-
PDF
- Split View
-
Views
-
Cite
Cite
Herwig Bachmann, Douwe Molenaar, Filipe Branco dos Santos, Bas Teusink, Experimental evolution and the adjustment of metabolic strategies in lactic acid bacteria, FEMS Microbiology Reviews, Volume 41, Issue Supp_1, August 2017, Pages S201–S219, https://doi.org/10.1093/femsre/fux024
- Share Icon Share
Abstract
Experimental evolution of microbes has gained lots of interest in recent years, mainly due to the ease of strain characterisation through next-generation sequencing. While evolutionary and systems biologists use experimental evolution to address fundamental questions in their respective fields, studies with lactic acid bacteria are often more directed by applied questions. Insight into population and genome dynamics are valuable for experimental design and data interpretation, and it is becoming increasingly apparent how different constraints limit and govern the outcome of microbial adaptation to a selective environment. Examples for such constraints are the finite membrane and cellular space which can lead to trade-offs between cellular strategies. A powerful perspective is that of resource allocation, which allows cells to maximise fitness. This impacts on metabolic strategies that have different protein/resource demands. This review focuses on parameters and forces that shape cellular optimisation processes and that are determining for the outcome of laboratory evolution experiments. Phenotypic changes of experimentally evolved lactic acid bacteria will be discussed in the light of the selection conditions and the prevailing constraints.
INTRODUCTION
Setting the scene
Lactic acid bacteria (LAB) are Gram-positive, non-spore forming, aerotolerant bacteria that display fermentative metabolism with usually lactic acid as the main metabolic end product. This classification is based on functional properties and it includes genera such as Lactococcus, Lactobacillus, Enterococcus, Pediococcus, Streptococcus, Leuconostoc and Oenococcus (Makarova et al.2006; Makarova and Koonin 2007). Typically, LAB are found as dominant organisms in many spontaneous fermentations of food and feed.
The fermentation of different foods was originally a spontaneous process that was predominantly determined by the microbes present in the raw materials. As the variation in the microbial community on such raw materials is considerable, such wild fermentations are difficult to reproduce and standardise. The need for consistent food quality culminated in the much better defined fermentation processes of today. In short, control is achieved through the pasteurisation of the raw materials and the subsequent use of starter cultures. Such cultures can consist of single strains or of (un)defined mixtures of strains and they are a major determinant for the organoleptic properties of the final product (Smid and Kleerebezem 2014). For instance, lactococci are often responsible for the acidification of cheese while so-called adjunct cultures (often lactobacilli) contribute little to acidification but are added for their role in flavour formation. Exopolysaccharide-producing LAB are used to alter textural properties, e.g. of yoghurt (Sieuwerts et al.2008). Next to the use of dairy starter cultures industrially produced, LAB are also used for the malolactic fermentation in wine, sausage fermentation, the conservation of silage or probiotic formulations.
Improving desired functionalities
Typically, the bacterial culture applied has a specific function in the fermented product; moreover, the strain or culture should be easy to produce and preserve. To achieve this, strain selection and improvement programmes are employed to either identify isolates or to select mutants with the preferred phenotypic properties. Phenotypes of interest include phage resistance, increased growth and acidification rate or biomass yield, increased formation of flavour volatiles or exopolysaccharides or altered sugar metabolism that allows to produce products with a lower caloric content (Derkx et al.2014).
Over the past decades, metabolic engineering strategies have been developed in many LAB to improve properties of industrial interest (Gaspar et al.2013). These studies significantly contributed to our knowledge on the metabolism of LAB and often they achieved impressive phenotypes. However, such strategies also have their limitations. First, dedicated knowledge on genes and pathways is needed for engineering strategies. Such knowledge is often inferred from other, known organisms and increasingly it is obtained from metabolic modelling approaches (Levering et al.2012). Nonetheless, metabolic engineering is based on existing knowledge and therefore unknown but potentially interesting cellular strategies will be missed. A second limitation is that for metabolic engineering the strain of interest needs to be genetically accessible which is often not the case for industrially relevant organisms (de Vos and Hugenholtz 2004; Daas et al.2016). It is for this reason that metabolic engineering strategies are often carried out in laboratory strains that were cured of prophages and plasmids (Górecki et al.2011; Tarazanova et al.2016). Finally, the approval of genetically modified organisms is very difficult in many countries and consumer acceptance of it is low. Therefore, it is currently not applied for industrial food fermentations.
As an alternative, strain improvement can be achieved by repetitive rounds of selection, of strains with the desired phenotype. Such strategies do not require recombinant DNA technology and they are more generic and applicable to all organisms. Drawbacks of such a selection regime are (i) a limited number of selective environments that can be created; (ii) the lack of control over the changes that are selected for and any non-desired changes that are co-selected; and, until recently, (iii) the very laborious process to characterise selected strains and the mechanisms underlying the phenotypic improvement. The latter rapidly improved with the emergence of next-generation sequencing technologies, and it led to a surge of activities in laboratory evolution, both in academia and industry. The other issues remain, but we will discuss that a deeper understanding of the driving forces of evolution—and the governing constraints—allows for creative designs and more predictable outcomes of experimental evolution experiments.
In this review, we will give an overview of what we think are the relevant parameters, forces and constraints that determine the outcome of a laboratory evolution experiment. A focus will be on how constraints can lead to trade-offs at the cellular level (e.g. proteome), and subsequently, what their impact might be on the metabolic strategies that cells deploy. We will review work on the experimental evolution of LAB from these perspectives and point out different experimental systems that can be exploited to select for a specific phenotype.
EXPERIMENTAL EVOLUTION AND PRINCIPLES GOVERNING ITS OUTCOME
Experimental evolution
Experimental evolution of microbes typically entails the prolonged cultivation of an organism in a controlled environment for hundreds to thousands of generations. Throughout the experiment parts of the population will be preserved and genotypic, as well as phenotypic, properties can be determined to assess the changes that occurred in the population over the course of the experiment. The culture conditions applied will have a major impact on the types of mutations that will be selected for, and it is therefore of importance to understand the underlying forces and population dynamics. Several principles governing the outcome of experimental evolution will be discussed in the following sections.
Fitness
During experimental evolution, there are a number of parameters that are important to understand the underlying population dynamics, which can aid in the experimental design. Obviously, central to all changes that are going to be observed is the fitness of the organisms that take part in the experiment. Fitness, however, is a concept with multiple facets. We have defined it previously (Bachmann et al.2016) as: ‘A relative measure of the success of replication of organisms that compete for the same external resources, measured as a consistent change of the population ratio of these organisms over a period of several generations’. An organism's fitness is thus determined by the environment and its capability to survive it and reproduce in it. The most sensitive assay to measure fitness is a competition assay, where for instance the wild type and an evolved strain are inoculated in equal ratios and subsequently co-cultured in the environment of interest. After one or more culturing steps, the ratios of the individual strains can be determined, either through the use of selective markers that were introduced initially or through deep sequencing of alleles that differ between the strains. The resulting data can be used to calculate the fitness of the individual strain (Elena and Lenski 2003).
During an evolutionary trajectory, multiple solutions can lead to a fitness increase of an organism, which is often visualised in a so-called fitness landscape. A fitness landscape is typically shown as a 3D plot where the x-axis and y-axis show for instance genetic variation of an organism and the z-axis shows the fitness of this genetic variant in a particular environment (Rabbers et al.2015). An evolutionary trajectory can be shown as a ‘walk’ through such a landscape and because selection favours fitter variants they will typically walk uphill, towards a (potentially local) peak. This can lead to organisms being stuck in a local optimum. It is generally assumed that if a population is placed in a new environment it is at some distance from a fitness peak and the longer it is propagated in the environment the closer it will move towards it. At the same time, variants with a higher fitness will move slower towards a fitness peak as a consequence of diminishing returns (Barrick and Lenski 2013).
The dynamics and degree of changes in environmental conditions are important in determining whether beneficial mutations can increase in frequency and fixate in the population. In very stable environments like a continuous culture, the selective pressure will be continuously on the same phenotypic property, and the rate of fixation will therefore depend on the fitness advantage conferred by the individual mutations. In an unpredictable environment that exposes an organism haphazardly to all kinds of conditions like different pH and temperature, a specific phenotypic characteristic might be selected for in one of the conditions but counterselected for in the subsequent one. An extreme case of unpredictability would be conditions that do not permit growth anymore. In between these extremes would be the repeated transfer in batch cultures, which can lead to a predictable time schedule of conditions i.e. exponential growth followed by a period of stationary growth phase, or environments that fluctuate between two or more stable conditions. The latter can be done in fixed intervals at different frequencies, which will determine the likelihood of evolving specific strategies. This is demonstrated by selection of yeast in environments with changing carbon sources. It resulted in mutants with altered catabolite repression properties leading to either generalist or specialist strategies (New et al.2014). Very interesting growth and survival strategies like bet-hedging or altered storage of compounds can also be selected in such environments (Beaumont et al.2009; Jiang et al.2014; Solopova et al.2014).
Another important part of the fitness equation is determined by changes brought about by other organisms. When mutants appear, conditions will change, even in a continuous culture. For example, mutants that have a higher affinity for substrates will decrease the substrate concentration when present in high frequencies. The presence or absence of others changes the environment, and thereby the fitness, and, consequently, the course of evolution (Bachmann et al.2011). A metaphor used to convey this idea is that of the dynamic fitness landscape, i.e. how the fitness changes with different chains of successive mutations. This makes fitness a moving target, in fact moving in unpredictable ways because the appearance of future mutants and their effects on the environment is to some degree unpredictable. As the number of species in an environment varies, they and their mutants will lead to even more complex effects on fitness. This results in an effect called frequency-dependent selection. It means that the fitness effect of one strategy depends on the strategies of others, and on the relative frequencies of these strategies in the population.
Finally, the biological material itself determines the fitness of a cell. The organism and its cellular constituents (genes, proteins, metabolites) provides what we will call cellular constraints: some of these constraints are hardwired and physico-chemical in nature, such as the solubility or diffusion rate of compounds; others are biological and can be molded by evolution to increase fitness.
