-
PDF
- Split View
-
Views
-
Cite
Cite
Diana Navrátilová, Petra Tláskalová, Petr Kohout, Pavel Dřevojan, Karel Fajmon, Milan Chytrý, Petr Baldrian, Diversity of fungi and bacteria in species-rich grasslands increases with plant diversity in shoots but not in roots and soil, FEMS Microbiology Ecology, Volume 95, Issue 1, January 2019, fiy208, https://doi.org/10.1093/femsec/fiy208
- Share Icon Share
Abstract
Microbial communities in roots and shoots of plants and in soil are important for plant growth and health and take part in important ecosystem processes. Therefore, understanding the factors that affect their diversity is important. We have analyzed fungal and bacterial communities associated with plant shoots, roots and soil over a 1 km2 area in a semi-natural temperate grassland with 1–43 plant species per 0.1 m2, to describe the relationships between plant and microbial diversity and to identify the drivers of bacterial and fungal community composition. Microbial community composition differed between shoots, roots and soil. While both fungal and bacterial species richness in shoots increased with plant species richness, no correlation was found between plant and microbial diversity in roots and soil. Chemistry was a significant predictor of bacterial and fungal community composition in soil as was also the spatial location of the sampled site. In this species-rich grassland, the effects of plants on the microbiome composition seemed to be restricted to the shoot-associated taxa; in contrast, the microbiomes of roots or soil were not affected. The results support our hypothesis that the effect of plants on the microbiome composition decreases from shoots to roots and soil.
INTRODUCTION
Microbes play an important role in the functioning of terrestrial ecosystems, where they inhabit various habitats and act together with macroorganisms to provide ecosystem services (Baldrian 2017). Microbes are often recognized as major drivers of ecosystem processes, but they also structure ecosystem composition, e.g. by their influence on plant diversity (van der Heijden, Bardgett and van Straalen 2008; Schnitzer et al. 2011). It has been estimated that at least 20 000 plant species need soil microbial mutualists to persist (van der Heijden, Bardgett and van Straalen 2008); in contrast, microbial plant pathogens play a significant role in plant species coexistence (Bagchi et al. 2014). Because of the role of vascular plants as the dominant primary producers in terrestrial ecosystems, the plant microbiome, a diverse, multicomponent system of plant associates, is highly important part of these ecosystems (Lebeis 2015). The major plant-associated habitats, aboveground (i.e. shoots) and belowground (i.e. roots), are highly distinct in composition of their microbial communities due to their different habitat characteristics (Lebeis 2015; Baldrian 2017).
Considering the vulnerability of living plant tissues to pathogen attack, it is not surprising that plants apply mechanisms that allow them to select their microbial associates (van der Heijden, Bardgett and van Straalen 2008; Lundberg et al. 2012). Roots of plants exclude certain bacteria and fungi and promote the establishment of others that represent their species-specific associates, including beneficial bacteria and mycorrhizal fungi (Meadow and Zabinski 2012; Hiiesalu et al. 2014; Dean et al. 2015). One of the observed drivers of this plant-microbiome specificity is the production of specific root exudates (Broeckling et al. 2008; Eisenhauer et al. 2017). Although the establishment of shoot microbial communities seems to be more stochastic, and these communities show considerable variation over time (Penuelas et al. 2012; Lebeis 2015), plant-host specificity of microbiomes is observed in the foliage as well (Wearn et al. 2012; Laforest-Lapointe, Messier and Kembel 2016; Vincent, Weiblen and May 2016).
In addition to selecting specific symbionts in their roots and shoots, plants were also demonstrated to affect microbial communities in soils (Barberán et al. 2015; Urbanová, Šnajdr and Baldrian 2015; Gibbons et al. 2017). This is not surprising if we consider that plant specific fungal root symbionts extend into soil, and dominant plants significantly affect soil nutrient contents and physicochemical properties (Šnajdr et al. 2013; Gibbons et al. 2017). Additionally, it is reported that recent plant activity moderates the rhizosphere environment by the specific composition of root exudates (Steinauer, Chatzinotas and Eisenhauer 2016).
