-
PDF
- Split View
-
Views
-
Cite
Cite
Ryan W Stidham, Deahan Yu, Xinyan Zhao, Shrinivas Bishu, Michael Rice, Charlie Bourque, Vinod V G Vydiswaran, Identifying the Presence, Activity, and Status of Extraintestinal Manifestations of Inflammatory Bowel Disease Using Natural Language Processing of Clinical Notes, Inflammatory Bowel Diseases, Volume 29, Issue 4, April 2023, Pages 503–510, https://doi.org/10.1093/ibd/izac109
- Share Icon Share
Abstract
Extraintestinal manifestations (EIMs) occur commonly in inflammatory bowel disease (IBD), but population-level understanding of EIM behavior is difficult. We present a natural language processing (NLP) system designed to identify both the presence and status of EIMs using clinical notes from patients with IBD.
In a single-center retrospective study, clinical outpatient electronic documents were collected in patients with IBD. An NLP EIM detection pipeline was designed to determine general and specific symptomatic EIM activity status descriptions using Python 3.6. Accuracy, sensitivity, and specificity, and agreement using Cohen’s kappa coefficient were used to compare NLP-inferred EIM status to human documentation labels.
The 1240 individuals identified as having at least 1 EIM consisted of 54.4% arthritis, 17.2% ocular, and 17.0% psoriasiform EIMs. Agreement between reviewers on EIM status was very good across all EIMs (κ = 0.74; 95% confidence interval [CI], 0.70-0.78). The automated NLP pipeline determining general EIM activity status had an accuracy, sensitivity, specificity, and agreement of 94.1%, 0.92, 0.95, and κ = 0.76 (95% CI, 0.74-0.79), respectively. Comparatively, prediction of EIM status using administrative codes had a poor sensitivity, specificity, and agreement with human reviewers of 0.32, 0.83, and κ = 0.26 (95% CI, 0.20-0.32), respectively.
NLP methods can both detect and infer the activity status of EIMs using the medical document an information source. Though source document variation and ambiguity present challenges, NLP offers exciting possibilities for population-based research and decision support in IBD.
Lay Summary
Extraintestinal manifestations of inflammatory bowel disease impact on patient experience, but are poorly captured by electronic health records. Natural language processing systems are capable of not only detecting extraintestinal manifestations, but also inferring activity information by automated analysis of clinical notes.
EIMs are one of several important aspects of IBD that are incompletely captured by administrative and diagnostic coding.
NLP can automatically extract patient-level EIM information and activity using electronic office notes, with accuracy approaching human chart review.
The ability to efficiently collect increasingly granular detail on the course and disease experience will improve the accuracy and personalization of treatment pathways, biomarker development, and precision of the prognosis in IBD.
Introduction
Though ulcerative colitis and Crohn’s disease (CD) principally cause inflammation in the gastrointestinal tract, extraintestinal manifestations (EIMs) are important symptomatic components of inflammatory bowel disease (IBD).1,2 EIMs can impact diverse organ systems including small- and large-joint arthritis (approximately 5%-25% of patients); eye-related inflammatory changes including uveitis, iritis, and episcleritis (5% of patients); and skin conditions like erythema nodosum, psoriasis, and pyoderma gangrenosum (0.5%-11% of patients).3 EIMs have been shown to be associated with the underlying disease mechanisms of IBD and have direct effects on quality of life.4-6 EIMs are associated with clinical outcomes, disease course, the need for biologic medications, future risk of surgery, and increased rate of IBD relapse.7 As a result, society and expert consensus statements suggest incorporating EIMs into therapeutic decision making.3,8
Despite their importance, EIMs remain poorly understood, in part owing to inconsistencies in the descriptions of EIM occurrence. Administrative diagnostic codes for EIMs are unreliable, inaccurate, and fail to convey EIM behavior at a given timepoint.9 The consequences of challenges identifying EIMs are exemplified by the wide variation in estimates of EIM prevalence, with reports ranging between 6% and 50% of patients experiencing at least 1 EIM.10,11 To more comprehensively improve our understanding of EIMs, describe phenotypes, and precisely treat IBD patients, better ways to collect EIM data are needed.
Natural language processing (NLP) presents an opportunity for improved information extraction of EIMs and other clinical detail from documents. NLP is form of artificial intelligence combining machine learning methodologies with text domain knowledge to extract information from documents.12,13 NLP methods have been used in other gastroenterology applications, including systematic extraction of information from endoscopy reports, and as aids in quality assurance and decision making.14,15 NLP has been used to discriminate frequently inaccurate diagnoses based on administrative codes being shown to help clarify specific diagnoses of liver disease.16 NLP methodologies have the potential to extract more detailed information from clinical narratives contained within electronic medical records (EMRs) and may improve our ability to describe patients with IBD at scale.17,18 EIMs are important components of IBD that are challenging to capture using administrative or diagnostic coding. The aim of our study was to develop a proof-of-concept NLP pipeline designed to automatically detect mentions of EIMs and infer EIM activity or status using electronic clinical documents.
Methods
Subject Selection
This retrospective single-center study was approved by the University of Michigan Institutional Review Board. Subject selection criteria included adults 18 years of age or older with a known diagnosis of CD or ulcerative colitis seen between January 1, 2014, and December 31, 2017. IBD diagnosis was verified by the presence of 4 or more International Classification of Diseases–Ninth Revision (ICD-9) and ICD–Tenth Revision (ICD-10) diagnosis codes on separate dates plus 1 or more IBD prescription medication orders.19 One document was used per subject, selecting the first gastroenterology outpatient office visit note available in the EMR (Epic Systems, Verona, WI, USA) to maximize variability. Information on document author type including IBD subspecialist, comprehensive gastroenterologist, advanced practice provider, or trainee was collected to understand the population of authors. Subject demographics, IBD type, disease duration, and medication exposure history were collected. ICD codes related to EIMs were collected from the EMR to evaluate the accuracy of administrative data for EIM identification. To maximize sensitivity, EIM diagnostic codes entered 3 days before and 7 days following the date of the clinical documentation were collected; 1 EIM diagnostic code was sufficient to be considered present.
