-
PDF
- Split View
-
Views
-
Cite
Cite
Claudio Fiocchi, Dimitrios Iliopoulos, IBD Systems Biology Is Here to Stay, Inflammatory Bowel Diseases, Volume 27, Issue 6, June 2021, Pages 760–770, https://doi.org/10.1093/ibd/izaa343
- Share Icon Share
Abstract
Systems biology is a rapidly advancing field of science that allows us to look into disease mechanisms, patient diagnosis and stratification, and drug development in a completely new light. It is based on the utilization of unbiased computational systems free of the traditional experimental approaches based on personal choices of what is important and what select experiments should be performed to obtain the expected results.
Systems biology can be applied to inflammatory bowel disease (IBD) by learning basic concepts of omes and omics and how omics-derived “big data” can be integrated to discover the biological networks underlying highly complex diseases like IBD. Once these biological networks (interactomes) are identified, then the molecules controlling the disease network can be singled out and specific blockers developed.
The field of systems biology in IBD is just emerging, and there is still limited information on how to best utilize its power to advance our understanding of Crohn disease and ulcerative colitis to develop novel therapeutic strategies. Few centers have embraced systems biology in IBD, but the creation of international consortia and large biobanks will make biosamples available to basic and clinical IBD investigators for further research studies.
The implementation of systems biology is indispensable and unavoidable, and the patient and medical communities will both benefit immensely from what it will offer in the near future.
INTRODUCTION
Searching the PubMed database for terms representing specific or general topics related to basic, translational, and clinical biomedicine provides objective information not only on how much attention a specific topic receives and is therefore perceived as important but also on how science progresses, takes new directions, and evolves over time. Accepting this premise and searching for the term “systems biology” in PubMed reveals a steady and rapid rise in the number of publications from around 1000 in the 1990s to 17,309 in 2019 and >11,000 by mid-2020. These publications cover a wide variety of topics, with cancer being the overwhelmingly dominant one, but they also include many other conditions, such as inflammatory bowel disease (IBD), which started with few sporadic reports around 2000 and had only 69 publications in 2019.
Is this a sign that systems biology is not important for IBD, or has it yet to catch the attention of the IBD community, which feels more at ease with classical biomedical studies and is largely unacquainted with advanced computational studies? The second possibility is most certainly the case, but recent trends are encouraging. In fact, a progressively increasing number of basic science manuscripts have been submitted to this journal in the last 5 years, from 3.7% in 2016, to 5.1% in 2017, 6.5% in 2018, 4.5% in 2019, and a jump to a predicted >20% by the end of 2020. This trend is not only a clear sign of incoming changes but more so of needed innovation, both of which are highly desirable considering the enormous challenges that IBD still poses to basic and clinical investigators alike. This review addresses the definition of systems biology, why it is needed in IBD, how it can be used, what can be expected from it, and how the IBD community can engage it.
WHAT IS SYSTEMS BIOLOGY?
The word “system” can be popularly defined as “a regularly interacting or interdependent group of items forming a unified whole” (https://en.wikipedia.org/wiki/System) or “a set of detailed methods, procedures and routines created to carry out a specific activity, perform a duty, or solve a problem” (http://www.businessdictionary.com/definition/system.html). Therefore, contained in the word “system” are 2 key concepts: one, that of multiple components interacting among themselves and second, that of the need for their functional integration to find a solution. When these 2 basic concepts are applied to biological issues, “systems biology” emerges as the “computational and mathematical analysis and modeling of complex biological systems using a comprehensive, as opposed to reductionist, approach to biological research” (https://en.wikipedia.org/wiki/Systems_biology). Systems biology was born in 2001 when it first appeared in a publication by Ideker et al1 as a new approach to decoding life. Subsequently, systems biology acquired a variety of other less dramatic meanings, becoming a household term in multiple fields of biology and medicine.2 In fact, systems biology does not stand alone but in the company of other comparable systems approaches, such as systems medicine and systems pharmacology.3 Although these systems are fundamentally conceptual, there is a need for proper tools to implement them at a practical level; these tools are provided by the field of bioinformatics. Bioinformatics is an interdisciplinary field that develops methods and software tools for understanding biological data, in particular when the data sets are large and complex (https://en.wikipedia.org/wiki/Bioinformatics), as in the case of IBD and countless other disorders of a neoplastic or inflammatory nature.4 Armed with these notions and tools, this review considers why systems biology is needed in IBD, how it can be used, and what to expect from it.
