-
PDF
- Split View
-
Views
-
Cite
Cite
Laurène Pécuchet, J. Rasmus Nielsen, Asbjørn Christensen, Impacts of the local environment on recruitment: a comparative study of North Sea and Baltic Sea fish stocks, ICES Journal of Marine Science, Volume 72, Issue 5, May/June 2015, Pages 1323–1335, https://doi.org/10.1093/icesjms/fsu220
- Share Icon Share
Abstract
While the impact of environmental forcing on recruitment variability in marine populations remains largely elusive, studies spanning large spatial areas and many stocks are able to identify patterns common to different regions and species. In this study, we investigate the effects of the environment on the residuals of a Ricker stock–recruitment (SR) model, used as a proxy of prerecruits' survival, of 18 assessed stocks in the Baltic and North Seas. A probabilistic principal components (PCs) analysis permits the identification of groups of stocks with shared variability in the prerecruits' survival, most notably a group of pelagics in the Baltic Sea and a group composed of gadoids and herring in the North Sea. The first two PCs generally grouped the stocks according to their localizations: the North Sea, the Kattegat–Western Baltic, and the Baltic Sea. This suggests the importance of the local environmental variability on the recruitment strength. Hence, the prerecruits' survival variability is studied according to geographically disaggregated and potentially impacting abiotic or biotic variables. Time series (1990–2009) of nine environmental variables consistent with the spawning locations and season for each stock were extracted from a physical–biogeochemical model to evaluate their ability to explain the survival of prerecruits. Environmental variables explained >70% of the survival variability for eight stocks. The variables water current, salinity, temperature, and biomass of other fish stocks are regularly significant in the models. This study shows the importance of the local environment on the dynamics of SR. The results provide evidence of the necessity of including environmental variables in stock assessment for a realistic and efficient management of fisheries.
Introduction
Fish stock–recruitment (SR) and, therefore, stock abundance is directly and indirectly influenced by pressures from fishery and environmental forcing, such as temperature (Planque and Frédou, 1999), salinity (Heikinheimo, 2008), and nutrients, such as nitrate and phosphate (Pearson and Rosenberg, 1978; Eero et al., 2011). SR models are essential for analytical stock assessments, and many practical challenges exist in fitting those models and forecasting future recruitment (Hilborn and Walters, 1992; Walters and Martell, 2004). Currently, the majority of the International Council for Exploration of the Sea (ICES) stock assessment and management strategies ignore the environmental forcing and focus on a time–invariant relationship between the stock biomass and the recruitment (www.ices.dk; ICES, 2014a, b, c). However, the size of the spawning-stock biomass alone cannot explain the recruitment variability. In some cases, the recruitment seems to even be independent of the stock biomass (e.g. for Baltic sprat, Margonski et al., 2010; Voss et al., 2012; Gulf of Riga herring, Raid et al., 2010; ICES 2014a, b, c, d). A steady-state SR model may fail to give a robust recruitment estimate under environmentally driven changes of the stock's productivity. In the early 2000s, successive recruitment failures in high biomass stock have been reported in the North Sea (e.g. herring, haddock, Norway pout, and sandeel in ICES, 2006). For those stocks, a traditional SR model would overestimate the recruitment in a forecast, with obvious consequences for fishery management and exploitation. Understanding the environmentally dependent productivity of fish stocks is necessary for the efficient utilization of marine fish resources.
The density-independent variability in recruitment can potentially be investigated by looking at the environmental forcing in the local stock habitat, especially at the spawning grounds. An increasing awareness of the need for an ecosystem approach to fishery management has led to the development of more complex SR models that include environmental considerations, allowing for a better understanding of fish ecology as well as predictions of recruitment strength in a changing environment (Gårdmark et al., 2011). Fish stocks are closely associated with certain distribution areas in certain periods and life stages, especially in spawning and feeding areas and seasons. The environmental variability in these areas (i.e. hydrographic conditions together with food availability and predation pressures) likely influences prerecruit survival (Olsen et al., 2011; Lusseau et al., 2014). The impact of the environmental variability on the recruitment depends on the localization of the stock in the species' distribution range (Myers, 1991). The impact is enhanced at the outer border of the species distribution (Myers, 1998; Brunel and Boucher, 2006). At the core of the distribution, the environmental variability is expected to have a less discernible impact on recruitment, while density-dependence effects become more important (Myers, 1991, 1998). The impacts of environmental variables such as temperature, zooplankton, and larval drift on recruitment have been extensively studied (e.g. Planque and Frédou, 1999; Mackenzie, 2000; Olsen et al., 2011). Other environmental variables have been less frequently studied but have nonetheless shown a significant impact on recruitment, such as oxygen (Köster et al., 2005b; Lindegren and Eero, 2013), salinity (Heikinheimo, 2008), and eutrophication (Pihl et al., 2005).
The main objective of this study is to investigate the potential impacts of various abiotic and biotic factors on the recruitment strength and variability of the North and Baltic Sea stocks assessed by ICES (www.ices.dk). The Baltic Sea is one of the largest brackish water sea areas in the Northeast Atlantic region with important variation in salinity, from the oligohaline waters in the Bothnian Sea (north) to the euhaline waters at the entry of the Kattegat (south) (Figure 1; HELCOM, 2009a). Because of the low salinity, only a few marine species occur in the Baltic region. Three species dominate the fish biomass in the Baltic: cod, herring, and sprat (Ojaveer et al., 2010). Salinity in the Baltic Sea is dependent on the inflows of highly saline and oxygen-rich Atlantic water from the North Sea through the Skagerrak and Kattegat. In low-level inflow years (and subsequent years), salinity can act as a limiting factor on Baltic Sea fish stock dynamics, resulting in lower productivity and greater mortality (Nissling, 2002; Köster et al., 2005a; Heikinheimo, 2008). Additionally, increasing nutrient loads in the Baltic have caused algal blooms and hypoxia over the last few decades (Veer et al., 1989; Rosenberg et al., 1990, 1996; Conley et al., 2002; HELCOM, 2009b). This has led to widespread hypoxic sea areas called “dead zones, ”including parts of the deep basins in the Baltic Sea, which are important spawning areas for some commercial fish species such as cod (HELCOM, 2009a). At salinities lower than 11 psu, cod eggs have negative buoyancy and will sink down to oxygen-depleted layers and die, minimizing the reproductive success (Nielsen et al., 2013). In contrast to the Baltic Sea, the North Sea is a species-rich ecosystem where temperature, rather than salinity, restricts species distributions (Künitzer et al., 1992). Due to increased sea temperature, the North Sea plankton communities have undergone a regime shift, with an increasing occurrence of warm-water zooplankton (Beaugrand et al., 2002; Edwards et al., 2007). Analogous changes have been observed in fish assemblages (Dulvy et al., 2008; Engelhard et al., 2010). These changing environmental conditions have not only affected species distribution (Beare et al., 2004; Perry et al., 2005) but also impacted fish productivity, and especially recruitment, with abnormally low survival rates being documented (North Sea cod, Beaugrand et al., 2003; North Sea herring, Payne et al., 2009).
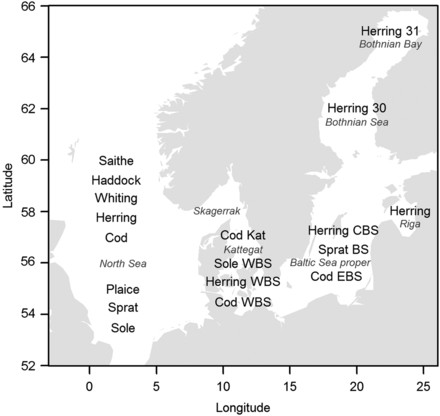
Map of the study area with the approximate centre of distribution of the 18 stocks.
