-
PDF
- Split View
-
Views
-
Cite
Cite
Yang Hu, Jian Cai, Ying Gong, Changqing Liu, Xingyu Jiang, Xiangming Tang, Keqiang Shao, Guang Gao, The collapse and re-establishment of stability regulate the gradual transition of bacterial communities from macrophytes- to phytoplankton-dominated types in a large eutrophic lake, FEMS Microbiology Ecology, Volume 99, Issue 10, October 2023, fiad074, https://doi.org/10.1093/femsec/fiad074
- Share Icon Share
Abstract
Eutrophic lakes often exhibit two alternative types: macrophytes-dominated (MD) and phytoplankton-dominated (PD). However, the nature of bacterial community types that whether the transition from the MD to the PD types occurs in a gradual or abrupt manner remains hotly debated. Further, the theoretical recognition that stability regulates the transition of bacterial community types remains qualitative. To address these issues, we divided the transition of bacterial communities along a trophic gradient into 12 successional stages, ranging from the MD to the PD types. Results showed that 12 states were clustered into three distinct regimes: MD type, intermediate transitional type and PD type. Bacterial communities were not different between consecutive stages, suggesting that the transition of alternative types occurs in a continuous gradient. At the same time, the stability of bacterial communities was significantly lower in the intermediate type than in the MD or PD types, highlighting that the collapse and re-establishment of community stability regulate the transition. Further, our results showed that the high complexity of taxon interactions and strong stochastic processes disrupt the stability. Ultimately, this study enables deeper insights into understanding the alternative types of microbial communities in the view of community stability.
Introduction
The bacterial communities from different environments fall into distinct robust clusters, which are interpreted as distinct community types (Thompson et al. 2017, Yu et al. 2021). Examples include the aquatic bacterial communities found in large eutrophic lakes, which frequently exist in contrasting states that are characterized either by macrophytes-dominated (MD) or phytoplankton-dominated (PD) states, in terms of diversity, composition and metabolic functions (Scheffer and van Nes 2007, Wang et al. 2020, Cao et al. 2021). However, the actual existence of these distinct bacterial community types remains hotly debated. For instance, in a meta-analysis, Arumugam et al. (2011) argued that distinct bacterial community types could be an artifact of small sample sizes, so that what one should expect is that the bacterial community types exist as continuous gradients, rather than as distinct clusters (Gibson et al. 2016). Although the bacterial communities have been frequently investigated in terms of composition, diversity and metabolic functions (Faust et al. 2015, Ronda and Wang 2022), we still do not yet grasp how bacterial communities transit from one type to another, leaving us with a limited understanding of bacterial community types.
The presence of bacterial community types is frequently explained by several possible mechanisms. The most frequently evoked one is the species sorting, which is referred to as the continuous response of bacterial communities to environmental gradients. Under this mechanism, many transitional community configurations exist so that community types are essentially a continuous gradient. The second mechanism is known as the multi-stability of bacterial communities (Gonze et al. 2017). Under this mechanism, when an environmental condition crosses the threshold, the bacterial community switches abruptly from one stable state to another. As the critical slowing down theory predicts, a biological system's stability declines in the vicinity of the tipping point, so that a small disturbance can push the biological system to an alternative state (Clements and Ozgul 2018). Despite their differences, both mechanisms fall under the conceptual framework of community stability. This idea posits that the structure of bacterial communities varies across states from one of stability to any degree of instability (Pettersson et al. 2020). Conceptually, bacterial communities can be described as a ‘stability landscape’. They occupy a landscape with an equilibrium state, and move away from its normal equilibrium state as the community stability decreases (Shaw et al. 2019, Philippot et al. 2021). When a community nears instability, a small perturbation is enough to push the community to an alternative type (Fukami and Nakajima 2011, Gibson et al. 2016), after which community stability returns under the new structure. However, due to the difficulty of quantifying community stability, the concept of the ‘stability landscape’ is qualitative. Recently, however, it was suggested that a stable community can limit the magnitude of population fluctuations, so that the community stays within a given type (Roberts et al. 2019). Thus, the degree of population fluctuations might serve as an index of community stability (Zelikova et al. 2014, Berga et al. 2017).