THE NEED OF SYSTEMS BIOLOGY IN IBD
The impulse to create new approaches to elucidate unresolved issues in medicine derives from 2 fundamental motives: the first is to better understand the cause and the mechanism of an illness, and the second is to create better solutions for hard-to-treat diseases,5 precisely as is the case in IBD. Despite the undeniable progress in the management of both Crohn disease (CD) and ulcerative colitis (UC) witnessed in the last few decades, treatment is nonetheless far from satisfactory, and essentially all new lines of therapy fundamentally still exploit immunosuppression as the tool to achieve the ultimate goal of curing IBD.6 The reason for pursuing immune modulation to treat IBD is understandable, but intuitively persisting in using it stifles innovation and delays the discovery of alternative solutions. The most advanced IBD therapies still aim at blocking or inhibiting single molecules, such as cytokines, chemokines, cytokine receptors, signaling molecules, adhesion molecules, and homing molecules, in any single therapeutic intervention.7 Incongruously, this one-drug-at-one-time approach disregards the widely accepted notion that IBD is multifactorial and that no single factor alone triggers or sustains IBD at any stage of disease evolution.8-10 Even very early-onset IBD, which is almost invariably caused by a specific immune defect, has an extensive list of monogenic etiologies and distinguishing clinical and laboratory features11 and an unpredictable and variable response to immunomodulatory and biological therapies.12 Therefore, if IBD is a complex multifactorial condition, then how can one expect a truly effective solution by targeting a single factor at a time? If IBD is caused by the integration of multiple factors, then it makes more sense to develop solutions that take that integration into account and where the result of integration discloses the therapeutic target. This sensible approach requires means that allow the integration of multiple biological factors and identify the result(s) of such integration; this is exactly what systems biology can do.
On a workable level, the reason why systems biology is needed is as follows: The detailed analysis of multiple factors and their integration leads to the emergence of “big data,” a term now popularized in many scientific, medical, social, financial, technical, and other fields. In the May 6, 2017 issue of The Economist, an article entitled “The World’s Most Valuable Resource Is No Longer Oil, but Data” points to the vital importance of amassing vast sums of knowledge in the world today to succeed in most fields of endeavor. Continuous technological advances have created and continue to generate massive amounts of information at an unprecedented pace. This is also true in the scientific and biomedical fields, where data become an invaluable asset for those able to take advantage of it.13 In biology and medicine, these increasingly large datasets are generated by high throughput technologies,14 like the Immunochip for IBD,15 and are conceived in a comprehensive way as omes, such as genomes, proteomes, and metabolomes, and their study creates the respective omics, ie, genomics, proteomics, and metabolomics, which symbolize the “omic era” we live in.16 The amount of information contained in each ome is too large for a single person to retain and analyze and is no longer accessible by traditional learning tools like articles, books, or even internet-based data and can only be visualized, utilized, and understood by using sophisticated computational tools like those available in the field of bioinformatics.17 As unintelligible and unmanageable big data may seem to the noninitiated, like most biomedical researchers and physicians, omics data are becoming more sensitive and facile and, by organizing medical information as cohesive molecular biology big data, they provide practical information useful for the diagnosis, classification, and treatment of human ailments.18 This process will take some time, but it is already happening in multiple fields of medicine, including IBD,19,20 proving that systems biology is not only here to stay but is also here to provide better understanding and management of CD and UC. It is important to remember that adopting systems biology for big data analysis does not diminish or exclude the importance of traditional bench-based in vitro and in vivo studies. On the contrary, the results of these studies represent the building blocks of big data, and one approach complements the other.21
BASIC NOTIONS OF IBD SYSTEMS BIOLOGY
Omes- and omics-based approaches were initially implemented in the field of cancer, followed by several other fields of medicine. Eventually the IBD community also recognized the value of omes and omics investigation,22 and consortia have been recently put together to effectively implement systems approaches for IBD.23, 24 To do so, some basic elements must be in place, such as the availability of high-quality biosamples from patients with IBD, access to electronic medical records, and systems biology and bioinformatics expertise. Next, the goal justifying a systems biology approach to an IBD-related issue must be judiciously decided a priori before starting any project because the types of biosamples and the bioinformatics tools to be chosen depend on the goal in mind. From the IBD investigator perspective, some familiarity is required with the concepts of omes and omics, omics data integration, biological networks, network medicine, and ultimately precision medicine, all topics individually discussed in the following sections.