In the present study, a top-down comparative approach is used because the causality and the processes of the impacts of climate, eutrophication, and biotic factors on fish recruitment are not yet fully known. Environment–recruitment studies have often been performed on a single stock basis, but comparative studies on variability in recruitment dynamics between different ecosystem components can help shed light on and determine specific trends according to environmental factors (Mueter et al., 2007; Megrey et al., 2009a). Such comparative studies can be performed by looking at the co-variation of a single-species between different ecosystems (Planque and Frédou, 1999; Brander and Mohn, 2004) or by studying a specific marine ecosystem with possible groupings of analogous species (Brunel and Boucher, 2007; Mueter et al., 2007; Megrey et al., 2009a, b). Because environmental forcing influences recruitment success, species sharing common ecological traits may display coherent spatial and temporal patterns in recruitment determined by the overall environmental fluctuations (Myers, 2001; Mueter et al., 2007). In this study, a comparative approach on both an inter- and intra-ecosystem basis is performed, and the variability in prerecruits' survival and their descriptors are compared between species and between and within the ecosystems. Overall tendencies and similar (or, in certain cases, conflicting) signals between stocks are investigated without explaining the causalities in the processes. To do so, a comparison of the time series of SR residuals, hereafter referred to as prerecruits' survival or survival, is made to identify whether a cross-regional pattern in survival can be identified. Then, the prerecruits' survival is analysed according to potentially impacting hydrographic and biotic variables determining the SR dynamics. The formulated hypotheses on the prerecruits' survival and their co-variability are investigated. These different hypotheses are not mutually exclusive and are consequently discussed as being integrated. The first part of this study will address potential co-variation in recruitment between the different stocks in North and Baltic Sea ecosystem. The null hypotheses are as follows:
H0 (1): There are no overall tendencies in survival at the ecosystem level, i.e. no similar patterns in the stock–recruits' residual anomalies between stocks (test on the significance of a generalized additive model (GAM) on the time series of prerecruits' survival at a p < 0.05 level).
H0 (2): The survival co-variability between stocks is independent of the stocks' geographical distribution (based on the grouping in principal components analysis, PCA, results for all stocks).
The second part of this study will address recruitment in relation to abiotic and biotic variables. The null hypotheses tested are the following:
H0 (3): There is no link between fish SR and biotic or abiotic variables for each assessed stock (based on the significance levels of environmental variables at p < 0.05 and if the percentage of variability in prerecruits' survival explained by the environmental variables is higher than expected by random variables).
H0 (4): There are no common explanatory variables of prerecruits' survival in the stocks of the same species (based on the significant variables of the final statistical model).
Material and methods
Prerecruits' survival derived from SR residuals
SR models were fitted for the different fish stocks using the recruitment (R) and spawning-stock biomass (SSB) from the respective ICES stock assessment working groups (Table 1). The recruitment was defined as the year-class abundance at the age the fish entered the fishery. The recruitment age spans from zero for North Sea haddock to 3 years old for North Sea saithe (Supplementary material, Table S1). In the ICES stock assessments, the SSB and the R are calculated by coupling the different scientific survey and commercial fishery data sampling time series with parallel-sampled age–length keys. However, this approach can induce uncertainty due to incomplete sampling coverage of the age–length keys in the resulting value of the stock numbers per age and, consequently, the SSB. The SSB is therefore calculated from the total stock number at age with the use of weight-at-age keys from the fishery data and a maturity matrix (percentage of the population mature at one age) from the fishery or survey data. This can also bias the results, especially for those stocks where the maturity ogive is temporally fixed in the assessment. In total, 18 stocks are studied, with 8 belonging to the North Sea, and 10 belonging to the Baltic Sea and the Skagerrak–Kattegat regions (Figure 1). Each stock analysed has a specific located spawning ground (and season), which is vertically characterized by the depth and vertical layer of egg occurrence. The scientific literature and other knowledge compiled in the ICES stock annexes associated with the ICES stock assessment working group reports provide this stock-specific information (e.g. spawning area and period, depth distribution of eggs). This information has guided the extraction of the environmental variables used in the present analyses (www.ices.dk; ICES 2014a, c, b; summarized in Supplementary material, Table S1).
Species . | Stock (abbreviation) . | Time-series length . | Assessment model . | Status of the biomass during the 1990–2009 compared with MSY(Btrigger) . | Current status of the stock (2014) . | |
---|---|---|---|---|---|---|
Biomass compared with MSY(Btrigger) . | Fishing pressure compared with F(MSY) . | |||||
CodGadus morhua | North Sea, eastern channel, Skagerrak (NS) | 1963–2011 | SAM (BH)* | Lower | Low | Overfished |
Baltic Sea 22-24 (WBS) | 1970–2011 | SAM (BH)** | Lower | Low | Overfished | |
Baltic Sea 25-32 (EBS) | 1966–2011 | XSA** | Unknown | Unknown | Unknown | |
Kattegat (Kat) | 1980–2011 | SAM* | Unknown | Low | No commercial fishing | |
HerringClupea harengus | Baltic Sea 25-32 (CBS) | 1974–2011 | XSA** | Around MSYB | Good | Not overfished |
Gulf of Riga | 1977–2011 | XSA** | Higher | Good | Not overfished | |
Skagerrak, Kattegat, 22-24 (WBS) | 1990–2011 | SAM*** | Around MSYB | Good | Overfished | |
North Sea (NS) | 1947–2011 | SAM*** | Above Blim | Good | Not overfished | |
Baltic Sea Bothnian Sea (30) | 1973–2011 | SAM** | Around MSYB | Good | Not overfished | |
Baltic Sea Bothnian Bay (31) | 1980–2011 | XSA** | Unknown | Unknown | Unknown | |
PlaicePleuronectes platessa | North Sea (NS) | 1957–2011 | XSA* | Around MSYB | Good | Not overfished |
SoleSolea solea | North Sea (NS) | 1957–2011 | XSA* | Around MSYB | Good | Overfished |
Skagerrak, Kattegat, 22-24 (WBS) | 1984–2011 | SAM** | Lower | Low | Overfished | |
SpratSprattus sprattus | Baltic Sea 22-32 (BS) | 1974–2011 | XSA** | Higher | Good | Overfished |
North Sea (NS) | 1974–2011 | SMS*** | Around MSYB | Good | Not overfished | |
WhitingMerlangius merlangus | North Sea, Eastern Channel (NS) | 1990–2011 | XSA* | Unknown | Unknown | Unknown |
SaithePollachius virens | North Sea, VI, IIIa (NS) | 1967–2011 | XSA* | Around MSYB | Low | Not overfished |
HaddockMelanogrammus aeglefinus | North Sea, Eastern Channel, IIIa (NS) | 1963–2011 | XSA* | Higher | Good | Not overfished |
Species . | Stock (abbreviation) . | Time-series length . | Assessment model . | Status of the biomass during the 1990–2009 compared with MSY(Btrigger) . | Current status of the stock (2014) . | |
---|---|---|---|---|---|---|
Biomass compared with MSY(Btrigger) . | Fishing pressure compared with F(MSY) . | |||||
CodGadus morhua | North Sea, eastern channel, Skagerrak (NS) | 1963–2011 | SAM (BH)* | Lower | Low | Overfished |
Baltic Sea 22-24 (WBS) | 1970–2011 | SAM (BH)** | Lower | Low | Overfished | |
Baltic Sea 25-32 (EBS) | 1966–2011 | XSA** | Unknown | Unknown | Unknown | |
Kattegat (Kat) | 1980–2011 | SAM* | Unknown | Low | No commercial fishing | |
HerringClupea harengus | Baltic Sea 25-32 (CBS) | 1974–2011 | XSA** | Around MSYB | Good | Not overfished |
Gulf of Riga | 1977–2011 | XSA** | Higher | Good | Not overfished | |
Skagerrak, Kattegat, 22-24 (WBS) | 1990–2011 | SAM*** | Around MSYB | Good | Overfished | |
North Sea (NS) | 1947–2011 | SAM*** | Above Blim | Good | Not overfished | |
Baltic Sea Bothnian Sea (30) | 1973–2011 | SAM** | Around MSYB | Good | Not overfished | |
Baltic Sea Bothnian Bay (31) | 1980–2011 | XSA** | Unknown | Unknown | Unknown | |
PlaicePleuronectes platessa | North Sea (NS) | 1957–2011 | XSA* | Around MSYB | Good | Not overfished |
SoleSolea solea | North Sea (NS) | 1957–2011 | XSA* | Around MSYB | Good | Overfished |
Skagerrak, Kattegat, 22-24 (WBS) | 1984–2011 | SAM** | Lower | Low | Overfished | |
SpratSprattus sprattus | Baltic Sea 22-32 (BS) | 1974–2011 | XSA** | Higher | Good | Overfished |
North Sea (NS) | 1974–2011 | SMS*** | Around MSYB | Good | Not overfished | |
WhitingMerlangius merlangus | North Sea, Eastern Channel (NS) | 1990–2011 | XSA* | Unknown | Unknown | Unknown |
SaithePollachius virens | North Sea, VI, IIIa (NS) | 1967–2011 | XSA* | Around MSYB | Low | Not overfished |
HaddockMelanogrammus aeglefinus | North Sea, Eastern Channel, IIIa (NS) | 1963–2011 | XSA* | Higher | Good | Not overfished |
Assessed stocks included in the calculation of the stock–recruit residuals with indication of the extent of the recruitment and spawning-stock biomass time series used. The assessment model used is also indicated alongside the SR model when used in the state-space fish stock assessment. The stock status in terms of SSB during the whole studied period of 1990–2009 as well as the current status of the stock and its fishing pressure are indicated (according to the ICES Advice, 2014; www.ices.dk).