Source Document Selection and Annotation of Clinical Office Notes for EIM Status
To maximize yield of annotation quality and note variety, a prescreening step identified notes mentioning any EIMs for review by 2 experienced IBD clinician reviewers (M.R., R.W.S.) each with over 10 years’ experience as an IBD clinician. First, reviewers indicated the presence or absence of specific EIMs types mentioned in the documents. Skin-related EIMs included erythema nodosum, pyoderma gangrenosum, psoriasis, and hidradenitis suppurativa. Ocular EIMs included uveitis, episcleritis, and iritis. Arthritis EIMs were also recorded and included spondyloarthropathies and inflammatory arthritis mentions; osteoarthritis and degenerative joint diseases were not included. Several infrequent or difficult to diagnose EIMs (eg, orofacial granulomatosis, cutaneous CD, Sweet’s syndrome, and neutrophilic dermatoses) typically diagnosed by nongastroenterologist specialists were not included in this study. Primary sclerosing cholangitis was not included in the analysis, as it is typically described as present or absent, rather than having a dynamic activity status.
Second, each reviewer was asked to determine specific EIM status description based on their interpretation of clinical documents. While a myriad of EIM descriptions could be annotated, specific EIM status were compressed for feasibility into 6 descriptive classes: negation (statement that an EIM is not present), resolved (statements that an EIM was present but has resolved), historical (only notation of history of an EIM without further comment on current EIM behavior), active-worse (either reported worsening of an EIM symptom and/or moderate-severe symptom intensity), or active-improved (both reported improvement of EIM symptom and/or mild symptom intensity). Finally, an activity-uncertain class was defined as mention of an EIM in which activity or status could not be determined. Reviewers were asked to make a single determination of EIM status for each reviewed document based on their inference of the author’s intention, as EIMs may have several mentions with different statuses in a document. The specific granular EIM statuses were also compressed to a general status classification of active, inactive, or activity-uncertain.
Reviewers labeled a preliminary set of 150 documents for training and to discuss, clarify, and revise EIM status definitions, as well as establish uniformity in handling ambiguous documentation. In the full document set labeled for training and testing, EIM status disagreements were adjudicated by both reviewers based on discussion and consensus; if consensus was not possible EIMs were labeled as the uncertain class. Additional descriptions of EIM and status classifications are included in the Supplemental Methods.
NLP EIM Pipeline Development
The NLP pipeline for predicting EIM status employed 6 steps for document analysis: (1) document preprocessing, (2) identification of EIM keywords and concepts, (3) tokenization of EIM description window and status concept identification, (4) negation detection, (5) EIM document section identification, and (6) document-level EIM status determination. Document preprocessing included identification of sentence boundaries, removal of special characters and superfluous punctuation, and part-of-speech analysis using the Natural Language Tool Kit functions in Scikit-learn Python modules (Figure 1).20 Additional EIM synonyms, abbreviations, and spelling variants were generated using the Unified Medical Language System Metathesaurus to account for high variation in author writing styles.21,22 Because EIM status information may not be contained in the same sentence, a 40-token window surrounding an identified EIM concept was searched for status descriptors. The document section in which an EIM was located was determined by mapping standardized document sections using a local implementation of SecTag.23,24 Standardized document sections included the assessment or plan, history of present illness, physical exam, past medical history, and medications.
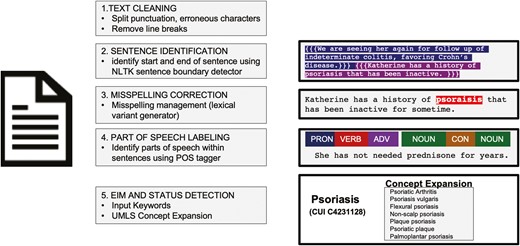
Document preprocessing for natural language processing analysis. Prior to analysis by the extraintestinal manifestation (EIM) natural language processing pipeline, documents were preprocessed to correct common errors and label document structure. First, text was cleaned with removal of erroneous punctuations and symbols, standardization of core punctuation, and removal of carriage returns and line breaks. Individual sentences were identified using a sentence identification tool in the Natural Language Tool Kit (NLTK) package, using punctuation, characters, and other features to identify individual sentences. A part-of-speech (POS) tagger was used to identify components of sentences as nouns, verbs, adverbs, contractions, etc. for aiding in understanding relationships and context. EIM and status concept keywords were expanded upon with an example of psoriasis shown. UMLS, Unified Medical Language System.
In the NLP EIM status classifier, each EIM mention was tagged with its document section and an inferred status (Figure 2). This was done by searching for EIM status descriptor keywords and concepts such as explicit or inferred negation, past EIM history, successful resolution of an EIM, or those indicating an improvement or worsening of an EIM. The NLP pipeline generated a single EIM status for each document. Because multiple EIM mentions can occur in the same document, conflicting EIM statuses were resolved using document section prioritization. Section prioritization order was (1) assessment or plan, (2) history of present illness, (3) past medical history, and (4) physical exam. When EIM status could not be determined, owing to either not detecting a status descriptor or multiple conflicting statuses within the highest priority document section, the NLP pipeline assigned the activity-uncertain label.
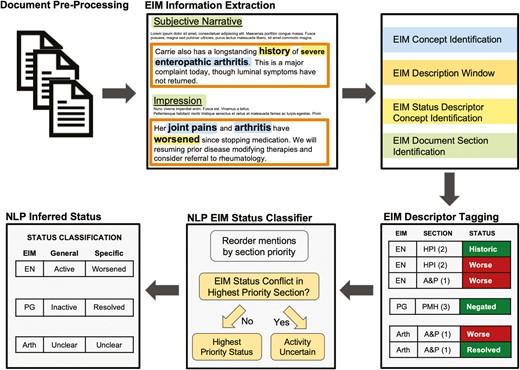
Natural language processing (NLP) document analysis pipeline to detect extraintestinal manifestation (EIM) status. Outpatient gastroenterology notes were extracted from the electronic medical record for identified patients. Document preprocessing includes steps of removal of extraneous characters, tokenization of phases, and part-of-speech labeling. EIM information extraction includes EIM concept identification, followed by an EIM description window. Within the description window, EIM status categories were identified using Unified Medical Language System–based concept expansion. The document section in which the EIM was identified using a SECtag approach. All EIM mentions within a document are then described by the document section it was located in and its status for that mention. The document-level EIM status classifier then relies on a section-priority approach to infer the overall intended EIM status at the point in time the document was written.