Behind the concept of ‘stability landscape’ lies the question of what disrupts and re-establishes stability. More than half a century ago, it was thought that less diverse communities were more prone to suffer oscillations in populations (Macarthur 1955). The corollary was that diversity promotes community stability (Lucini et al. 2020). However, this perspective was challenged by May, who argued that increasing diversity might destabilize the community (May 1972). The crux of this paradox lies within the fact that studies of diversity alone leave an important aspect of community structure unexamined: species interactions. In nature, bacteria tend to exist within complex networks, which are connected by antagonistic and mutualistic interactions (Faust and Raes 2012). Recent evidence has demonstrated that the balance of species interactions and their topological structures largely determine the stability of bacterial communities (Qian and Akcay 2019, Sanchez-Palencia and Francoise 2020, Barber et al. 2022). Further, the complex bacterial community normally comprises a few core taxa and large numbers of satellite taxa. In particular, the core sub-community has been demonstrated to have more complicated species interactions (Yan et al. 2021), and is expected to have a closer relationship with community stability. Another associated and intriguing question is whether community assembly processes closely relate to stability, given the crucial role of stochastic and deterministic processes in shaping the community types (Stegen et al. 2013). For example, stochastic processes can direct a microbial community of single source to distinct types by chance, even under identical environmental conditions (Zhou et al. 2013). Likewise, deterministic processes can also to some extent guide the microbial community to a different type, such as seen in fecal microbiota transplantation therapy (Gibson et al. 2016). However, from the viewpoint of stability, we still lack direct connections between species interactions, community assembly processes and the emergence of alternative community types.
As a typical large shallow lake, Lake Taihu, in eastern China, is known to exist in two alternative regimes: MD and PD states. As a consequence, the bacterial communities in Lake Taihu correspondingly exist as either MD or PD types. Up to now, most studies compared only static snapshots of bacterial communities between the MD and PD types (Wang et al. 2020, Cao et al. 2021), leaving the sequential dynamics of bacterial communities from MD to PD type unexplored. To better understand the nature of alternative community types, we spatially divided Lake Taihu into 12 subdivisions that spanned a gradient of trophic states using a fine-grained spatial sampling approach. These subdivisions serve as analogues of stages in the ecological succession of bacterial communities from MD to PD types. Ultimately, the objectives of this study were to investigate how different lake regimes differentiate bacterial communities and affect community stability and assembly. Accordingly, we hypothesized that: (1) bacterial communities showed a transition from the MD type to the PD type driven by the increasing eutrophication; and (2) community stability mediated by taxon interactions and community assembly processes largely contributes to this transition. Testing these hypotheses helps illuminate the nature of bacterial community types, and helps elucidate the dynamics of bacterial community under environmental disturbances.
Materials and Methods
Study area and sampling strategy
Lake Taihu (30°55′40″-31°32′58″N, 119°52′32″-120°36′10″E) is a typical shallow freshwater lake in China, and is located in the subtropical monsoon climate area. The seasonal distribution of precipitation is uneven, and the rainfall in the flood season accounts for about 60% of the total volume (Zhang et al. 2017). The water surface of Lake Taihu is approximately 2338 km2. Its west and southwest sides are hilly and mountainous, while the east side is dominated by plains and water networks. The average water depth of Taihu Lake is 1.9 m. Lake Taihu is situated in a typical river network area with about 219 rivers. Most of them are influent-effluent currents, leading to the flow moving alternately backwards and forwards. However, these rivers around the lake have a relatively small impact on the overall lake flow. The lake movement is strongly affected by the wind flow, which has obvious interannual variations. Specifically, the southeast wind prevails in summer, while the northwest wind prevails in winter (Hu et al. 2020).
Over the past 60 years, rapid agricultural and industrial growth has produced a strong gradient in the trophic state of the lake, which increases from southeast to northwest. This trophic gradient leads to contrasting lake regimes with clearer water dominated by macrophytes in the southeast region and more turbid water dominated by phytoplankton in the northwest (Janssen et al. 2014). Sampling locations were described in our previous study (Xie et al. 2020). In summary, 120 sampling sites were located in a systematic grid across Lake Taihu, from which samples were collected in September 2013. Sampling methods are described in detail in Xie et al. (2020). To imitate the transition of bacterial communities from MD to PD types, a method of space-for-time substitution was adopted in this study, which subdivides the lake into subzones with a sliding window approach. The rationale behind this method lies in the low eutrophication in the whole lake prior to the 1950s (Li et al. 2008), while increasing human activity promotes the heterogeneity, leading to water quality decreasing from northwest to southeast (Wang et al. 2022). As a consequence, environmental filtering mainly regulates the bacterial community, but not initial conditions and priority effects. Briefly, this method employed overlapping rectangles (Fig. 1, angle 45°, 80 km x 8 km; long axis running southwest to northeast) placed consecutively by an interval of 5 km. Ultimately, this procedure created 12 subzones, which were analogous to 12 stages (St1-St12) of ecological succession of the bacterial community from MD to PD types.