BH, Beverton and Holt; XSA, extended survivor analysis; MSY(Btrigger): a biomass reference point that triggers a precautionary management response within the ICES MSY framework; F(MSY), fishing mortality consistent with achieving maximum sustainable yield (MSY).
ICES 2013. Report of the Working Group on the Assessment of Demersal Stocks in the North Sea and Skagerrak (WGNSSK).
ICES 2013. Report of the Baltic Fisheries Assessment Working Group (WGBFAS).
ICES 2013. Report of the Herring Assessment Working Group for the Area South of 62N (HAWG).
Species . | Stock (abbreviation) . | Time-series length . | Assessment model . | Status of the biomass during the 1990–2009 compared with MSY(Btrigger) . | Current status of the stock (2014) . | |
---|---|---|---|---|---|---|
Biomass compared with MSY(Btrigger) . | Fishing pressure compared with F(MSY) . | |||||
CodGadus morhua | North Sea, eastern channel, Skagerrak (NS) | 1963–2011 | SAM (BH)* | Lower | Low | Overfished |
Baltic Sea 22-24 (WBS) | 1970–2011 | SAM (BH)** | Lower | Low | Overfished | |
Baltic Sea 25-32 (EBS) | 1966–2011 | XSA** | Unknown | Unknown | Unknown | |
Kattegat (Kat) | 1980–2011 | SAM* | Unknown | Low | No commercial fishing | |
HerringClupea harengus | Baltic Sea 25-32 (CBS) | 1974–2011 | XSA** | Around MSYB | Good | Not overfished |
Gulf of Riga | 1977–2011 | XSA** | Higher | Good | Not overfished | |
Skagerrak, Kattegat, 22-24 (WBS) | 1990–2011 | SAM*** | Around MSYB | Good | Overfished | |
North Sea (NS) | 1947–2011 | SAM*** | Above Blim | Good | Not overfished | |
Baltic Sea Bothnian Sea (30) | 1973–2011 | SAM** | Around MSYB | Good | Not overfished | |
Baltic Sea Bothnian Bay (31) | 1980–2011 | XSA** | Unknown | Unknown | Unknown | |
PlaicePleuronectes platessa | North Sea (NS) | 1957–2011 | XSA* | Around MSYB | Good | Not overfished |
SoleSolea solea | North Sea (NS) | 1957–2011 | XSA* | Around MSYB | Good | Overfished |
Skagerrak, Kattegat, 22-24 (WBS) | 1984–2011 | SAM** | Lower | Low | Overfished | |
SpratSprattus sprattus | Baltic Sea 22-32 (BS) | 1974–2011 | XSA** | Higher | Good | Overfished |
North Sea (NS) | 1974–2011 | SMS*** | Around MSYB | Good | Not overfished | |
WhitingMerlangius merlangus | North Sea, Eastern Channel (NS) | 1990–2011 | XSA* | Unknown | Unknown | Unknown |
SaithePollachius virens | North Sea, VI, IIIa (NS) | 1967–2011 | XSA* | Around MSYB | Low | Not overfished |
HaddockMelanogrammus aeglefinus | North Sea, Eastern Channel, IIIa (NS) | 1963–2011 | XSA* | Higher | Good | Not overfished |
Species . | Stock (abbreviation) . | Time-series length . | Assessment model . | Status of the biomass during the 1990–2009 compared with MSY(Btrigger) . | Current status of the stock (2014) . | |
---|---|---|---|---|---|---|
Biomass compared with MSY(Btrigger) . | Fishing pressure compared with F(MSY) . | |||||
CodGadus morhua | North Sea, eastern channel, Skagerrak (NS) | 1963–2011 | SAM (BH)* | Lower | Low | Overfished |
Baltic Sea 22-24 (WBS) | 1970–2011 | SAM (BH)** | Lower | Low | Overfished | |
Baltic Sea 25-32 (EBS) | 1966–2011 | XSA** | Unknown | Unknown | Unknown | |
Kattegat (Kat) | 1980–2011 | SAM* | Unknown | Low | No commercial fishing | |
HerringClupea harengus | Baltic Sea 25-32 (CBS) | 1974–2011 | XSA** | Around MSYB | Good | Not overfished |
Gulf of Riga | 1977–2011 | XSA** | Higher | Good | Not overfished | |
Skagerrak, Kattegat, 22-24 (WBS) | 1990–2011 | SAM*** | Around MSYB | Good | Overfished | |
North Sea (NS) | 1947–2011 | SAM*** | Above Blim | Good | Not overfished | |
Baltic Sea Bothnian Sea (30) | 1973–2011 | SAM** | Around MSYB | Good | Not overfished | |
Baltic Sea Bothnian Bay (31) | 1980–2011 | XSA** | Unknown | Unknown | Unknown | |
PlaicePleuronectes platessa | North Sea (NS) | 1957–2011 | XSA* | Around MSYB | Good | Not overfished |
SoleSolea solea | North Sea (NS) | 1957–2011 | XSA* | Around MSYB | Good | Overfished |
Skagerrak, Kattegat, 22-24 (WBS) | 1984–2011 | SAM** | Lower | Low | Overfished | |
SpratSprattus sprattus | Baltic Sea 22-32 (BS) | 1974–2011 | XSA** | Higher | Good | Overfished |
North Sea (NS) | 1974–2011 | SMS*** | Around MSYB | Good | Not overfished | |
WhitingMerlangius merlangus | North Sea, Eastern Channel (NS) | 1990–2011 | XSA* | Unknown | Unknown | Unknown |
SaithePollachius virens | North Sea, VI, IIIa (NS) | 1967–2011 | XSA* | Around MSYB | Low | Not overfished |
HaddockMelanogrammus aeglefinus | North Sea, Eastern Channel, IIIa (NS) | 1963–2011 | XSA* | Higher | Good | Not overfished |
Assessed stocks included in the calculation of the stock–recruit residuals with indication of the extent of the recruitment and spawning-stock biomass time series used. The assessment model used is also indicated alongside the SR model when used in the state-space fish stock assessment. The stock status in terms of SSB during the whole studied period of 1990–2009 as well as the current status of the stock and its fishing pressure are indicated (according to the ICES Advice, 2014; www.ices.dk).
BH, Beverton and Holt; XSA, extended survivor analysis; MSY(Btrigger): a biomass reference point that triggers a precautionary management response within the ICES MSY framework; F(MSY), fishing mortality consistent with achieving maximum sustainable yield (MSY).