Data Analysis
Reviewer performance was reported as paired reviewer detection of EIMs and agreement on general and specific EIM status using Cohen’s kappa statistic for assessing agreement with 95% confidence intervals (CIs). NLP document preparation, processing, and EIM prediction models were implemented in Python 3.6, Scikit-learn, and an in-house clinical text processing pipeline. Proof-of-concept NLP pipeline performance was reported as accuracy, specificity, and sensitivity compared with the adjudicated human reviewer results. NLP pipeline EIM status prediction performance was assessed for both specific EIM status and general EIM status labels.
Results
Subject and Document Characteristics
Of the 4108 patients that met selection criteria, 1240 unique patients were selected for analysis as having 1 (30.2%) or more EIMs mentioned based on the notation of a screening EIM concept keyword. The median age was 41.8 years, men comprised 47.4% of the cohort, and 52.1% of subjects had CD (Table 1). The document dataset comprised 82 different authors, with 11 IBD subspecialists authoring 38.7%, 42 comprehensive nonspecialist gastroenterologists authoring 42.9%, and 29 trainees (fellows, residents, medical students) authoring 18.5% of the document set notes. Biologic exposure occurred in 26.8% of patients, and nearly half had a history of exposure to an immunomodulator. Based on results from reviewers, 1702 unique EIMs were identified in the document set; one note could contain multiple EIMs.
Age, y | 41.8 ± 14.2 |
Male | 588 (47.4) |
IBD type, Crohn’s disease | 646 (52.1) |
Smoking history | 336 (27.1) |
Medication exposure | |
5-ASA | 522 (42.1) |
Thiopurine | 459 (37) |
Methotrexate | 95 (7.7) |
Biologic exposure | |
Adalimumab | 197 (15.9) |
Infliximab | 169 (13.6) |
Certolizumab pegol | 16 (1.3) |
Golimumab | 5 (0.4) |
Vedolizumab | 71 (5.7) |
Ustekinumab | 36 (2.9) |
Age, y | 41.8 ± 14.2 |
Male | 588 (47.4) |
IBD type, Crohn’s disease | 646 (52.1) |
Smoking history | 336 (27.1) |
Medication exposure | |
5-ASA | 522 (42.1) |
Thiopurine | 459 (37) |
Methotrexate | 95 (7.7) |
Biologic exposure | |
Adalimumab | 197 (15.9) |
Infliximab | 169 (13.6) |
Certolizumab pegol | 16 (1.3) |
Golimumab | 5 (0.4) |
Vedolizumab | 71 (5.7) |
Ustekinumab | 36 (2.9) |
Values are mean ± SD or n (%).
Abbreviation: 5-ASA, 5-aminosalicylic acid; IBD, inflammatory bowel disease.
Age, y | 41.8 ± 14.2 |
Male | 588 (47.4) |
IBD type, Crohn’s disease | 646 (52.1) |
Smoking history | 336 (27.1) |
Medication exposure | |
5-ASA | 522 (42.1) |
Thiopurine | 459 (37) |
Methotrexate | 95 (7.7) |
Biologic exposure | |
Adalimumab | 197 (15.9) |
Infliximab | 169 (13.6) |
Certolizumab pegol | 16 (1.3) |
Golimumab | 5 (0.4) |
Vedolizumab | 71 (5.7) |
Ustekinumab | 36 (2.9) |
Age, y | 41.8 ± 14.2 |
Male | 588 (47.4) |
IBD type, Crohn’s disease | 646 (52.1) |
Smoking history | 336 (27.1) |
Medication exposure | |
5-ASA | 522 (42.1) |
Thiopurine | 459 (37) |
Methotrexate | 95 (7.7) |
Biologic exposure | |
Adalimumab | 197 (15.9) |
Infliximab | 169 (13.6) |
Certolizumab pegol | 16 (1.3) |
Golimumab | 5 (0.4) |
Vedolizumab | 71 (5.7) |
Ustekinumab | 36 (2.9) |
Values are mean ± SD or n (%).
Abbreviation: 5-ASA, 5-aminosalicylic acid; IBD, inflammatory bowel disease.
Human Reviewer Detection of EIMs and Agreement on EIM Status
Among the 1702 identified mentions, EIMs were composed of arthritis (54.4%), ocular disease (17.2%), psoriasis (17.0%), erythema nodosum (4.0%), pyoderma gangrenosum (3.8%), and hidradenitis suppurativa (3.8%) EIM types. Overall, reviewer detection of EIM mention was excellent, with both reviewers agreeing on detection in 94.6% of all EIMs (range by EIM type 87.3%-96.6%) (Table 2). Reviewers agreed on exact EIM activity status in 76.2% of EIMs identified (κ = 0.74; 95% CI, 0.70-0.78). Exact agreement on specific activity class varied by EIM type, ranging from very good for arthritis (κ = 0.74) and psoriasis (κ = 0.75) to only fair for hidradenitis suppurativa (κ = 0.45).