Omes and Omics
The concept that IBD results from the combined effect of environmental, genetic, microbial, and immune factors is firmly established,25 but various other factors are certainly involved in the pathogenesis of IBD considering its highly complex and chronic inflammatory nature.26 For the most part, investigations of the 4 traditionally accepted IBD components are still being conducted separately from each other.8, 27-29 At the same time, there is an increasing realization that each of them must be investigated in its totality rather than choosing and studying single constituents, such as the effect of smoking, a particular genetic variant, a specific microbe, or an immune cell subset. By replacing the study of single constituents with the study of their totality, omes of IBD are built, such as the exposome, the genome, the microbiome, and the immunome, and their respective study become the exposomics, genomics, microbiomics, and immunomics of IBD. Each IBD ome deserves an in-depth evaluation, which will generate more information that will make big data progressively bigger. Regardless of how big each dataset may be, the study of IBD omes should not be restricted to a chosen few because a variety of additional omics exist that are relevant to IBD pathobiology, such as epigenomics, proteomics, metabolomics, and lipidomics.30-33 With the advent of newer and technologically sophisticated methodologies, such as single cell multi-omics and spatial transcriptomics,34, 35 the types and complexity of omics will certainly increase, which will put an additional burden on the process of omics data integration discussed in the next section.
Omics Data Integration
If in IBD there is a convergence of environmental, genetic, microbial, immune, and other effects, this convergence then implies that IBD results from the combination of whatever omes are involved in its pathogenesis; this in turn makes clear the need to understand their functional integration to fully understand the disease.10, 36 Studying multi-omics data is critical because it results in more than the sum of its parts,37 not simply from a magnitude perspective but, far more important, from the biological perspective of how etiological factors come together to regulate health and disease.38, 39 An integrative approach to disease can provide invaluable scientific and medical information, such as the prediction of cytokine responses,40 the prediction of cancer survival,41 the subtyping of autism disorders,42 the classification of chronic obstructive pulmonary disease,43 or the identification of biomarkers predicting IBD relapse.44 Multi-omics information becomes even more valuable when data are profiled longitudinally over time.45, 46 A limited number of reports on multi-omics data integration in IBD have so far been published,44, 47-51 but the establishment of IBD consortia and an increasing awareness by the IBD community will certainly stimulate more studies with multi-omics data.23, 24
Tools for Omics Data Integration
A large number of computational tools exist for multi-omics data integration and interpretation, each tool utilizing different methods, data, and languages, and providing different types of information.52 Omics datasets for IBD can also be analyzed with different tools and algorithms, but choosing which ones should be used depends on the types of datasets and the questions being asked. Following are a few representative examples. Cytoscape is one of the most popular for being a user-friendly, open-source computational platform that allows the integration and visualization of regulatory networks and subnetworks.53 Cytoscape can integrate different IBD omics with clinicopathological parameters, visualize IBD’s regulatory network, and identify its hubs. Notably, Cytoscape has the unique feature of including hundreds of software plug-ins, which allow for more specialized and focused analyses. For example, GenePro is a Cytoscape app that can generate a more detailed visualization and analysis of the IBD interactome.54 More recently, the Cy3D network app was integrated into Cytoscape, allowing the 3-dimensional visualization of a biological network and hub identification (http://apps.cytoscape.org/apps/cy3d). To understand the structure of a biological network, it is important to be able to study a comprehensive set of parameters, including the numbers of nodes, edges, connected components, centralization, clustering coefficients, and subnetwork neighborhood connectivities. All of these can be studied with the NetworkAnalyzer Cytoscape plugin.55 Another valuable aspect of other computational tools is their ability to evaluate network changes over time, as typically happens when IBD transitions from active disease to remission to relapse. These changes can be assessed with the novel tool DyNet, which enables investigators to perform dynamic network analysis of how the IBD network is rewired during disease progression.56
Biological Networks
The next step to take advantage of omics data integration is to understand how the intertwined biological components of a physiological or pathological process, like IBD, physically and functionally interact and regulate one another. This step requires a grasp of the concept of a biological network, a system that provides a formal mechanism for the representation, measurement, and modeling of the relationship among the various elements that make up the network.57, 58 Most networks are based on graph theory, a branch of mathematics used to study communications of multiple points connected by lines (https://www.britannica.com/topic/graph-theory). In practice, a network is represented by a graph where points (nodes) are connected directly or indirectly to other nodes by lines (edges). In biological networks a node can be any molecule, such as DNA, RNA, a gene, an enzyme, a cytokine, a metabolite, a microbial product, and so on, and the edges establish a unidirectional or bidirectional communication between 2 or multiple nodes (Fig. 1A). In a simplistic way, a structure is created in which most elements can relate to the others, just like in live systems, but the strength and the effect of the relationship can vary substantially depending on the types and location of the nodes within the network.57 In some networks there are a number of densely connected nodes forming modules, highly interlinked clusters or regions of the network that tend to be centrally located within the network and control vital functions, as functional modules do, or regulate and control a disease state, the so-called disease modules59 (Fig. 1B). Note also that networks are inherently dynamic and change because of the acquisition or loss of components (nodes) and links.60 Moreover, the timing and duration of the interactions among nodes also change depending on the need to adapt to maintain homeostasis in health or to cause a biological derangement in a disease process.58