Mechanisms of genetic variation
The mutation supply rate is the product of the mutation rate and the effective population size (Gerrish and Lenski 1998; de Visser et al.1999). While in experimental evolution, as well as for industrial strain improvement, mutating agents are regularly used to increase the mutation supply, this might not always have the desired effect on accelerating adaptive evolution. It was shown theoretically and experimentally that clonal interference leads to competition between variants with different beneficial mutations within a population. In asexual populations, this puts a speed limit on adaptive evolution (Gerrish and Lenski 1998; de Visser et al.1999) indicating that, given a sufficiently big population size, an increased mutation rate might be of limited value. In contrast, sexual reproduction removes the speed limit imposed by clonal interference through the possibility of combining different beneficial mutations in one lineage.
More than a single evolutionary trajectory may lead to an evolved ‘optimal phenotype’. If one defines the end point of an evolutionary experiment as the particular optimal phenotype, then there will be additional factors besides the ones described above, which will condition the outcome. In essence, the deeper the scanning of the sequence landscape, by increasing the likelihood of more combinations of spontaneous mutations to occur within any given individual of the population, the more likely the end point of the evolution experiment is not stuck in a local optimum. This can be prevented by increasing the population size, or increasing the length of the experiment. As it is not possible to scan all sequence permutations that may occur for any given organism, it is impossible to know whether or not the end point is stuck in a local optimum. Interestingly, even after 50 000 generations of experimental evolution beneficial mutations are still being selected for, indicating that after such an extended period cells do not seem to be stuck in a local fitness peak but are still evolving (Tenaillon et al.2016).
Population size, selective pressure and genetic variation determine population dynamics
Apart from the selective advantages set by the environment, there are other design parameters that affect the course of evolution (Perfeito et al.2007). Important ones in this context are the effective population size, which is strongly dependent on population bottlenecks occurring, for example, at inoculation of repetitive batch cultures, and clonal interference, which is particularly important in clonally, i.e. asexually reproducing organisms. Evolution depends on the presence of genetic variation in a population. Genetic variation determines the rate at which a population can adapt to an environment. The level of genetic variation or genetic variance of a population changes over time and is the result of processes that promote its appearance and disappearance. A high mutation rate leads to a high genetic variance, but a large population also contains more variance than a smaller.
The interplay of effects of population size, mutation rates, selection, etc. on genetic variance has been studied in theoretical idealised reproduction models. A null model of this type assumes, for example, a constant population size, absence of selection, equal chance of reproduction for all individuals and random mating if applicable. Real populations are not constant in size, and are subject to selection, and will, at equal population size as an ideal population, have lower genetic variance. To quantitate the effects of such processes on genetic variation, their effect on the effective population size of a population is calculated. The effective population size of a population is the number of individuals Ne of a constant, ideal model population that would contain the same amount of genetic variation as the true population. Usually, Ne is much smaller than the time-averaged size of a true population. For example, for a repetitive batch transfer the effective population size approximately equals the population size at the time of inoculation (N0) times the number of generations (g) in each batch, i.e. Ne ≈ N0 × g (Lenski et al.1991).
The effective population size not only determines the genetic variance of a population but also its capacity to fixate a mutation. Mutations with small beneficial effects on fitness will only be able to fixate in populations with large effective population size. In small effective populations they cannot escape the random effects of genetic drift, and will usually be lost (Kimura 1983). However, other neutral, less beneficial or even slightly detrimental mutations will accumulate and can be fixed by chance in small-sized populations. Hence, selection and fixation of beneficial (adaptive) mutations only happen in large enough populations. For the design of an evolution experiment, it is not so much a correct calculation of the effective population size Ne per se that is of importance, but rather the realisation which factors affect genetic variation and how strong these effects are. Most importantly, a very low effective population size severely reduces both rate of evolution and reproducibility of evolution experiments.
Selection itself reduces genetic variation because a genetic variant with high fitness displaces variants with lower fitness (Fig. 1). In clonal, asexually reproducing populations like bacterial populations, this can lead to an extreme situation where one mutant with very high fitness overtakes a complete population. This is called a selective sweep. Temporarily, this leads to an extremely low genetic variation, because only a single clone with a highly beneficial mutation is fixed in a population at the cost of all other mutations. This effect is called ‘clonal interference’ (Imhof and Schlotterer 2001). In sexually reproducing populations, the effect of selection on decrease in genetic variation is less pronounced because other, less beneficial mutations can be mixed in, through recombination, with the most beneficial mutation before it is fixed in a population.
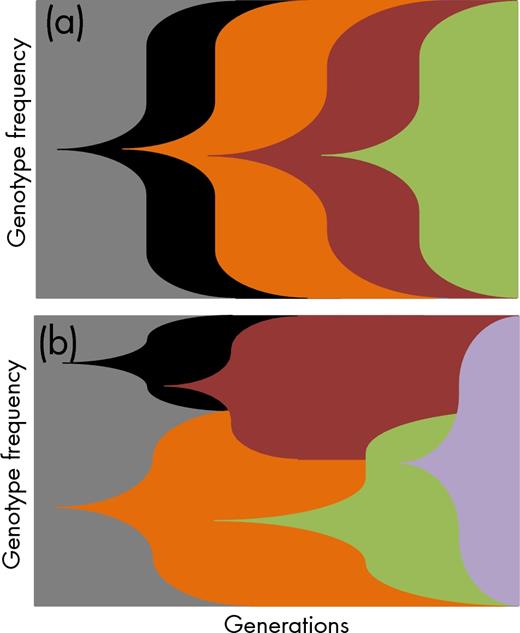
Muller plots showing the succession of genotypes in a culture throughout experimental evolution. If the mutation supply rate is low typically, one mutation will get fixed before a new mutation occurs (a). If the mutations supply rate is high through, e.g. higher mutation rates or bigger population sizes, multiple genotypes will compete within a population. This allows for the selection of more complex genotypes as more beneficial mutations can accumulate in the competing variants (b). Adapted from Barrick and Lenski (2013).
Any less beneficial or even detrimental mutations that were present in the original mutant may also be fixed. These are said to hitchhike on the selected beneficial mutation. A type of mutation that is often found to hitchhike on beneficial mutations is one causing a hypermutable phenotype (Sniegowski, Gerrish and Lenski 1997). Such mutator mutations themselves are usually detrimental, or at best neutral for fitness, but the large number of putatively beneficial mutations that arise in them often cause their temporal rise and fixation in a population under selective pressure.
While it might seem obvious we would also like to note that the time of fixation of a particular mutation is of importance for the interpretation of the genotype diversity in an evolving culture. Early appearance of a mutant would lead to a higher frequency in a culture but that does not necessarily mean that it occurs at a higher rate. This realisation is important for the determination of mutation frequencies which are often based on the spontaneous emergence of antibiotic-resistant mutants. This was already realised by Luria and Delbrück (1943) who developed a dedicated protocol for the determination of mutation frequencies termed a fluctuation assay.
THE ROLE OF CONSTRAINTS
At any time scale, cells will reach the best possible response and corresponding fitness, where ‘best’ implies that eventually, at some place, some constraint is being hit. What the nature of the constraint is, is very much dependent on the environment and the organism's make up. Under nutrient excess conditions, such as in early batch growth on a rich medium, it is likely that internal cellular constraints limit the growth rate, such as the genome duplication rate or the protein synthesis rate. In a nutrient-limited chemostat, uptake is most likely a constraining factor, rather than internal machinery. It is, however, not always clear which constraint is being hit under which conditions, for example, under stress.
Moreover, there are many different constraints, at many different levels (Fig. 2). They are either physical/chemical or biological in nature, and act at the protein level, at the proteome level, at the cellular level or even at the population level. The environment can also put constraints on the systems, or pushes some constraint over some others. For example, it was recently shown in yeast that the cost of making protein (defined as the decrease in specific growth rate upon protein overexpression) depends on the conditions: under nitrogen limitation translation bears most of the costs, but a phosphorus limitation shifts this to transcription, most likely due to a shortage of nucleotides (Kafri et al.2016).
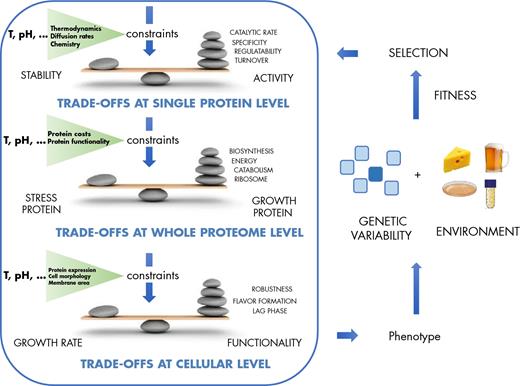
Cellular constraints occur at different levels. Examples: At the single protein level, activity trades off with stability, at the proteome level stress proteins trade off with growth related proteins and at the whole cell level, growth rate could trade off with functional properties such as robustness or lag times. Exposure of the resulting phenotype to a given environment will select for mutants with alterations in their protein allocation.
In many cases, some of the constraints affect different functionalities at the same time, and when this happens, trade-offs occur between these functionalities. For example, it was shown in many different organisms that growing fast comes at the cost of stress resistance (Ihssen 2004; Brauer et al.2007). Conversely, stationary Lactococcus lactis cells tend to be more robust to stress (Rochat et al.2005) and increased stress tolerance in LAB was also found that under near zero growth conditions (Ercan et al.2015). Fast growth also often comes at the cost of biomass yield (Jasmin, Dillon and Zeyl 2012; Bachmann et al.2013). A study investigating temperature adaptation in Escherichia coli showed that about 1/3 of 24 lines adapted to growth at low temperature showed no high temperature trade-off, where else the other strains did (Bennett and Lenski 2007). This indicates that such a trade-off is generally likely but it does not always occur. It is also important to realise if the phenotype of an organism is far from an optimum, improvements of two properties that potentially trade-off with each other can still be made until the phenotype hits a trade-off line (Bachmann et al.2015). Underlying mechanisms can be at the protein level, the cellular level or a combination of the two. While for an individual phenotype the mechanistic explanations are most likely found at the protein or cellular level during evolution genome dynamics itself play an important role.