Comparison of Paired Human Reviewers on EIM Status Determination . | ||||||
---|---|---|---|---|---|---|
EIM Status . | Reviewer 1 . | |||||
Not Active . | Active . | |||||
Negated . | Historical . | Resolved . | Improved . | Worsened . | Uncertain . | |
Negated | 166 | 2 | 8 | 3 | 1 | 11 |
Historical | 8 | 516 | 11 | 14 | 1 | 108 |
Resolved | 16 | 3 | 63 | 8 | 3 | 12 |
Improved | 2 | 5 | 11 | 94 | 11 | 42 |
Worsened | 2 | 2 | 1 | 12 | 114 | 43 |
Uncertain | 3 | 10 | 10 | 21 | 21 | 344 |
Comparison of Paired Human Reviewers on EIM Status Determination . | ||||||
---|---|---|---|---|---|---|
EIM Status . | Reviewer 1 . | |||||
Not Active . | Active . | |||||
Negated . | Historical . | Resolved . | Improved . | Worsened . | Uncertain . | |
Negated | 166 | 2 | 8 | 3 | 1 | 11 |
Historical | 8 | 516 | 11 | 14 | 1 | 108 |
Resolved | 16 | 3 | 63 | 8 | 3 | 12 |
Improved | 2 | 5 | 11 | 94 | 11 | 42 |
Worsened | 2 | 2 | 1 | 12 | 114 | 43 |
Uncertain | 3 | 10 | 10 | 21 | 21 | 344 |
Comparison of Human Reviewer Detection and Agreement on EIM Status . | ||||||
---|---|---|---|---|---|---|
EIM Type . | Detection Accuracy . | Specific EIM Agreement . | General EIM Agreement . | |||
Accuracy . | Agreement . | Accuracy . | Agreement . | |||
Kappa (95% CI) . | Kappa (95% CI) . | |||||
Arthritis | 96.6% | 0.80 | 0.74 (0.69-0.80) | 0.89 | 0.78 (0.74-0.82) | |
Psoriasis | 90.8% | 0.72 | 0.75 (0.66-0.85) | 0.81 | 0.62 (0.52-0.73) | |
Ocular EIM | 96.4% | 0.70 | 0.69 (0.58-0.80) | 0.85 | 0.71 (0.62-0.78) | |
Erythema nodosum | 87.3% | 0.85 | 0.79 (0.62-0.96) | 0.88 | 0.74 (0.57-0.91) | |
Pyoderma gangrenosum | 92.3% | 0.75 | 0.72 (0.53-0.91) | 0.89 | 0.77 (0.61-0.93) | |
Hidradenitis suppurativa | 96.4% | 0.63 | 0.45 (0.13-0.77) | 0.86 | 0.39 (0.03-0.76) | |
Overall | 94.6% | 0.76 | 0.74 (0.70-0.78) | 0.88 | 0.76 (0.73-0.79) |
Comparison of Human Reviewer Detection and Agreement on EIM Status . | ||||||
---|---|---|---|---|---|---|
EIM Type . | Detection Accuracy . | Specific EIM Agreement . | General EIM Agreement . | |||
Accuracy . | Agreement . | Accuracy . | Agreement . | |||
Kappa (95% CI) . | Kappa (95% CI) . | |||||
Arthritis | 96.6% | 0.80 | 0.74 (0.69-0.80) | 0.89 | 0.78 (0.74-0.82) | |
Psoriasis | 90.8% | 0.72 | 0.75 (0.66-0.85) | 0.81 | 0.62 (0.52-0.73) | |
Ocular EIM | 96.4% | 0.70 | 0.69 (0.58-0.80) | 0.85 | 0.71 (0.62-0.78) | |
Erythema nodosum | 87.3% | 0.85 | 0.79 (0.62-0.96) | 0.88 | 0.74 (0.57-0.91) | |
Pyoderma gangrenosum | 92.3% | 0.75 | 0.72 (0.53-0.91) | 0.89 | 0.77 (0.61-0.93) | |
Hidradenitis suppurativa | 96.4% | 0.63 | 0.45 (0.13-0.77) | 0.86 | 0.39 (0.03-0.76) | |
Overall | 94.6% | 0.76 | 0.74 (0.70-0.78) | 0.88 | 0.76 (0.73-0.79) |
Abbreviations: CI, confidence interval; EIM, extraintestinal manifestation.
Comparison of Paired Human Reviewers on EIM Status Determination . | ||||||
---|---|---|---|---|---|---|
EIM Status . | Reviewer 1 . | |||||
Not Active . | Active . | |||||
Negated . | Historical . | Resolved . | Improved . | Worsened . | Uncertain . | |
Negated | 166 | 2 | 8 | 3 | 1 | 11 |
Historical | 8 | 516 | 11 | 14 | 1 | 108 |
Resolved | 16 | 3 | 63 | 8 | 3 | 12 |
Improved | 2 | 5 | 11 | 94 | 11 | 42 |
Worsened | 2 | 2 | 1 | 12 | 114 | 43 |
Uncertain | 3 | 10 | 10 | 21 | 21 | 344 |
Comparison of Paired Human Reviewers on EIM Status Determination . | ||||||
---|---|---|---|---|---|---|
EIM Status . | Reviewer 1 . | |||||
Not Active . | Active . | |||||
Negated . | Historical . | Resolved . | Improved . | Worsened . | Uncertain . | |
Negated | 166 | 2 | 8 | 3 | 1 | 11 |
Historical | 8 | 516 | 11 | 14 | 1 | 108 |
Resolved | 16 | 3 | 63 | 8 | 3 | 12 |
Improved | 2 | 5 | 11 | 94 | 11 | 42 |
Worsened | 2 | 2 | 1 | 12 | 114 | 43 |
Uncertain | 3 | 10 | 10 | 21 | 21 | 344 |
Comparison of Human Reviewer Detection and Agreement on EIM Status . | ||||||
---|---|---|---|---|---|---|
EIM Type . | Detection Accuracy . | Specific EIM Agreement . | General EIM Agreement . | |||
Accuracy . | Agreement . | Accuracy . | Agreement . | |||
Kappa (95% CI) . | Kappa (95% CI) . | |||||
Arthritis | 96.6% | 0.80 | 0.74 (0.69-0.80) | 0.89 | 0.78 (0.74-0.82) | |
Psoriasis | 90.8% | 0.72 | 0.75 (0.66-0.85) | 0.81 | 0.62 (0.52-0.73) | |
Ocular EIM | 96.4% | 0.70 | 0.69 (0.58-0.80) | 0.85 | 0.71 (0.62-0.78) | |
Erythema nodosum | 87.3% | 0.85 | 0.79 (0.62-0.96) | 0.88 | 0.74 (0.57-0.91) | |
Pyoderma gangrenosum | 92.3% | 0.75 | 0.72 (0.53-0.91) | 0.89 | 0.77 (0.61-0.93) | |
Hidradenitis suppurativa | 96.4% | 0.63 | 0.45 (0.13-0.77) | 0.86 | 0.39 (0.03-0.76) | |
Overall | 94.6% | 0.76 | 0.74 (0.70-0.78) | 0.88 | 0.76 (0.73-0.79) |
Comparison of Human Reviewer Detection and Agreement on EIM Status . | ||||||
---|---|---|---|---|---|---|
EIM Type . | Detection Accuracy . | Specific EIM Agreement . | General EIM Agreement . | |||
Accuracy . | Agreement . | Accuracy . | Agreement . | |||
Kappa (95% CI) . | Kappa (95% CI) . | |||||
Arthritis | 96.6% | 0.80 | 0.74 (0.69-0.80) | 0.89 | 0.78 (0.74-0.82) | |
Psoriasis | 90.8% | 0.72 | 0.75 (0.66-0.85) | 0.81 | 0.62 (0.52-0.73) | |
Ocular EIM | 96.4% | 0.70 | 0.69 (0.58-0.80) | 0.85 | 0.71 (0.62-0.78) | |
Erythema nodosum | 87.3% | 0.85 | 0.79 (0.62-0.96) | 0.88 | 0.74 (0.57-0.91) | |
Pyoderma gangrenosum | 92.3% | 0.75 | 0.72 (0.53-0.91) | 0.89 | 0.77 (0.61-0.93) | |
Hidradenitis suppurativa | 96.4% | 0.63 | 0.45 (0.13-0.77) | 0.86 | 0.39 (0.03-0.76) | |
Overall | 94.6% | 0.76 | 0.74 (0.70-0.78) | 0.88 | 0.76 (0.73-0.79) |
Abbreviations: CI, confidence interval; EIM, extraintestinal manifestation.