ICES 2013. Report of the Working Group on the Assessment of Demersal Stocks in the North Sea and Skagerrak (WGNSSK).
ICES 2013. Report of the Baltic Fisheries Assessment Working Group (WGBFAS).
ICES 2013. Report of the Herring Assessment Working Group for the Area South of 62N (HAWG).
Assuming a lognormal error structure, the recruitment indices were log-transformed to stabilize the variance. The logarithmic residuals ε represent the unexplained variability in recruitment originating from the environmental variability and/or measurements errors. In the following analyses, the residuals were used as a proxy of the survival of prerecruits. Hence, when the residuals were positive, the survival was higher than expected from the SSB, and the inverse was the case for negative residuals. We fitted the Ricker SR model using the entire available data time series but used the residuals corresponding to the hydrographic dataset covering the 1990–2009 period for the recruitment analyses. The Ricker α parameter was significant for all stocks (not shown), except for cod in the Kattegat. The β parameters were non-significant for the Gulf of Riga and IIIa-Western Baltic herring, Western Baltic cod, and North Sea haddock, whiting and cod. The lack of compensation on the Ricker curve for these six stocks could have arisen from a biomass during the time series that was too low to permit the internal competition that characterizes the compensation effects. This could be especially true for the Western Baltic cod, for which the biomass was low and fluctuating around MSY(Btrigger), which is the biomass reference point that triggers a precautionary management response within the ICES MSY framework (see ICES advice www.ices.dk and Table 1), during the entire time series. For the five other stocks, the biomass was at least two times higher than MSY(Btrigger) during several years.
Investigation of autocorrelation
The autocorrelation in the time series of R and SSB and the SR residuals was not taken into account in this study for several reasons. In an exploratory analysis, the first-degree autocorrelation was integrated in the SR-model, and the AIC of the Ricker models with and without the autocorrelation was compared. Half of the stocks had a first-degree autocorrelation resulting in a significantly lower AIC when the autocorrelation was taken into account (not shown). However, when the time series of the residuals of the models with and without correction for the first-order autocorrelation were compared, only a few minor differences were observed, but the main trends were the same (not shown). Furthermore, the time-series residuals are the result of an incomplete model, as the environmental variables are integrated in a second step and the correction for correlation could hide the impacts of environmental variability. The residual correlation of the final statistical model (Equation (1)) will therefore be checked for autocorrelation. Consequently, to the present study, it was decided not to use the time series corrected for the first-order autocorrelation. This is mainly because we are not interested in the slowly changing, long-term environmental variations for which statistical challenges exist with autocorrelation (Pyper and Peterman, 1998). Instead, we focus on investigating the impact of the year-to-year variability of the environmental variables.
Co-variation of SR residuals between the stocks
The time series of the logarithmic SR residuals were compared between stocks to investigate whether certain cross-regional patterns, for example, co-linearity, for all stocks or groups of stocks were found. Fish species do not have the same amplitude of variability in their recruitment indices, and small pelagic species such as herring and sprat (clupeids) have larger amplitudes than gadoids. Therefore, the residuals were standardized (mean = 0, SD = 1) to enable comparison between the different stocks. A GAM analysis was performed on the North Sea and Baltic Sea stock SR residuals to investigate trends between stocks. A probabilistic PCA (pPCA) was used to explore patterns of co-variability between the different prerecruit survival rates of the stocks. The probabilistic framework permits a PCA to be performed on an incomplete dataset (Tipping and Bishop, 1999). Therefore, a longer time series can be used. Here, the pPCA was used to perform an analysis of data reaching back to the 1980s, although the time series for some stocks began thereafter (western Baltic herring and sole and North Sea whiting).
Environmental variables (ERGOM)
The environmental variables were extracted from the model HBM-ERGOM. This is an advanced three-dimensional hydrodynamic model system that couples an ocean circulation model (HBM) with a bio-geo-chemical model (ERGOM: Ecological ReGional Ocean Model; Neumann, 2000). The HBM model is driven by atmospheric deposition and riverine input, and it simulates the responses of local physics (temperature, salinity, and oxygen) and nutrient loads (N, P) to climatic variability. The ERGOM model has been used to either describe the dynamics of nutrients (Neumann, 2007) or the dynamics of biological compounds when coupled to an individual-based model (Gurkan et al., 2013; Maar et al., 2013). Eilola et al. (2011) compared the model output with observation data for three Baltic Sea ecosystem models, including the ERGOM model, to study the robustness of the model outputs. In general, the nutrient distribution was in agreement with the empirical data. In terms of magnitude, the dissolved inorganic nitrogen (DIN) and the dissolved inorganic phosphorus were well represented for the Baltic. The ERGOM model was initially developed for Baltic Sea studies, but its implementation has been extended to the North Sea (Maar et al., 2011). For the North Sea, Maar et al. (2011) calibrated the model and showed that the validations were generally in “good” to “very good” agreement with observation data of surface salinity, temperature, DIN, phosphate, and chlorophyll a. They also showed good agreement with bottom oxygen concentrations. The coupled HBM-ERGOM model has high resolution in time and space (5 nautical miles horizontally and 77 layers vertically). This model has been chosen for this study because of its high horizontal and vertical resolution, which can more precisely describe the conditions at the spawning grounds experienced by the early-life stages of the investigated fish stocks. It also permits the inclusion of various environmental variables, notably, eutrophication levels, through the nutrient variables (nitrate, phosphate) in the model. However, the outputs of the model were only available for a recent 20-year period (1990–2009), which restricts the stock-by-stock analyses to this contemporary phase. The variable outputs used were as follows: The main spawning grounds of each stock were located and used for the extraction of each environmental variable. The spawning range used may not fully overlap spatially or temporally with that of the critical stage when limiting stages (e.g. egg, larval, or juvenile survival) occupy different habitats. The limiting stages, however, are currently unknown, and the HBM-ERGOM model does not cover the full extent of the spawning grounds of stock with northern distribution in the North Sea, such as the haddock and saithe stocks. This can influence the results due to the extraction of the environmental variables on a non-representative area of the spawning ground. The hydrographical data used were 2-month means corresponding to the peak spawning period for a given stock and extracted at the egg occurrence depth layer. On this basis, a strong assumption is made that prerecruits' survival is mainly driven by the environmental conditions during their early-life stages, particularly during the egg stages. Such a general assumption is justified through several studies (Heath, 1992; Baltic cod and sprat, Köster et al., 2003; Baumann et al., 2006). The output from the hydrodynamic modelling is used in the analysis of prerecruits' survival using the environmental conditions experienced during the first 2 months of the life cycle: egg and early larval development.
- Temperature (°C)
- Oxygen (mmol/m)3
- Salinity (PSU)
- Nutrients (nitrate NO3, phosphate PO4) (mmol/m3)
- Zooplankton (kg dry weight/m3; sum of the micro-zooplankton and meso-zooplankton)
- Chlorophyll A (mmol/m3)
- Current speed (m/s)
- Windstress (N/m2)
Preselection of explanatory variables
In total, there were, on average, 11 abiotic and biotic variables characterizing the environmental conditions experienced by the early-life stages of each stock (Supplementary material, Table S3). With only 20 years of data, these variables had to be reduced in the initial model to avoid co-linearity (i.e. redundancy) in the explanatory variables and to avoid over-fitting of the GAM model (Equation (1)). In a first step, we established a correlation matrix of all the environmental variables extracted at the stock spawning ground. Only variables that correlated with each other with a Pearson coefficient (r) of <0.70 were kept to avoid co-linearity problems. When two variables correlated with r > 0.70, the variable that individually explained the largest part of the deviance of the prerecruits' survival time series was kept. The pairs of variables that were highly correlated were different as a function of the stocks' spawning grounds, but some pairs were more regularly correlated, including oxygen and temperature, chlorophyll and zooplankton, and currents and windstress. Therefore, the preselection of the correlated pairs as a function of the deviance explained preselected out, on average, three variables from the initial pool of 11 (Supplementary material, Table S3). Hence, after this preselection step, an average of eight variables was retained in the initial model (Equation (1)). The HBM-ERGOM output variables were not transformed, whereas the ICES estimated stock biomasses of the hypothetically interacting stocks were log transformed.