The selection for specific microbiomes in various living parts of plants and in soil should, in theory, lead to a positive link between plant and microbial alpha diversity, i.e. local number of taxa (Wardle 2006; Prober et al. 2015; Urbanová, Šnajdr and Baldrian 2015). Although this topic has puzzled scientists for a long time, specifically considering the soil microbiome, the results remain inconclusive. While some studies find limited associations between plant and soil microbial alpha diversity (e.g. McElroy, Papadopoulos and Adl 2012; Prober et al. 2015; Tedersoo et al. 2016), there are several others where such relationships were demonstrated, both in experimental setups (Milcu et al. 2013) and in real ecosystems (Meadow and Zabinski 2012; Hiiesalu et al. 2014; Yang et al. 2017). However, such evidence exists mostly for microbes with obligately symbiotic lifestyle. Additionally, for root microbes, there are reports that demonstrate links between plant diversity and diversity of microbes, especially fungi (Millard and Singh 2010; Meadow and Zabinski 2012; Gao et al. 2013), as well as others that show no link or show a more pronounced effect of soil chemistry (Millard and Singh 2010; David, Seabloom and May 2016). For plant shoot endophytes, studies linking host plant and microbe alpha diversity are missing, but the host specificity often varies (Carrell and Frank 2015; Vincent, Weiblen and May 2016).
At present, the results appear to be largely inconclusive (Fierer 2017). This lack of consensus is probably at least partially due to the experimental limitations of studies performed so far. While environmental conditions are easy to control in manipulative experiments, it is difficult to achieve equivalent numbers of plant species and their relative abundances as those in natural conditions (Waldrop et al. 2006; Milcu et al. 2013; Schlatter et al. 2015; Eisenhauer et al. 2017). In contrast, the analysis of natural plots may introduce problems in terms of spatial scales, where the space itself, soil properties or climate are known to be additional factors affecting the diversity of both plants and microbes (Wardle 2006; de Vries et al. 2012; Gao et al. 2013; Chen et al. 2017). Detection of plant-microbial diversity link also depend on the sampling design of the study; for example, plant diversity may be estimated over large plots, while only small samples are used for the enumeration of microbial diversity (Regan et al. 2014; Prober et al. 2015). Finally, the results from forests ecosystems are also difficult to interpret due to the multi-layered structure of vegetation (Peay, Baraloto and Fine 2013; Prescott and Grayston 2013; Urbanová, Šnajdr and Baldrian 2015). It appears that a reasonable experimental design that allows for the proper analysis of a suitable model is required, yet this task is difficult to achieve.
In this study, we explore a small area of natural grasslands (<1 km2) that allows us to a large extent avoid the limitations of the previously studied systems, that is to reduce the differences in climate, bedrock and soil properties. Steep plant species gradient (1–43 species per 0.1m2) within this scale allows us to analyze the relationships between the alpha diversity of plants and those of fungi and bacteria that would not be strongly confounded by other factors. By sampling all the aboveground plant biomass, the whole-root biomass, and the whole block of soil of each plot, we aimed to collect sufficiently representative samples for metabarcoding analyses of the bacterial and fungal communities associated with plant shoots, roots and soil.
We expect that the effects of plant species on associated microbes depend on the properties of each compartment (i.e. shoot, root and soil), since these differ in connectedness of their microbiomes: in shoots, microbiomes of individual plants are separated; in soil, the microbiome is common while the roots of plants are spatially separated, although connected by common fungal symbionts. The plant compartment properties also differ in the prevailing source of colonization (i.e. local soil for roots, atmosphere long-distance transport for shoots; Lebeis 2015). Thus we hypothesize that (i) the plant species richness has a decreasing effect on microbial richness from shoots through roots to soil, (ii) stronger plant diversity effect can be observed when plant phylogenetic diversity is taken into account considering that their traits are likely more conserved at short phylogenetic distances (Martiny, Treseder and Pusch 2012).
In addition to the question about plant-microbiome diversity relationship, we investigated which additional factors contributed to the assemblage of the microbial communities and how important the plant community composition was as a driver of microbial community assemblage. Because of the known associations between fungi and plant roots and the extension of fungal mycelia from roots into soil, we hypothesize that (iii) there is a stronger effect of plant diversity and community composition on the diversity and community composition of fungi than on bacteria in roots and soil. For the same reason, and because bacteria are spatially constrained to soil microhabitats, such as individual soil pores that are outside the influence of plants (Fierer and Jackson 2006; Fierer 2017), we hypothesize that (iv) soil bacterial community composition will be more affected by soil chemistry than the fungal communities.