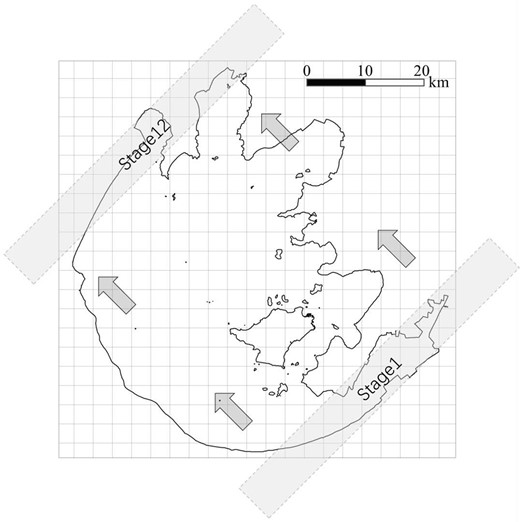
Surface water (top 50 cm) was collected with a 5-L Schindler sampler. Water was pre-filtered with 5-μm pore-sized filters to remove large plankton and other particles, after which bacterioplankton were captured on 0.22-μm pore-sized polycarbonate filters. Water temperature, pH and dissolved oxygen (DO) were measured in situ by a multiparameter water quality sonde (YSI 6600 V2, Yellow Springs Instruments Inc., USA). Measurements of water transparency, total suspended solids (TSS), total nitrogen (TN), total dissolved nitrogen, total phosphorus (TP), total dissolved phosphorus, chlorophyll a (Chl a) and dissolved organic carbon (DOC) were as described previously (Xie et al. 2020).
DNA extraction, sequencing and data processing
Crude DNA was extracted from 111 samples using PowerSoil DNA Isolation Kit (Mo Bio Laboratories, Inc., Carlsbad, CA, USA) according to the manufacturer's protocol. DNA quality was measured with a NanoDrop ND-2000 spectrophotometer (NanoDrop, ND-2000, Thermo Scientific, Wilmington, DE, USA). The V4 hypervariable region of the 16S rRNA gene was amplified using the primers 515F (5′-GTGYCAGCMGCCGCGGTAA-3′) and 806R (3′-GGACTACNVGGGTWTCTAAT-5′). Polymerase chain reaction (PCR) amplification was performed in a 50-μL reaction mixture, containing 5 μL of 10 × PCR buffer, 4 μL of MgCl2 (25 mmol L−1), 0.5 μL of each primer (10 μmol L−1 each), 30 ng of quantified template DNA measured by PicoGreen dsDNA Assay Kit (Invitrogen, Carlsbad, CA, USA) and 0.4 μL of Taq polymerase (5 U μL−1; Fermentas, USA). PCR cycling was conducted in a thermocycler (Applied Biosystems Veriti Thermal Cycler) by a touchdown program: denaturation at 94°C for 5 min, 11 cycles of denaturation at 94°C for 1 min, annealing at 65°C for 1 min (the temperature was decreased by 1°C every cycle until 55°C was reached) and extension at 72°C for 1 min. Nineteen additional cycles were performed at an annealing temperature of 55°C, followed by a final extension at 72°C for 10 min. To reduce PCR-induced biases, PCR products were purified with Agencourt AMPure XP SPRI magnetic beads (Beckman Coulter, Tokyo, Japan). The DNA quantity was measured with a NanoDrop 2000 spectrophotometer (Thermo Scientific, Waltham, MA, USA). Paired-end reads (2×250 bp) were produced by sequencing pooled amplicons from each library on an Illumina MiSeq platform at Magigen Biotechnology (Guangzhou, China). Raw reads from each library were trimmed and assembled using CLC Genomics Workbench (version 6.0.2, CLC Bio, Denmark). A quality score of 0.05 was used for trimming. Sequences were discarded if they (1) contained more than one ambiguous nucleotide, (2) lacked a complete barcode and primer at one end, or (3) were shorter than 200 bp after removal of the barcode and primer. Parameters used for de novo assembly were: mismatch cost of 2, insert cost of 3, minimum contig length of 200 bp and similarity of 0.8. Sequences were clustered into operational taxonomic units (OTUs) using a 97% similarity cutoff. The representative sequence of each phylotype was aligned against the SILVA database (release 132) with a confidence threshold of 80%. All archaeal and chloroplast sequences were removed before downstream analysis. A model testing tool was run to identify the most suitable model for creating a phylogenetic tree, and recommended the maximum likelihood phylogeny method.
Inference of interspecific interactions
We constructed interspecific interactions between bacterial OTUs using the CoNet plugin (v. 1.1.1.beta) in Cytoscape software (v. 3.5.1), as described previously (Faust and Raes 2016). To reduce noise and false-positive predictions, network inclusion was restricted to OTUs that had at least 80% sample appearance. In each network, pairwise associations between OTUs were simultaneously identified by an ensemble of correlations (Pearson and Spearman coefficients) and distance metrics (Kendall distance, Bray–Curtis distance and Kullback–Leibler dissimilarity measures). The initial 500 top- and bottom-ranking edges were maintained in the network. A total of 1000 permutation scores and 1000 bootstrap scores were calculated for each edge and each measure of association. The measure-specific P-values from multiple interaction metrics were merged using the Simes method (Sarkar and Chang 1997). The false discovery rate correlation was performed using the Benjamini–Hochberg multiple procedure (Benjamini and Hochberg 1995). To test the reliability of networks, 1000 Erdös–Rényi random networks were obtained with the R package ‘igraph’ using the same numbers of nodes and edges as the empirical networks. Properties of co-occurrence networks were calculated, including the number of nodes and edges, degree, transitivity, density, average path distance (APD), diameter and betweenness. To infer the interspecific interactions of core sub-community, OTUs that appeared in all samples were defined as the core OTUs, which consist of the core network.