Analysis of prerecruits' survival: GAM on a stock basis
A backward selection was used to retain the best model according to the general cross-validation (GCV) score. The GAM was performed in R using the package mgcv (Woods, 2006). The GAM tends to overfit the data (Kim and Gu, 2004). Therefore, the GCV score is penalized by increasing the gamma value that is inherent to its calculation to 1.4 (normally set to 1 as default). A larger γ value permits assigning a higher weight to the number of D.F. of the model in the GCV scores and avoids too large a number of explanatory variables (Kim and Gu, 2004). In the present study, a maximum of four explanatory variables were retained in the final model. If more than four explanatory variables were retained in the backward selection, and even if they were all significant, the variable explaining the least deviance was removed. The data of the biotic and abiotic variables were assumed to have a Gaussian distribution. For the period 1990–2009, a Gaussian distribution of the prerecruits' survival response variable was also assumed. However, with this limited dataset, the assumption of a normal distribution of the survival was violated for four stocks (Kolmogorov–Smirnov test). Consequently, greater precautions in interpreting the results of the survival modelling need to be taken for these stocks. For all statistical methods used, the underlying distribution of the data was tested to investigate the appropriateness of the methods, and the residuals of the final model were checked for normal distribution and autocorrelation.
A significance level test was performed by modelling the prerecruit survival of each stock in function of eight random time series of environmental noise (standardized normal explanatory variables with mean = 0 and SD = 1). This analysis was executed 1000 times (1000 iterations) for each stock, and the mean deviance explained, together with the SD, was extracted for further analysis. This procedure allows us to investigate whether the deviance explained by the environmental variables of the survival in the final model is potentially due solely to random signals in the many environmental variables used in the model (Equation (1)). Eight explanatory variables were generated to correspond to the initial model (Equation (1). This approach allows the determination of whether a combination of four variables will always explain a large part of the survival deviance when the 20 recruits' survival data are modelled in function of eight explanatory variables. The same backward method as in the GAM model (Equation (1)) is used (smoother constrained to a quadratic relationship and a γ value of 1.4).
Results
Co-variation in SR residuals between the stocks
The system-wide prerecruits' survival residuals from the SR models obtained from the North Sea (Figure 2a) and Baltic Sea (Figure 2b) stocks, respectively, appear divergent but with some periodicity for the two ecosystems. In the North Sea, a system-wide pattern among stocks included in this study is noticeable with general recruitment success at the beginning of the 1980s, followed by a period (1990–2000) of recruitment fluctuations among stocks and between years (Figure 2a). During the 2000s, a global below-average survival rate phase is apparent. This phase is especially driven by the low survival of the herring, haddock, and saithe stocks. Overall, it is quite clear that the survival index follows similar trends among stocks, suggesting that environmental forcing may affect the various stocks in similar ways. When analysed altogether, there is a significant (p < 0.01) negative linear relationship between the North Sea stock survival with the intercept in 1990 (white line Figure 2a). The recruitment for all Baltic Sea stocks collectively fluctuated between rather high and low values from the mid-1970s until 1990, after which no clear pattern across stocks is apparent (Figure 2b). As in the North Sea, the early 1980s appears to have been a good recruitment period, with high survival for sprat and Bothnian Bay and Sea herring. In the 2000s, in contrast to the North Sea, the survival rate appears rather spurious for the different Baltic stocks, with no overall pattern or trend. The Baltic Sea pelagic stocks (Figure 2b, blue line) appear to fluctuate consistently and seem to determine the mean survival signal for the combined stocks in the ecosystem (dark line Figure 2b). This is the case until the early 2000s, where they dissociate from each other. There is strong evidence of between-stock shared variability in survival strength with significant overall variation in survival, and therefore, the null hypothesis of no overall prerecruit survival tendencies between the stocks H0(1) is rejected.
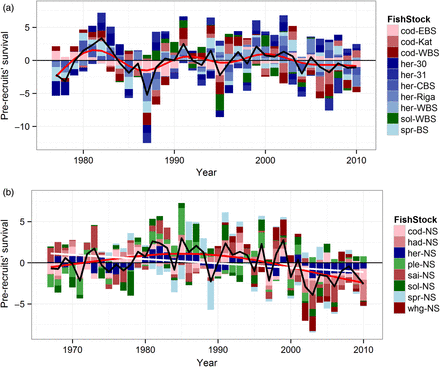
Time series of the standardized residuals of a Ricker SR model for the North and Baltic Sea stocks included for both ecosystems. (a) Standardized prerecruits’ survival for the North Sea stocks from 1967 to 2010. Sprat and Whiting residuals time-series begins in 1974 and 1990, respectively. The smoothed curve is the result of a GAM on all stock–recruit residuals and is shown in red (D.F. = 2.6, p < 0.001). The white regression line represents the significant decreasing prerecruits survival from a linear model of all stocks survival (p < 0.01, not in scale). (b) Standardized prerecruits’ survival for the Baltic Sea stocks from 1977 to 2010. Herring in the Bothnian Bay (31) and sole and herring in the western Baltic time-series residuals begin in 1980, 1984, and 1990, respectively. The smoothed curve is the result of a GAM on all stock–recruit residuals and is shown in red (D.F. = 8.2, p < 0.001). In both graphs, the roundfish species are represented in red colours, the flatfish species in green, and the pelagic species in blue. The black line corresponds to the mean value of the standardized prerecruits’ survival in each ecosystem (not in scale).
Therefore, a pPCA was performed to investigate which stocks have co-varying survival time series and to plot the two principal temporal trends in the overall survival. The two first principal components (PCs) explain 35% of the survival variability for the 18 stocks studied. The first (PC1) and the second (PC2) explain 19.4 and 16%, respectively, of the variability encountered in the stocks' survival time series. The PC1 principally reflects the variability in the North Sea stocks' SR residuals—except the sprat—and the cod and herring stocks in the Kattegat–Western Baltic (Figure 3a). Furthermore, it should be noted that the time series of the PC1 depicts an important negative phase in the survival in the 2000s (Figure 3b). Such a recruitment failure has also been documented in other studies, notably in the North Sea, for some single stocks (e.g. Payne et al., 2009). The PC2 reflects the variability of a group of small pelagic fish in the Baltic Sea and, to a lesser extent, of gadoids and herring in the North Sea (Figure 3a). In contrast to the PC1 time series, there are no pronounced negative or positive phases in the survival (Figure 3c). The bi-plot of the two PCs (loadings of each stock along the PC1 and PC2 axes, Figure 3a) allows us to distinguish groups of co-varying stocks. In general, the stocks from the North or the Baltic Seas are differentiated in the bi-plot. Two groups are especially pronounced in this context: a group of small pelagics in the Baltic Sea (Central Baltic, Gulf of Riga and Bothnian Sea herring and Baltic sprat) and a group of gadoids and herring in the North Sea (saithe, herring, haddock, and whiting). Hence, the null hypothesis H0(2) that the co-variability between stocks is independent of their geographic distribution is rejected.
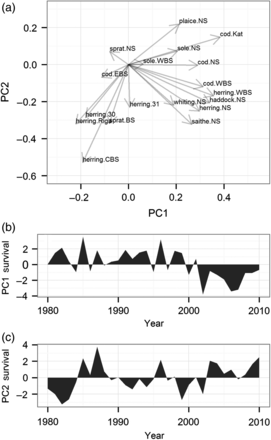
Results of probabilistic PCA identifying common spatial patterns of co-variability. (a) Biplot of the two principal components of a pPCA on all stocks studied for the period 1980–2010, and (b and c) the corresponding PC time series.