MATERIALS AND METHODS
Study site and sampling design
The study was carried out in the Čertoryje National Nature Reserve in the eastern part of the Czech Republic in Central Europe (48.85°N, 17.41°E, 350–430 m a. s. l.), which represents an extensive complex of species-rich and semi-dry wooded meadows. Local plant species diversity reaches the highest numbers ever recorded on Earth at a spatial scale between 0.1 and 49 m2 (Wilson et al. 2012). The meadows are developed on deep, slightly calcareous soil that dries out during low-precipitation periods in summer. The meadows are mown once a year in June or July as a part of nature conservation management; for more details see Jongepierová (2008) and Chytrý et al. (2015). Vegetation sampling was performed within 2 days in June 2014, before summer mowing.
Twenty-five sampling plots, each with an area of 0.1 m2 (Supplementary Table 1), were distributed over ∼1-km2 area and selected to cover the widest available gradient of local vascular plant species richness (i.e. from 1 to 43 species, the latter being the highest number ever recorded globally for this plot size). Presence/absence of all vascular plant species was recorded at each plot, and the number of species (i.e. plant species richness) was counted. Plants were determined by botanists familiar with local flora following the taxonomy and nomenclature of Kubát et al. (2002). Complete aboveground vegetation biomass was collected at each plot. Furthermore, the complete block of soil from the entire plot, up to a depth of 10 cm, was removed, and both vegetation and soil blocks were transported to the laboratory, stored at 4°C and processed within 5 days. The samples of aboveground plant biomass (i.e. shoots), belowground plant biomass (i.e. roots) and soil were separated, resulting in 75 samples in total. The roots were manually separated from the soil, and the fresh root biomass was recorded. All attached soil was washed away from roots, and roots were briefly air dried. Soil was sieved through a 5-mm sieve, and two 25-g subsamples were collected, representing mixed bulk soil sample for the plot. Sieves were sterilized between plots. The whole shoot and root biomass were both cut with scissors into <1-cm pieces and thoroughly mixed. The samples from all three compartments were freeze-dried and stored at −20°C. The content of available P, N, Ca, Mg, K, the pH and the C/N ratio were determined in the soil samples. C content was measured in a commercial laboratory using sulphochromic oxidation, and N content was measured by sulphuric acid mineralization with the addition of selenium and sodium sulphate and the conversion to ammonium ions. Other elements were measured after Mehlich III extraction. The pH was determined after mixing 1 g of soil with 10 mL of distilled water. The soil organic matter content was determined as the loss on ignition at 650°C. The soil moisture was calculated as the difference in mass before and after freeze-drying.
Molecular analysis
Freeze-dried shoots and roots were milled into a fine powder. DNA from shoots and roots was isolated with a DNeasy Plant Maxi kit (Qiagen), and two extractions were performed per sample using a 250-mg sample per extraction. DNA from soil was extracted using a modified SK method (Sagova-Mareckova et al. 2008), omitting the CaCl2 treatment step. Two extractions were performed per sample using a 250-mg sample per extraction. Extracted DNA was subsequently cleaned with a GeneCleanTurbo kit (MP Biomedicals Solon), and DNA extracts were pooled for each sample.
The eubacterial primers 515F/806R (Caporaso et al. 2012) and fungal primers nu-SSU-0817/nu-SSU-1196 (Borneman and Hartin 2000) were used to amplify bacterial 16S and fungal 18S rDNA. The fungal primer pair used to target SSU was chosen because amplification with combinations of ITS-targeting primers (Ihrmark et al. 2012) yielded excessive amounts of plant-derived amplicons (>95% in multiple samples of plant roots or shoots). In contrast, the used primers combine good coverage of fungi and efficient exclusion of plant DNA—less than 5% in all samples (Borneman and Hartin 2000; Prévost-Bouré et al. 2011). PCR amplifications were performed with three independent PCR reactions per sample as described previously (Žifčáková et al. 2016). PCR reactions contained 2.5 μL of 10x buffer for DyNAzyme DNA Polymerase, 0.75 μL of bovine serum albumin (20 mg mL−1), 1 μL of each primer (0.01 mM), 0.5 μL of PCR Nucleotide Mix (10 mM each), 0.75 μL polymerase (2 U μL−1 DyNAZyme II DNA polymerase, 1:24 Pfu DNA polymerase) and 1 μL of template DNA. Cycling conditions for fungal amplicons were 94°C for 5 min, 35 cycles at 94°C for 1 min, 56°C for 1 min, 72°C for 1 min and a final extension at 72°C for 10 min. Conditions for bacterial amplicons were 94°C for 5 min, 35 cycles at 94°C for 1 min, 50°C for 1 min, 72°C for 1 min and a final extension at 72°C for 10 min. The PCR products of each sample were pooled and purified using a MinElute Purification Kit (Qiagen). Equimolar mixtures of amplicons were used for library preparation using the TruSeq® DNA PCR-Free LT Kit (Illumina) and sequenced on the Illumina MiSeq platform.
