-
PDF
- Split View
-
Views
-
Cite
Cite
Roblyn A. Kendall, Jerry X. Mitrovica, Radial resolving power of far-field differential sea-level highstands in the inference of mantle viscosity, Geophysical Journal International, Volume 171, Issue 2, November 2007, Pages 881–889, https://doi.org/10.1111/j.1365-246X.2007.03546.x
- Share Icon Share
Summary
For two decades leading to the late 1980s, the prevailing view from studies of glacial isostatic adjustment (GIA) data was that the viscosity of the Earth's mantle increased moderately, if at all, from the base of the lithosphere to the core—mantle boundary. This view was first questioned by Nakada & Lambeck, who argued that differential sea-level (DSL) highstands between pairs of sites in the Australian region preferred an increase of approximately two orders of magnitude from the mean viscosity of the upper to the lower mantle, in accord with independent inferences from observables related to mantle convection. We use non-linear Bayesian inference to provide the first formal resolving power analysis of the Australian DSL data set. We identify three radial regions, two within the upper mantle (110–270 km and 320–570 km depth) and one in the lower mantle (1225–2265 km depth), over which the average of viscosity is well constrained by the data. We conclude that: (1) the DSL data provide a resolution in the inference of upper mantle viscosity that is better than implied by forward analyses based on isoviscous regions above and below the 670 km depth discontinuity and (2) the data do not strongly constrain viscosity at either the base or top of the lower mantle. Finally, our inversions also quantify the significant bias that may be introduced in inversions of the DSL highstands that do not simultaneously estimate the thickness of the elastic lithosphere.
1 Introduction
The detailed depth variation of viscosity in the Earth's mantle remains a contentious issue. Analyses of observables related to mantle convection, such as long-wavelength geoid anomalies, have consistently preferred a mantle viscosity that increases by more than an order of magnitude from the upper to the lower mantle (e.g. Hager 1984; Ricard et al. 1984; Richards & Hager 1984; Forte & Peltier 1991; King & Masters 1992). However, viscosity profiles inferred from geophysical observables associated with glacial isostatic adjustment (GIA) have been characterized by less consensus.
McConnell (1968) derived the Fennoscandian Relaxation Spectrum (i.e. decay time of strandline uplift versus spatial wavelength) for a plane Earth and inferred a significant, factor of ∼25, increase of viscosity from the upper mantle to the top portion of the lower mantle. In contrast, the first global, spherical Earth analyses of GIA data argued for an essentially isoviscous mantle, or, at most, a moderate (factor of ∼2) increase of viscosity from the base of the lithosphere to the core—mantle boundary (CMB) (Cathles 1975; Peltier & Andrews 1976). Subsequent studies by Peltier and colleagues (e.g. Wu & Peltier 1983, 1984; Tushingham & Peltier 1991), based on relative sea level (RSL) and anomalies in rotation and gravity, further supported this conclusion, and this led to arguments that transient effects (i.e. timescale dependence) in viscosity were required to reconcile the disparate inferences from convection and GIA data (e.g. Peltier 1985; Sabadini et al. 1985; Peltier et al. 1986; Yuen et al. 1986). However, the prevailing view of an isoviscous mantle on GIA timescales was countered by the GIA-based analysis of Nakada & Lambeck (1989) and Lambeck & Nakada (1990) (henceforth the NL studies), who argued for an approximately two order of magnitude increase in viscosity from the upper to lower mantle.
A complication in inferring mantle viscosity from GIA data is that the raw observables are sensitive to uncertainties in the space—time geometry of the Late Pleistocene ice loads. To limit this sensitivity, the NL analyses were based on the differential amplitude of sea-level highstands recorded in the Australian region. In this area, RSL variations subsequent to the last glacial maximum are generally characterized by a rapid sea-level rise during the deglaciation phase, followed, in the last 4–6 kyr, by a gradual decrease extending to the present-day. Although the ensuing highstand amplitudes are relatively insensitive to the geographical distribution of the former ice loads, they are sensitive to remnant melting following the primary phase of deglaciation. This sensitivity can be significantly reduced by considering the difference in highstand amplitudes between two relatively nearby sites (we use the notation DSL to refer to these differential sea-level highstands). Using a set of such DSL data, NL inferred an elastic lithospheric thickness (LT) in the range 70–80 km, an upper mantle viscosity (νum) of 2 − 3 × 1020 Pa s, and a lower mantle viscosity (νlm) of ∼1022 Pa s.
Nakada & Lambeck (1991) compared this result with inferences they derived from sea-level data sets in other regions, including Hudson Bay, Northwestern Europe and Pacific islands. The Pacific island data suggested an upper mantle viscosity less than 1020 Pa s, while the remaining three data sets preferred νum∼ 3 × 1020 Pa s. In contrast, these three data sets were best fit by lower mantle viscosities ranging from 4 × 1021 Pa s for Europe (in agreement with later work by Lambeck et al. 1998) to 3 × 1022 Pa s for Hudson Bay. This variation led them to argue for the presence of lateral heterogeneity in mantle viscosity.