When compressing EIM status to more general active vs inactive classification, reviewer agreement was similar to specific status classifications. Reviewers agreed on 85.8% EIM general activity status judgments across all EIMs, constituting very good agreement (κ = 0.76; 95% CI, 0.73-0.79). Again, agreement on general activity status also varied by EIM type, ranging between very good for arthritis activity (89.4%; κ = 0.78) to only fair for hidradenitis suppurativa (86.2%; κ = 0.39). Across all EIM mentions, 10.6% were classified as activity-uncertain by human reviewers due to either insufficient or conflicting EIM status information even after adjudication. Activity-uncertain status ranged from 4.9% (arthritis) to 31.1% (hidradenitis suppurativa). In addition, the dataset was skewed toward inactive EIMs (historic, negated, or resolved EIM mentions), comprising 72.9% of the reviewed EIM instances.
Performance of NLP EIM Status Prediction Compared With Human Reviewers
Compared with human reviewers, automated NLP detection of EIM mentions was nearly perfect, with an accuracy of 97.8% and a sensitivity and specificity of 0.961 and 0.991, respectively, compared with adjudicated human review. The NLP pipeline was unable to determine EIM status in 24.0% of EIMs and ranged from as low as 6.7% for erythema nodosum to 51.6% for hidradenitis suppurativa. NLP and human reviewers agreed on EIM uncertainty status in 61.3% of cases. The 1240 documents were automatically processed by the NLP pipeline in approximately 6 hours, compared with approximately 200 hours (10 minutes per document) required by each human reviewer.
NLP prediction of specific EIM status had fair performance compared with human reviewers across all EIMs, with an overall accuracy, sensitivity, specificity, and agreement of 92.4%, 0.77, 0.95, and κ = 0.77 (95% CI, 0.75-0.79), respectively (Table 3). The accuracy and agreement of the automated NLP pipeline for specific EIM status was similar to the agreement between paired human reviewers with overall EIM classification kappa of 0.77 vs 0.76, respectively. Similar to human reviewers, the automated NLP pipeline performance was lowest for ocular and hidradenitis EIMs (κ = 0.54 and κ = 0.56, respectively).
EIM Type . | Number of Instances . | All EIM Predictions . | |||||
---|---|---|---|---|---|---|---|
Adjudicated Certain . | NLP Certain . | Accuracy . | Sensitivity . | Specificity . | Agreement . | ||
Kappa (95% CI) . | |||||||
Arthritis | 925 | 95.1% | 81.6% | 0.95 | 0.85 | 0.97 | 0.84 (0.81-0.87) |
Psoriasis | 287 | 87.9% | 69.3% | 0.91 | 0.73 | 0.95 | 0.76 (0.70-0.81) |
Ocular EIM | 292 | 78.8% | 67.5% | 0.85 | 0.54 | 0.91 | 0.54 (0.48-0.61) |
Erythema nodosum | 73 | 89.6% | 86.2% | 0.95 | 0.86 | 0.97 | 0.85 (0.77-0.93) |
Pyoderma gangrenosum | 66 | 82.8% | 69.7% | 0.96 | 0.86 | 0.97 | 0.92 (0.86-0.98) |
Hidradenitis suppurativa | 64 | 68.8% | 48.4% | 0.87 | 0.61 | 0.92 | 0.56 (0.37-0.74) |
Overall | 1707 | 89.4% | 75.9% | 0.92 | 0.77 | 0.95 | 0.77 (0.75-0.79) |
EIM Type . | Number of Instances . | All EIM Predictions . | |||||
---|---|---|---|---|---|---|---|
Adjudicated Certain . | NLP Certain . | Accuracy . | Sensitivity . | Specificity . | Agreement . | ||
Kappa (95% CI) . | |||||||
Arthritis | 925 | 95.1% | 81.6% | 0.95 | 0.85 | 0.97 | 0.84 (0.81-0.87) |
Psoriasis | 287 | 87.9% | 69.3% | 0.91 | 0.73 | 0.95 | 0.76 (0.70-0.81) |
Ocular EIM | 292 | 78.8% | 67.5% | 0.85 | 0.54 | 0.91 | 0.54 (0.48-0.61) |
Erythema nodosum | 73 | 89.6% | 86.2% | 0.95 | 0.86 | 0.97 | 0.85 (0.77-0.93) |
Pyoderma gangrenosum | 66 | 82.8% | 69.7% | 0.96 | 0.86 | 0.97 | 0.92 (0.86-0.98) |
Hidradenitis suppurativa | 64 | 68.8% | 48.4% | 0.87 | 0.61 | 0.92 | 0.56 (0.37-0.74) |
Overall | 1707 | 89.4% | 75.9% | 0.92 | 0.77 | 0.95 | 0.77 (0.75-0.79) |
Abbreviations: CI, confidence interval; EIM, extraintestinal manifestation; NLP, natural language processing.