Bioinformatic analysis and statistics
The amplicon sequencing data were processed using the pipeline SEED 2.0.1 (Větrovský, Baldrian and Morais 2018). Briefly, pair-end reads were merged using fastq-join (Aronesty 2013), and sequences were quality trimmed. Chimeric sequences were detected using UCHIME, which is included in Usearch 7.0.1090 (Edgar 2010), and deleted. The resulting sequences were clustered into operational taxonomic units (OTUs) using UPARSE implemented within Usearch 7.0.1090 (Edgar 2013) at a 98% similarity level for fungi and a 97% level for bacteria. A consensus sequence was constructed for each OTU, and the closest hits at the genus or species level were identified using UNITE (Koljalg et al. 2013) and GenBank, and all non-bacterial OTUs (typically <30%) and non-fungal OTUs (<5%) were deleted. As a measure of fungal and bacterial alpha diversity, OTU richness was calculated for 6500 or 8000 randomly chosen sequences per sample, respectively. These sequence numbers were chosen according to sample with minimum sequence count. DNA sequences: NCBI SRA: PRJNA421086.
All statistical analyses were performed with the vegan, nlme, betapart and ecodist packages of R (R Core Team 2016). In all cases, differences at P < 0.05 were considered to be statistically significant.
General least squares (GLS) models were built to separately identify the main predictors of bacterial and fungal OTU richness in each of the studied compartments. Soil parameters (C, N, P, Ca, Mg, K, C/N, C/P, N/P, pH, soil organic matter (SOM) and moisture), plant species richness and root biomass were used as predictor variables. Multicollinearity between predictor variables was checked by calculating the variance inflation factor (VIF); variables with VIF >10 were excluded prior to model selection. The following variables were included in the final model for both the fungal and bacterial datasets: moisture, P, N, C/N, pH, Mg, K, Ca, N/P and plant species richness for shoot compartment, plus root biomass for root and soil compartments. One to five predictors were used for the creation of GLS models. The best model was selected according to the corrected Akaike information criterion (AICc). The robustness of the best model was further evaluated by averaging models that fell into the 95% AICc confidence set. Beta coefficients (slopes) of individual models were weighted according to their Akaike weight across all models and evaluated as the mean ± 95% confidence intervals. Zero values were conservatively used for non-significant variables in individual models. Variables were considered significant when their confidence intervals excluded the value zero.
We tested also effect of plant phylogenetic diversity on microbial OTU richness, by building GLS models as described above, using plant phylogenetic diversity as one of predictor variables. Phylogenetic diversity of plant communities was calculated as Faith's PD (Faith 1992) using the picante 1.6–2 package (Kembel et al. 2010) in R. A dated, ultrameric phylogenetic tree for the plant species found in our samples was prepared by pruning the DaPhnE 1.0 supertree (Durka and Michalski 2012), with a few missing taxa added to the existing nodes. The root node was included in the calculation, meaning that PD is the sum of branch lengths connecting the plant species recorded at each plot to the most recent ancestor of these species.
Community analysis was based on fungal and bacterial OTUs with at least 10 and 20 sequences in the whole dataset, respectively. OTU abundances across samples were standardized using the Hellinger transformation, and Bray–Curtis dissimilarity was used as a measure of dissimilarity between samples. Soil chemistry data were log-transformed (except for pH) and reduced to a Euclidean distance matrix. The resulting OTU and soil matrices were used in subsequent analyses.
Non-metric multidimensional scaling (NMDS) ordination based on the Bray–Curtis dissimilarities was used to visualize differences in the fungal and bacterial communities in shoots, roots and soil between samples. To describe the effect of sample location, principal coordinates of neighbor matrices (PCNM) vectors were constructed based on the geographical distances among samples (Borcard and Legendre 2002). Space was then represented by those PCNM vectors that were significantly related to microbial community composition. Soil was characterized by the PCA axes that were constructed based on the matrix of soil chemistry data (C, N, P, Ca, Mg, K, pH and SOM) and soil moisture. The first three PCA axes, which explained 93% of the variability (i.e. PC1 62%, PC2 17% and PC3 14%), were used as the soil chemistry proxy. The plant community composition matrix was used to calculate the dissimilarity matrix based on the Sørensen index. The matrix was subsequently used to calculate PCA. The first four PCA axes, which explained 62% of the data variability (i.e. PC1 33%, PC2 14%, PC3 8% and PC4 7%), were used to represent the plant community composition in subsequent analysis.