The robustness of such an argument is complicated by several factors. First, with the exception of the DSL data from Australia, the remaining data sets involved raw RSL histories, and were thus sensitive to the adopted surface loading history. Second, these inferences were all based on simple three parameter (LT, νum, νlm) forward analyses, and thus they do not provide a measure of the detailed depth dependence on viscosity of the various data sets. This dependence must be unique given the distinct spatial scales associated with the ice loading over Fennoscandia and Laurentia and the ocean loading at Australian coastal and Pacific island sites. Thus, the differences cited above may reflect a limitation of the forward model parametrization rather than necessarily reflecting lateral rheological structure.
To assess the radial resolving power of GIA data sets requires the application of formal geophysical inversion procedures. To date, such inversions have focused largely on decay times derived from postglacial emergence curves. The uplift of previously glaciated regions is characterized by (relatively) free decay, and the associated RSL curves may be accurately described by a simple exponential form (Andrews 1970; Walcott 1980). Whereas the amplitude of this form is a function of the local ice load thickness, the decay time is relatively insensitive to the finer details of the load history, and Mitrovica & Peltier (1993) have thus advocated their use in constraining the radial profile of mantle viscosity. As an example, Mitrovica (1996) analysed the postglacial decay time of Angermanland in central Fennoscandia to revisit the classic Haskell (1935) constraint on mantle viscosity (1021 Pa s). He demonstrated that previous inferences of an isoviscous mantle (e.g. Tushingham & Peltier 1991) were biased by a misinterpretation of the Haskell average as a constraint on the mean upper mantle viscosity; a resolving power analysis indicated that the true average extended to ∼1400 km depth. Moreover, joint, multilayer inversions of a suite of mantle convection data and GIA observables, including decay times from Fennoscandia, Hudson Bay (where resolving kernels extend from ∼400 to 1800 km depth; Mitrovica & Forte 1997) and a revised Fennoscandian Relaxation Spectrum (Mitrovica & Forte 2004), indicate that both classes of data may be simultaneously reconciled by a viscosity profile characterized by a significant increase with depth. Specifically, the most recent inference involved a three order of magnitude increase from the upper mantle (∼4 × 1020 Pa s) to the top of the lower mantle (∼2 × 1021 Pa s) and a high viscosity peak (1023 Pa s) at 2000 km depth. A similar reconciliation of GIA and convection data sets was reported by Kaufmann & Lambeck (2000).
The main objective of the present paper is to revisit the influential analysis of NL within the framework of (Bayesian) inverse theory. Specifically, our goal will be to provide the first formal assessment of the radial resolving power of the DSL data set; that is, to identify the specific radial averages of mantle viscosity that are resolved by the far-field data. Given the specific aim of the present study, our analysis follows as closely as possible the original NL study. In particular, we will use the same site pairs to define the DSL data. Moreover, the global ice load we adopt will be very similar to the model constructed by NL. One notable change from the earlier studies will be the theory we adopt for predicting postglacial RSL variations. Since the early 1990s there have been significant improvements in this theory [see Mitrovica & Milne (2003) for a recent review], and we are compelled here to adopt these improvements.
To begin, we summarize those parts of the analysis procedure that are distinct from NL, in particular, the Bayesian inversion algorithm and the new aspects of the sea-level theory. We note that the former includes parameters for both mantle viscosity and lithospheric thickness. Next, we establish the resolving power of the DSL data adopted by NL using a set of synthetic data inversions.
2 Theory
2.1 The generalized sea-level equation
We solve the generalized sea-level equation developed in Mitrovica & Milne (2003) using the numerical algorithm derived in Kendall et al. (2005). This equation extends the traditional GIA theory of Farrell & Clark (1976) to accurately account for time dependence in shoreline geometry arising from local transgression and regression of water or changes in grounded marine-based ice.


Although the generalized eq. (1) holds for any type of earth model, our calculations assume spherical symmetry and are based on the extended pseudo-spectral technique described by Kendall et al. (2005) for this special case. In this regard, we adopt a truncation at spherical harmonic degree and order 128. Predictions of the change in sea level require a formalism for computing the deformational and gravitational response to the surface mass load. For this purpose we adopt Maxwell viscoelastic Love number theory (Peltier 1974). The elastic and density structure of the earth model is given by the seismically determined PREM (Dziewonski & Anderson 1981). We have reconstructed an ice load history that is similar to the ARC3+ANT3 ice history of Nakada & Lambeck (1988).