Statistical analyses
All analyses and visualizations were performed in the R environment (version 3.2.2, http://www.r-project.org) using the RStudio interface (version 1.1.463).
An unconstrained principal component analysis (PCA) was performed on water physicochemical parameters to explore the variance of the aquatic environment. The comprehensive trophic level index (TLI), which integrates multiple physicochemical parameters, was used to classify the degree of trophic state of each successional stage, as described in Liu et al. (2020). Statistical differences in environmental parameters between distinct successional stages were tested using the non-parametric Kruskal–Wallis test. Non-linear relationships were checked by fitting a generalized additive model between the response and explanatory variables.
For the 16S sequencing data, rarefied OTU tables were created using the minimum numbers of reads (25 869 reads) among all samples. Relative abundance of specific phylogenetic groups was computed as the number of sequences affiliated with the group divided by the total number of sequences per sample. Richness, Shannon and Faith's PD indices were calculated as a measure of alpha-diversity for the communities. Statistical differences of each index were tested by the non-parametric Kruskal–Wallis test. Analysis of Bray-Curtis dissimilarity-based non-metric multidimensional scaling (NMDS) was used to visualize the beta-diversity of bacterial communities. Variation within each successional stage was calculated using the Euclidean distance of all samples to the centroid of each stage. Hierarchical clustering was used to separate the 12 successional stages into groups. We used analysis of similarity (ANOSIM) and PERMANOVA with 999 permutations to test for differences in bacterial community composition between distinct successional stages. A score of 1 in the ANOSIM test statistic r indicates complete separation of communities; a score of 0 indicates no separation. Comparison of the relative abundance of individual phyla was conducted with STAMP (version 2.1.3).
We used the analytical framework proposed by Stegen et al. (2013) to evaluate the relative influence of stochastic and deterministic processes, and to identify the contribution of specific assembly mechanisms for each bacterial community. This approach uses the beta mean nearest taxon distance (βMNTD) to represent the pairwise phylogenetic turnover between communities, and the beta-nearest taxon index to represent the environmental impacts calculated by the standard deviation of the observed βMNTD from the βMNTD of the null model. To test the phylogenetic signal across short phylogenetic distances, we estimated each OTU niche value for TLI and explored the correlations between niche differences and phylogenetic distances by Mantel correlogram (Fig. S1). When beta-nearest taxon index (βNTI) < –2 and ≥2 were identified as homogeneous selection and heterogeneous selection, respectively. Moreover, 999 random permutations of communities generate a null distribution of Bray–Curtis dissimilarity, and a Raup–Crick metric (RCbray) is subsequently calculated by comparing empirically observed Bray–Curtis and simulated null distribution. The RCbray < –0.95 and RCbray≥0.95 were identified as homogenizing dispersal and dispersal limitation, respectively. When the |βNTI|<2 and |RCbray|<0.95 were identified as Undominated (Dini-Andreote et al. 2015).
As described previously (Yuan et al. 2021), community stability was calculated using the sample×OTU matrix as
where v is the number of samples taken from the individual successional stages, S is the total number in the community and yi, k is the relative abundance of OTU k in sample i. Higher values of community stability indicate a more stable community. If community structure has no change, that is, yi, k = miniyi, k, this stability index is equal to 1; if community structure is completely different, that is, miniyi, k = 0, this stability index is 0. Statistically, the correlations between community stability and environmental and network topological parameters were tested by Pearson two-tailed correlation.
Data deposition
The raw sequence data reported in this paper have been deposited in the Genome Sequence Archive (Genomics, Proteomics, and Bioinformatics 2017) of the BIG Data Center, Beijing Institute of Genomics (BIG), Chinese Academy of Sciences, under the accession number CRA007806. The data are publicly accessible at https://bigd.big.ac.cn/gsa.
Results
Physicochemical parameters across the transition from the MD to the PD regime
An unconstrained PCA was used to analyze the variance of the physicochemical parameters across the transition from the MD to the PD regime. Axis1 and Axis2 accounted for 78.8% and 15.6% of variation, respectively. Axis1 mainly associated with nutrients such as TN, TP and with Chl a, while Axis2 strongly correlated with physical properties including water transparency and TSS. The directional path from successional St1 to successional St12 was congruent and moved in lockstep with the trophic gradient of the lake, as measured by the TLI (Fig. 2A). Hierarchical clustering analysis divided the 12 stages into three clusters: St1-St4, St5-St10 and St11-St12 (Fig. 2B). In general, the physicochemical parameters in St1-St4 and St11-St12 were significantly more variable than in St5-St10 (Fig. S2, Kruskal–Wallis test, P < 0.01). On average, the physicochemical parameters between any of the hierarchical clusters were significantly distinct (PERMANOVA test, P < 0.001), but there were no differences in parameters between any consecutive stages (Kruskal–Wallis test, P > 0.05). Of the various physicochemical parameters, water transparency exhibited a gradual decreasing trend along the path from St1 to St12, while DOC showed a concave-upward trend line. All other parameters showed generally increasing trends from St1 to St12 (Fig. 2C). Physicochemical parameters were not significantly different between consecutive stages (Kruskal–Wallis test, P > 0.05).