EIM Type . | Number of Instances . | All EIM Predictions . | |||||
---|---|---|---|---|---|---|---|
Adjudicated Certain . | NLP Certain . | Accuracy . | Sensitivity . | Specificity . | Agreement . | ||
Kappa (95% CI) . | |||||||
Arthritis | 925 | 95.1% | 81.6% | 0.95 | 0.85 | 0.97 | 0.84 (0.81-0.87) |
Psoriasis | 287 | 87.9% | 69.3% | 0.91 | 0.73 | 0.95 | 0.76 (0.70-0.81) |
Ocular EIM | 292 | 78.8% | 67.5% | 0.85 | 0.54 | 0.91 | 0.54 (0.48-0.61) |
Erythema nodosum | 73 | 89.6% | 86.2% | 0.95 | 0.86 | 0.97 | 0.85 (0.77-0.93) |
Pyoderma gangrenosum | 66 | 82.8% | 69.7% | 0.96 | 0.86 | 0.97 | 0.92 (0.86-0.98) |
Hidradenitis suppurativa | 64 | 68.8% | 48.4% | 0.87 | 0.61 | 0.92 | 0.56 (0.37-0.74) |
Overall | 1707 | 89.4% | 75.9% | 0.92 | 0.77 | 0.95 | 0.77 (0.75-0.79) |
EIM Type . | Number of Instances . | All EIM Predictions . | |||||
---|---|---|---|---|---|---|---|
Adjudicated Certain . | NLP Certain . | Accuracy . | Sensitivity . | Specificity . | Agreement . | ||
Kappa (95% CI) . | |||||||
Arthritis | 925 | 95.1% | 81.6% | 0.95 | 0.85 | 0.97 | 0.84 (0.81-0.87) |
Psoriasis | 287 | 87.9% | 69.3% | 0.91 | 0.73 | 0.95 | 0.76 (0.70-0.81) |
Ocular EIM | 292 | 78.8% | 67.5% | 0.85 | 0.54 | 0.91 | 0.54 (0.48-0.61) |
Erythema nodosum | 73 | 89.6% | 86.2% | 0.95 | 0.86 | 0.97 | 0.85 (0.77-0.93) |
Pyoderma gangrenosum | 66 | 82.8% | 69.7% | 0.96 | 0.86 | 0.97 | 0.92 (0.86-0.98) |
Hidradenitis suppurativa | 64 | 68.8% | 48.4% | 0.87 | 0.61 | 0.92 | 0.56 (0.37-0.74) |
Overall | 1707 | 89.4% | 75.9% | 0.92 | 0.77 | 0.95 | 0.77 (0.75-0.79) |
Abbreviations: CI, confidence interval; EIM, extraintestinal manifestation; NLP, natural language processing.
Regarding the prediction of general EIM status (active, inactive, uncertain), the NLP pipeline had an accuracy, sensitivity, and specificity of 94.1%, 0.92, and 0.95, respectively, with very good overall agreement to adjudicated human review (κ = 0.76; 95% CI, 0.74-0.79). NLP pipeline agreement with human reviewers ranged from excellent for arthritis (97.2%; κ = 0.84) and erythema nodosum (98.6%; κ = 0.82) to only fair for hidradenitis suppurativa (94.1%; κ = 0.47) (Table 4). Notably, human reviewers had similar poor agreement for hidradenitis suppurativa.
EIM Type . | Number of Instances . | All EIM Predictions . | |||||
---|---|---|---|---|---|---|---|
Adjudicated Certain . | NLP Certain . | Accuracy . | Sensitivity . | Specificity . | Agreement . | ||
Kappa (95% CI) . | |||||||
Arthritis | 925 | 95.1% | 81.6% | 0.97 | 0.91 | 0.98 | 0.84 (0.81-0.88) |
Psoriasis | 287 | 87.9% | 69.3% | 0.93 | 0.93 | 0.92 | 0.72 (0.65-0.79) |
Ocular EIM | 292 | 78.8% | 67.5% | 0.86 | 0.87 | 0.86 | 0.56 (0.48-0.64) |
Erythema Nodosum | 73 | 89.6% | 86.2% | 0.99 | 0.99 | 0.99 | 0.82 (0.67-0.97) |
Pyoderma gangrenosum | 66 | 82.8% | 69.7% | 0.99 | 0.99 | 0.97 | 0.74 (0.63-0.86) |
Hidradenitis suppurativa | 64 | 68.8% | 48.4% | 0.94 | 0.96 | 0.85 | 0.47 (0.28-0.66) |
Overall | 1707 | 89.4% | 75.9% | 0.94 | 0.92 | 0.95 | 0.76 (0.74-0.79) |
EIM Type . | Number of Instances . | All EIM Predictions . | |||||
---|---|---|---|---|---|---|---|
Adjudicated Certain . | NLP Certain . | Accuracy . | Sensitivity . | Specificity . | Agreement . | ||
Kappa (95% CI) . | |||||||
Arthritis | 925 | 95.1% | 81.6% | 0.97 | 0.91 | 0.98 | 0.84 (0.81-0.88) |
Psoriasis | 287 | 87.9% | 69.3% | 0.93 | 0.93 | 0.92 | 0.72 (0.65-0.79) |
Ocular EIM | 292 | 78.8% | 67.5% | 0.86 | 0.87 | 0.86 | 0.56 (0.48-0.64) |
Erythema Nodosum | 73 | 89.6% | 86.2% | 0.99 | 0.99 | 0.99 | 0.82 (0.67-0.97) |
Pyoderma gangrenosum | 66 | 82.8% | 69.7% | 0.99 | 0.99 | 0.97 | 0.74 (0.63-0.86) |
Hidradenitis suppurativa | 64 | 68.8% | 48.4% | 0.94 | 0.96 | 0.85 | 0.47 (0.28-0.66) |
Overall | 1707 | 89.4% | 75.9% | 0.94 | 0.92 | 0.95 | 0.76 (0.74-0.79) |
Abbreviations: CI, confidence interval; EIM, extraintestinal manifestation; NLP, natural language processing.
