibrinogen was not causal with respect to CHD and thus could not be a mediator45. He continued to moan about the sad state of epidemiology8,46,47(Figure 4). A.N.P. became almost entirely focussed on HIV infection, including cohort studies and randomized controlled trials (RCTs) among people with HIV, studies of transmission, and modelling to try to usefully inform policy (if anybody would listen).
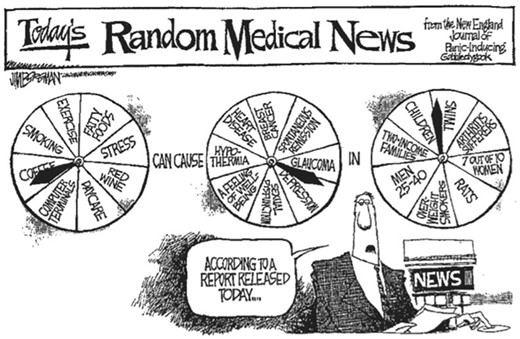
Is this the most widely used cartoon in the epidemiological literature?47 Reproduced with permission from Oxford University Press, UK.
Our paper was published in an epidemiology journal, whereas the epidemiology of greatest impact is published in general medical journals. Consider the perennial issue of alcohol and health: Jackson et al. reported a case-control study in the BMJ purporting to show that moderate alcohol consumption protected against CHD.48 We responded by reanalysing the published summary statistics and, citing the reprinted paper,28 suggested that the findings could well reflect residual confounding.35 The authors were not impressed, saying it ‘was extremely unlikely that the issues raised ... account for [the] protective association between alcohol consumption and CHD’.48 Recent studies using reliable effect estimation strategies suggest these authors were much too sanguine in their conclusions; unfortunately it appears that alcohol consumption does not generate the protective effects imagined.49,50
Two years after our paper appeared, back-to-back papers demonstrating ‘independent’ effects of taking vitamin E supplements on CHD risk in large cohort studies were published in the New England Journal of Medicine,51,52 and (in a manner fully concordant with how the papers were presented) were widely reported as demonstrating substantial benefit of such supplements.53 Use of supplements containing vitamin E shot up to encompass nearly half of the adults in the USA.54 RCTs subsequently demonstrated no discernable protective effect of vitamin E supplementation,55 although it took many years for this to influence usage.56 Similar papers continue to appear in the highest impact general medical journals (as the reader can easily verify by perusing recent issues of such journals at whatever date they are reading this). In response to the intimation that observational studies of vitamin C and vitamin E suggesting substantial protection against CHD reflected confounding57 it was countered that this was not plausible, using arguments akin to (but much weaker than) Cornfield’s better formulated inequality.58 Subsequent work extending our 1991 approach to many confounders demonstrated that confounding was indeed a plausible explanation, both empirically59 and theoretically.60 The same arguments ring on today, defending why probably spurious epidemiological associations should not be attributed to confounding.
The band played on
Writing in 1989 Melissa Austin reviewed the triglyceride, HDL-C and CHD story and concluded that epidemiology had little to offer; the ‘final answer as to whether triglyceride is a causal risk factor for coronary heart disease must come from the biological sciences, and the complexity of the epidemiological results likely reflects the intricate metabolic processes involved’.61 At the time we were distressed by this suggestion that conventional observational epidemiology was essentially worthless with respect to investigating the major (then) contemporary issue in cardiovascular disease epidemiology. However time proved her right, although perhaps not in precisely the way she anticipated.