An example of a forward prediction for four sites in the Australian region is shown in Fig. 1. (The location of these sites, and others included in the analysis below, is given in Fig. 2.) The predicted highstands at 6 kyr bp coincide with the end of the deglaciation phase in the ARC3+ANT3 history. The sea-level curves show a dramatic rise in consequence of the meltwater flux during the main deglaciation phase. Subsequently, the curves tend to exhibit a small decrease to the present-day which yields a characteristic sea-level highstand. The sea-level fall during the interglacial is driven by the combined effects of processes known as ocean syphoning and continental levering (for a recent review, see Mitrovica & Milne 2002). Ocean syphoning describes the fall in far-field sea level resulting from the movement of water from the far-field to the near-field as the peripheral bulge subsides. Continental levering refers to the tilting of continents due to the differential loading across the continental margin.
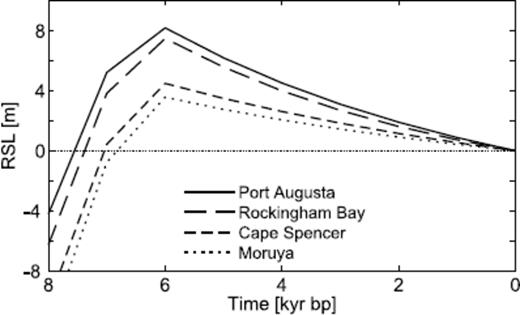
Forward predictions showing typical RSL highstands at four sites in Australia.
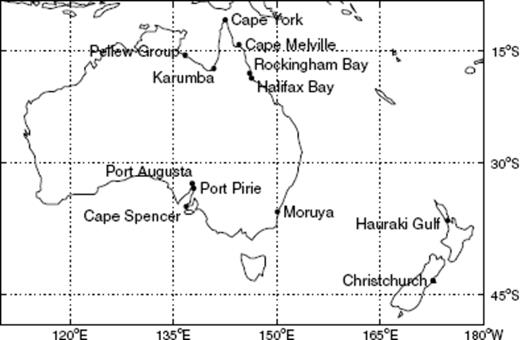
Location of sites at which synthetic RSL highstands are computed.

2.2 Bayesian inversion



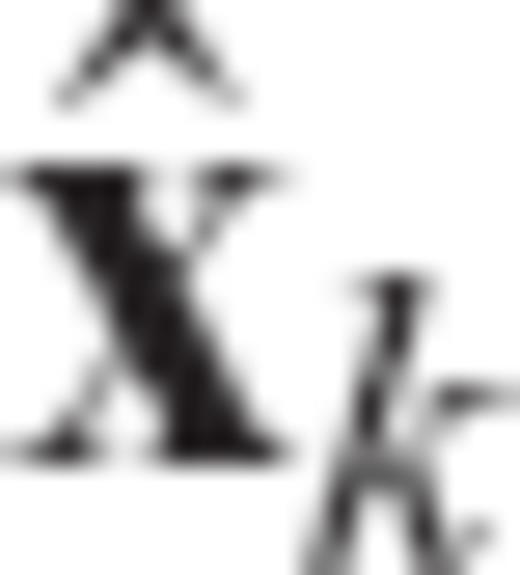
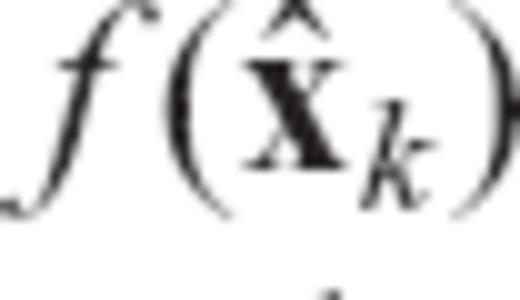
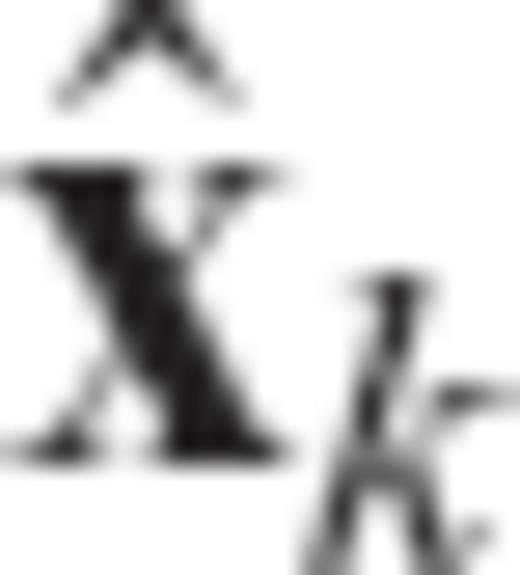



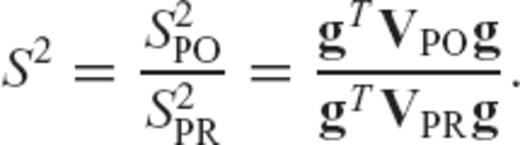
3 Results
We begin by presenting Fréchet kernels for sea-level highstands computed using a model close to that preferred by NL. These kernels provide a measure of the detailed sensitivity of the data to depth-dependent variations in mantle viscosity and changes in the elastic thickness of the lithosphere. Next, we perform a set of inversions using synthetic (i.e. numerically derived) DSL values for the 14 site pairs adopted by NL and listed in Table 1. The first such inversion is based on a prior constraint of a two-layered viscosity model. We next extend this simple case to consider a multilayered viscosity profile and examine the detailed resolving power of the synthetic data. These inversions are performed with and without observational errors applied to the synthetic data. The locations of the sites that define the DSL highstands are given in Fig. 2.