Variation partitioning was used to separate the effects of major sources of variation (that is sample location in space, soil variables and plant community composition, further referred to as space, soil and plant community) on microbial communities. The constructed PCNM and PCA vectors described above were used as space, soil and plant community proxies in the partitioning analysis. Variation partitioning was performed using the varpart function of the package vegan (Oksanen et al. 2007). The varpart function partitions unbiased adjusted coefficients of determination (adjR2), as suggested by (Peres-Neto et al. 2006). Communities in shoots, roots and soil were analyzed separately. The significance of partial segments was tested using RDA with 999 permutations.
The effect of compartment and plant species richness on fungal and bacterial community structure was tested using PERMANOVA with 999 permutations and using the Adonis function in vegan. Adjusted R2 values were calculated based on the Adonis results to check for model quality.
RESULTS
Soil properties and vegetation
The soil was slightly acidic, with a pH between 5.4 and 6.8, and had relatively small variations in N content (0.6%–0.9%) and C/N ratio (6.6–11.0). However, plots differed considerably in the amount of available P, ranging from 2 to 20 ppm; thus, their C/P and N/P ratios also differed considerably. Root mass also differed substantially among plots, ranging from 0.4 to 4.0 kg/m−2 (Supplementary Table 1). According to the vegetation survey, plant species richness ranged from very low (three plots with 1–3 species) to high (up to 43 plant species). Interestingly, the vegetation composition was quite variable, and all of the plots with the lowest diversity hosted different plants. The grasses Festuca rubra, Bromus erectus and Brachypodium pinnatum were the most commonly occurring plant species (Supplementary Table 2).
Fungal diversity and community composition
In total, 487 500 sequence reads of fungal SSU rDNA were clustered into 12 480 OTUs. The average fungal OTU richness was 470 in shoots (ranging from 303 to 629), 448 in roots (ranging from 187 to 594) and 495 in soil (ranging from 312 to 650). Based on the 95% confidence intervals of the beta coefficients from the GLS models (i.e. 637 models for shoots and 1023 models for roots and soil), none of the tested environmental variables (i.e. soil chemistry: P, N, C/N, pH, Mg, K, Ca and N/P; and soil moisture, root biomass and plant species richness) affected fungal OTU richness in root or soil samples. In contrast, in shoot samples, fungal OTU richness showed consistent significant correlations with plant species richness. The relationship between the plant species and fungal OTU richness was positive and explained 28% of the variation in the data (Fig. 1a). Plant phylogenetic diversity showed similar trend but weaker correlation (see Supplementary Fig. 1). In addition to the plant species richness, none of the tested variables affected the fungal OTU richness in the shoots.
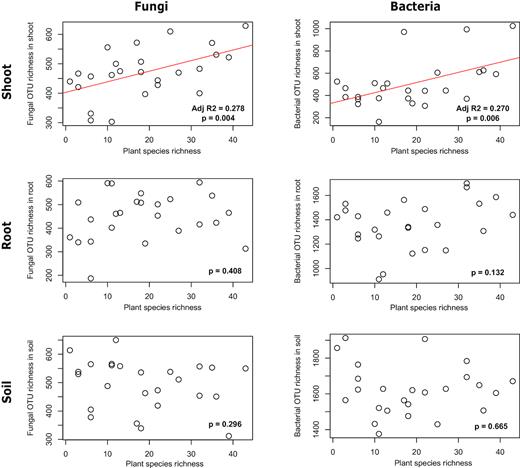
Correlations between fungal and bacterial OTU richness and plant species richness. The lines represent the significant linear regression models with the best fit.
Fungal sequences belonged mainly to Ascomycota (58%) and Basidiomycota (30%), and were followed by Mucoromycota (5%), Chytridiomycota (2%) and other fungal phyla (5%). The shoot compartment was predominantly colonized by the ascomycetous orders Hypocreales (13%), Capnodiales (13%) and Pleosporales (9%); in contrast, the basidiomycetous order Agaricales prevailed in the roots (32%) and soil (20%, Fig. 2).