EIM Type . | Number of Instances . | All EIM Predictions . | |||||
---|---|---|---|---|---|---|---|
Adjudicated Certain . | NLP Certain . | Accuracy . | Sensitivity . | Specificity . | Agreement . | ||
Kappa (95% CI) . | |||||||
Arthritis | 925 | 95.1% | 81.6% | 0.97 | 0.91 | 0.98 | 0.84 (0.81-0.88) |
Psoriasis | 287 | 87.9% | 69.3% | 0.93 | 0.93 | 0.92 | 0.72 (0.65-0.79) |
Ocular EIM | 292 | 78.8% | 67.5% | 0.86 | 0.87 | 0.86 | 0.56 (0.48-0.64) |
Erythema Nodosum | 73 | 89.6% | 86.2% | 0.99 | 0.99 | 0.99 | 0.82 (0.67-0.97) |
Pyoderma gangrenosum | 66 | 82.8% | 69.7% | 0.99 | 0.99 | 0.97 | 0.74 (0.63-0.86) |
Hidradenitis suppurativa | 64 | 68.8% | 48.4% | 0.94 | 0.96 | 0.85 | 0.47 (0.28-0.66) |
Overall | 1707 | 89.4% | 75.9% | 0.94 | 0.92 | 0.95 | 0.76 (0.74-0.79) |
EIM Type . | Number of Instances . | All EIM Predictions . | |||||
---|---|---|---|---|---|---|---|
Adjudicated Certain . | NLP Certain . | Accuracy . | Sensitivity . | Specificity . | Agreement . | ||
Kappa (95% CI) . | |||||||
Arthritis | 925 | 95.1% | 81.6% | 0.97 | 0.91 | 0.98 | 0.84 (0.81-0.88) |
Psoriasis | 287 | 87.9% | 69.3% | 0.93 | 0.93 | 0.92 | 0.72 (0.65-0.79) |
Ocular EIM | 292 | 78.8% | 67.5% | 0.86 | 0.87 | 0.86 | 0.56 (0.48-0.64) |
Erythema Nodosum | 73 | 89.6% | 86.2% | 0.99 | 0.99 | 0.99 | 0.82 (0.67-0.97) |
Pyoderma gangrenosum | 66 | 82.8% | 69.7% | 0.99 | 0.99 | 0.97 | 0.74 (0.63-0.86) |
Hidradenitis suppurativa | 64 | 68.8% | 48.4% | 0.94 | 0.96 | 0.85 | 0.47 (0.28-0.66) |
Overall | 1707 | 89.4% | 75.9% | 0.94 | 0.92 | 0.95 | 0.76 (0.74-0.79) |
Abbreviations: CI, confidence interval; EIM, extraintestinal manifestation; NLP, natural language processing.
Performance of Using Administrative Codes for Defining the Presence or Absence of EIMs
To better understand the value of EIM detection using NLP compared with administrative codes, ICD-10 codes for EIMs entered at the time of the encounter were compared with human annotations for determination of active EIM status. Assuming the presence of a diagnostic code indicates the presence of an active EIM at a given time, administrative data had overall poor performance, with an accuracy, sensitivity, and specificity of 73.3%, 0.32, and 0.83, respectively (Table 5). Agreement between EIM general activity status and the presence or absence of EIM administrative codes was also poor (κ = 0.26; 95% CI, 0.20-0.32). This analysis indicates that using diagnostic codes to measure the presence of EIMs will fail to capture the majority of active EIMs in IBD patients.
Performance of Using Diagnostic Codes to Infer General EIM Activity Compared With Human Reviewers
EIM Type . | Accuracy . | Sensitivity . | Specificity . | Agreement . |
---|---|---|---|---|
Kappa (95% CI) . | ||||
Arthritis | 0.79 | 0.22 | 0.91 | 0.14 (0.03-0.25) |
Psoriasis | 0.53 | 0.62 | 0.48 | 0.09 (0.00-0.20) |
Ocular EIM | 0.74 | 0.16 | 0.98 | 0.17 (0.01-0.33) |
Erythema Nodosum | 0.85 | 0.67 | 0.87 | 0.37 (0.01-0.73) |
Pyoderma gangrenosum | 0.69 | 0.48 | 0.84 | 0.33 (0.10-0.56) |
Hidradenitis suppurativa | 0.69 | 0.71 | 0.62 | 0.25 (0.01-0.49) |
Overall | 0.73 | 0.32 | 0.83 | 0.26 (0.20-0.32) |
EIM Type . | Accuracy . | Sensitivity . | Specificity . | Agreement . |
---|---|---|---|---|
Kappa (95% CI) . | ||||
Arthritis | 0.79 | 0.22 | 0.91 | 0.14 (0.03-0.25) |
Psoriasis | 0.53 | 0.62 | 0.48 | 0.09 (0.00-0.20) |
Ocular EIM | 0.74 | 0.16 | 0.98 | 0.17 (0.01-0.33) |
Erythema Nodosum | 0.85 | 0.67 | 0.87 | 0.37 (0.01-0.73) |
Pyoderma gangrenosum | 0.69 | 0.48 | 0.84 | 0.33 (0.10-0.56) |
Hidradenitis suppurativa | 0.69 | 0.71 | 0.62 | 0.25 (0.01-0.49) |
Overall | 0.73 | 0.32 | 0.83 | 0.26 (0.20-0.32) |
Abbreviations: CI, confidence interval; EIM, extraintestinal manifestation.
Performance of Using Diagnostic Codes to Infer General EIM Activity Compared With Human Reviewers
EIM Type . | Accuracy . | Sensitivity . | Specificity . | Agreement . |
---|---|---|---|---|
Kappa (95% CI) . | ||||
Arthritis | 0.79 | 0.22 | 0.91 | 0.14 (0.03-0.25) |
Psoriasis | 0.53 | 0.62 | 0.48 | 0.09 (0.00-0.20) |
Ocular EIM | 0.74 | 0.16 | 0.98 | 0.17 (0.01-0.33) |
Erythema Nodosum | 0.85 | 0.67 | 0.87 | 0.37 (0.01-0.73) |
Pyoderma gangrenosum | 0.69 | 0.48 | 0.84 | 0.33 (0.10-0.56) |
Hidradenitis suppurativa | 0.69 | 0.71 | 0.62 | 0.25 (0.01-0.49) |
Overall | 0.73 | 0.32 | 0.83 | 0.26 (0.20-0.32) |
EIM Type . | Accuracy . | Sensitivity . | Specificity . | Agreement . |
---|---|---|---|---|
Kappa (95% CI) . | ||||
Arthritis | 0.79 | 0.22 | 0.91 | 0.14 (0.03-0.25) |
Psoriasis | 0.53 | 0.62 | 0.48 | 0.09 (0.00-0.20) |
Ocular EIM | 0.74 | 0.16 | 0.98 | 0.17 (0.01-0.33) |
Erythema Nodosum | 0.85 | 0.67 | 0.87 | 0.37 (0.01-0.73) |
Pyoderma gangrenosum | 0.69 | 0.48 | 0.84 | 0.33 (0.10-0.56) |
Hidradenitis suppurativa | 0.69 | 0.71 | 0.62 | 0.25 (0.01-0.49) |
Overall | 0.73 | 0.32 | 0.83 | 0.26 (0.20-0.32) |
Abbreviations: CI, confidence interval; EIM, extraintestinal manifestation.