3.1 Fréchet kernels
As discussed in Section 2.1, relative sea-level variations in the far-field are driven by two processes. Ocean syphoning is a long-wavelength effect that is sensitive to the dynamics of the peripheral bulge and is therefore expected to show the same sensitivity to mantle viscosity as the integrated deformational response across the subsiding peripheral bulge. Fréchet kernels for the present-day radial velocity at sites spanning the Laurentian peripheral bulge (frames D, E, F of fig. 12 in Mitrovica et al. 1994) show a sensitivity to viscosity at all depths. Indeed, for sites located on the outer edge of the bulge region, sensitivity to the lower mantle remains nearly constant down to the CMB. Sites spanning the position of maximum subsidence through to the outer flank of the peripheral bulge show a maximum sensitivity to viscosity at depths near the 670 km boundary. We therefore expect the component of far-field RSL resulting from syphoning to exhibit sensitivity to changes in viscosity at all depths within the mantle. Far-field relative sea level is also driven by continental levering. Continental levering can occur on both long-wavelength scales (across whole continents) as well as on a variety of local scales (along peninsulas, for example; Nakada & Lambeck 1989). This is due to the mix of spatial wavelengths that define the ocean load in regions surrounding the continents and islands.
Fréchet kernels for RSL highstands were computed numerically using equations analogous to (7) and (8), where we have used RSL highstands in place of DSL. The computations adopt a lithospheric thickness of 71 km, and constant upper and lower mantle viscosities of 0.5 × 1021 and 5 × 1021 Pa s. The RSL kernels (Fig. 3) show the greatest sensitivity to viscosity within model layers in the upper mantle, where local levering effects can cause the sensitivity to vary dramatically from site to site. There is also a non-negligible sensitivity to the lower mantle viscosity resulting from the long-wavelength effects mentioned above. This sensitivity shows a similar trend from site to site, and is therefore expected to decrease significantly when DSL highstands are constructed.

Numerically computed RSL Fréchet kernels for the individual sites considered in this study. The earth model used in the computations is defined by an elastic lithosphere of thickness LT = 71 km, νum = 0.5 × 1021 Pa s and νlm = 5 × 1021 Pa s. The vertical dashed line indicates the 670 km depth boundary.
Numerically computed DSL kernels are plotted in Fig. 4 for 12 of the 14 pairs of sites treated by NL (see caption). The sensitivity to variations in viscosity within upper mantle layers resulting from the shorter wavelength signal of local continental levering effects remains large. As with the RSL kernels, this sensitivity can vary greatly between pairs of sites. In contrast, the sensitivity evident in the predicted RSL signal, resulting from processes such as ocean syphoning and long-wavelength ocean-driven continental levering, is partially cancelled by considering the difference in these highstands. Thus, as predicted from the RSL kernels, the sensitivity to the viscosity in lower mantle layers has been significantly reduced, though it has not been eliminated entirely. Notable examples of this sensitivity are the kernels for Rockingham Bay—Moruya and Cape York—Rockingham Bay.
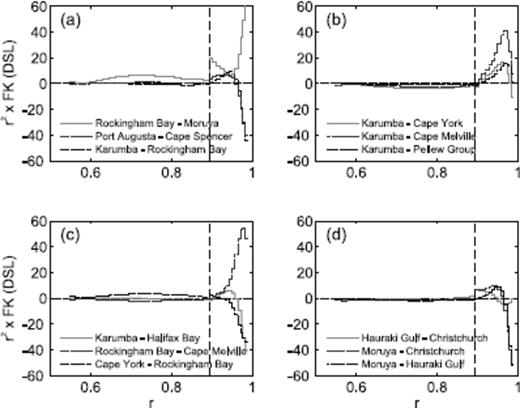
Numerically computed DSL Fréchet kernels for the pairs of sites treated in this study. Note that the kernel for Port Pirie—Cape Spencer is nearly identical to that for Port Augusta—Cape Spencer in (a) and has been omitted from the figure for clarity; similarly, the kernel for Halifax Bay—Moruya is nearly identical to that for Rockingham Bay—Moruya in (a), and has also been omitted. The earth model used in the computations is defined by LT = 71 km, νum = 0.5 × 1021 Pa s and νlm = 5 × 1021 Pa s.