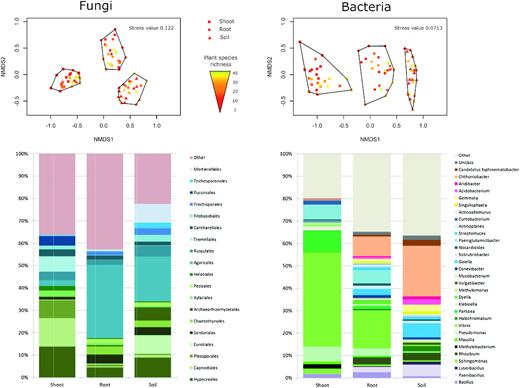
Differences in microbial community composition in shoots, roots and soil. Nonmetric multidimensional scaling (NMDS) diagrams show fungal and bacterial community compositions on a single-sample level. The effects of compartments on microbial communities were highly significant in PERMANOVA tests, explaining 34% and 50% of the variability in OTU composition for fungi and bacteria, respectively (adjR2 = 0.34, adjR2 = 0.50, P < 0.001 in both cases). Plant species richness had no significant effect on any of the studied compartments. Barcharts show relative abundances of the most abundant fungal orders and bacterial genera in each compartment.
NMDS ordination showed a clear separation of fungal communities among different compartments (shoots, roots and soil), but no clear pattern was detected for the effect of plant species richness on fungal communities (Fig. 2). Congruently, PERMANOVA revealed a high significance of the factor compartment (P < 0.001), which explained 34% (adjR2 = 0.34) of the variation in fungal community composition; however, plant species richness did not show any relationship with fungal community composition (P = 0.117).
The fungal communities in root and shoot compartments did not reveal any correlation with spatial distribution, and therefore, no PCNM vectors were used in the variation partitioning analysis of root and shoot associations with fungal communities. In contrast, one PCNM vector was significantly correlated with soil fungal communities and therefore it was included in the variation partitioning analysis of fungal communities in soil.
Variation partitioning analysis (Fig. 3) revealed that the plant community composition was the main determinant of the fungal community composition in the shoot compartment (adjR2 = 0.13; df = 4; P < 0.001). The effect of the plant community on the fungal community composition was smaller in root and soil compartments, and no significant effects were observed. However, the relative importance of soil chemistry showed an opposite trend. It was found that soil chemistry had only negligible effects on shoot- and root-associated fungal communities (2% each), though fungal communities in soil were more affected by soil chemistry (8%). The spatial distribution of the sampling plots had no effect on the fungal community composition in the plant shoots and roots, but spatial distribution significantly affected the community composition of soil fungi (adjR2 = 0.06; df = 1; P = 0.024).
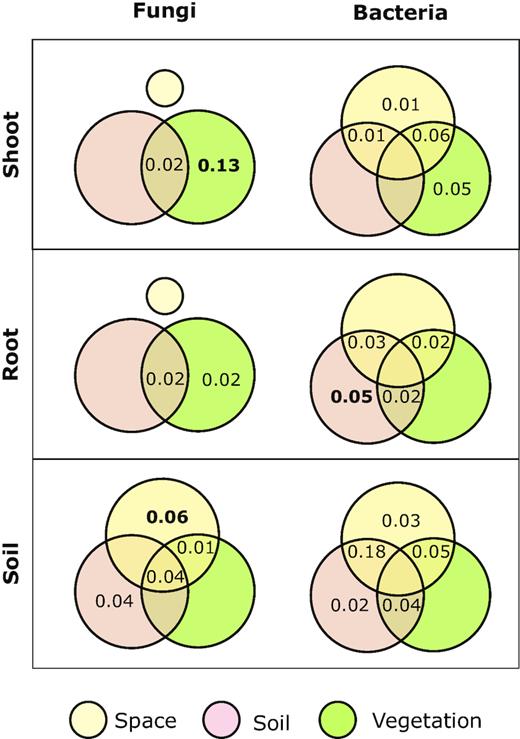
Variation partitioning of the effects of space, soil characteristics and plant species composition on the composition of grassland communities of fungi and bacteria. Values show the percentage of variation explained as adjusted R2 by each fraction in shoot, root and soil compartments. Values in bold were significant. Intersection areas present variability shared by two neighboring segments. No PCNM spatial vector was significant for the fungal community in shoot and root compartments; hence, it was excluded from the analysis.