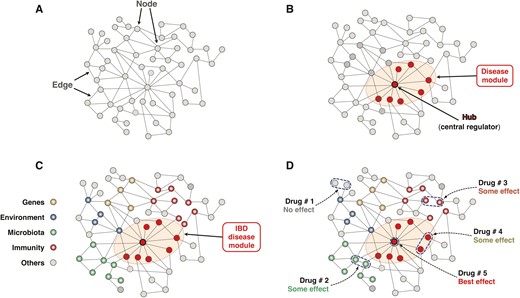
Schematic representation of the basic components and function of a biological network. A, The network is represented by a graph where nodes are connected to other nodes by edges. The communication between 2 or more nodes can be direct or indirect, and it is established by the edges in a unidirectional or bidirectional way. B, Within a biological network, densely connected nodes form modules that can be functional modules that control key functions of the network or disease modules that regulate and control a disease condition. Hubs are the central regulators of a module or a network and tend to be centrally located within a module. C, Schematic representation of the IBD interactome. The biological network underlying IBD pathobiology is composed of the genome, exposome, microbiome, immunome, and any other ome involved in its causality or mechanism. The centrally located module represents the IBD disease module that controls the overall function of the IBD interactome. D, Distinct therapeutic interventions on the IBD interactome and anticipated levels of effectiveness. Drug 1 acts on peripherally located and pathogenically less relevant nodes and will have an inconsequential effect on the interactome. Drug 2 acts on microbiome-related nodes and may exert some beneficial effect, like Drug 3, which acts on immunome-related nodes. Drug 4 acts on some nodes within the disease module and may also provide some beneficial effects. Drug 5 will have the best therapeutic effect because it specifically targets the hub of the disease module, which controls the IBD interactome.
The generation and integration of multi-omics data from cellular, animal, or human biomaterials form the procedural base for the creation of biological networks and the identification of the dominant interactions that determine the outcome of the network.61 These determinant interactions within a network can underlie specific pathological process like inflammation,62 or drive human diseases,63, 64 as some molecular regulators in IBD apparently do.65 Interestingly, neoplastic, inflammatory, and metabolic disorders tend to share disease modules enriched with disease-associated single-nucleotide polymorphisms, suggesting that common pathways are involved in the susceptibility to complex diseases.66 These overlapping pathways are linked to basic endophenotypes like inflammation, fibrosis, and thrombosis that play a role in a multitude of inflammatory and autoimmune conditions.67 Using the disease module model described in the paragraph above, inflammation, fibrosis, and thrombosis are mediated by the inflammasome, fibrosome, and thrombosome subnetworks that are part of the individual regulatory networks of many other chronic inflammatory conditions like IBD.
Interactomes
The view of biological networks has been taken a step farther with the conceptualization of the interactome. The term “interactome” first appeared in the literature in 1999 to describe multiple molecular interactions (protein-DNA, protein-RNA, and protein-protein) in the fly.68 Because by definition an interactome translates complex biological events, its understanding requires a systems biology approach that allows one to integrate and analyze all relevant physical interactions.69 This comprehensive view of the interactome was further consolidated and adopted in higher systems to illustrate a biological network with subunits (subnetworks) that are physically and functionally linked into a whole and was then applied to human diseases.59, 70-72 Interactomes have been described in a variety of conditions, including asthma,73 chronic obstructive pulmonary disease,74 type 2 diabetes,75 cardiovascular disease,76 psychiatric disorders,77 and even COVID-19 infection.78
In the case of IBD, the interactome can be defined as a highly complex biological network composed of the genome, exposome, microbiome, immunome, and any other ome involved in its etiology or pathogenesis (Fig. 1C). Within the IBD interactome, abnormalities in 1 or more omes causes the derangement of 1 or more regulatory subnetworks and the formation of an IBD disease module mediating intestinal inflammation.36
The concept of an IBD interactome derives from and complements multi-omics IBD studies, but it offers a far more comprehensive and cohesive picture of the biological intricacies of IBD molecular underpinnings and opens the way to the discovery of unsuspected targets for therapy.79 This is possible because interactomes, like any other biological, physical, social, and other network, are susceptible to disruption, ie, the loss of those parts within a network that are essential to proper function, such as the flow of communication in a computer system, the fluctuation of values in financial markets, or the specific molecular and cellular activities mediating a physiological response.80 The potential for disruption implies that the function of an interactome and its outcome are controllable,81 which can be done by identifying the key elements that control the network and making them targets for intervention (Fig. 2).82 Translating this concept to an interactome mediating a disease state, by identifying and targeting the controller(s) of the networks, usually the hub(s) of a disease module, the whole network falls apart and the disease is downgraded or even eliminated.82 This particular aspect is further discussed in the section of this review discussing therapeutic expectations from IBD systems biology.