In Table 1, we present the contributions to the total change δDSL based on a uniform perturbation of δlog ν = 0.3 to the upper and lower mantle and the Fréchet kernels in Fig. 4. That is, δDSLlm is the change in DSL expected from a uniform perturbation of δlog ν = 0.3 (i.e. a factor of two change in viscosity) in the lower mantle, and similarly for δDSLum. Clearly, the sensitivity of the DSL predictions to bulk variations in the lower mantle viscosity is comparable to a similar variation in upper mantle viscosity at many site pairs.
Previous studies of DSL data have been limited to isoviscous upper and lower mantle regions and, hence, to bulk changes in viscosity within each. The depth-dependent kernels in Fig. 4 indicate that these bulk measures miss important sensitivities at shorter radial scales. Consider, for example, the results in Table 1 for Karumba—Rockingham Bay, which indicate a relatively minor (in comparison to other site pairs) sensitivity to bulk changes in upper mantle viscosity. Examining the sensitivity kernel for this site pair as a function of depth in Fig. 4(a) shows that there is, in fact, a relatively significant sensitivity to variations in viscosity at the base of the lithosphere. However, since the kernel for this DSL prediction changes sign within the upper mantle, partial cancellation occurs when considering the bulk sensitivity.
To complete the analysis of the Fréchet kernels, we also present the results of eq. (8) in Table 1, where, for illustrative purposes, δDSLlt is listed for a perturbation of δLT = 10 km. (Thus we are comparing, in Table 1, changes in DSL predictions arising from changes in LT of 10 km versus a factor of two increase in either νum or νlm.) There is considerable variation in the sensitivity to lithospheric thickness between site pairs. In some cases this sensitivity is as expected. For example, Port Augusta and Cape Spencer lie at opposite ends of a long, narrow inlet, and therefore predictions of DSL for this site pair will be sensitive to the influence of the choice of LT on the predicted amplitude of levering (and relatively less sensitive to changes in νum and νlm; NL). In contrast, the DSL prediction for Rockingham Bay−Cape Melville shows a marked insensitivity to changes in LT, which is likely a consequence of the location of these sites relative to the coastline. These sites lie parallel to the broad, levering-induced tilt of the coast, and thus taking the difference in the RSL predictions to compute the DSL will strongly reduce the sensitivity to LT. (Note that Port Augusta and Cape Spencer lie perpendicular to the tilting.) However, the prediction Rockingham Bay−Cape Melville does show a strong sensitivity to changes in viscosity within the top half of the upper mantle (Fig. 4c).
The results presented in Figs 3, 4 and Table 1 were all obtained using the same earth model (LT = 71 km, νum = 0.5 × 1021 Pa s and νlm = 5 × 1021 Pa s). Since the predictions (and hence the inversions) are non-linear functions of the earth model, the Fréchet kernels (i.e. the depth sensitivity) for these DSL predictions will also be a function of the earth model. To explore this issue, in Fig. 5 we show kernels computed using a suite of earth models defined by LT = 71 km, νum = 0.5 × 1021 Pa s and uniform lower mantle viscosities ranging over two orders of magnitude. Fig. 5(a) shows the results for Port Augusta−Cape Spencer. As the viscosity in the lower mantle increases, the sensitivity to the viscosity within the lower mantle tends to decrease. The sensitivity to the transition zone shows a monotonic change as the lower mantle viscosity of the earth model is increased, while the sensitivity to the shallow upper mantle remains high in all cases. In contrast, for Rockingham Bay—Moruya (Fig. 5b) the sensitivity to the lower mantle changes less predictably, and only decreases to near-zero at the highest value of the adopted viscosity within this region. Near the 670 km boundary the change in sensitivity is non-monotonic. Again, the sensitivity to the shallow upper mantle viscosity remains high.
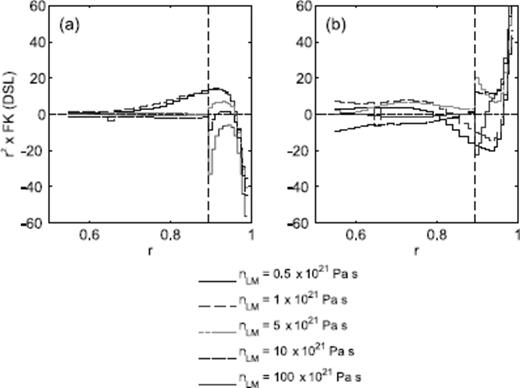
Numerically computed Fréchet kernels for the DSL highstand of (a) Port Augusta—Cape Spencer and (b) Rockingham Bay—Moruya. The earth model in each of the computations is defined by LT = 71 km, νum = 0.5 × 1021 Pa s and a lower mantle viscosity as labelled.