Bacterial diversity and community composition
In total, 596 968 sequence reads of bacterial 16S rRNA were clustered into 19 423 OTUs. The average bacterial OTU richness was 504 in shoots (ranging from 163 to 1025 OTUs), 1361 in roots (ranging from 912 to 1699) and 1622 in soil (ranging from 1376 to 1913). As in the case of fungi, none of the tested environmental variables significantly affected bacterial OTU richness in root or soil samples, as was demonstrated by the 95% confidence intervals of the beta coefficients from the GLS models. In contrast, the bacterial OTU richness in shoots was significantly correlated with plant species richness. The relationship between plant species and bacterial OTU richness was positive and explained 27% of the variation in the data (Fig. 1b). As in case of fungi, plant phylogenetic diversity showed similar trend but weaker correlation than plant species richness (see Supplementary Fig. 1). In addition to plant species richness, none of the tested variables affected bacterial OTU richness in shoots.
Bacterial sequences belonged mainly to Proteobacteria (50%) and Actinobacteria (20%). In shoots, the most abundant bacterial genera were Vibrio, Pantoea and Pseudomonas, all of which belong to Gammaproteobacteria. In roots, the most abundant bacterial genera were Vibrio, Chthoniobacter (phylum Verrucomicrobia) and Paeniglutamicibacter (phylum Actinobacteria). In soil, the most abundant bacterial genera were Chthoniobacter, Gaiella (phylum Actinobacteria) and Paenibacillus (phylum Firmicutes; Fig. 2).
NMDS ordination showed a clear separation of bacterial communities among the different compartments, but no clear pattern was detected for the effect of plant species richness on bacterial communities (Fig. 2). In accordance, PERMANOVA revealed the factor compartments were highly significant (P < 0.001), explaining 50% of the variation in the bacterial community composition (adjR2 = 0.5); however, plant species richness did not show a significant relationship with bacterial community composition (P = 0.213).
Bacterial communities were more spatially structured than fungal communities. Two, one and four PCNM vectors showed significant correlations with shoot, root and soil bacterial communities, respectively. These vectors were subsequently used in the variation partitioning analysis of the bacterial communities.
Although variation partitioning analyses did not reveal significant effects of any of the tested factors, spatial distribution and plant community composition together explained 12% of variation in the bacterial community in the shoot compartment (Fig. 3). Soil parameters were significant predictors of bacterial community composition in roots, explaining 5% of the variation (adjR2 = 0.05; df = 3; P = 0.035). Neither the sole effect of space or plant community was significant; however, the combined effect of soil and space, together with the pure effect of soil chemistry, explained 9% of the variation in the root bacterial community. The variation partitioning analysis explained the high overall variation (32%) of the soil bacterial communities. Most of the explained variability (23%) was attributed to soil chemistry and spatial distribution.
DISCUSSION
Both bacterial and fungal communities were found to be distinct among the three studied compartments. However, for both bacteria and fungi, some overlap with shared microbial taxa was observed between soil and roots and between roots and shoots. This is in agreement with a previous observation on grassland plants (Wearn et al. 2012) and supports our assumptions of different sources of colonization for aboveground and belowground microbial communities and different drivers of community assembly. In accordance with the observations on the plant specificity of root microbiomes (Vandenkoornhuyse et al. 2003; Dean et al. 2015), roots appear to have a significant and species-specific selector of root-associated microbes (Ma et al. 2017).
To date, plant diversity has been the most frequently tested driver explaining the diversity of plant or soil microbiomes. Our study has demonstrated a significant positive correlation between the plant species richness and the richness of bacteria and fungi, but this correlation existed only for the shoot communities; no link was observed between plant and microbial diversity in roots and soil. This is in line with our hypothesis of the decreasing effect of plant diversity from shoots through roots to soil. Positive correlations were previously observed mainly between plant diversity and diversity of symbiotic mycorrhizal fungi (Meadow and Zabinski 2012; Hiiesalu et al. 2014). For other fungal guilds, like saprotrophs, the effect of plant litter type on community was observed (e.g. Chapman and Newman 2010), but is usually more connected to chemistry of litter than identity of plant species itself (e.g. Wu et al. 2011). Thus the observed plant-microbe diversity link in our study is most likely driven by symbiotic organisms, while other guilds of soil microorganisms, such as saprotrophs, might be less affected.