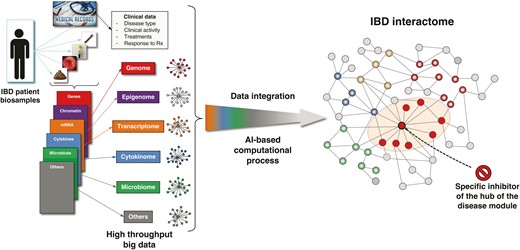
Sequential steps leading to the development of a drug that specifically disrupts the IBD interactome. Various types of biosamples (blood, serum, biopsies, stools) are collected from a patient with IBD, and the derived omics datasets are analyzed in the context of the patient’s medical records to generate individual networks for each ome. These networks are then integrated by an artificial intelligence computational process to build the IBD interactome and identify its central regulator (hub). Once identified, computational medicinal chemistry is applied to develop a specific inhibitor(s) that will specifically target the hub, induce the disruption of the IBD disease module, and eliminate or hinder its pathogenic activity.
Network Medicine
The preceding sections on omics, omics data integration, network biology, and interactomes share common themes of complexity, variability, and heterogeneity, all elements present in any real-life physiological or pathological process. It is clear that IBD is a condition in which complexity, variability, and heterogeneity are inherent and become evident at the diagnostic, classification, and management level. Thus, a solution to the multiple challenges of IBD must consider all of them while at the same time be customized to individual patients or subgroups of patients.10 Herein, we propose that network medicine can provide that solution.
Network science is not new,58, 83 but its application to biology and medicine is more recent, and the paradigm of network medicine applied to human diseases only emerged approximately a decade ago.59, 70, 84 The reason for its emergence is the realization that diseases in general, and particularly diseases like cancer, autoimmunity, and chronic inflammatory diseases, are extremely complex at the pathophysiological level. The 20th-century paradigm of one genotype, one phenotype, one disease has been gradually replaced by the far more complex and dynamic 21st-century paradigm of multiple genotypes linked to multiple mechanisms and phenotypes that progressively evolve over time.85 Thus, the gene-centric view of one gene, multiple forms of disease(s) is moving to a network-centric view in which multiple genes are associated with one or multiple phenotypes, which more truthfully reflects the reality of clinical medicine.86 The reductionist model of looking for one very specific single abnormality that is the cause of a complex disease like IBD should be abandoned; in fact, no single gene variant, environmental factor, microbial imbalance, or immune anomaly has ever been shown to cause typical CD or UC. If this hypothesis is correct, then it makes more sense to adopt a holistic model, where all potential components of IBD are taken into account and functionally merged to unravel the underlying molecular mechanisms and identify truly specific therapeutic targets.87
A holistic approach is the essence of network medicine, which embraces the complexity of multiple influences on disease and relies on many different types of networks.88 The reliance of network medicine on different types of networks implicitly requires the comprehensive assemble of omics data into big data and the transformation of big data into biological networks to discover the interactomes at the core of each complex disease. In other words, a variety of systems biology and bioinformatics steps are necessary to implement network medicine.21 Fortunately, a vast number of computational tools exist that can perform a huge array of tests, such as network visualization and integration,53 detection of key signaling pathways in regulatory networks,89, 90 recognition of human phenotypes,91 identification of transcriptional circuits and tissue- and cell type–specific networks,92, 93 mapping of disease mechanisms,94 and prediction of disease molecular determinants and drug repositioning.95 It is important to remember that whatever tools are utilized, they may yield different views of the subnetworks and of the interactome, so each approach must be carefully selected keeping in mind the goal of the disease network investigation. From a clinical perspective, as in IBD, the investigator may want to focus on practical and therapeutically valuable goals, such as target identification for the development of novel therapeutics, the ultimate goal of precision medicine.79
Precision Medicine
The term “precision medicine” is now popular not only in scientific and clinical circles but also in society at large because it brings the hope that treatment of a particular condition will be customized to the need of individual patients and will be more effective beyond any treatment available so far. There are various definitions of precision medicine (also called personalized, individualized medicine or high-definition medicine),96, 97 but one of the most straightforward is that of the National Institutes of Health U.S. National Library of Medicine Precision Medicine Initiative: “Precision medicine is an emerging approach for disease treatment and prevention that takes into account individual variability in genes, environment, and lifestyle for each person” (https://medlineplus.gov/genetics/understanding/precisionmedicine/definition/).98 Hopes for new discoveries of innovative approaches to challenging diseases are always very high, but precision medicine has the actual potential to drastically improve if not revolutionize the practice of medicine (Editorial. Big hopes for big data. Nat Med. 2020;26:1). Precision medicine can deliver precise classifications of patients,99 stratify patients at a molecular level,100 predict cancer survival,101 discover reliable biomarkers,102 specify disease-associated microbiota,103 provide new insights into type 2 diabetes and essential hypertension,75, 104 personalize management of pulmonary hypertension,105 and address a series of additional prospects that may improve how we practice medicine. Precision medicine has yet to be routinely applied to IBD, but its concept and value are being recognized,106 and publications are recently appearing in which network analysis is used to group patient subsets,47 discover biomarkers,44, 49 define the microbial ecosystem,51 classify treatment outcomes,48 or find the molecular drivers of IBD heterogeneity.107 Although highly innovative, most of these studies are not necessarily so comprehensive as to integrate all available information and build complete interactomes of the various CD and UC subtypes, identify their regulatory hubs, and develop specific inhibitors. These are logistically difficult, costly and time-consuming tasks, but there is no doubt that they will be progressively accomplished in the near future and that a systematic approach to IBD precision medicine will successfully emerge.