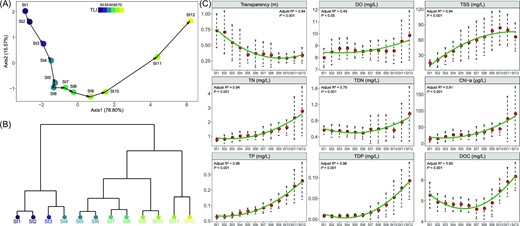
Change of physicochemical parameters from St1 to St12: (A) the principal component analysis of aquatic environment; (B) the clustering analysis based on Euclidean distance; (C) the variation of specific parameters from St1 to St12.
Dynamics of bacterial communities and community assembly processes across the transition from MD to PD types
On average, sequencing produced 28 486 high-quality reads per site, all of which were clustered into a total of 1382 OTUs. Bacterial α-diversity, as expressed by Richness, Shannon and Faith's PD, increased slightly from St1 to St12 with no significant differences between any pair of stages (Fig. 3A, Kruskal–Wallis test, P > 0.05). They all showed significantly positive relationships with Chl-a, DO, TN and TP (Pearson test, P < 0.05, Fig. S3). By connecting the consecutive stages from St1 to St12 to represent community trajectory, the NMDS plot displayed a directional transition of bacterial communities from the MD to the PD type (Fig. 3B). Clustering analysis grouped the 12 stages into three clusters: St1-St6, St7-St9 and St10-St12 (Fig. 3C), which could be defined as the MD type, the intermediate type and the PD type, respectively. Although there were no detectable differences between any two consecutive stages in each cluster (PERMANOVA test, P > 0.05), any stage in the MD type was significantly distinct from any stage in the PD type (PERMANOVA, P < 0.05). Bacterial communities in the MD and the PD types were significantly less variable than in the intermediate state (Fig. S4), as indicated by comparing the average Euclidean distance in each stage. Moreover, the Gaussian kernel density estimation found that the frequency distribution of bacterial communities from St1 to St12 showed bimodality along the NMDS1 scores.
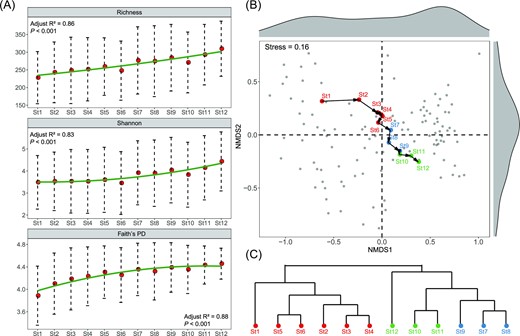
α-diversity (A), β-diversity (B), and the clustering analysis based on Bray–Curtis distance (C) of bacterial communities from St1 to St12.
The bacterial communities within Lake Taihu were numerically dominated by the phyla Firmicutes (50.9%±9.2%), followed by Proteobacteria (18.8%±1.9%) and Actinobacteria (12.8%±2.0%). The top 11 dominant phyla changed notably from St1 to St12 (Fig. 4A). STAMP analysis indicated that the relative abundance of these phyla did not change significantly between consecutive stages (STAMP analysis, P > 0.05). However, seven out of the 11 dominant phyla significantly differed between both the MD type and the intermediate type and between the intermediate state and the PD type (Fig. 4B, STAMP analysis, P < 0.05), which generally showed three distinct abundance distributions. The proportion of Actinobacteria and Proteobacteria changed little between the MD and the PD types. Firmicutes and Bacteroidetes decreased in proportion from the MD to the PD types, whereas Planctomycetes, Cyanobacteria and Verrucomicrobia all increased, reaching moderately high abundance in the PD type.
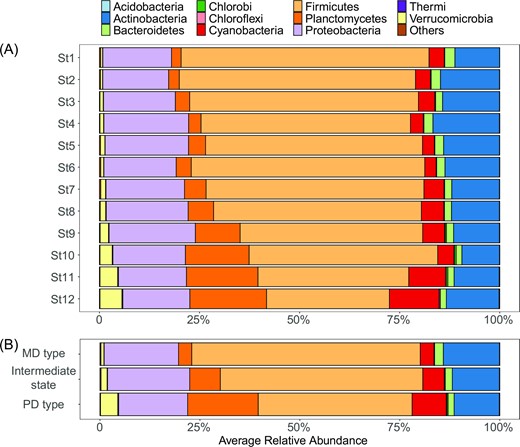
Bacterial community profile from St1 to St12 (A) and from MD to intermediate type to PD types (B).