Constraints at the DNA level
Although the number of generations that can be encompassed during experimental evolution might seem relatively high, it is in general not long enough to allow for the evolution of novel proteins or protein functions. Directed enzyme evolution is a term used in protein engineering and it comprises repetitive rounds of mutagenesis and selection for particular enzyme activities. This approach did allow for the selection of new enzymatic activities but typically few mutations are giving big effects on enzyme activity and diminishing returns and trade-offs constrain further optimisation (Tokuriki et al.2012). It is at least partially due to these limitations that mutations that are often found to give rise to new phenotypes are not related to the evolution of new functionalities but rather to changes in the expression levels of existing genes such as global transcription regulation or the upregulation or downregulation of key enzymes/transporters involved in a particular phenotype. For instance, the upregulation of a cellobiose PTS system that also transports lactose with a low affinity was found to be responsible for the appearance of lactose-positive mutants in populations of a lactose-negative L. lactis strain (Solopova et al.2012). Similarly, the upregulation of peptide transporters allowed a L. lactis plant isolate to grow much better on milk (Bachmann et al.2012). The limited number of proteins in an organism does therefore constrain experimental evolution.
However, there are several mechanisms at the DNA level that allow for a more rapid innovation within an organism than simple mutational events such as single nucleotide polymorphisms, small deletions or insertions. The most obvious one—but not so relevant for laboratory evolution—is horizontal gene transfer. Such transfer of functional genes from other organism is well described and it was shown to play an important role during the evolution of LAB (Makarova and Koonin 2007). In LAB, insertion sequences (IS), which are defined as small (<2.5 kb) DNA segments that only encode information for their own transposition to multiple sites in a target molecule, are relatively common. In L. acidophilus, 17 of such IS elements are found (Altermann et al.2005) while in lactococci up to 130 (Wegmann et al.2007) and in L. helveticus DPC4571 213 IS elements are described (Callanan et al.2008). The mobilisation of IS elements was found to occur with high frequencies in different evolution experiments with LAB. In a L. lactis, lactate dehydrogenase mutant IS981 (Bongers et al.2003) and IS905 (Gaspar et al.2007) was found to activate an alternative (ldhB) gene after selection for fast growth. The transposition of either IS element led to the insertion of a –35 promoter element at the correct spacing upstream of the native –10 promoter region of ldhB, thereby activating transcription. Interestingly, in other selection studies, the same principle of IS-mediated promoter activation was found to upregulate an inactivated nisin production gene cluster (Dodd, Horn and Gasson 1994) or at multiple occasions glucose transport (Bachmann et al.2013). In another study, the experimental evolution of L. lactis for 1000 generations under various environmental conditions (growth and starvation, shaken and stationary) identified 9 IS981-mediated mutations in 12 parallel evolved populations (de Visser et al.2004). The above examples indicate that IS elements can quickly affect transcription levels of individual genes in LAB and that it occurs at high frequencies. Despite the importance of IS elements seen in numerous experimental evolution studies, genome rearrangements such as IS transposition are not always analysed in re-sequencing data. This is caused by the high number of IS elements in LAB which, due to high-sequence similarity, make de novo assemblies but also read mapping on template genomes difficult.
The results of re-sequencing 264 clones taken at regular intervals throughout 50 000 generations lasting evolution experiment with E. coli gave interesting insight into the genome dynamics. Out of 12 parallel evolved lines, 6 lines accounted for more than 96% of the mutations found. These lines evolved hypermutable phenotypes due to alterations in DNA repair mechanisms. Interestingly, four populations that were hypermutable after 10 000 generations showed a decline in synonymous mutations between 40 000 and 50 000 generations which was explained by the appearance of antimutator alleles (Wielgoss et al.2013; Tenaillon et al.2016). Similarly to the findings in E. coli, such mutator phenotypes were also identified during the experimental evolution of L. lactis (Bachmann et al.2012) and L. rhamnosus (Douillard et al.2016). Another remarkable finding of 50 000 generations of laboratory evolution is that the average genome length of the 12 propagated lines declined with 64 kb (∼1.4%) relative to the ancestor strain (Tenaillon et al.2016). Such genome reduction is consistent with the idea that the adaptation to a relatively rich or stable environment is accompanied by the loss of genes not necessary in that particular environment. This mechanism is also thought to be responsible for the relatively small genomes of some LAB that are well adapted to rich environments (Makarova et al.2006; Makarova and Koonin 2007). Recently, the genome of a frequently used laboratory strains of lactococci, NZ9000, was ‘streamlined’ by the deletion of four non-essential DNA regions that account for 2.83% of the total genome. This alteration in the genome lead to increase in the growth rate and a 3- to 4-fold increase of nisin-induced expression of different heterologous proteins (Zhu et al.2017), suggesting that the burden of non-essential DNA and its expression products in a cell can be considerable.
Positive epistatic interactions between different genes require the occurrence of particular combinations of mutations, which due to low frequency constrain evolutionary trajectories. In other words, particular phenotypes might be difficult to select for, not because of physical or biochemical limitations, but rather because a particular combination of mutations would have to co-occur in the same organism which is an unlikely event and can lead to organisms being stuck in a local fitness peak. A study addressing such evolutionary constraint found that the repeated switching between different environments allowed organisms to move out of local fitness peaks. The authors explained this by pervasive cross-environmental trade-offs that, by alternating selection, allow a repositioning of fitness peaks in a way that organisms can pass through fitness valleys (de Vos et al.2015).
While mutation rates seem relatively similar for most bacteria, it is interesting how some organisms evolved mechanisms that lead to a seemingly more dynamic genome such as the mentioned species with a high number of IS elements. When it comes to mutation rates an exception within LAB is Oenococcus oeni, which does not have DNA mismatch repair systems. This leaves Oenococcus with a mutation rate that is 10- to 1000-fold higher than in phylogenetically closely related species such as Leuconostoc and Pediococcus (Marcobal et al.2008). While it seems not clear what the evolutionary advantage of such an increased mutation rate would be, it would be interesting to see if it can lead to faster adaptations during adaptive evolution experiments or if a lower mutation rate would evolve in time as seen in a long-term evolution experiment with E. coli (Tenaillon et al.2016).
Although effects on the DNA level cannot easily be seen loose from the proteins the DNA encodes, it is clear that there are several constraints imposed by the DNA and genome dynamics which play a role during adaptive evolution.
Constraints at the protein level
It is generally accepted that the construction of an active site in an enzyme is thermodynamically unfavourable because functional amino acid residues are often polar or charged or catalytic residues have unfavourable backbone angles (Tokuriki et al.2008). The protein sequence of an enzyme is usually optimised to ensure high function and stability. During enzyme evolution, changes in the catalytic site that would improve substrate affinity will therefore only have a reasonable chance of fixation if they do not significantly decrease enzyme stability. However, since kinetic parameters are constrained by thermodynamics, as e.g. apparent from the Haldane relationship (Cornish-Bowden 2014), changes in catalytic activity and substrate affinity are not independent, and hence will trade off. An increase in the kcat of an enzyme thus has to be compensated for by either a lowered affinity (Km) or increased kcat in the reverse direction. It is for these reasons that an understanding of mutational effects is important for the interpretation of population dynamics in selection experiments, as well as for engineering strategies (Tokuriki et al.2008).
The analysis of 548 individual mutations that resulted from the directed evolution of 22 enzymes revealed that mutations that modulated function had in general a destabilising effect on the protein. Interestingly, it was also shown that mutations with no obvious effect on catalytic function seemed to have a stabilising effect on the protein. From this, the authors conclude that the evolution of new functions is dependent on compensatory, stabilising amino acid changes in protein regions that are irrelevant to its function (Tokuriki et al.2008).
Probably one of the best studied constraints at the protein level relates to thermostability and enzyme activity where a temperature rate dependence seems generally accepted (Elias et al.2014). An interesting experiment termed a ‘weak link’ approach encompassed the chromosomal replacement of the wild-type adenylate kinase (adk) in the thermophilic bacterium Geobacillus stearothermophilus with that of the mesophilic bacterium Bacillus subtilis. After this gene replacement, the strain could not grow at temperatures higher than 55°C anymore due to Adk denaturation. Cultivation of the strain for 1500 generations in a turbidostat with a gradual temperature increase from 55°C to 70°C resulted in six mutant alleles allowing Adk to function at high temperature while remaining maximum enzyme activity. Detailed analysis showed that enzyme stability and function are the main determinants for the outcome of evolution (Couñago, Chen and Shamoo 2006; Peña et al.2010).
In lactococci, keto-acid decarboxylases (Kdc) are known for their function of converting branched chain keto acids into aldehydes that give a malty chocolate-like flavour with a very low odour threshold (Smit, Smit and Engels 2005). In an unrelated application, the same enzyme is also used for the production of keto acid derived higher alcohols like isobutanol which is preferably carried out with thermophilic bacteria. As only two enzymes with Kdc activity are reported for thermophiles, a directed evolution approach was taken to engineer keto isovalerate decarboxylase (kivd) of L. lactis to be thermostable. For this purpose, almost 8000 kivd mutants were screened for enzyme activity after high temperature treatment and eventually point mutations giving the desired effect were combined. This resulted in a kivd variant with 10.5-fold increased residual activity after 1 h pre-incubation at 60°C and 13°C increase in melting temperature (Soh et al.2017). With the increase in thermostability also the kcat measured at 50°C more than doubled in comparison to the wild-type gene. It would be interesting to know if this adaptation came at the cost of decreased activities at low temperatures but these data were not reported by the authors. Given the constraints dictated by chemistry and physics that act on proteins, such costs and in general trade-offs between desired properties of enzymes will be unavoidable. In the next section, we will discuss how such constraints act at the cellular level.
Constraints at the cellular level (resource allocation)
In the last decade, constraint-based modelling of genome-scale metabolic networks has become very powerful, also for LAB (Teusink and Smid 2006; Saulnier et al.2011; Xu et al.2015) to either predict the implications of environmental constraints on growth (e.g. of L. plantarum; Teusink et al.2009), to identify the relevant constraints from experimental data (Teusink et al.2006; Stevens et al.2008) or to explore metabolic capabilities, including flavour formation, such as in Streptococcus thermophilus (Pastink et al.2009) or L. lactis (Flahaut et al.2013). However, these models only act at the metabolic level, and hence, they miss much biology and the corresponding relevant constraints that are needed to explain many adaptive phenomena relevant for LAB. One of these is the failure of genome-scale metabolic models to a priori predict lactic acid production as an optimal strategy in LAB. Yet, we find in many adaptive evolution experiments with LAB that faster growth is accompanied by more lactate formation (Bongers et al.2003; Bachmann et al.2013). The reason why optimisation with genome-scale metabolic models does not work for LAB has been fully explained, e.g. in the study by Teusink et al. (2009); in short, stoichiometric models predict only yields (Santos et al.2011), and in most experimental setups—and in nature—growth rate is more important for fitness. We will come back to the mystery of lactic acid production by LAB—or the general phenomenon of overflow metabolism in many microorganisms—a number of times in the remainder of this review, as there are a number of different explanations for it. The explanation that we believe is the most powerful will be explained next.