3.2 Inversions of synthetic data
To begin, we generate a suite of synthetic DSL highstands, y, using an earth model with a lithospheric thickness of 71 km, and a mantle consisting of constant upper and lower mantle viscosities of 0.5 × 1021 and 5 × 1021 Pa s. We begin the iterations with a starting model, , defined by LT = 96 km, νum = 1021 Pa s and νlm = 1022 Pa s. Throughout this section, we set the prior model, xPR, equal to the starting model.
The first inversions are based on a prior assumption of a two-layered viscosity model. To this end, we construct a prior covariance matrix which consists of a high (essentially perfect) correlation between each model layer in the upper and, separately, the lower mantle. That is, the model values are strongly correlated within each of these two mantle layers, but there is zero prior correlation between layers on either side of the 670 km depth boundary. This special case is consistent with the usual assumption in forward modelling of GIA data of isoviscous upper and lower mantle regions. The prior viscosity variances are chosen to be sufficiently weak (we adopt a value of 50, or approximately a ± 7 order of magnitude change) to ensure that the inversions are not influenced by this parameter. Furthermore, the prior variance for the lithospheric thickness is 3600 km2 (corresponding to ±60 km standard deviation).
In Fig. 6, we show the results for inversions based on three different synthetic data sets. The first (solid line) represents an inversion of the 14 site pairs in the NL study for which observed values were provided. The second (dashed line) extends this data vector to 50 pairs of sites from Australia and the South Pacific (these sites are taken from the broader range of locations listed in the NL study, but for which observed data was not provided). The third inversion (dotted line) includes the same 14 site pairs as the first data set, but in this case noise has been added to the forward RSL predictions at each site in an effort to simulate real data uncertainties. The site-specific RSL error was generated using a population of random numbers with a standard deviation of 0.3 m. The abscissa on the plot represents the iteration counter, and the horizontal dotted line shows the model [LT in frame (a) and νum and νlm in frame (b)] used to compute the synthetics (i.e. the ideal solution of the inverse problem).
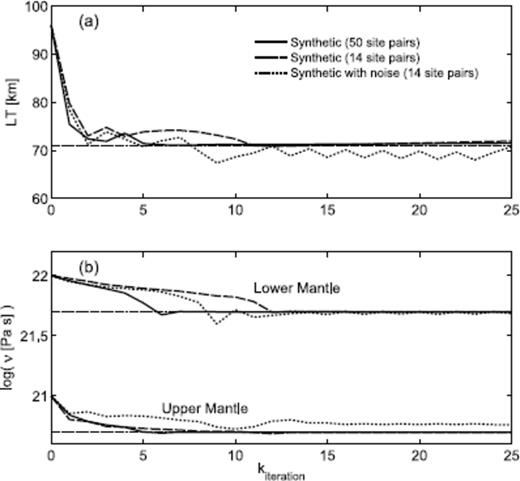
Results for inversions based on three different sets of synthetic data, shown as a function of iteration counter, k. In each case, the starting model is defined by LT = 96 km, νum = 1021 Pa s, νlm = 1022 Pa s, and the synthetic model (with and without noise applied) is LT = 71 km, νum = 0.5 × 1021 Pa s, νlm = 5 × 1021 Pa s. The remaining details of the inversions are described in the text.
The results for the inversion of DSL from 14 pairs of sites (Fig. 6) show that convergence requires on the order of 10 iterations, and is thus relatively slow. This indicates that the inverse problem retains significant non-linearity, even with the logarithmic parametrization of the viscosity. Indeed, one-step inversions will yield poor results, for example, νlm remains relatively close to the starting model of 1022 Pa s after a single iteration. The slow convergence is clearly a function of the information content of the 14 DSL highstands, and it is thus useful to compare the inversion with the results based on the larger data set. Extending the synthetic data set to include 50 site pairs significantly decreases the number of iterations for convergence of both the LT and νlm estimates, but has little effect on the convergence of νum.
To simulate the inversion of real data, we degrade the information content within the 14 DSL highstands by including random observation errors. In this case, it is interesting to note that convergence is still achieved at roughly 10 iterations (although the estimate for LT tends to oscillate). However, the converged values for LT and νum do not match the synthetic. Specifically, LT is somewhat too low, and νum too high. The discrepancy is relatively small for the level of observational error we have applied, but it is a first indication of a correlation between errors in the estimate of these two parameters.
We explore this correlation further in Fig. 7, where we show the converged values of each of the three parameters as a function of the variance of the lithospheric thickness adopted in each inversion. We use the same synthetic and starting models described above, include 14 site pairs (with no errors applied) in the inversions, and a prior viscosity covariance of 50. When the prior variance for the lithospheric thickness is very small (that is, when the lithospheric thickness is constrained in the inversion to remain close to the prior value of 96 km), the viscosity in both the upper and lower mantle converge to values significantly different from those used to compute the synthetics (LT = 76 km). As we weaken the constraint on LT, and thus allow the estimate of this parameter to match more closely the synthetic model value, the viscosity estimates improve. However, these estimates do not converge to the correct values of νum and νlm until the variance of LT is set above approximately 2000 km2. This exercise indicates that an accurate inference of mantle viscosity on the basis of DSL highstands requires a simultaneous estimate of (a weakly constrained) lithospheric thickness.