A distinct and beneficial feature of precision medicine for IBD or any other complex disease is its unbiased nature.108 Data are derived from high-throughput technologies free of human preconceived ideas,14 and therefore the integration of multi-omics data, the assembling of biological networks, and the construction of a disease interactome are determined by mathematical algorithms without the interference of scientific preferences, personal biases, or subjective interpretations.2, 4 These features generate impartial results that reflect the true underlying pathobiology of IBD and can lead to the discovery of new therapeutic targets that do not belong to the customary immune or microbial targets selected because they result from curated human manipulation.
Predicaments in IBD Systems Biology
As mentioned at the beginning of this review, the widespread availability of hundreds of analytical tools applicable to biomedical big data has caused a true explosion in the number of publications where a systems biology–based approach has been utilized to investigate a disease process. Although this development may be good from a visibility and awareness perspective, caution must be exerted in regard to the methodology used, the correctness of the data, the interpretation of the results, and the validity of the conclusions, primarily because of incorrect or superficial ways that bioinformatics is used by investigators who may be familiar with the analytical tools but not with the biology of the disease they are probing. Examples of some of the challenges afflicting the indiscriminate use of systems biology in IBD and other conditions are discussed below.
Most systems biology tools have been developed to study cancer109 and not chronic inflammatory diseases such as IBD. In cancer, specific genetic alterations (eg, mutations) are a dominant driver of its pathogenesis, resulting in a relatively stable gene-driven interactome, which may not be the case for complex immune-mediated diseases where nongenetic components exceed known and missing heritability,110 as in the case for gut microbiota.111 Therefore, following the exact computational approach used to develop the interactome of breast, renal, or lung cancer112-114 is not appropriate for building the interactome of patients with IBD with a particular phenotype, and the resulting regulatory network, biomarkers, or therapeutic targets may not be correct. Ongoing studies support this assumption (data not published). Thus, considering the probable instability of the IBD interactome in individual patients and its variability from patient to patient, IBD interactomes should be generated based on omics data obtained at multiple timepoints from carefully phenotyped patients.
Another consideration is which and how many omics data should be selected for a successful computational analysis. A general rule is to use as much omics data as practically feasible even on a relatively small number of biosamples rather than using few omics data from a large number of biosamples.115 In IBD there are several genome-wide association studies that have used thousands of IBD DNA samples to identify genetic variants and single-nucleotide polymorphisms related to CD and UC.28 However, it is far more difficult to collect different biomaterials such as blood, biopsies, and stool samples from thousands of patients with IBD. The cancer research community has shown that the use of multiple omics datasets from hundreds, and not thousands, of patients is sufficient for an accurate determination of disease subtypes and the identification of novel gene hubs.116 Specifically, the use of 2 to 5 omics datasets has allowed studies to reach statistical power using between 200 and 500 biosamples,117,118 and a similar approach should also work for IBD. Thus, there is no single right or wrong answer to the question of how many IBD omics datasets are necessary to adequately perform an IBD systems biology analysis. What is essential, however, is to know which biomaterials are accessible and the cost of performing multiple omics, and, most important, to carefully define a priori the specific aim of the IBD computational analysis.