Positive correlations have also been occasionally observed between the plant and microbial diversity of soil fungi (Wang et al. 2016, 2017; Yang et al. 2017). However, in these reports, results are likely affected by the large sampling areas analyzed, since the response of plants and microbes to physicochemical parameters can be similar (Wang et al. 2016). Our results are comparable to those of Prober et al. (2015) and Dassen et al. (2017), showing that, at a local scale, plant alpha diversity patterns were poorly related to those observed for fungi, bacteria or archaea.
When the phylogenetic diversity of plants was used as predictor variable, it was poorer predictor of microbial species richness than plain plant species richness. This is in contrast to our expectations, as there has been evidence that closely related plant species harbor bacterial communities that are more similar (Redford et al. 2010).
For plant communities, soil chemistry appears to be an important predictor of species richness, which typically decreases as nutrient content increases (Stevens et al. 2004). Here we have not observed links between soil chemistry and microbial diversity, which may partly be due to the low variability in several of the soil chemistry variables within the small area we studied. However, a recent report about the link between soil chemistry and diversity of fungal root endophytes (David, Seabloom and May 2016), indicates that this question requires further attention.
This study tested the effects of plant community composition, soil chemistry and space on the composition of microbial communities. By itself, plant community composition was the main determinant of fungal community composition in shoots. For bacteria, the combination of plant community and space explained most of the observed variation, but none of the pure effects was significant. We did not find any observable effect of plant composition on bacteria in roots and soil. Although specificity of fungal endophytes was found in some forest trees (Balint et al. 2013), the extent of this specificity varies (Baldrian 2017). Interestingly, in tropical grasslands, host-plant specificity was not observed (Higgins et al. 2014). Contrary to our initial expectation and the results from forest ecosystems (Barberán et al. 2015; Urbanová, Šnajdr and Baldrian 2015), the plant community effect was not observed in soil. This may be because trees, as dominant organisms, affect soil chemistry (Šnajdr et al. 2013), and their influence is difficult to separate from the effects of chemistry. In a temperate grassland, plant effects were reported to be significant in summer, when plants were active, but not in spring and autumn (Regan et al. 2014), showing the importance of plant activity.
Here, soil chemistry was identified as an important driver of bacterial community composition in soil and roots. Pure or shared effects of chemistry explained 24% of the variation in soil and 10% of the variation in roots. These values were lower for fungi (8% and 2%, respectively). This is in agreement with a previous grassland study that supported our assumption that some bacteria may inhabit soil microhabitats that experience a limited influence of plants and, rather, were determined by nutrient content (Fierer 2017; Lladó, López-Mondéjar and Baldrian 2017). The fact that space was another significant driver for soil fungi, even at the small scale of this analysis, indicates the importance of local conditions, which should be considered independent from the plant cover.
There is an apparent discrepancy between the observations on the specific composition of root microbiomes of individual plant species and the inconsistent reports of the correlations between plant diversity and diversity of microbiomes in roots or soil—such as the absence of such correlations in this study. There may be multiple explanations for this discrepancy: (i) plant microbiomes may be host specific, but they may still share a considerable proportion of microbial taxa; obviously, not all relationships are one-on-one, as is apparent by the existence of mycelial networks that are shared by multiple plants (Baldrian 2017) and (ii) in natural communities, the contribution of individual plants is unequal, e.g. concerning the standing root biomass; while some plants are locally highly abundant and produce high biomass, other taxa may be present as tiny individuals. These and other factors should be considered in future research.
In addition to the plant community composition and species richness, there are obviously other important drivers of the microbiome composition that are compartment-specific. This study supports the idea that although bacteria and fungi share some common drivers of community composition, such as the plant community composition in shoots, other drivers are more specific for individual groups. For example, space appears to be an important driver of soil microbial community composition, affecting the composition of bacterial and fungal communities differently, both directly and indirectly. It is possible that the pool of soil microorganisms may reflect also local factors that were not measured in our study. This factor could theoretically have different effect on unicellular microbes, which are usually constrained to soil microhabitats and filamentous ones, which can span larger soil volume. This observation, however, requires additional research in the future.
ACKNOWLEDGEMENTS
We thank Zdeňka Lososová for providing us a version of the DaPhnE tree with additions of local species.
FUNDING
This work was supported by the Ministry of Education, Youth and Sports of the Czech Republic (LM2015055, LTT17022). P.K. was also supported by Charles University in Prague (NPUI LO1417, MSMT). M.C. and P.D. were supported by the Czech Science Foundation (14-36079G).
Conflicts of interest. None declared.