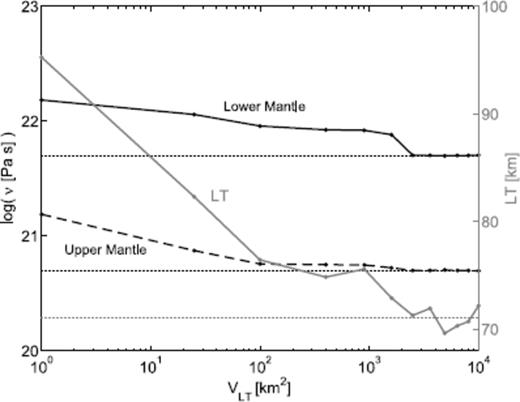
Converged results (after 25 iterations) as a function of the covariance of the lithospheric thickness adopted in the inversion. The synthetic and starting models are described in the text. Note that the axis on the left is for the viscosity, while LT is labelled on the right.
We turn next to the case where synthetic data are inverted under the assumption that there are no a priori correlations between the individual mantle layers. That is, we no longer constrain the inversion to a two-layered viscosity model. We adopt the same starting earth model as before. Moreover, we begin with a noise-free synthetic data set consisting of DSL highstands for 14 site pairs generated from forward predictions based on an earth model defined by LT = 71 km, νum = 0.5 × 1021 Pa s and νlm = 5 × 1021 Pa s. As for the two-layered inversions, we adopt prior variances of 50 for the (log of) mantle viscosity and 3600 km2 for the lithospheric thickness.
The thick solid line in Fig. 8 shows the converged model for this case. This result appears to be consistent with the model used to compute the synthetics for layers throughout the upper mantle and within the middle of the lower mantle. There is, however, disagreement at both the top and bottom of the lower mantle, where we note that the Fréchet kernels in Fig. 4 show little sensitivity. We explore this disagreement further in the next section where we present results for a formal resolving power analysis.
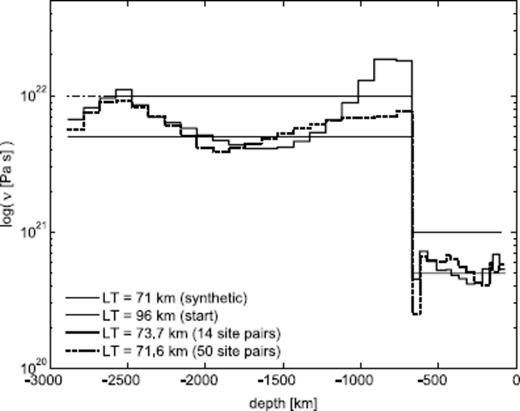
Results of converged (after 25 iterations) inversions with no prior correlations between each of the 34 mantle layers. The thick solid and dotted lines represent inversions based on 14 and 50 synthetic DSL data, respectively. The synthetic and starting models are prescribed on the figure. In the 14 data inversion the prior variances are 50 for the (log) viscosity parameters and 3600 km2 for the lithospheric thickness parameter. The former is reduced to 10 for the 50 data inversion.
3.3 Resolving power analysis

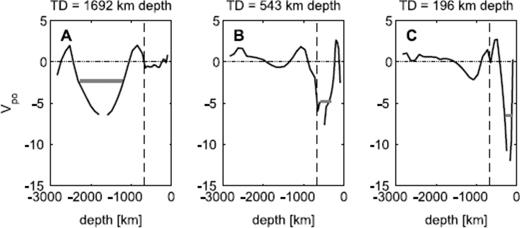
Posterior covariances for 3 different target depths (TD), as labelled on each frame, for the inversion of the 14 DSL data (thick solid line, Fig. 8). The solid grey horizontal line on each frame represents our measure of the radial resolving power at the associated target depth (see Table 2 and text). The diagonal element (i.e. the posterior variance for the given target depth) is positive and off the top-scale of the plot.
The spread of the covariances provides the first detailed measure of the radial resolving power of the NL data set, and the thick horizontal line on each frame represents the radial region over which averages were obtained. These lines define three radial regions extending from 110 to 270 km, 320 to 570 km and 1225 to 2265 km depth; that is, spreads of ∼160 to ∼250 km for the two upper mantle targets and ∼1040 km for the target in the middle of the lower mantle.
Table 2 provides the volumetric average of the inverted model values within each of these three regions (labelled as A, B and C). These averages are 20.73 and 20.74 within the upper mantle, and 21.68 in the lower mantle, in excellent agreement with the averages of the model used to compute the synthetics (20.69 and 21.69 in the upper and lower mantle, respectively). We conclude that the inversion has accurately retrieved averages of the synthetic model over the three radial regions resolved by the 14 DSL data. The inversion also yields an estimate of the lithospheric thickness of 73.7 km, which is in good agreement with the value (LT = 71 km) used to compute the synthetics.