Community stability and its drivers during the transition from MD to PD types
Taxon interactions within bacterial communities changed dramatically from the MD to the PD type based on the 12 community networks (Fig. 5), whose reliability and non-randomness were tested by comparison with their corresponding random networks (Table S1). Within these 12 bacterial communities, the proportion of positive edges far exceeded that of negative edges (82.82% vs. 17.18%). However, there was a gradually increasing trend of negative edges from the MD to the PD type (from 11.04% to 26.73%). On average, there were approximately 97 nodes and 1034 edges across the networks, with the lowest number of nodes and the highest number of edges occurring in the intermediate type, indicating communities were most tightly connected in this state. Across the St1-to-St12 network, there was a gradually decreasing ratio of positive to negative interaction, declining from 7.68 to 2.79. Network topological parameters also implied that the community in the intermediate state was more complex than either the MD or the PD type, which was implied by the concave-downward trends of degree and density and by the concave-upward trends of APD, diameter and betweenness (Fig. 5). In addition to the networks of each of the 12 communities, the core sub-network of bacterial communities across all stages was also explored. This consisted of 30 shared nodes across all 12 community networks. Topological parameters across the whole network exhibited non-linear change. By contrast, edge, degree, density and transitivity of the whole network decreased linearly from St1 to St12, while APD, diameter and betweenness increased linearly, which implied a rising incompact structure of core bacterial communities from the MD to the PD type.
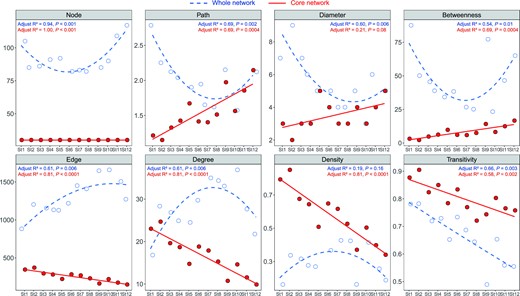
Changes of network topological parameters from St1 to St12 for the whole network and the core sub-network of bacterial communities.
To reveal the community assembly processes responsible for structuring the bacterial communities across the 12 stages, the phylogenetic β-nearest taxon index (βNTI) was calculated based on null model analysis (Fig. 6A). The βNTI distribution gradually shifted from deterministic processes operating within St1-St2 (βNTI < −2), to stochastic processes within St3-St10 (−2 < βNTI < 2), and ultimately back to deterministic processes within St11-St12 (βNTI > −2). Additionally, the relative contribution of different ecological processes was quantified (Fig. 6B, Fig. S5). Within the deterministic processes, the homogenous selection showed a constant trend (23.43%), whereas the heterogenous selection was higher in the MD and PD types than in the intermediate state (29.22% vs. 19.26%). Within the stochastic processes, the contribution of dispersal limitation was generally constant (10.91%), while the homogenous dispersal gradually increased from St1 to St9 (from ∼20% to ∼50%) and then decreased from St9 to St12 (from ∼50% to ∼13%). Last, the undominated processes slightly increased from St1 to St7 and oscillated from St8 to St12.
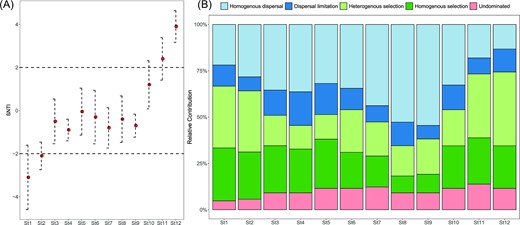
Change in the β-nearest taxon index (A) and the contribution of different ecological processes to the assembly of the bacterial community from St1 to St12 (B).
The community stability showed a concave-upward trend across the transition of bacterial communities from the MD to the PD types (Fig. 7A). Stability decreased sharply from St1 to St7, at which point it reached its minimum. It then increased sharply from St7 to St12. This implies that stability is higher in the MD and PD types than in the intermediate states. Further, there was a significant relationship between community stability and the whole network topological parameters (Pearson test, P < 0.05). Specifically, community stability positively associated with the number of nodes, APD and betweenness, and negatively associated with density and degree (Pearson test, P < 0.05, Fig. 7B). However, community stability did not significantly associate with the topological parameters of the core sub-network (Pearson test, P > 0.05, Fig. 7B). By contrast, there were no significant relationships between community stability and any of the physicochemical parameters (Pearson test, P > 0.05).