In recent years, a powerful paradigm has been developed that can explain many metabolic regulatory strategies, and which is complementary to genome-scale modelling. It is the so-called resource allocation perspective, i.e. the idea that cellular resources are limited, and hence provide constraints on the functioning of cells. A system resource is a quantity of a system that can, for the sake of reasoning, be regarded as conserved on the short term. For example, when the system is a factory, conserved resources are its financial buffer, its working space and its employees. These quantities will not change quickly, and all decisions made concerning production processes have to be taken within the boundaries of these limits on system resources. For example, investment in one production line can only take place at the cost of investment in another line, because these investments use the same limited financial buffer. Investment decisions will often depend on the anticipated profit of the alternatives. Similarly, trade-offs exist in a microorganism between the synthesis of proteins for different metabolic pathways. In fact, the synthesis of all proteins is limited by the total amount of protein in a cell. Just as the profit of a factory will be determined by the type and amount of products that it produces, so will the fitness of an organism be determined by the nature and concentration of proteins.
This simple view of cellular economy is helpful in reasoning about growth strategies (Beg et al.2007; Molenaar et al.2009). Indeed, in an earlier LAB review (Teusink, Bachmann and Molenaar 2011), we explained the lack of flux control by glycolytic enzymes as found by Peter Jensen's lab (Koebmann et al.2002), in view of such optimality—in the optimum the derivative of the flux to a change in protein level will be 0. In a very recent more extensive study of 85 proteins in Saccharomyces cerevisiae, the Segal group found that wild-type expression levels were optimal under glucose growth conditions, but less so under galactose growth—illustrating perhaps the predominance of glucose as carbon source in nature—and the laboratory (Keren et al.2016). Similarly, the condition-dependent proteome of E. coli (Schmidt et al., 2015) combined with a genome-scale model that allows the prediction of protein utilisation revealed that under certain conditions almost half of the proteome is unused and that during experimental evolution the fraction of unused proteins decreased (O’Brien, Utrilla and Palsson 2016). Another example is the regulation of the protein synthesis machinery, like ribosomes and associated protein factors, relative to all other anabolic, catabolic and structural proteins is such that it maximises the growth rate at different substrates (Scott et al.2014; Bosdriesz et al.2015). The experimental observations on this regulation are in accordance with models of cellular economy that assume a constant total protein resource, leading to a trade-off between investment in the synthesis of the different protein fractions (Scott et al.2010).
This total protein resource allocation perspective has been very powerful in explaining many aspects of metabolic regulation, at least in model organisms such as E. coli and S. cerevisiae. This includes catabolite repression (You et al.2013; Wortel et al.2014), responses to antibiotics treatment (Scott et al.2010) and overflow metabolism cq. the (bacterial) Crabtree effect (Molenaar et al.2009; Basan et al.2015). In the latter case, respiration is observed at low growth rates while cells start to produce fermentation products such as lactate, ethanol or acetate at increasing growth rate. The explanation is that respiration is the more metabolically efficient mode of energy catabolism—it provides much more ATP per glucose—but requires more protein resources than overflow metabolism or fermentation (The difference between fermentation and overflow metabolism is not always made very clear. Fermentation is the process by which excess catabolic electrons are disposed off by reduction of an endogenous substrate, as opposed to an external electron acceptor such as oxygen or nitrate (which is then called respiration). In this sense, acetate production by E. coli is overflow metabolism, not fermentation). As protein investment into biosynthesis increases with growth rate, including ribosomes, the protein fraction available for energy catabolism becomes smaller, and higher growth rates can only be obtained by trading in protein—costly machinery for cheap fermentation machinery—at the cost of yield. This then could also explain a trade-off that is often observed between growth rate and growth yield: efficiency apparently costs more protein. Why this is necessary per se, however, i.e. which constraints at deeper levels are causing this trade-off, is still not entirely clear to us. Thermodynamics has been suggested as an explanation for the trade-off between rate and yield (Pfeiffer and Bonhoeffer 2002), as chemical flux near equilibrium is proportional to free-energy dissipation, and so, being fast requires ‘wasting’ free energy. However, it remains unclear how this thermodynamic relationship extends far from equilibrium and with the biological option of enzyme catalysis. Yet, it is clear that metabolic reactions that operate close to equilibrium experience less thermodynamic driving force and thus require more or faster enzymes to sustain high flux (Noor et al.2016), which brings us back to protein costs.
There are, however, also indications that total protein is not the only cellular resource constraint leading to trade-offs and affecting optimal regulation. Many LAB, notably L. lactis, show an anaerobic version of the (bacterial) Crabtree effect, i.e. a shift between mixed acid and homolactic fermentation at increasing growth rate and or sugar levels (Goel et al.2012). In a recent extensive functional genomics study on this effect in L. lactis MG1363, it was found that (cytosolic) protein levels do not seem to constrain metabolism at all: a 4-fold change in growth rate and hence flux was accompanied by minor changes at the mRNA and protein level (Goel et al.2015). The lack of protein-level regulation under glucose limitation was also found in yeast (van Hoek, van Dijken and Pronk 2000) and B. subtilis (van Hoek, van Dijken and Pronk 2000; Chubukov et al.2013), but is in contrast with earlier studies in L. lactis IL1403 (Lahtvee et al.2011). The latter study was done in A-stats, i.e. in continuous cultures where the dilution rate is gradually increased over the course of the experiment, rather than that different chemostats at different dilution rates are compared. It is unclear if that could explain the difference in physiology observed.
The lack of protein-level regulation in these studies may be explained by the nutrient-limited conditions in the chemostat, which could indicate that constraints at the transport or membrane level are more relevant in this case. In fact, there is debate over whether conserved total protein, conserved total space in the cell (also called ‘crowding constraint’) or conserved total membrane proteins are the system resources that explain the optimality of observed shifts in metabolic strategies, and in particular the shift between respiration and fermentation even under aerobic conditions (Zhuang, Vemuri and Mahadevan 2011; Vazquez and Oltvai 2016). In fact, theory tells that as long as any of these constraints hits more than one metabolic strategy—and they all do—they will cause a trade-off between these strategies (de Groot, Bruggeman, Planque, Teusink, unpublished). It is very likely that, similar to the multiplicity of system resources in a factory, all of these constraints will lead to trade-offs that are relevant under some conditions, but not under others. As stated, membrane protein constraints are likely to be more relevant under nutrient-limited chemostat conditions.
This then points very strongly to the environment as an important factor in setting the relevant constraints. What's more, the unpredictability of the environment also leads to intriguing dilemmas and trade-offs that root also in the resource allocation paradigm. Proteins invested in growth cannot be invested into stress resistance or alternative nutrient uptake systems. Therefore, the dilemmas have to do with the costs of being prepared: when, i.e. under which dynamic or unpredictable conditions, does it pay off to reduce your current fitness to be able to rapidly respond to environmental changes? How many cells of a population can be killed by a sudden stress before fitness-reducing coping strategies start to pay off? We found one example in LAB where this may be relevant—although this is speculation from our side. Arioli et al. (2013) showed that inhibition of LDH by sodium oxamate increases haem-induced respiration and subsequent (well-known; Gaudu et al.2002) robustness to starvation, but also resulted in a 1 h longer lag phase upon inoculation. This raises the question, whether such a delay is tolerated by evolution. It is clearly a trait evolution can act on (New et al.2014). There are no general answers to these questions, but awareness of such dilemmas, rooted in cellular constraints, help in understanding how the dynamic and unpredictable environment shapes fitness-enhancing strategies.
Constraints imposed by the environment
Environmental constraints are mainly physical-chemical limitations that restrict growth and they can be divided into two types. Abiotic constraints are determined by the environment itself while constraints of biotic origin are the alteration of the environment as a consequence of growth (Fig. 3).
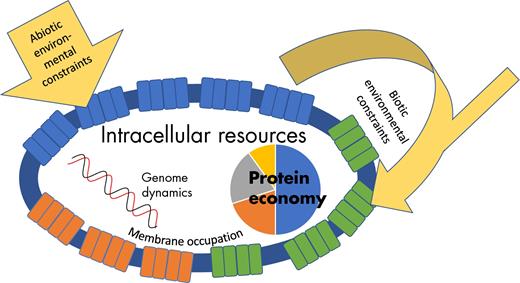
The fitness of a cell is determined by cellular and environmental constraints. Cellular constraints include the allocation of a finite amount of proteins over different functional categories and the occupation of the cell membrane. Environmental constraints can be of abiotic origin e.g. temperature or oxidative stress or of biotic origin such as production of organic acids by the organism itself or by organisms in the same environment.
Abiotic constraints
Examples for abiotic constraints are variations in growth temperature, osmolarity or acidity. As long as conditions are permissive to growth, natural selection leads to the adaptation of organisms to these constraints. The classification of bacterial species from phsychrotrophs to thermophiles indicates adaptation to different growth temperatures and is an example where abiotic constraints shape the outcome of evolution. Naturally, adaptation to a high temperature comes at the cost of decreased fitness at low temperature and vice versa, pointing to the existence of trade-offs (Bennett and Lenski 2007). However, cells are usually able to grow in a range of conditions, where not being in the optimal environment leads to what is usually considered stress response in the cell. The optimisation of a cell is therefore driven by a broad range of interacting factors which also becomes obvious when analysing laboratory-evolved organisms.
In LAB, there are several examples of strains that were experimentally adapted to environmental conditions that trigger relatively strong stress responses. The selection of L. lactis strains from plates containing 2–5 mM H2O2 resulted in seven mutants that showed good acidification properties in milk and up to 10 000-fold increased survival when exposed to oxidative stress. The authors reasoned that resistance to oxidative stress can play an important role in microbial consortia and subsequently showed that one of the isolated L. lactis mutants survived more that 1000-fold better during co-culturing with two strains of Lactobacillus delbrueckii that produced H2O2. Interestingly, co-culturing with the mutant lactococci strain also led to an increased survival of the lactobacilli. However, they could not attribute this effect to a removal of H2O2 from the consortium indicating that other interactions played an additional role (Rochat et al.2005).