Environmental predictors of prerecruits' survival
The percentage of survival explained by the environmental variables in the final model, namely, the model with the best GCV score and no more than four variables, varies between 9.5% for North Sea sole and 89.1% for North Sea whiting (Table 2). For 15 of the 18 stocks studied, >50% of the deviance is explained. The most frequent explanatory variables are the current (which is present in the final model for 13 of 18 stocks), fish stock biomass (10/18), and the salinity (9/18). The current variable is absent in the final model for the two sole stocks and three Baltic Sea herring stocks. The biomass of competitor fish stock variable is absent in the final model for all cod stocks. The salinity variable is present in eight of the ten Kattegat–Baltic Sea stocks, except the sprat and sole, and is absent in the final model for every North Sea stock except sprat. The individual variables that explain most of the deviance in the final stock-specific GAMs are current (the most significant variable in the final model of 4 of 18 stocks: North Sea saithe, haddock and herring, and Baltic Sea sprat), nitrate (4/18: Kattegat and eastern Baltic Sea cod, western Baltic and Bothnian Sea herring) and temperature (4/18: North Sea cod and sole, Bothnian Bay and gulf of Riga herring) (Table 2). Across ecosystems (regions), significant explanatory variables are found for each species except sole in the Baltic Sea. Hence, the null hypothesis H0(3) of no relation between SR and biotic or abiotic variables for each of the assessed stocks is rejected for all stocks except for sole in the Baltic Sea. Certain environmental variables are related to residual recruitment variability for several stocks of the same species, but the relations associated with each variable differ. Among the six herring stocks studied, a few variables are common in the explanatory variables (Table 2). Current and temperature are statistically significant in three of the six stocks, and salinity is significant for four of the five Baltic stocks. For all the Baltic stocks, salinity is retained in the model, but the form of the relationships varies (e.g. Supplementary material, Figure S2). The salinity values are highly different between the stocks, from 12 PSU in the Kattegat–Western Baltic to only two PSU in the Bothnian Bay. Temperature also emerges as an important explanatory variable for the three stocks located in the Northern Baltic Sea (Table 2). Among the four cod stocks studied, only current is significant for all four stocks. The nitrate variable is significant in three of the four stocks, and hence, it seems to impact cod recruitment as well (Table 2). In the two sprat stocks, current is also the only common significant variable. Globally, for the species where different stocks have been studied (cod, herring, and sprat), only current is a significant variable in the stocks of herring and sprat, and no common significant variable is present for the stocks of cod. The null hypothesis H0(4) of no common explanatory variables of prerecruits' survival in the stocks of the same species cannot be rejected.
Species . | Stock . | Final model . | Dev expl. (%) . |
---|---|---|---|
Cod | EBS | NO3*** +PO4** +Current* +Salinity* | 68.6 |
WBS | Salinity*** + Current*** +Oxygen*** +Zooplankton* | 82.4 | |
Kattegat | NO3*** + Current** + Temperature** +SalinityNS | 75.8 | |
NS | Temperature ** + Current* +NO3* +PO4NS | 67.1 | |
Herring | Riga | Temperature **+Herring CBS Biomass* + Salinity NS | 52.4 |
CBS | Zooplankton** +Windstress* +Salinity* +Sprat Biomass* | 57.5 | |
WBS | NO3*** + Current***+Salinity*** + OxygenNS | 86.3 | |
NS | Current*** +Gadoid* +Windstress* | 66.8 | |
30 | NO3***+Temperature*** +Salinity** + PO4* | 84.2 | |
31 | Temperature*** +Salinity*** +Herring 30 Biomass*** + Current* | 88.8 | |
Sprat | BS | Current**+PO4* + NO3NS | 44.6 |
NS | Gadoids Biomass*** + Current*** +NO3*** +Salinty*** | 77.3 | |
Sole | WBS | NS Sole BiomassNS | 9.5 |
NS | Temperature** + Plaice Biomass* + PO4NS +WindstressNS | 60.5 | |
Plaice | NS | PO4*** + Current** +Gadoids Biomass* | 62.5 |
Whiting | NS | Herring*** + Chlorophyll***+Gadoids Biomass** + Current* | 89.1 |
Saithe | NS | Current*** + Chlorophyll***+Zooplankton*** + PO4* | 73.9 |
Haddock | NS | Current* +Herring Biomass* | 40.5 |
Species . | Stock . | Final model . | Dev expl. (%) . |
---|---|---|---|
Cod | EBS | NO3*** +PO4** +Current* +Salinity* | 68.6 |
WBS | Salinity*** + Current*** +Oxygen*** +Zooplankton* | 82.4 | |
Kattegat | NO3*** + Current** + Temperature** +SalinityNS | 75.8 | |
NS | Temperature ** + Current* +NO3* +PO4NS | 67.1 | |
Herring | Riga | Temperature **+Herring CBS Biomass* + Salinity NS | 52.4 |
CBS | Zooplankton** +Windstress* +Salinity* +Sprat Biomass* | 57.5 | |
WBS | NO3*** + Current***+Salinity*** + OxygenNS | 86.3 | |
NS | Current*** +Gadoid* +Windstress* | 66.8 | |
30 | NO3***+Temperature*** +Salinity** + PO4* | 84.2 | |
31 | Temperature*** +Salinity*** +Herring 30 Biomass*** + Current* | 88.8 | |
Sprat | BS | Current**+PO4* + NO3NS | 44.6 |
NS | Gadoids Biomass*** + Current*** +NO3*** +Salinty*** | 77.3 | |
Sole | WBS | NS Sole BiomassNS | 9.5 |
NS | Temperature** + Plaice Biomass* + PO4NS +WindstressNS | 60.5 | |
Plaice | NS | PO4*** + Current** +Gadoids Biomass* | 62.5 |
Whiting | NS | Herring*** + Chlorophyll***+Gadoids Biomass** + Current* | 89.1 |
Saithe | NS | Current*** + Chlorophyll***+Zooplankton*** + PO4* | 73.9 |
Haddock | NS | Current* +Herring Biomass* | 40.5 |
The significance of the explanatory variables included in the final model, that is, the model with the best GCV score and no more than four variables, is represented (***p < 0.001; **p < 0.01; *p < 0.5; NSnot significant). For each stock, the single variable that explains most of the variability is in bold type. The deviance explained by the final model is reported. The bold values correspond to GAMs that perform better than random variables (>55%), and the values bolded and italicized are for GAMs that explain >80% of the recruits' variability.