Discussion
In this proof-of-concept study, we show the potential for using NLP analysis of clinical narratives to offer more detail describing EIMs in IBD. NLP outperforms diagnostic codes for both detecting EIMs and inferring their status. EIM detection and agreement was generally very good and demonstrated similar performance to the agreement between human reviewers. In addition, automated methods could estimate specific characterizations of EIMs with good performance. A significant number of EIM references did not have a discernable status, owing to either insufficient information or conflicting statuses that could not be resolved even following human adjudication. In situations in which EIM documentation was not ambiguous, automated methods were capable of specific EIM information extraction tasks.
Automated NLP methods did not perfectly replicate human performance and more frequently were unable to make a status determination: why? Common factors impacting NLP ability to detect EIM status included separation of the EIM with status descriptors referenced in distant parts of the document. In addition, EIM status would often be conflicting within the same document section, particularly in scenarios when the author referenced past, present, and possible futures of EIMs behavior within the same section. The NLP pipeline was not designed to specifically detect temporal or anatomic references. As a result, an EIM could be active in one anatomic location but resolved in another, presenting both a prediction and annotation challenge. NLP methods are often challenged on hedging and speculation by authors, as language cues fail to clearly convey whether a phrase is describing the current moment or a possible future.
In addition, though the section-priority method frequently resolves EIM status conflicts, we found that the optimal prioritization of document sections was not static. The priority or importance of document sections may itself be dynamic, with different EIMs or clinical scenarios being better served by context-driven section priorities. Determining the optimal priority level for the history of present illness vs physical exam vs assessment and plan document sections based on clinical scenario will be investigated in future work.
Some EIMs, including ocular and dermatologic EIMs, used unique descriptors of activity that were inconsistent and contributed to NLP status prediction failures. Finally, often authors would alternate between specific EIMs and general references that were undetected by the NLP pipeline. For example, an author may reference psoriasis without commenting on its behavior but in the next paragraph of text reference a rash containing status information indicating worsening. While a human reviewer can easily infer that the rash is referencing the psoriasis, NLP pipelines are challenged to connect those concepts and ensure that the co-reference is correct.
Enhanced detail on individual patients using existing text found in the EMR could substantially impact population-based research on EIMs and other concepts in IBD. As an example, studies examining the associations EIMs among anti-integrin vs anti-tumor necrosis factor (TNF) users have had conflicting results using clinical trial data compared with real world administrative datasets.25-28 Ananthakrishnan et al9 used NLP to identify arthritis-related EIMs, reporting that vedolizumab users experienced more arthritis-related EIMs compared with anti-TNF users (46.1% vs 28.5%; P = .002). As in our study, they found that the sensitivity and specificity of NLP for detecting arthritis EIMs (0.83-0.92) was superior to ICD-9 diagnostic codes (0.52-0.89). In other work, NLP analysis of clinical notes doubled the detection of anti-TNF biologic use, increased detection of patients with fistula (12% vs 36%) or strictures (25% vs 40%), and improved detection of surgeries and hospitalizations compared with using medication lists, problem lists, and administrative data within a health system EMR.29,30 These works highlight the added value of NLP over existing data sources for understanding granular features of disease how NLP information can modify outcome assessment, and the potential for future detailed datasets at population scale.
Several limitations impact immediate implementation of NLP applications and should be considered when interpreting these results. First, NLP pipelines must be generalizable, accounting for the myriad of variation in documentation styles, templates, and author experience for broad applicability. Our work incorporated over 80 different authors, helping support potential generalizability of NLP performance, though this is not a substitute for formal validation using documents from multiple medical centers. Second, while our document set likely reflected real-world EIM prevalence, the EIM types collected were unbalanced, with a strong bias toward inactive status classifications. Generating a balanced dataset would require the reading of tens of thousands of documents, which was not feasible at this proof-of-concept phase.
Though clinical documentation is often considered ground truth, the information in notes may not be reliable. The aim in the study was evaluate NLP accuracy and performance for extracting EIM detail as documented by clinician, though this does not ensure the “correctness” of the information found in the note. However, NLP could conceivably help validate diagnoses and reported assessments in individual notes by examining longitudinal sets of documents for clinical information such as EIMs. Future work will aim to utilize a more diverse set of documents, improve dataset balance of EIM type and status, and explore methods for EIM diagnosis verification.
Though in its infancy, we expect a near future where NLP is used to automatically collect and organize complex clinical descriptions of patients with IBD. NLP pipelines similar to those presented here are methodologically agnostic, with expectations of near identical performance in common EMR environments such as Cerner, Epic Systems, CPRS, and others. What will be required to make NLP systems a practical reality is rigorous validation of performance across a wide variety of authors by experience level, location, and local healthcare information technology environments. Present experimentation with NLP tools requires centers have expertise and resources for complex bioinformatics and specific resources for computational linguistics. However, in time NLP toolkits will be easily deployed within healthcare systems and are likely to be directly integrated into the EMR with minimal tuning and optimization required.
Conclusions
We demonstrate the performance of a pilot system aimed to both detect the presence and describe the status of EIMs in IBD. Analyses of population data currently using diagnostic, procedural, and admission codes are hindered by the absence of granular disease descriptors. NLP analysis of ubiquitous clinical documents stored in EMRs could capture the nuanced data separating the experiences of individual IBD patients. Beyond descriptions of EIMs explored in this article, NLP systems could be designed to extract other useful clinical detail regarding phenotype, medication use and tolerance, and prior history. Expect NLP tools to have a major impact in IBD care and researcher over the coming years.
Author Contributions
R.W.S. was involved in study design, data collection, data abstraction, statistical analysis, manuscript drafting. D.Y. was involved in data science and engineering, statistical analysis, manuscript drafting. X.Z. was involved in data science and engineering, statistical analysis, manuscript drafting. S.B. was involved in data collection, data abstraction, manuscript drafting. M.R. was involved in data collection, data abstraction, manuscript drafting. C.B. was involved in data collection, data abstraction, manuscript drafting. V.G.V.V. was involved in study design, data collection, data science and engineering, statistical analysis, manuscript drafting.
Funding
R.W.S. and V.G.V.V. received investigator-initiated study funding support from AbbVie.
Conflicts of Interest
R.W.S. has served as a consultant for, advisory board member for, or received research grants from AbbVie, Janssen, Takeda, Gilead, Eli Lilly, Merck, Exact Sciences, and CorEvitas. All remaining authors have no conflicts of interest relevant to this publication.