The adaptation of L. lactis to growth at increased temperature (37.5°) led to the isolation of stable heat resistant mutants which, however, showed a hypersensitive response to osmotic stress. The identified strains shared mutations in llmg_1816, a gene encoding a membrane-bound stress signalling protein of the GdpP family. This protein shows c−di−AMP phosphodiesterase activity, which is strongly inhibited by the stringent response signalling molecule ppGpp. While gdpP was shown to be involved in the stress response in several Gram-positive bacteria, the underlying mechanism remains to be determined (Smith et al.2012). Interestingly, while mutations in llmg_1816 lead to heat resistant but salt hypersensitive mutants, they also identified strains that were heat resistant but did not show a trade-off when exposed to high salt concentrations. The repeated isolation of strains with a trade-off shows that in this specific case they are likely to be identified, yet this is an indication that this specific trade-off does not seem to be governed by cellular constraints. In an approach similar to the experiments by Smith et al., Lb. helveticus was selected for growth at elevated temperature which resulted in a strain with higher autolytic capacity in cheese-like conditions. The authors conclude that this approach is promising for the selection of strains that could lead to accelerated cheese ripening due to the increased enzyme release during cell lysis (Spus et al.2017).
In another study L. lactis was adapted by slowly increasing the growth temperature to 39° over a period of 860 generations. The characterisation of a mutant strain showed 33% increased growth—and 12% increased acidification—rates at 38°C. Full-genome re-sequencing revealed 15 mutations. Introduction of the individual mutations in the wild type resulted in improved thermal tolerance for some of them, but none of the single mutations were able to achieve the tolerance of the selected mutant. Mutations that were suggested to have a higher significance on the observed phenotype were described to (i) affect the expression of a chaperon and a riboflavin transporter, (ii) have global effects on transcription and fatty acid composition through a mutation in the ribosomal subunit rpoC and (iii) alter phospholipid biosynthesis through a point mutation in cdsA, a CDP diglyceride synthase (Chen et al.2015). This and the study by Smith et al. (2012) evolved L. lactis MG1363 at high temperature. While Smith et al. repeatedly finds a mutation in a c−di−AMP phosphodiesterase, this mutation was not found by Chen et al. The two studies differ in that the first one isolated individual colonies after plating and incubating them at 37.5°C while the second one gradually increased the temperature throughout serial propagation over an extended period. We do not know how repeatable the outcome of the gradual temperature increase during growth of L. lactis is, but the results indicate—not unexpectedly—that while selecting for increased fitness during temperature stress, relatively subtle environmental differences can lead to different outcomes. These results are consistent with slightly different fitness landscapes between the different environments and cells migrating towards local fitness peaks in the respective landscapes.
In addition to obvious physical constraints such as temperature or osmolarity, also the availability of substrate constrains the strategies available to a cell for growth and survival. Depending on an organism's auxotrophies, extracellular resources can be simple nitrogen, carbon and trace elements or more complex molecules such as amino acids, nucleotides or fatty acids. The importance of their availability is reflected in several cellular mechanisms that allow the sensing of nutrients, which is usually coupled to a metabolic response. In higher organisms such as yeast, such sensing mechanisms seem more prevalent and sophisticated (Conrad et al.2014) but also LAB are able to sense molecules such as amino acids (den Hengst et al.2005) or nucleotides (Bachmann, Kleerebezem and van Hylckama Vlieg 2008). To our knowledge, it is not known if LAB have also dedicated sensors for carbon sources but it is obvious that their availability has a major impact on the metabolism of a cells (this will be discussed in more detail later). Besides abiotic constraints that are independent of microbial activity, the environment can be modulated by organisms which can lead to different types of constraints.
Constraints of biotic origin
We define constraints of biotic origin as fitness-restricting conditions that occur in an environment due to biotic activity. Such constraints can originate from any organism that occupies a specific niche and it includes pure cultures that modify their environment to the extent that they restrict their own growth/fitness. Examples for the latter would be the production of sublethal levels of organic acids, i.e. lactic acid or ethanol by microbial metabolism (MacLean and Gudelj 2006). In literature it has been suggested that the biological reason for the secretion of organic acids is that this is part of a biological warfare where the secreted compound prevents competing organisms from growing because they are likely to have a lower tolerance to it (Piskur et al.2006). In the same paper, this idea was extended with the Make-Accumulate-Consume hypothesis, i.e. the excreted organic acids or alcohols can be oxidised again after the killing is over. This view was disputed with the argument that the underlying assumption of an organism spending energy on warfare would be vulnerable to invasion by cheating organisms, which does not seem to occur (Molenaar et al.2009). Moreover, many LAB do make and accumulate lactate, but do not consume it afterwards; rather, cells stop growing because of a low pH, even before the glucose is consumed.
In LAB, the tolerance to, and production of, lactic acid is an important phenotypic property which is also of industrial interest. For this reason, the isolation of LAB with increased lactate resistance and production levels has been a topic of research for decades (Rallu et al.2002). One strategy to improve the acid tolerance in Lactobacillus was by combining classical strain selection techniques with genome shuffling. To create some degree of diversity on the initial target strain, it was either cultured in a chemostat at low pH or the strain was chemical mutagenised and subsequently cultured on pH-gradient plates. Genome shuffling was carried out with the obtained isolates by multiple rounds of protoplast fusion. This resulted in strains that could grow at substantially lower pH and that showed up to 3-fold increase in lactate production (Patnaik et al.2002). Similar genome shuffling strategies with the aim to enhance lactic acid production were performed for Lb. rhamnosus (Yu et al.2008) and Lb. delbrueckii (John, Gangadharan and Madhavan Nampoothiri 2008). The adaptation of Leuconostoc mesenteroides in medium containing increasing concentrations of lactate over more than a year resulted in a roughly 2-fold increase in D lactic acid production (Ju, Kim and Lee 2016). The re-sequencing of three independently adapted strains shared a mutation in atpC a gene encoding a F0F1 ATPase ε subunit, which lead to a truncated protein. Overexpression in E. coli showed a 7.7-fold increase in cell viability for the truncated atpC in comparison to the wild-type gene. The authors hypothesise that the ATPase is involved in maintaining the intracellular pH at a higher level and thereby increasing viability.
Other examples of constraints of biotic origin would be the secretion of antibiotics, substrate depletion or the expression of extracellular substrate degrading/capturing enzymes/molecules. Several constraints of biotic origin are inherently linked to what is considered a public good to the bacterial cell and their utilisation leads to a dilemma during (experimental) evolution.
THE DILEMMA WITH PUBLIC GOODS
The evolution of microbial traits, which are at least in part determined by costs and benefits in relation to shared extracellular molecules, form a special case. A game theoretical approach assumes that the usage of common resources can be either fast or efficient. From the perspective of an individual, the fitness would be maximised if the resource is consumed quickly and reproduction is at the highest rate. In contrast, from the perspective of the population, the resource should be used efficiently and the total population size should be maximised. The resulting evolutionary dilemma has been termed ‘the tragedy of the commons’ (Hardin 1968; MacLean 2008). An example of microbes competing for a common resource is the expression of extracellular proteases by LAB. Dairy isolates of Lactococcus lactis have usually multiple amino acid auxotrophies and are adapted to utilise milk proteins. For this they express dedicated oligopeptide transporters and intracellular peptidases as well as an extracellular protease. The extracellular protease degrades milk proteins into peptides (Kunji et al.1996) that can be taken up and further utilised by the cell. For good growth in milk, the protease is essential but it was also shown that 5%–10% protease-positive strains in a mixed culture supply enough peptides to reach maximum growth rates (Bachmann et al.2011). However, it was observed already during the 1930s of the last century that upon repeated propagation pure cultures of lactococci gradually decrease their performance in milk (Harriman and Hammer 1931). This was later related to a loss of a plasmid encoded extracellular protease (McKay and Baldwin 1975) and it was shown that a protease carrying plasmid was completely lost from a culture after 300 generations of serial propagation in milk (Bachmann et al.2012). What occurs here is that proteolytic-negative strains do not have the burden of expressing the protease but they do have a benefit if peptides are being supplied by other strains in the culture. These so called ‘Cheaters’ invade the population of protease-positive ‘Cooperators’ and in the extreme case this leads to the extinction of the protease-positive strains (Bachmann et al.2011). The fact that the protease-positive strain is highly unstable during propagation in milk raises the question how such a trait can evolve in the first place and be maintained subsequently. The answer lies in environmental constraints. It was shown that the diffusion of peptides to neighbouring cells is limited at low cell densities, which gives more benefit to the protease-producing cell while the protease-negative cell does not get enough peptides to grow at the maximum rate. Under such conditions, the cost of protease expression is more than compensated by the peptides that become available from casein degradation and this strategy will be favoured by selection (Bachmann et al.2011). On the other hand, a high fraction of protease-positive cells in the culture will lead to higher overall peptide concentrations, which will favour the selection of the protease-negative variant. With lower frequencies of protease-positive strains, the benefit for protease-negative strains diminishes leading to frequency-dependent selection. While such frequency-dependent selection would argue that protease-positive strains should be maintained at least at a low frequency, in milk the complete extinction of protease-expressing strains was repeatedly observed. This is most likely due to the fact that protease-negative strains can grow on free amino acids and peptides in milk, although with a slower growth rate and lower final cell densities. The example of cheating mutants invading a pure population points out several relevant forces/concepts that are of importance during experimental evolution:
Biotic activity alters the environment and thereby alters selective forces.
The fitness advantage in the particular case of the protease-positive strains is dependent on the frequency of cheaters and cooperators in a culture.
The evolution and stabilisation of a cooperative trait is facilitated in a spatially structured environment.
Selection in a laboratory or industrial setting does not necessarily select for the strategy that would seem logical when adapting to a specific medium i.e. one would not expect that upon propagation in a specific medium the growth rate and yield would decrease.
Altered phenotypes can occur very rapidly (much quicker than one would expect based on standard mutation rates in case of plasmid loss).