Species . | Stock . | Final model . | Dev expl. (%) . |
---|---|---|---|
Cod | EBS | NO3*** +PO4** +Current* +Salinity* | 68.6 |
WBS | Salinity*** + Current*** +Oxygen*** +Zooplankton* | 82.4 | |
Kattegat | NO3*** + Current** + Temperature** +SalinityNS | 75.8 | |
NS | Temperature ** + Current* +NO3* +PO4NS | 67.1 | |
Herring | Riga | Temperature **+Herring CBS Biomass* + Salinity NS | 52.4 |
CBS | Zooplankton** +Windstress* +Salinity* +Sprat Biomass* | 57.5 | |
WBS | NO3*** + Current***+Salinity*** + OxygenNS | 86.3 | |
NS | Current*** +Gadoid* +Windstress* | 66.8 | |
30 | NO3***+Temperature*** +Salinity** + PO4* | 84.2 | |
31 | Temperature*** +Salinity*** +Herring 30 Biomass*** + Current* | 88.8 | |
Sprat | BS | Current**+PO4* + NO3NS | 44.6 |
NS | Gadoids Biomass*** + Current*** +NO3*** +Salinty*** | 77.3 | |
Sole | WBS | NS Sole BiomassNS | 9.5 |
NS | Temperature** + Plaice Biomass* + PO4NS +WindstressNS | 60.5 | |
Plaice | NS | PO4*** + Current** +Gadoids Biomass* | 62.5 |
Whiting | NS | Herring*** + Chlorophyll***+Gadoids Biomass** + Current* | 89.1 |
Saithe | NS | Current*** + Chlorophyll***+Zooplankton*** + PO4* | 73.9 |
Haddock | NS | Current* +Herring Biomass* | 40.5 |
Species . | Stock . | Final model . | Dev expl. (%) . |
---|---|---|---|
Cod | EBS | NO3*** +PO4** +Current* +Salinity* | 68.6 |
WBS | Salinity*** + Current*** +Oxygen*** +Zooplankton* | 82.4 | |
Kattegat | NO3*** + Current** + Temperature** +SalinityNS | 75.8 | |
NS | Temperature ** + Current* +NO3* +PO4NS | 67.1 | |
Herring | Riga | Temperature **+Herring CBS Biomass* + Salinity NS | 52.4 |
CBS | Zooplankton** +Windstress* +Salinity* +Sprat Biomass* | 57.5 | |
WBS | NO3*** + Current***+Salinity*** + OxygenNS | 86.3 | |
NS | Current*** +Gadoid* +Windstress* | 66.8 | |
30 | NO3***+Temperature*** +Salinity** + PO4* | 84.2 | |
31 | Temperature*** +Salinity*** +Herring 30 Biomass*** + Current* | 88.8 | |
Sprat | BS | Current**+PO4* + NO3NS | 44.6 |
NS | Gadoids Biomass*** + Current*** +NO3*** +Salinty*** | 77.3 | |
Sole | WBS | NS Sole BiomassNS | 9.5 |
NS | Temperature** + Plaice Biomass* + PO4NS +WindstressNS | 60.5 | |
Plaice | NS | PO4*** + Current** +Gadoids Biomass* | 62.5 |
Whiting | NS | Herring*** + Chlorophyll***+Gadoids Biomass** + Current* | 89.1 |
Saithe | NS | Current*** + Chlorophyll***+Zooplankton*** + PO4* | 73.9 |
Haddock | NS | Current* +Herring Biomass* | 40.5 |
The significance of the explanatory variables included in the final model, that is, the model with the best GCV score and no more than four variables, is represented (***p < 0.001; **p < 0.01; *p < 0.5; NSnot significant). For each stock, the single variable that explains most of the variability is in bold type. The deviance explained by the final model is reported. The bold values correspond to GAMs that perform better than random variables (>55%), and the values bolded and italicized are for GAMs that explain >80% of the recruits' variability.
The normality of the model residuals was confirmed using normal Q–Q plots for most of the stock models, but slight deviations from normality were observed, especially for the North Sea haddock, sole and sprat, and Riga and western Baltic Sea herring. There were no significant correlations in the final model residuals of any of the stocks (significance level p < 0.05). The final models obtained are very sensitive, and sometimes the final explanatory variables that are not statistically significant can be replaced by others without drastically affecting the GCV model score. Only the variable explaining the relatively highest part of the deviance appears robust.
The average deviance of SR residuals explained by random configurations of eight explanatory variables in the GAM framework ranged from 50 to 55%. This high percentage of deviance is explained by the small time series used and by the many explanatory variables. When a time series of only 20 points is used, there is an increasing risk of finding false combinations of significant explanatory parameters when their numbers increase in the initial model. This average of 50–55% explained by random combinations of variables is high and thus justifies the critical evaluation of the results obtained here and in other studies applying similar approaches. Consequently, on average, ∼50–55% of the variability was attributable to random signals in the environment. The best models, however, explained >55% of the residual variability for 15 of 18 stocks, >70% for 8 stocks, and >80% for 5 stocks (Table 2, Figure S2). Therefore, it seems unlikely that the deviance explained in the final models comes exclusively from random noise in the environmental variables, especially for the eight stocks in which a high percentage of deviance is explained. Furthermore, in this analysis the explanatory variables have not been chosen marginally but are known to have potential impacts on the recruitment strength according to the literature.
Discussion
Co-variation between stocks and environmental forcing: ecosystem-scale processes
The aim of this study was to explain the variability in prerecruits' survival of 18 ICES-assessed stocks in the North Sea and the Baltic Sea. The residuals of a Ricker SR model for each of the assessed stocks spread over the Baltic Sea and North Sea were used as a proxy for prerecruits' survival and were analysed with respect to the dependence of potential abiotic or biotic environmental factors and variables. Many studies have analysed recruitment variability and possible environmental explanatory parameters on a single stock basis. The present study is original in its comparative approach to the analysis of several species and different stock survival rates. The biplot of the first two PCs of the stocks' SR residual analysis permitted grouping of the stocks that covariate (Figure 3a). The groups that could be identified generally belong to the same ecoregion. The area-specific partitioning found here can express the pressure of the local environment on the survival rather than geographical large-scale processes, such as the North Atlantic Oscillation (NAO; see Supplementary material, Figure S3 for a correlation test between the NAO and prerecruits' survival). Nonetheless, large-scale processes such as the NAO have been found to affect recruitment through its influence on local environmental variables, such as temperature, salinity, oxygen, and turbulence (e.g. cod, Stige et al., 2006). These results consolidate the sound principle of disaggregated extractions of the environmental variables performed in the present study given the local scale differences.
The study of similar variability in the SR residuals between the stocks leads to the discovery of a recurrent below-average survival for the North Sea stocks since the 2000s (Figure 1). This post-2000 recruitment failure is especially apparent in the PC1 performed on the time series of SR residuals for the 18 stocks studied (Figure 3b). This recruitment failure since the 2000s has already been documented for herring in the North Sea (Payne et al., 2009). In the present study, it is emphasized that this contemporary below-average recruitment is common for several commercially important North Sea and Kattegat–Western Baltic fish stocks, such as North Sea haddock, saithe and whiting, and western Baltic cod and herring. Payne et al. (2009) and Edwards et al. (2007) related the below-average phase of herring recruitment to changes in the plankton community. In our study, only the environmental variable current is significant in the aforementioned six stocks, whereas the zooplankton variable is significant in only two of these stocks.
Influence of environmental variables on recruitment across stocks: nitrate, current, and temperature
The hydrographic and biotic variables considered in this study explained a significant amount of residual variability for 17 of the 18 investigated stocks in the North and Baltic Seas. The environmental variables investigated failed to explain a significant amount of the residual variability in only one case, that is, the Kattegat–Western Baltic Sea sole, with only 9% of the recruits' variability explained. The low variability explained for some stocks can be caused by the assumptions made in the extraction of the variables. Indeed, the hypothesis that the variability in SR residuals is driven by the early-life stages of the stocks, defined by the spatiotemporal constraint of 2-month average and egg layer occurrence in the extraction of the data, may have been too strong for these stocks. An exploration of the critical early-life stages for recruitment would help in extracting more temporally precise environmental data and would give greater insight into the SR dynamics. Nonetheless, in this study, we found significant results with some variables that are regularly present in the final models, particularly the variables that explain most of the variability of the stock recruits' residuals. The environmental variables, and their associated potential ecosystem processes, that best explain recruitment according to the present analyses are related to the variables current, temperature, and nitrate.
An increase in nitrate can enhance primary production and cause harmful and toxic algal blooms (HELCOM, 2009a). This enhancement of nitrate can also lead to the depletion of oxygen in the water and increase the mortality of fish eggs developing in these hypoxic layers, such as for cod stocks in the central Baltic Sea. Of the four cod stocks, the nitrate concentration was significant for three of them, and it was the variable explaining most of the deviance in the final model for the Kattegat and eastern Baltic cod. However, there are two concerns regarding the nitrate variable for the Kattegat and North Sea stocks: first, the relationship is highly influenced by one value; second, the variability was extremely small. Nonetheless, the nitrate concentrations in the Bornholm Basin (central Baltic), which is an important cod spawning area (Nielsen et al., 2013), depicted a negative relationship with the residuals, stressing the potential strong impacts of nutrient loadings and, hence, eutrophication on cod recruitment here. We acknowledge that nitrate may not directly impact the prerecruits' survival, but it is representative as a descriptor for the eutrophication processes. The nutrient concentrations are important in relation to reproduction in cod stocks in the Baltic Sea, as the low salinity constrains the cod to spawn in the deep central Baltic basins, where high nutrient loads and oxygen depletion are more likely to occur (Lehmann and Hinrichsen, 2002; Diaz and Rosenberg, 2008).