In addition to the type of the biosamples, their quality is fundamentally important to extract correct information on the diverse omes present in the blood, serum, tissue, or stools. When and how were the samples collected? Were the samples processed right after procurement or after storage? How were they stored—in a reliable biobank119 or in an individual investigator’s refrigerated space? Was quality control performed at the time of procurement, just before processing, or not at all?120 The last issue is crucial when endoscopic biopsies from patients with CD or UC are used and classified as “noninflamed,” “inflamed,” or “healed” based only on endoscopic inspection without histological confirmation, as is almost universally the case in IBD reports. The tissue environment tunes and selects cellular diversity and function121 and implicitly impacts on the results, which may or may not correctly interpreted in light of a subsequent noninflamed, inflamed, or healed histological evaluation of the biopsy.122
Another significant problem is the widespread and easy access to public omics data repositories like the Gene Expression Omnibus of the National Center for Biotechnology Information,123 which health care professionals increasingly use because of convenience rather than generating fresh omics data from scratch by going through the labor of carefully selecting and recruiting patients and collecting biomaterials in a biologically and clinically defined setting. The use of public omics data is potentially fraught with multiple and serious, if not fatal, problems, such as the combination of data from multiple uncontrolled patient cohorts and centers, heterogeneity of the patient cohorts, missing clinical information, mixing of treated and untreated patients, merging adult and pediatric datasets, and lack of biological and clinical knowledge of the disease under study. It is easy to see how these severe flaws yield incorrect and meaningless information that unfortunately gets published when it is not properly weeded out at the editorial level.
Finally, most clinical, translational, or basic investigators not trained in bioinformatics are extraordinarily challenged when reading reports on big data and attempting to make sense of complex data derived from systems biology algorithms and visualization tools that look like large, incomprehensible “hairballs”.17 Investigators can become frustrated and discouraged; we revisit this key issue in the concluding section on future perspectives.
Taking all the above issues into account, it becomes clear that various obstacles still exist to the generation and integration of omics data, the construction of biological networks, the identification of disease interactomes, and the discovery of novel therapeutic targets. Among several challenges, some of the most common are the heterogeneity of patients at the biological and clinical level, the different origin of patient biosamples, the variable types of input data, the unknown multiplicity of biological interactions, the different technologies for generating high-throughput data, and the choice of bioinformatics tools.124 Nevertheless, all these challenges and limitations can be solved step-by-step as the acquisition of data is systematized, the quality of the collected data is standardized, and the value of utilizing omics data integration is increasingly appreciated.
EXPECTATIONS FROM IBD SYSTEMS BIOLOGY
An IBD systems biology approach encourages the IBD community to achieve some important practical milestones, improve our understanding of IBD pathobiology, and ultimately provide better patient care. Specifically, some of the milestones include the following:
IBD patient molecular classification: IBD is a highly heterogeneous disorder at multiple levels, and an IBD systems biology approach can classify patients into specific molecular rather than clinical subtypes that share the same clinicopathological and molecular parameters. This is a key step to move toward IBD precision medicine because such analysis may result in the reclassification of UC and CD. In fact, a recent study found that IBD disease location (colonic vs ileal) distinguishes disease pathobiology better than clinical classification.125
Specific biomarkers: The characterization of subtypes of patients with IBD would be improved with the identification of unique and accurate biomarkers. An IBD systems approach would predict the best noninvasive molecular biomarkers associated with specific clinical parameters. Furthermore, this approach would contribute to the development of companion diagnostics and the identification of biomarkers that correlate with IBD drug responses.
New target identification: The use of computational tools (ie, Cytoscape and its plugins) integrating IBD molecular and clinical datasets can lead to the discovery of new genes, proteins, and bacteria that are central regulators of the IBD interactome (Fig. 2). These computationally identified new targets will need to be further tested in IBD cellular and animal models to confirm their functional relevance to IBD pathogenesis.
Drug repurposing: The concept of drug repurposing or repositioning involves the investigation of existing U.S. Food & Drug Administration (FDA)-approved drugs for new therapeutic purposes.126, 127 Repurposing expedites the drug discovery and development process by reducing the number of required clinical steps and the time and cost for a medicine to reach the IBD market. An IBD systems approach can match the IBD interactome with the molecular signatures of FDA-approved drugs by using computational tools like the Connectivity Map software.128 This strategy could identify FDA-approved drugs to be evaluated in IBD preclinical models and then moved directly to phase II human clinical trials.
Systems biology–based randomized controlled trials: Future randomized controlled trials could be carried out based on the CD and UC molecular subtype rather than the traditional CD and UC phenotypic classification. This work could be done through the cooperative efforts of academic medical centers, pharmaceutical companies, and the FDA, which have plentiful biosamples from well-phenotyped IBD cohorts at their disposal. This novel approach may eventually lead to the abolition of traditional clinical trials performed separately for UC or CD and may be replaced by system biology–based precision medicine clinical trials129 based on patients with IBD with specific molecular and clinical characteristics.