The dilemma with public goods can also be extended to metabolic interdependencies between different species in complex microbial consortia. There is an increasing realisation that the evolution of such interdependencies is at least in part determined by the level of privatisation of metabolic interactions (Estrela, Morris and Kerr 2016). In complex dairy starter cultures, metabolic interactions between different members of the consortium are well described. They include the cross feeding of peptides, amino acids, vitamins, organic acids and nucleotide precursors in yoghurt (Sieuwerts et al.2008), kefir (Stadie et al.2013) or cheese (Piveteau, Condon and Cogan 1995). Currently, such interactions are best described for the yoghurt consortium which consists of two species. Lactobacillus delbrueckii subsp. bulgaricus is a protease-positive strain that supplies the protease-negative Streptococcus thermophilus with amino acids and peptides. In return, S. thermophilus produces CO2, pyruvate, formate, folate, long-chain fatty acids and ornithine. The exchange of those metabolites is described to be a mutually beneficial interaction, which promotes the growth of both species (Sieuwerts et al.2008). In a study addressing the evolution of microbial interactions, synthetic consortia of Escherichia coli strains were engineered for amino acid cross feeding. Interestingly, the authors found that a metabolic division of labour between the engineered strains lead to an overall increased growth rate (Pande et al.2014). This opens potentially interesting approaches for the experimental evolution of microbial consortia.
SELECTIVE PRESSURE AND METABOLIC SHIFTS
The shift between respiration and fermentation (or mixed acid and homolactic fermentation in LAB) has been explained with different theories. One approach is through game theory as described with the tragedy of the commons. Fermentation is then viewed as an inevitable, cheating strategy, consuming all the sugars as fast as possible at the cost of the overall cell yield. In contrast, the respiration strategy uses substrate efficiently (but at a slower rate) leading to a higher overall population density and biomass (Pfeiffer, Schuster and Bonhoeffer 2001). Theory predicts that introducing spatial structure should enable the evolution of cooperation (Nowak and May 1992; Sachs et al.2004). The underlying concept is that individual cells get the chance to grow without having to compete for the available substrate with other, possibly faster growing organisms. Such compartmentalisation that allowed the privatisation of the common substrates for individual cells was realised by culturing cells in water in oil emulsions droplets. The droplet contains the growth medium with individual cells and they are separated from their neighbouring cells by the oil phase in the emulsion. Serial propagation of Lactococcus lactis in this system allowed us to select for slow-growing, high-yield metabolic strategies (Bachmann et al.2013). From an experimental point of view, the results are very consistent with fast growth being correlated to overflow metabolism while slow growth, i.e. cell yield selection in emulsion, is correlated with efficient metabolism.
Apart from privatising public goods, there is one other theoretical possibility for respiration to co-exist with fermentation, even in well-mixed environments: if the fermentation product would inhibit the fermenter more than the respirer. This is not unlikely, as only the cells producing the ethanol or lactate will directly experience product inhibition. Then, at a critically high frequency of fermenters, the fermentation product would start to inhibit so much that respirers can grow equally fast (MacLean and Gudelj 2006; Wortel et al.2016).
Thus, the evolutionary basis for the shift between respiration and fermentation, or the shift between mixed acid and homolactic fermentation, can be explained by several different types of reasoning (see for a more detailed review, Goel et al.2012). We have discussed chemical warfare, and Make-Accumulate-Consume; we here added the Tragedy of the Commons. The problem with this game-theoretical explanation is that it does not give any mechanistic explanations, as it simply postulates a trade-off between growth rate and yield: it does not explain that trade-off, as the resource allocation model does. However, remember that the resource allocation explanation requires another trade-off: that efficient metabolism costs more protein, and this trade-off is also postulated. Still, we feel resource allocation is currently the most promising approach, as the connection to biochemistry and biophysics is more direct, and resource allocation is capable of explaining many more regulatory phenomena. Finally, its concepts can be readily implemented into the genome-scale metabolic modelling format, discussed in the future outlook.
APPROACHES TO EXPERIMENTAL EVOLUTION
The outcome of experimental evolution in the laboratory (Table 1) highly depends on the starting point, the biotic and abiotic constraints, and the duration of the experiment. Before starting such a study, it is wise to carefully consider what will one be selecting for. Perhaps in these types of biological experiments more than any other, standardisation is crucial to ensure reproducible outcomes enabling a thorough understanding of the process that is being studied.
Organism . | Types of protocol used . | Selective conditions(s) . | Main finding . | Reference . |
---|---|---|---|---|
Lactococcus lactis | Serial propagation | Starvation/growth, aerated/not shaken | IS element-mediated mutations are frequently occurring | de Visser et al. (2004) |
Lactococcus lactis | Serial propagation | Plant isolate adapted to growth in milk | Genome erosion; importance of nitrogen metabolism for growth in milk; one mutator strains identified | Bachmann et al. (2011) |
Lactobacillus rhamnosus | Serial propagation | Salt, bile and shear stress | Chromosomal rearrangements and mutator strains were identified | Douillard et al. (2016) |
Lactococcus lactis | Serial propagation | Fast growing variants of ldh deletion mutant were selected | IS981 activated the ldhB gene which lead to switch from mixed acid fermentation to homolactic fermentation | Bongers et al. (2003) |
Lactobacillus plantarum | Serial propagation | Surviving passage of the murine gastro intestinal tract | Repetitive rounds of exposure to mouse GIT resulted in higher GIT survival rates | van Bokhorst-van de Veen et al. (2013) |
Lactococcus lactis | Serial propagation (unintentional selection) | ldh deletion mutant showed unexpectedly homolactic metabolism; ldh-positive variant was probably selected unintentionally during propagation after strain construction (see also Bongers et al.2003) | IS905 activated ldhB gene and lead to switch from mixed acid fermentation to homolactic fermentation | Gaspar et al. (2007) |
Lactococcus lactis | Dominant selection | Growth of MG1363 (lac- strain) on lactose | Upregulation of cellobiose PTS allows growth on lactose | Solopova et al. (2012) |
Lactococcus lactis | Dominant selection | Growth on medium containing H2O2 | Isolation of SpOx mutants (spontaneous oxidative stress resistant) | Rochat et al. (2005) |
Lactococcus lactis | Dominant selection | Growth at elevated temperature | Mutation in c-di-AMP specific phosphodiesterase leads to heat resistance and salt hypersensitivity | Smith et al. (2012) |
Lactobacillus helveticus | Dominant selection | Growth at elevated temperature | Increased growth rate at elevated temperature lead to increased autolysis under cheese-like conditions | Spus et al. (2017) |
Lactococcus lactis | Emulsion propagation | Selection for a high number of offspring | Selection for cell yield resulted in slow growing cells with high biomass yield and mixed acid fermentation | Bachmann et al. (2013) |
Lactococcus lactis | Chemostat | Carbon limitation at various dilution rates | Mutations in ccpA, a global regulator of carbon metabolism, were found across different conditions | Price et al. (2010, unpublished) |
Organism . | Types of protocol used . | Selective conditions(s) . | Main finding . | Reference . |
---|---|---|---|---|
Lactococcus lactis | Serial propagation | Starvation/growth, aerated/not shaken | IS element-mediated mutations are frequently occurring | de Visser et al. (2004) |
Lactococcus lactis | Serial propagation | Plant isolate adapted to growth in milk | Genome erosion; importance of nitrogen metabolism for growth in milk; one mutator strains identified | Bachmann et al. (2011) |
Lactobacillus rhamnosus | Serial propagation | Salt, bile and shear stress | Chromosomal rearrangements and mutator strains were identified | Douillard et al. (2016) |
Lactococcus lactis | Serial propagation | Fast growing variants of ldh deletion mutant were selected | IS981 activated the ldhB gene which lead to switch from mixed acid fermentation to homolactic fermentation | Bongers et al. (2003) |
Lactobacillus plantarum | Serial propagation | Surviving passage of the murine gastro intestinal tract | Repetitive rounds of exposure to mouse GIT resulted in higher GIT survival rates | van Bokhorst-van de Veen et al. (2013) |
Lactococcus lactis | Serial propagation (unintentional selection) | ldh deletion mutant showed unexpectedly homolactic metabolism; ldh-positive variant was probably selected unintentionally during propagation after strain construction (see also Bongers et al.2003) | IS905 activated ldhB gene and lead to switch from mixed acid fermentation to homolactic fermentation | Gaspar et al. (2007) |
Lactococcus lactis | Dominant selection | Growth of MG1363 (lac- strain) on lactose | Upregulation of cellobiose PTS allows growth on lactose | Solopova et al. (2012) |
Lactococcus lactis | Dominant selection | Growth on medium containing H2O2 | Isolation of SpOx mutants (spontaneous oxidative stress resistant) | Rochat et al. (2005) |
Lactococcus lactis | Dominant selection | Growth at elevated temperature | Mutation in c-di-AMP specific phosphodiesterase leads to heat resistance and salt hypersensitivity | Smith et al. (2012) |
Lactobacillus helveticus | Dominant selection | Growth at elevated temperature | Increased growth rate at elevated temperature lead to increased autolysis under cheese-like conditions | Spus et al. (2017) |
Lactococcus lactis | Emulsion propagation | Selection for a high number of offspring | Selection for cell yield resulted in slow growing cells with high biomass yield and mixed acid fermentation | Bachmann et al. (2013) |
Lactococcus lactis | Chemostat | Carbon limitation at various dilution rates | Mutations in ccpA, a global regulator of carbon metabolism, were found across different conditions | Price et al. (2010, unpublished) |
Organism . | Types of protocol used . | Selective conditions(s) . | Main finding . | Reference . |
---|---|---|---|---|
Lactococcus lactis | Serial propagation | Starvation/growth, aerated/not shaken | IS element-mediated mutations are frequently occurring | de Visser et al. (2004) |
Lactococcus lactis | Serial propagation | Plant isolate adapted to growth in milk | Genome erosion; importance of nitrogen metabolism for growth in milk; one mutator strains identified | Bachmann et al. (2011) |
Lactobacillus rhamnosus | Serial propagation | Salt, bile and shear stress | Chromosomal rearrangements and mutator strains were identified | Douillard et al. (2016) |
Lactococcus lactis | Serial propagation | Fast growing variants of ldh deletion mutant were selected | IS981 activated the ldhB gene which lead to switch from mixed acid fermentation to homolactic fermentation | Bongers et al. (2003) |
Lactobacillus plantarum | Serial propagation | Surviving passage of the murine gastro intestinal tract | Repetitive rounds of exposure to mouse GIT resulted in higher GIT survival rates | van Bokhorst-van de Veen et al. (2013) |
Lactococcus lactis | Serial propagation (unintentional selection) | ldh deletion mutant showed unexpectedly homolactic metabolism; ldh-positive variant was probably selected unintentionally during propagation after strain construction (see also Bongers et al.2003) | IS905 activated ldhB gene and lead to switch from mixed acid fermentation to homolactic fermentation | Gaspar et al. (2007) |