Current is the variable that is the most frequent among the explanatory variables of the final models, and it is significant for 11 of the 18 models. It explains most of the variability for the North Sea herring, haddock, saithe, and Baltic sprat. In the present work, the current values corresponded to the vector length of the vertical and horizontal current components; there was no information on the current direction. Here, then, the current variable likely refers to the effect of mixing and turbulence on early-life stages. A higher degree of water mixing can affect the primary production and, consequently, the food availability for fish larvae. Such a scheme can especially be observed in upwelling areas (Cury and Roy, 1989). The water turbulence is consequently expected to affect recruitment, among others, through a higher feeding success of larvae (Mackenzie, 2000). The presence of the current variables in many final models could also indicate the importance of the transport of the early-life stages into higher-quality habitats. Intensive work has been performed in studying larval drift and its potential impacts on recruitment strength. For example, the retention and dispersion of early-life stages from the spawning ground is considered to be one of the key processes impacting fish recruitment (Hinrichsen, 2001). According to Urho (1999), the dispersal of larvae may help in the understanding of SR relationships, and the ability of larvae to reach a suitable nursery habitat may impact year-class strength. Many hydrodynamic models, notably in the Baltic Sea (Hinrichsen, 2001; Hinrichsen et al., 2002), already use the drift of particles to simulate larval drift and to relate the latter to recruitment strength (e.g. Baltic sprat, Baumann et al., 2006). An important goal of this study was to identify potential linkages between the environment and recruitment. Such larval drift models can be used for evaluating SR for stocks that have been shown to depend significantly on current, notably for the gadoids and herring in the North Sea.
Temperature is known to have crucial impacts on fish life history that influence distribution, growth and recruitment. Studies show the differential impacts of temperature variability on recruitment as a function of the distribution range of a single-species stock in an ecosystem (e.g. Atlantic cod in Planque and Frédou 1999; Mantzouni and MacKenzie 2010). In the present study, the temperature variable was significant in the final model for six stocks and was the main explanatory variable for four stocks. Therefore, with an occurrence in only one-third of the stocks, temperature appeared less important than other factors, such as current. This can be because most of the stocks studied are not at their temperature tolerance ranges and distribution borders. Correlations of temperature to recruitment are especially strong at the distribution borders of the species (Myers, 1998). In the present study, only the North Sea cod stock, among the cod stocks investigated, had temperature as the main explanatory variable of the prerecruits' survival. The North Sea cod stock is located at its lower temperature tolerance range and distribution and is confronted with a warmer mean annual temperature than the Baltic Sea cod stocks. For some stocks, this study is in agreement with previous studies that found that temperature significantly impacts recruitment, such as for the North Sea cod (Olsen et al., 2011) and sole (Rijnsdorp et al., 1992) and the Gulf of Riga herring (Cardinale et al., 2009). Nonetheless, for several stocks, we have not found a significant impact of temperature on recruitment, in contrast to other studies, such as for central Baltic sprat (Köster et al., 2003; Margonski et al., 2010), herring (Cardinale et al., 2009), and North Sea herring (Nash and Dickey-collas, 2005). These differences might be due to the inclusion of different explanatory variables in our model, such as nutrients and currents, which ended up as main explanatory variables, whereas the other studies did not considered so many environmental parameters at the same time.
Species with different spawning strategies
In this study, we investigated fish species with different life history traits, including open water and substrate spawners and pelagic or demersal early-life stages. These different species' life history characteristics could trigger different effects of the environment on the survival. The herring stocks are substrate spawners and, therefore, need a specific substrate to depose their eggs. This substrate selectivity can impact the mortality of herring eggs (Rajasilta et al., 1989). The other stocks studied are open water spawners, with the eggs occurring in the upper water layers, except the Baltic Sea cod and sprat due to the salinity restriction (Westin and Nissling, 1991; Karaseva and Ivanovich, 2010), and they do not have this substrate restriction during the egg stages. Furthermore, the variability of the extracted environmental variables from the HBM-ERGOM model for the stocks' specific spawning areas, months, and egg layer depths differ between the different stocks' spawning months and depth. The environmental variables are not expected to have the same impact on these two groups of stocks with different spawning strategies. Chlorophyll, zooplankton, and windstress are environmental parameters that are likely to have a greater influence on stocks with open water spawning strategies because higher values and variability occur in the medium-surface water layers for these parameters (e.g. plankton distribution in the Baltic Sea, Kahru et al., 1984). Inversely, the phosphate and oxygen parameters could be expected to have a greater influence on stocks with eggs occurring at the bottom layer, where the phosphate concentration is higher and oxygen depletion occurs (Eilola et al., 2009). Among the nine stocks of our study having bottom layer (demersal) eggs, only one had a rather unexpected variable influencing recruitment as the single variable that explains most of the variability, that is, zooplankton concentration for CBS herring. The nitrate and the oxygen concentrations, as expected, appeared to have a greater influence on bottom occurring eggs (Table 2).
Fishing pressure on the environment–recruitment relationships
Fish stocks that have been heavily fished will have an eroded age and length structure with a reduced mean age and mean length and, consequently, will be less resilient to environmental variability (Ottersen et al., 2006; Anderson et al., 2008). Fishing depletes the older, larger, and more experienced fish. This can impact the recruitment of stocks with fewer cohorts participating in reproduction (Longhurst, 2002). The variability in recruitment is higher at low population levels (Myers, 2001). Several stocks in our study are currently heavily exploited, and some are overfished, with a biomass under MSY(Btrigger). During the studied period of 1990–2009, many stocks were under or fluctuating around MSY(Btrigger). This was particularly the case for the western Baltic Sea cod and the North Sea cod, plaice and sole stocks (Table 1). North Sea haddock, Baltic sprat, and gulf of Riga herring were the three stocks that had a biomass higher than MSY(Btrigger) for the period 1990–2009, and they were also among three of the four stocks where the environmental variables failed to explain the prerecruits' survival variability (Table 2, deviance explained <55%). In contrast, among the stocks where the environment explained a large part of the survival variability, the biomass was lower, unknown, or fluctuating around MSY(Btrigger) (Table 2, deviance explained >80%). These observations are in agreement with the higher environment–recruitment link when the fishing pressure has eroded the age and length composition of the spawners (Longhurst, 2002; Ottersen et al., 2006) and when the biomass is relatively low (Brander, 2005; Lindegren and Eero, 2013).
Different environmental conditions, described by different environmental regimes, can provoke a change in the productivity of the stock and hence modify the relationship between the spawning-stock biomass and the recruitment (Köster et al., 2009). If an ecosystem shifts towards a less stable and suitable environment for a fish stock, the same spawning biomass will produce less recruitment than under the average conditions before this shift. Hence, under a changing environment, there is an increasing need to integrate the abiotic and biotic factors in the stock assessment to improve the forecast of the stocks' productivity. The present study takes a first step in this direction by clearly showing that for the large majority of stocks, recruitment appears dependent on the environmental variability in the early-life stage habitats. The environment can be integrated into the stock assessment either indirectly by evaluating present or simulating future environmental conditions or directly by integrating environmental variables into a stock–recruitment relationship. In the context of environmentally sensitive stocks, exacerbated by the heavy fishing pressure driving the populations to lower biomass and eroding the age and length structure in the stocks, the integration of the environment into the assessment is an important step to increasing successful fishery management in relation to stocks.
Supplementary data
Supplementary material is available at the ICESJMS online version of the manuscript.
Acknowledgements
This study was funded by the Danish Strategic Research Council Project IMAGE (MAFIA). ASC acknowledges financial support from EU FP7 project OpEc (contract no 283291) for contributing to the present work. We thank Manuel Hidalgo and three anonymous reviewers for their useful comments that greatly improved the quality of the paper.
References
Author notes
Handling editor: Manuel Hidalgo