As we have already discussed, many large-scale epidemiological ventures continued to suggest that modifying HDL-C could ‘generate substantial additional benefits’13 with respect to CHD prevention. Only two approaches proved useful in studying this issue: RCTs and Mendelian randomization (MR).62,63 The first large-scale RCT targeting HDL-C—ILLUMINATE—appeared in 2007, and alarmingly found that such therapy, if anything, elevated cardiovascular disease risk.64 It was suggested that ILLUMINATE had utilized a drug with off-target adverse effects, and that the
‘results of the ILLUMINATE trial have led some observers to question the entire concept of targeting HDL cholesterol therapeutically. However, torcetrapib therapy is just one mechanism for raising levels of HDL cholesterol—and with a flawed molecule to boot. There remains substantial reason for cautious optimism regarding the therapeutic targeting of the metabolism of HDL and reverse cholesterol transport’65
During the period of a post-ILLUMINATE consensus that viewed elevated HDL-C as remaining a target for CHD prevention, the above editorialist responded to a suggestion that genetic data intimated that elevated HDL-C was not causal for CHD66 by stating
‘The field is … in equipoise regarding the effect of Cholesterol Ester Transfer Protein (CETP) inhibition on cardiovascular risk. This question will be definitively answered only with another clinical-outcome trial with a clean CETP inhibitor devoid of off-target effects’.67
Proceeding in parallel with the HDL-C elevating RCTs,68 and building on earlier work,69 MR studies70–74 provided accumulating evidence that HDL-C, raised through several different genetic mechanisms, was not protective against CHD. Together, the substantial evidence that HDL-C elevated by a wide range of pharmaceutical agents or a large number of genetic variants had no benefit has made it clear that circulating levels of HDL-C, in themselves, are not protective with respect to CHD. Given, the above editorialist’s views on the need for an RCT with a ‘clean CETP inhibitor’,67 it is ironic that when a trial employing such an agent managed to reduce CHD75 this was due to the small degree of non-HDL cholesterol-lowering induced by treatment, not to the very substantial increase in HDL-C, which had no effect74,76 (Figure 5). Whether all of the hundreds of millions of dollars invested in trials of HDL-C elevation would have been spent if robust MR evidence had been available at the time of their planning is a topic for speculation.
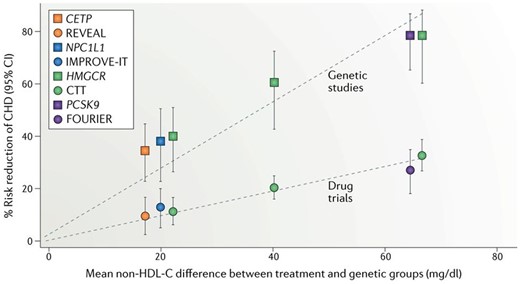
Drug treatment (circles) and genetic proxy (squares) effects on reducing non-HDL-cholesterol levels and the corresponding reduction in risk of coronary heart disease (CHD), from matching drug trials and Mendelian randomization (MR) analyses. As anticipated the trials (lasting ∼5 years) produce about 40% of the risk reduction seen with a lifetime difference in exposure levels, the latter being what MR studies estimate. The CETP inhibitor trial and the MR study are in orange. Note, all of the anticipated effect is seen with reduction in non-HDL-C; the substantial elevation of HDL-C produces no additional benefit. The three values from the Cholesterol Treatment Trialists’ statin collaboration (CTT; green circles) are derived, from left to right, from: five trials of more versus less statin; 17 trials of statin versus placebo with <50 mg/dl average difference in non-HDL-C levels; and four trials of statin versus placebo with >50 mg/dl average difference in non-HDL-C levels. CETP, cholesteryl ester transfer protein; HMGCR, 3-hydroxy-3-methylglutaryl-CoA reductase; NPC1L1, Niemann-Pick C1-like protein 1; PCSK9, proprotein convertase subtilisin/kexin type 9. Reprinted from.74 Reproduced with permission from Springer Nature, USA.
‘Independent risk factors’ in the age of the directed acyclic graph
Here is not the place to detail developments in the teaching and practice of causal inference within epidemiology over the past 30 years. Suffice it to say, many believe there has been a ‘causal revolution’ that has ripped through the corpse that was our pre-modern epidemiology.8,77,78 The introduction of a formal approach to causal inference and use of directed acyclic graphs (DAGs) has certainly been valuable in rendering transportable insights regarding, e.g. how to conceptualize and implement the appropriate control of confounding or how to recognize potential collider bias.79,80 In Box 2 we attempt to reconstruct the sort of schematic formulation (not a formal DAG, but this could in principle be encoded as such) of potential causal pathways linking HDL-C, triglycerides and CHD that could have been constructed in 1991. We then provide a deliberately simplified overview of what is now known about these processes.