Lactococcus lactis | Dominant selection | Growth of MG1363 (lac- strain) on lactose | Upregulation of cellobiose PTS allows growth on lactose | Solopova et al. (2012) |
Lactococcus lactis | Dominant selection | Growth on medium containing H2O2 | Isolation of SpOx mutants (spontaneous oxidative stress resistant) | Rochat et al. (2005) |
Lactococcus lactis | Dominant selection | Growth at elevated temperature | Mutation in c-di-AMP specific phosphodiesterase leads to heat resistance and salt hypersensitivity | Smith et al. (2012) |
Lactobacillus helveticus | Dominant selection | Growth at elevated temperature | Increased growth rate at elevated temperature lead to increased autolysis under cheese-like conditions | Spus et al. (2017) |
Lactococcus lactis | Emulsion propagation | Selection for a high number of offspring | Selection for cell yield resulted in slow growing cells with high biomass yield and mixed acid fermentation | Bachmann et al. (2013) |
Lactococcus lactis | Chemostat | Carbon limitation at various dilution rates | Mutations in ccpA, a global regulator of carbon metabolism, were found across different conditions | Price et al. (2010, unpublished) |
Organism . | Types of protocol used . | Selective conditions(s) . | Main finding . | Reference . |
---|---|---|---|---|
Lactococcus lactis | Serial propagation | Starvation/growth, aerated/not shaken | IS element-mediated mutations are frequently occurring | de Visser et al. (2004) |
Lactococcus lactis | Serial propagation | Plant isolate adapted to growth in milk | Genome erosion; importance of nitrogen metabolism for growth in milk; one mutator strains identified | Bachmann et al. (2011) |
Lactobacillus rhamnosus | Serial propagation | Salt, bile and shear stress | Chromosomal rearrangements and mutator strains were identified | Douillard et al. (2016) |
Lactococcus lactis | Serial propagation | Fast growing variants of ldh deletion mutant were selected | IS981 activated the ldhB gene which lead to switch from mixed acid fermentation to homolactic fermentation | Bongers et al. (2003) |
Lactobacillus plantarum | Serial propagation | Surviving passage of the murine gastro intestinal tract | Repetitive rounds of exposure to mouse GIT resulted in higher GIT survival rates | van Bokhorst-van de Veen et al. (2013) |
Lactococcus lactis | Serial propagation (unintentional selection) | ldh deletion mutant showed unexpectedly homolactic metabolism; ldh-positive variant was probably selected unintentionally during propagation after strain construction (see also Bongers et al.2003) | IS905 activated ldhB gene and lead to switch from mixed acid fermentation to homolactic fermentation | Gaspar et al. (2007) |
Lactococcus lactis | Dominant selection | Growth of MG1363 (lac- strain) on lactose | Upregulation of cellobiose PTS allows growth on lactose | Solopova et al. (2012) |
Lactococcus lactis | Dominant selection | Growth on medium containing H2O2 | Isolation of SpOx mutants (spontaneous oxidative stress resistant) | Rochat et al. (2005) |
Lactococcus lactis | Dominant selection | Growth at elevated temperature | Mutation in c-di-AMP specific phosphodiesterase leads to heat resistance and salt hypersensitivity | Smith et al. (2012) |
Lactobacillus helveticus | Dominant selection | Growth at elevated temperature | Increased growth rate at elevated temperature lead to increased autolysis under cheese-like conditions | Spus et al. (2017) |
Lactococcus lactis | Emulsion propagation | Selection for a high number of offspring | Selection for cell yield resulted in slow growing cells with high biomass yield and mixed acid fermentation | Bachmann et al. (2013) |
Lactococcus lactis | Chemostat | Carbon limitation at various dilution rates | Mutations in ccpA, a global regulator of carbon metabolism, were found across different conditions | Price et al. (2010, unpublished) |
Strain stocks are composed of a very large number of individuals that are genetically very similar but not necessarily identical. During the revival of cryopreserved stocks one can end up with diverse pre-cultures if steps are not taken to prevent this. Protocols should be implemented to prevent this and to ensure that the starting point of multiple parallel laboratory (evolution) experiments is as much as possible the same (Ryall, Eydallin and Ferenci 2012). Here, most academic labs could improve a lot by learning from the experience of industrial companies that develop highly standardised starter cultures, namely of LAB. Generally, this involves a complete documentation of cultivation history and resorting to aliquoting of large cultures into single-use cryopreserved stocks that are not exposed to freeze–thaw cycles; this approach was taken by Goel et al. (2015). The growth stage of the pre-culture and inoculation density should also be standardised and closely monitored. A special case relating to the standardisation of experimental procedures is the unintended adaptation of strains when they are transferred from original isolation source into a laboratory environment. This ‘domestication’ is poorly investigated for most species. However, for Escherichia coli it was shown that within 2–3 days of laboratory culturing of wild isolates phenotypic changes in metabolism, colony morphology and fitness started to appear. While some parallelisms were found for repeated adaptations, the changes were not uniform (Eydallin et al.2014). One can expect that due to big selective advantages the adaptations after the initial transition to the laboratory are quickly selected for. However, adaptations are likely to keep accumulating. The Lactococcus lactis models strain MG1363 is broadly used and recently its plasmid carrying ancestor NCDO712 was fully sequenced. A comparison showed that since the diversion of MG1363 from NCDO712 in 1983, 11 SNPs got fixed in either strain. Out of the 11 mutations, 5 coded for protein changes and 1 was in a predicted promoter region (Tarazanova et al.2016). It is unclear if the identified mutations are caused by genetic drift or if they have functional consequences, but it is important to realise evolution is ongoing also during the limited number of transfers that occurred for these strains over the years in different laboratories.
For prolonged cultivation, the mentioned interplay between biotic and abiotic constraints should be taken into account during experimental design. If for instance the objective of the study would involve ruling out abiotic constraints, such as external pH, from the evolutionary process, then the design of the experiment must include pH control. Unfortunately, not all interactions between life and its surroundings are as well understood and easy to control as the mechanistic link between growth and acidification in LAB. This sometimes makes experimental design a process more iterative than one would wish for.
Serial propagation
The most commonly used cultivation mode in experimental evolution is serial propagation, and naturally, the longest laboratory evolution experiments have been carried out in this fashion (Tenaillon et al.2016). This is most likely related to convenience and technical ease rather than any other specific reason. In fact, serial propagations may present several drawbacks, even if experiments are carefully designed. For example, it is very difficult to maintain one and the same selection pressure throughout each batch of cultivation and even more so throughout the course of the laboratory evolution experiment itself. Typically, in these cultivations cells are thought to be selected on the basis of their growth rate, but this is a rather simplistic view. In the batch cultivation, the levels of nutrients and fermentation products in the case of LAB will be varying at a rate that is linked to growth. As the latter evolves, the first will become faster. This may result that cells are exposed for longer to growth-inhibiting pH values, or nutrient-starved conditions. Either way, the selected phenotype will unlikely be the one that simply grows fastest. Rather, a combination of traits will most likely be selected, and the extent to which each one contributes to the overall fitness gain is hard to fully understand, and thus far, impossible to predict. Nevertheless, the LAB field is ripe with examples of very interesting laboratory evolution experiments carried out in serial batch, from which we have been able to get quite some insight on how these organisms may have evolved across ecological niches (Bachmann et al.2012). There are several examples of serial propagations of LAB of which some were already mentioned above.
Lactococcus lactis was adapted for 1000 concentrations under starvation and growing conditions as well as shaken and not aerated. As expected the fitness of the evolved strains increased when compared to the ancestors and the detailed analysis pointed towards a big role of IS elements in the adaptation of the strains (de Visser et al.2004). IS elements also played an important role in recovery of fast growing homolactic phenotypes of L. lactis strains defective in their lactate dehydrogenase (ldh). Once study found repeatedly the appearance of ldh-positive strains upon serial propagation of the strains while in a second study ldh-positive variants took over the population immediately after strain construction. In both cases, IS elements were responsible for the activation of an alternative ldhB gene in the strain (Bongers et al.2003; Gaspar et al.2007). Interestingly, in a strain with deletions in ldh and ldhB the serial propagation for up to 280 generations did not result in an increased growth rate and/or a reversion towards higher lactate production, pointing towards the constraint on growth rate imposed by mixed acid production (Bachmann et al.2013).
In another study, the L. lactis plant isolate KF147 was serially propagated in milk in an attempt to mimic the transition from the plant to the dairy environment, which mainly based on comparative genomics analysis, is suggested to have occurred in nature (Siezen et al.2005; van Hylckama Vlieg et al.2006). After 1000 generations of growth in milk, single colony isolates from three independently evolved cultures showed increased fitness, growth rates and biomass yields in milk. Genome re-sequencing revealed the loss of a 51-kb transposon in two out of three cultures. The lost DNA fragment coded mainly for carbon utilisation genes of carbohydrates typically occurring in plant material but not in milk. This is consistent with the idea of genome erosion when adapting to rich environments. On the other hand, mutations related to amino-acid metabolism were identified. Seen the amino acid auxotrophies in L. lactis and the fact that the adapted strains could not degrade milk proteins through an extracellular protease, the optimisation of peptide uptake and amino acid metabolism was consistent with expected changes. Interestingly, in two out of three adapted isolates, two identical point mutations in a peptide transport system were identified. One of the mutations was in the promoter region of the operon encoding for the peptide transporter which coincided with an upregulation of this operon. The second mutation was the deletion of an adenine residue in a poly-A stretch of a pseudogene in the same operon. This deletion of a single nucleotide restored the original reading frame of the gene and thereby restored a functional gene. This example demonstrates that a limited number of mutations that lead to changes in nitrogen metabolism can have profound effects of the fitness of lactococci in milk (Bachmann et al.2012).
The serial propagation of Lactobacillus rhamnosus throughout 1000 generations under four different conditions and the subsequent re-sequencing of 10 isolates from each of the four conditions revealed numerous single nucleotide polymorphisms, genome rearrangements and also found mutator phenotypes.
A variation of serial propagation that is typically done in vitro is the repeated transfer of Lb. plantarum that survived the passage through the murine intestinal tract. Three repeated transfers resulted in the identification of strains with significantly increased persistence in the murine intestinal tract, and re-sequencing of isolated strains revealed mutations in genes encoding cell envelope and energy metabolism associated functions (van Bokhorst-van de Veen et al.2013).