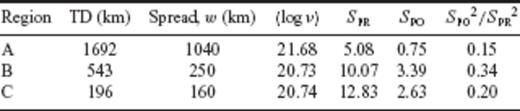
Results of the inversion for each of the target depths considered in Fig. 9.
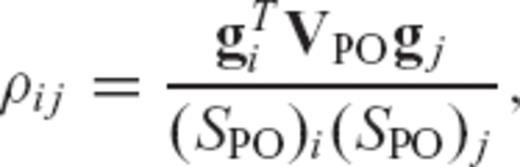
The inversion shown by the thick solid line in Fig. 8 makes clear the limited information content of the 14 DSL data. These data resolve relatively fine structure in the upper mantle, but the resolving power within the lower mantle is significantly weaker and confined to the middle of this region. As in the last section, we performed a test in which we inverted a larger data base of 50 DSL synthetics. The results for this case (thick dashed line, Fig. 8) suggest significantly improved resolution of shallow (top 500 km) lower mantle structure.
4 Conclusions
In this paper, we have revisited the influential analysis performed by Nakada & Lambeck (1989) and Lambeck & Nakada (1990) using an updated sea-level theory and a formal Bayesian inverse theory in order to examine the resolving power of their original data set.
The non-linear, iterative inversion of highstand data requires the calculation of Fréchet sensitivity kernels. We have found that although the site-specific RSL predictions showed the greatest sensitivity to viscosity variations within the upper mantle, there was a non-negligible sensitivity to variations of lower mantle viscosity. Local continental levering effects caused the former sensitivity to vary dramatically from site to site; in contrast, the latter senstivity resulted from long-wavelength ocean loading and syphoning effects and, therefore, showed a similar trend from site to site. Thus, although the DSL predictions (which consider the difference in the highstand amplitude between pairs of sites) retained a strong sensitivity to variations in the upper mantle viscosity, the sensitivity to lower mantle viscosity variations was partially cancelled. Nevertheless, we found that at many site pairs, the sensitivity of DSL predictions to a bulk variation in the lower mantle viscosity was comparable to a similar bulk variation in upper mantle viscosity. The sensitivity of the DSL predictions to changes in lithospheric thickness was also examined; site pairs that lie parallel to the broad, levering-induced tilt of the coast show less sensitivity to this parameter than do site pairs that lie perpendicular to the tilting (see NL).
The inversions were based on synthetically-generated DSL highstands, beginning with results based on a prior assumption of a two-layered viscosity model. In this case, the inversion of DSL from 14 pairs of sites showed a relatively slow convergence, indicating that, despite the logarithmic parametrization of the viscosity, the inverse problem retains significant non-linearity. The rate of convergence significantly improved when a larger set of 50 synthetic DSL data were used. Degrading the original 14 DSL highstands by including random observation errors did not significantly reduce the rate of convergence; however, the converged values of the lithospheric thickness and the upper mantle viscosity did not match the earth model used to generate the synthetics. Furthermore, an accurate inference of mantle viscosity on the basis of DSL highstand requires a simultaneous estimate of (a weakly constrained) lithospheric thickness.
Next, we performed an inversion in which no prior correlation between model parameters was included. In this case, the posterior covariance matrix indicated that the data resolved the volumetric average viscosity within three radial regions extending from 110 to 270 km, 320 to 570 km and 1225 to 2265 km depth. The posterior volumetric averages computed over these regions, as well as the lithospheric thickness, were all in excellent agreement with the model values used to compute the synthetics. Moreover, the posterior variances of these averages were reduced by a factor of 3–7 from the prior variances. This indicates that the DSL highstand data have a resolving power that is better than is captured by forward analyses based on isoviscous upper and lower mantle regions.
In future work, we will extend these estimates of resolving power to invert a set of DSL highstands compiled from the geological record. A preliminary analysis of the specific observational constraints adopted by NL at the 14 site pairs listed above was characterized by relatively unstable inversions. Accordingly, our extended analysis will incorporate newer observational constraints collected over the last two decades (e.g. Belperio et al. 2002). The synthetic inversions described above (e.g. Fig. 8) suggest that it may be possible to improve the resolution of radial viscosity structure inherent to the NL data set, particularly in the shallow lower mantle, by extending the number and coverage of the DSL site pairs. Constraints derived from an improved and expanded data set could then be compared with those derived from other load-insensitive regional data sets (site-specific decay times from Hudson Bay and Fennoscandia, and the Fennoscandian Relaxation Spectrum) to formally assess the necessity of invoking lateral variations in Earth structure.
Acknowledgments
This work was supported by GEOIDE, NSERC, and the Canadian Institute for Advanced Research.
References