Causal diagrams were introduced by the geneticist Sewall Wright around 192081,82 in the form of path diagrams, and whilst these have had some applications in epidemiology83–86 they were relatively little used, despite their popularity in sociology.87 The modern use of causal diagrams in epidemiology could be seen to originate in the work of Robins in the mid-1980s88,89 and then crystalized around the DAG formularization, with discussion and use increasing since the late 1990s.90 Here we give a schematic representation—not as a formal DAG—of how potential causal effects could have been conceptualized in 1991. These various potential pathways could in principle be represented by a series of DAGs, but our aim here is simply to convey the general level of uncertainty that existed when we wrote the paper republished in the IJE.28
The double-headed dashed arrows represent links between correlated measures for which it is uncertain whether there is a causal effect in either direction, or an unknown underlying causal factor that influences them both (i.e. as suggested by double-headed arrows in Wright's path diagrams, when either interpretation could be the case). If underlying causes of CHD influence HDL-C more strongly than triglycerides (which they may not influence at all), then this could generate a strong statistical association between HDL-C and incident CHD (which may be particularly strong if it is the early stages of atherosclerotic disease that influence HDL-C, for example). If asked to draw what are now called DAGs in 1991 we would have found this impossible, because the resolution of these node-to-node links was simply not defensible on appeal to the ‘background knowledge’ that modern causal inference theory indicates is essential for constructing a meaningful DAG.90 Absent nodes and absent arrows in DAGs encode causal assumptions that can invalidate the enterprise.91 For example increased understanding of the role of Apo B in indexing the atherogenicity potential of lipoproteins would lead to a substantial addendum to our vastly over-simplified formulation above.92–94
What is clear is that the implementation of formal rules of causal inference in epidemiological studies has not, in itself, addressed the intrinsic limitations of using epidemiology to try to understand complex causal mechanisms. Thus the same plethora of sad ‘independent effects’ are reported, although now apparently bolstered by a nod towards causal inference and often a DAG95 (see Box 3 of96). Indeed, we find it difficult to believe that methodologies other than RCTs and MR would have converged on a solid answer with respect to the effect on risk of CHD of modifying circulating levels of HDL-C. Certainly a vast number of epidemiological studies, of different types, supported the consensus assessment that HDL-C was protective.
Thinking back to some of the open questions in chronic disease epidemiology when we entered the field—why was stomach cancer incidence declining? What was the major aetiological factor in cervical cancer? Was alcohol protective against CHD? What caused peptic ulcer? Was inflammation important in cardiovascular disease?—to which we could add whether HDL-C was protective against coronary disease—it is noticeable how biological understanding, often unaccompanied by epidemiology, played the major role in advancing knowledge of the kind that could helpfully inform disease prevention or treatment strategies. If the major contribution of the ‘causal revolution’ in epidemiology is seen in terms of how we conceptualize and represent the world a simple test exists: could these ever more clever methods, if applied to the epidemiological data that already existed in considerable volume well before the turn of the 21st century, have produced useful findings on HDL-C and CHD? If applied to the vastly richer databases available today—absent RCTs and MR findings—could researchers using contemporary causal inference tools do any better? A defensible DAG can probably still not be drawn, but this has not hindered the resolution—at least at the pragmatic level—of the epidemiological question regarding HDL cholesterol levels and CHD. More telling is the paucity of papers that present the inability to construct plausible DAGs as the reason why the authors have not generated yet more ‘thousands of pages of research’.97
Acknowledgements
Thanks to Shah Ebrahim, Sander Greenland, Michael Holmes, John Lynch, Caroline Relton and Jonathan Sterne for helpful comments on an earlier draft of this commentary. G.D.S works in the Medical Research Council Integrative Epidemiology Unit at the University of Bristol, which is supported by the Medical Research Council (MC_UU_00011/1).
Conflict of interest: As this commentary is focused on an earlier paper of ours we are clearly highly conflicted and will almost certainly have seen our work through rose-tinted spectacles.
References
The Guardian. https://www.theguardian.com/science/2019/apr/19/david-thouless-obituary (19 April
HPS3/TIMI55-REVEAL Collaborative Group.