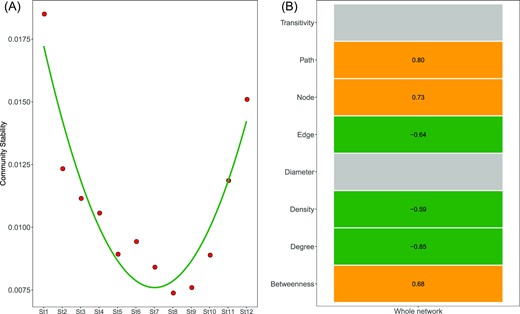
Community stability of bacterial communities from St1 to St12 (A) and its relationships with network topological parameters (B). The gray tile indicates the insignificant relationships.
Discussion
By spatially dividing Lake Taihu into 12 geographic areas that represent successional stages ranging from clearer water dominated by macrophytes to more turbid water dominated by phytoplankton, we were able to model the ecological dynamics of bacterial communities along an increasing trophic gradient. In terms of α- and β-diversity and compositional structure, our results demonstrate that bacterial communities were comparable between any pair of consecutive stages. Given the gradually increasing trophic status from St1 to St12, our results confirmed the first hypothesis that the bacterial communities most likely show continuous responses to gradually changing environments. This finding is also rooted in observations from other fields. For example, during the drinking water treatment process, sequentially changing chemical treatments tends to steer an aquatic community through a continuum (Zhang et al. 2017). More importantly, our results indicated that although the bacterial community existed across a continuum, the communities could still be clustered into three groups: the MD type, the intermediate type and the PD type. As has been shown previously in other lakes (Wang et al. 2020, Cao et al. 2021), the bacterial communities were significantly different between the MD and PD types, suggesting that in eutrophic lakes, the shift from the MD to the PD state produces alternative community types. Together, these two observations address one of the initial questions raised in the introduction: the bacterial community transits from MD to PD types gradually.
Inspired by the concept of a stability landscape, theoretical studies have regarded the transition of bacterial community types as an ecological transition that shifts communities from stability to instability, then back to stability (Gibson et al. 2016, Pettersson et al. 2020). In the present study, the stability pattern of bacterial communities along the trophic gradient quantitatively validated this assumption. First, Gaussian kernel density estimation suggested that the frequency distribution of the bacterial community state from St1 to St12 showed bimodality along the NMDS1 scores, that most bacterial classes tended to be either rare or abundant in the MD and PD types. Given this, it is expected that two alternative stable states exist in the MD and PD types. Second, the stability of bacterial communities was lower in the intermediate state than in the alternative MD and PD types. These two results are therefore congruent with the prediction of dynamical systems theory that alternative stable states are separated by an intermediate unstable region (Lahti et al. 2014, Carrier-Belleau et al. 2022). Further, by regarding the ordination plots as the phase space, the NMDS plot highlighted the higher dispersal distribution of bacterial communities in St7-St9, suggesting that the intermediate state is less attractive than the contrasting alternative types, which may correspond to the transitional phase between alternative stable states. From the collective evidence, we conclude that collapse and re-establishment of stability may steer the transition of bacterial communities from the MD to the PD type.
Given the apparent relationship between community stability and the transition of alternative community types, a second intriguing question is how do bacterial communities maintain their stability. Diversity is widely thought to guarantee the stability of biotic communities, owing to the high redundancy in rich communities (Biggs et al. 2020, Lucini et al. 2020). However, our results largely counter this perspective, in that, across all stations, α-diversity of bacterial communities showed no correlation with the community stability. Instead, there was even a downward trend in stability with increasing diversity from St1 to St7. This suggests that diversity has a detrimental effect on stability, consistent with the pioneering work of May (1972). In an effort to reconcile this paradox, recent empirical observations and theoretical predictions have posited that the relationship between diversity and stability is not due strictly to a richness effect (McCann 2015), but is also mediated by species interactions (Okuyama and Holland 2008, Montesinos-Navarro et al. 2019).
In general, bacterial species interact considerably with each other either by positive interactions, which reflect the degree of cooperative behaviors, or by negative interactions, which indicate the magnitude of competitive behaviors (Faust and Raes 2012). Across the St1-to-St12 network, there was a gradually decreasing ratio of positive to negative interaction, declining from 7.68 to 2.79, suggesting a switch from more mutualistic to more antagonistic behaviors as trophic status increased. As the stress gradient hypothesis predicts, high degrees of stress encourage mutualistic interactions between bacterial species as a mechanism to cope with the increasing stress (David et al. 2020, Hesse et al. 2021). Across the transition of bacterial communities from MD to PD types, the increasing trophic state of Lake Taihu serves to relieve at least some nutrient stress, such that bacteria can survive with reduced benefits from mutualist partners. This in turn leads to a decreasing ratio of mutualistic to antagonistic interactions (Hernandez et al. 2021).