Achieving the above and other milestones will drastically change how patient diagnosis and classification, target identification, and drug development have until now been conceived and developed in IBD. Following the remarkable advances in immunology and the discovery of molecules and pathways controlling immune and inflammatory responses, a series of targets and inhibitors has been identified based on experimental systems or fortuitous discoveries, as in the case of tumor necrosis factor-α inhibition by specific blocking antibodies.130 Adopting this approach has led to the development and clinical use of an ever-expanding armamentarium of inhibitors for molecules that directly or indirectly mediate diverse aspects of immune regulation.131 In addition, this approach has led to therapeutic success for both CD and UC, but its effectiveness is only observed in subsets of patients and can diminish or become lost with time. The same can be said about other less-specific therapies like immunomodulators, anti-inflammatory drugs, or antibiotics. All of them are still fundamentally based on a one-target, one-drug approach in the face of undeniable evidence that IBD is multifactorial and extremely complex at the biological and clinical level. When drugs are matched to a specific but single biomarker (like a genotype), they fail to yield clinically relevant benefits132; unfortunately, the pharmaceutical industry is still mostly focused on developing drugs that hit a single target as specifically as possible.133
Complex diseases require complex therapies,5 either the use of multiple drugs that act on different targets within the disease network or the administration of a single drug that specifically targets the central regulator (hub) of the disease module of a regulatory network shared by biologically homogeneous patients (Fig. 1D). Neither of these 2 goals can be achieved by using the traditional one-target, one-drug approach but instead by embracing a combination of network pharmacology, drug-target networks, network medicine, and precision medicine.134-136 Evidence of the effectiveness of integrated approaches is increasing, as shown by experimental and clinical reports.18, 137-140
FUTURE PERSPECTIVES
What was once called a “fishing expedition,” ie, the emerging of omics research in biological sciences in the late 1990s,141 is now a solidly established scientific discipline holding the promise to revolutionize medicine as we know it. Anticipating the inevitable incorporation of systems biology and network medicine into medical practice, the fundamental issue that emerges is how medical professionals, such as physicians specializing in the care of patients with IBD, can take advantage of its power to make exact diagnoses, uncover specific biomarkers, predict disease course, and implement a customized treatment strategy. All of this requires first an open-minded acceptance by the medical community of the coming of medicine in the digital age142 and, second, a knowledge of systems biology that is still not part of what is taught in medical schools or used in daily practice. Although the acceptance of digital medicine is an intellectual step, acquiring new knowledge and applying bioinformatics tools are challenging material tasks. These issues are discussed by Obermeyer and Lee in an insightful perspective on the future of medicine.143 They point out the profound mismatch between the limited abilities of the human mind and the seemingly unlimited complexity of medicine, and the lack of training of medical students in how to develop, interpret, and apply algorithms that are transforming clinical practice.143 Some programs have been created that offer the means to be trained in precision medicine,144 but they are far from being eagerly sought out by medical professionals, and the convergence of human and artificial intelligence for precision medicine is still far away.145
Thus, if a physician sincerely desires to use systems biology not for the sake of personal knowledge but for application to medical problems like the ones faced in the care of patients with IBD, only 2 solutions exist, at least for now: one is to devote a few years to additional training and learn the tools of computer science and bioinformatics, a choice that very few will make; the other is to create bridges between network and translational scientists by collaborating with experts who are already trained in these sciences and are willing and interested in applying their skills in a cooperative and equal plane to solve the biomedical challenges of the disease of interest.146 The second option seems to be the most practical and logical one, with the additional advantage of creating multidisciplinary teams spanning machine learning, chemistry, and biology147 and whose know-how can then be applied to a variety of other medical problems.148
Major deviations from a predictable, though unsatisfactory, situation are habitually poorly received by medical professionals because innovation brings the uncertainty and discomfort of not knowing what to do and the inconvenience of learning procedures different from those that are already in place or becoming involved in formal studies that will take time and disrupt an established professional routine. At the same time, most people always look for something better, such as physicians caring for IBD patients and constantly searching for better results. In the near future, better management of IBD will unquestionably require the use of systems biology to provide much improved and customized management based on network medicine. In the current era of big data, this improvement cannot be achieved through traditional apprenticeship and clinical trial models, and the future generation of IBD experts will have no choice but develop new skills or build collaborations to use big data.149 This advancement will be promoted by new organizations like the International Network Medicine Consortium, which is being designed to develop the right mindset among all types of health care providers and to put at their disposal the tools necessary to improve health care in a global way.150 Thus, IBD physicians will soon find themselves facing a “resistance is futile” situation, where refusing to accept the inevitable is pointless, a situation where “if you can’t beat them, join them.”
Conflicts of interest: C.F. received speaker fees from UCB, Sandoz, Janssen, and Genentech and is a consultant for Athos Therapeutics. Inc. D.I. is president and chief executive officer of Athos Therapeutics, Inc.
REFERENCES