If the selective pressure during cell propagation is very high, mutants can be identified in a single selection round which was termed ‘dominant selection’ by Derkx et al. (2014). This approach has been successfully applied with LAB (Table 1). A recent study related to plate selection was based on the ability of cells to colonise a large solid matrix across an increasing gradient of a growth inhibitor e.g. antibiotic (Baym et al.2016). We anticipate that similar approaches may be an effective way to select for strains with improved industrial traits.
Continuous cultivation
Laboratory evolution experiments carried out under continuous cultivation, though considerably more laborious, do overcome limitations highlighted above found for serial batch propagations. The chemostat is the most used continuous cultivation setup. It allows the cultivation of a microbial population under a specific nutrient limitation and at a specific growth rate defined by the experimentalist, theoretically for an infinite number of generations (Novick and Szilard 1950). It has been repeatedly used in evolution experiments with popular model organisms such as E. coli (Weikert, Sauer and Bailey 1997; Wang et al.2010) and Saccharomyces cerevisiae (Adams et al.1985; Mashego et al.2005), for instance to investigate how organisms adapt to specific nutrient limitations. Reports within the LAB field for these sort of experiments are nearly non-existent to the best of our knowledge. However, prolonged parallel chemostat cultivations at different dilution rates have been carried out for L. lactis under glucose-limited conditions for several hundred generations (Price, C. et al. unpublished). Cells from the evolved populations have been characterised phenotypically (Price et al.2010) and their genome sequence has been deduced. Remarkably, a single amino-acid mutation in the global regulator CcpA was found to be selected across all prolonged cultivations irrespective of the set dilution rate. This mutation rendered the evolved cells fitter in a constant glucose-limited environment clearly at a cost of reduced performance in dynamic environments such as simple batch cultivation, where the parent strain was found to be much fitter. These preliminary findings corroborate previous suggestions that LAB have fine-tuned their metabolism and regulatory networks such that they would behave optimally in conditions where the concentration of C-source may vary drastically (Goel et al.2015).
Besides the chemostat, there are several other continuous cultivation methods described to be useful in systems microbiology (Adamberg, Valgepea and Vilu 2015). Within the context of experimental evolution, the turbidostat would be particularly relevant, since there the biomass concentration is kept constant by diluting the growing population with fresh medium at a rate identical to which the population is growing (Bryson and Szybalski 1952). This, contrary to what is the case during serial batch propagations, enables populations to be evolved at maximal growth rate without fluctuations in any other external environmental factor (e.g. pH, fermentation products, etc.). However, prolonged turbidostat cultivation presents some serious technical hurdles that compromise its applicability within this context. For instance, the online measurement of biomass concentration, typically using an OD probe, becomes very rapidly unreliable throughout the course of the evolution experiment. This is due to both calibration issues, but more dramatically when using LAB, simply to clogging. The metabolism of LAB, more specifically the fact that the acidification rate is most often directly associated with growth rate, does present opportunities to perform prolonged cultivation in a continuous regime, while overcoming the technical hurdles just listed. Online pH monitoring is much easier to achieve and much more robust to cultivation time if properly set up. So by monitoring directly pH, rather than biomass concentration, and continuously diluting the culture with fresh medium such that the natural acidification rate is neutralised, cells can be kept growing at maximal acidification rate (a proxy for maximal growth rate in LAB) indefinitely. Such a setup is known as a pH auxostat and has been used within our groups to adapt L. lactis MG1363 to the pentose sugar ribose, on which it would only be able to grow very slowly at first. Quite successfully, the original growth rate was increased by nearly 5-fold, while selecting directly for increased acidification rate. Re-sequencing of the evolved stocks revealed the genetic underpinning to be a point mutation in one of the subunits of ribose ABC-type transport system present in L. lactis (Branco dos Santos F. et al. unpublished data).
In a direct comparison between serial batch and continuous cultures, the serial batch has the advantages of being less laborious, easier to maintain and less equipment/media is needed, while continues cultures allow the creation of a much better controlled and constant (at the short time scale) environment. For experimental evolution, it is important to realise what phenotypes can be selected for with these different approaches and what the practical consequences are to make an informed decision on the method of choice.
Propagation in spatially structured environments
Competition for resources that are made freely available to all individuals of the population will have a considerable effect on the behaviour of cells (see sections ‘The role of constraints’ and ‘The dilemma with public goods’). Thus far, the vast majority of the laboratory evolution experiments performed did not spatially restrict the individual members of the evolving population, although examples do exist. For instance, the selection of increased cellulase expression in Aspergillus niger was achieved by repetitive culturing on agar plates with cellulose as a carbon source (Patyshakuliyeva et al.2016). Recent technological advances that, compared to agar plates, allow a more stringent compartmentalisation of millions of individual cells as they are based on serial propagation in water in oil emulsions (Bachmann et al.2013). The compartmentalisation of individual cells in the droplets of an emulsion prevents substrate competition (the substrate is privatised). Cells that produce more offspring per droplet (be it accompanied by slower growth or not) will increase in frequency and upon breaking and re-inoculating a new emulsion more droplets will be seeded with the mutant cells. Serial propagation in this regime will enrich for the cells with the highest number of offspring. This protocol was applied to L. lactis MG1363 and it lead to the selection of cells that showed mixed acid fermentation instead of homolactic metabolism (Bachmann et al.2013). Mixed acid metabolism results in three ATP molecules per consumed glucose and it is therefore more efficient than homolactic metabolism which produces only two ATP molecules per glucose molecule. While the selected mutant showed a higher cell and biomass yield, it grew slower than the ancestral cells confirming the yield rate trade-off described above. Interestingly, the slow growing mutant strain with a high cell yield reverted within 90 generations of serial batch propagation to a fast growing homolactic phenotype. Genome re-sequencing showed that the phenotype with a high cell yield had a compromised glucose uptake system while in the revertants the same compromised glucose uptake system was upregulated through a mutational event. The isolation of more efficient cells and their reversion through selection in well-designed environments is consistent with our theoretical understanding on spatial structure during selection and the resource allocation concepts described. It would be interesting to see if the continuation of the selection in a spatially structured environment could increase metabolic efficiency even further e.g. through alteration of maintenance requirements. As the described protocol selects for cell yield, another potential outcome of such prolonged selection would be a smaller cell size which would be interesting if one wants to study constraints relating to volume/surface ratios of cells.
Furthermore this experimental approach should also allow to study and possibly select for microbial interactions, which would be highly relevant to study complex microbial consortia.
CONCLUSIONS
Experimental evolution is increasingly being used for the study of fundamental biological principles but also for the improvement of industrially interesting microorganisms. While improved DNA sequencing technologies allow the fast analysis of adapted strains, the increased interest for organisms used in food fermentation is also driven by the fact that experimentally evolved strains are not genetically modified and can be readily used in industrial applications.
Evolutionary biologists have embraced bacteria as model organisms for it allows them to work with big populations that have short generation times and that can be easily cultured. They developed lots of theory on population dynamics and the fixation of mutations and how this is influenced by parameters such as mutation rates, epistasis, the selective pressure imposed and others. This knowledge is highly relevant and should be embraced by people working on industrial strain improvement.
Recent developments in the area of cellular economy are also highly relevant to experimental evolution approaches. The different strategies available to a cell are competing for finite resources to optimise fitness in a given environment. Such strategies can be of metabolic nature i.e. respiration vs fermentation or mixed acid fermentation vs homolactic metabolism or metabolic and growth-related proteins can compete with stress resistance proteins. Whatever the competition, it is the fitness of an organism that determines which strategy wins. The number of examples for trade-offs that occur as a consequence of finite resources and the related allocation dilemma is still limited. Well-known described examples are a yield/rate trade-off for microbial growth (MacLean 2008; Bachmann et al.2013) and relationships between slow growth rates and improved stress resistance (Bittner, Kriel and Wang 2014). However, there is a reason to believe that trade-offs are of importance to many other phenotypes that are selected for during experimental evolution. Especially at high growth rates, when the protein synthesis machinery takes up much of the cellular resources, trade-offs with other proteins are expected. This is nicely demonstrated in a study with Escherichia coli. Cells originating from carbon limited chemostats that were run at a low dilution rate were found to be able to utilise much more different substrates compared to the cells originating from conditions with a high dilution rate (Ihssen and Egli 2005).
Moreover, the resource allocation perspective can be implemented into the genome-scale metabolic modelling format, bringing such models to the next level of predictions (King et al.2015). The basic idea is that for each metabolic flux in the metabolic network, the presence of an enzyme is implied, which requires the synthesis of proteins. The latter can be fully mapped out as metabolic reactions, drawing amino acids and ATP from the metabolic network. Thus, metabolism and expression of proteins are fully coupled in such ‘ME’ models: these models can shift metabolic strategies as environmental constraints change, see O’Brien et al. (2014) for an elaborate example in E. coli, and Nilsson and Nielsen (2016) for a more coarse-grained example in yeast.
As many industrial applications and experimental evolution approaches are aimed at selecting for fast growing strains, the understanding of underlying trade-offs seems highly relevant. Their understanding in combination with a good comprehension of the selective forces in the different culture systems described should allow to create more directed selective conditions for the selection of new phenotypes.
While these developments should certainly help to select for novel strains, there are still numerous phenotypes that would be of industrial interest but cannot be selected for in any of the culture systems described above. For instance, it is currently not possible to select for a phenotypic property that cannot be linked to growth, be it growth rate or cell yield. Such properties could be the production of specific enzymes or metabolites such as flavour volatiles that are of industrial interest. For some phenotypes, enzyme or metabolite measurements on individual cells/colonies followed by selection forms a low throughput alternative while flow cytometry might be a high throughput solution. The latter is typically limited by the availability of fluorescent probes that are needed as reporters for a specific phenotype. Microfluidic and droplet-based systems in combination with biosensors might form an interesting alternative for such selection campaigns in the future (Meyer et al.2015).
While lots of progress on genome dynamics and cellular resource allocation was made in recent years, there are still many open questions on how different constraints influence trade-offs and eventually the fitness of an organism in a particular environment. The understanding of underlying biological concepts should allow to improve fermentation processes and design experimental evolution protocols for the selection of new phenotypes.
FUNDING
BT and DM acknowledge funding from the Innovative Training Network (ITN) MicroWine. BT and HB acknowledge funding from the Technologiestichting STW project 13858.
Conflict of interest: None declared.