Compared with random taxon interactions, empirical taxon interactions in each successional stage formed a self-structured and self-sustaining assortment of community networks, which exhibited unique topological characteristics (Barberan et al. 2012). Our results demonstrated that the community networks from Stages 7–10 harbored the fewest number of nodes, the largest edges and degrees, the shortest average path lengths and the lowest diameter and betweenness. All of these parameters indicate that bacterial communities are more complex in the intermediate type than they are in the alternative MD and PD types (Mougi and Kondoh 2012, Kondoh and Mougi 2015). The instability of bacterial communities in the intermediate state implies that complexity may serve as a destabilizing factor (Landi et al. 2018, Yonatan et al. 2022). Additional observations that support this implication include significant positive correlations between community stability and node, average path length and betweenness, and negative correlations between stability and density and degree. A candidate mechanism for the adverse effect of complexity on stability is that higher complexity often brings dependency among species. Accordingly, if one species in a dependent relationship varies in abundance, it tends to pull others down with it in a feedback loop that is termed a ‘mutual downfall’ (Coyte et al. 2015, Stone 2020). Notably, this destabilizing mechanism is threshold-based, and bacterial communities lose stability only if complexity is above some critical level (Landi et al. 2018, Pettersson et al. 2020). Thus, pressures selecting for stability tend to constrain complexity of bacterial communities by pushing them toward either small and highly interacting communities, or large but weakly interacting communities (Yonatan et al. 2022).
In addition to taxon interactions, our study also explored the role of assembly processes in community stability. A large body of research has demonstrated that bacterial communities are governed by a combination of deterministic and stochastic processes in different habitats and distinct dynamical stages (Dini-Andreote et al. 2015, Chang and HilleRisLambers 2016). Recent studies have found that deterministic processes were primarily responsible for shaping the assembly of bacterioplankton communities in oligotrophic, mesotrophic and hypertrophic shallow lakes (Llames et al. 2017, Aguilar and Sommaruga 2020). Our results showed that the primary processes directing community assembly shifted from deterministic to stochastic, and back to deterministic as communities progressed across trophic gradients from mesotrophic to hypereutrophic. In general, productive environments promote random dispersal such as ecological drift and colonization, which increases the importance of stochastic processes (Chase 2010, Zhou et al. 2021). In addition, the areas of mesotrophic to moderately eutrophic states in Lake Taihu are located in its central region, which are subjected to strong wind-induced disturbances that likely enhance the randomness of bacterial dispersal. As a consequence, in these areas, stochastic processes would be expected to dominate over deterministic processes. By contrast, deterministic processes dominated community assembly in areas that were moderately hypereutrophic, consistent with the idea that habitat filtering increases with environmental extremes. In hypereutrophic states, increased stress favors better-adapted species, while constraining the least adapted, which strengthens homogeneous selection (Geng et al. 2022). Notably, our results indicate that the dominance of stochastic processes was synchronized with the unstable transitional state, which implies that stochastic processes may weaken the stability of bacterial communities. This implication is exactly what the phenomenon of critical slowing down predicts. Stochastic processes cause a system to switch back and forth between alternative states by disrupting their stability. Ultimately, random shocks trigger a change in state from MD to PD types in those bacterial communities with low resilience to disturbance (Brock and Carpenter 2010).
Conclusion
Understanding how bacterial community types transition is important in elucidating the dynamics of microbial communities. This study divided the transition of bacterial communities from MD to PD types along a trophic gradient into 12 stages. Our results demonstrate that bacterial communities were not significantly different between any consecutive stages, suggesting that transition to alternative bacterial communities appears in a continuous gradient. At the same time, stability of bacterial communities was significantly lower in the intermediate type than in the MD and PD types, hinting that the collapse and re-establishment of stability steers the transition toward alternative types. Further, our results showed that high complexity of species interactions and strong stochastic processes disrupt the stability, leading the transition of alternative community types. Ultimately, we conclude that stability regulates the gradual transition of bacterial communities from MD to PD types along a trophic gradient.
Author contributions
Yang Hu (conceptualization, data curation, formal analysis, writing – original draft), Jian Cai (formal analysis), Ying Gong (methodology), Changqing Liu, Xingyu Jiang (investigation), Xiangming Tang, Keqiang Shao and Guang Gao (conceptualization).
Acknowledgements
We gratefully acknowledge Taihu Laboratory for Lake Ecosystem Research (TLLER) and Jingchen Xue for helping with sample collection and water chemical analysis.
Conflict of interest
We declare that we have no financial and personal relationships with other people or organizations that can inappropriately influence our work, there is no professional or other personal interest of any nature or kind in any product, service and/or company that could be construed as influencing the position presented in, or the review of, the manuscript entitled.
Funding
This work was funded by Jiangsu Province Carbon Peaking and Carbon Neutrality Science and Technology Innovation Project (BK20220018), National Natural Science Foundation of China (41907207), Open Fund of the Key Laboratory of Water Governance in the Taihu Lake Basin of the Ministry of Water Resources (Yk922001-C5).