-
PDF
- Split View
-
Views
-
Cite
Cite
Filippo Crea, New level of evidence grading system for Guidelines, artificial intelligence, and adult congenital heart disease, European Heart Journal, Volume 46, Issue 20, 21 May 2025, Pages 1875–1878, https://doi.org/10.1093/eurheartj/ehaf298
- Share Icon Share
For the podcast associated with this article, please visit https://dbpia.nl.go.kr/eurheartj/pages/Podcasts.
Guidelines play an important role in fulfilling the mission of scientific societies. In the past 2 years, nine Guidelines have been published by the European Society of Cardiology (ESC).1–8 This Focus Issue opens with two Special Articles which revise the level of evidence (LOE) grading system for Guideline recommendations. The first Special Article is entitled ‘2024 Revision of the level of evidence grading system for ESC clinical practice guideline recommendations I: therapy and prevention’ by Peter Jüni from the University of Oxford, UK, and colleagues.9 The LOE grading system for ESC Clinical Practice Guidelines classifies the quality of the evidence supporting a recommendation. However, the current taxonomy does not fully consider the optimal study design necessary to establish evidence for such recommendations. Therefore, two separate taskforces of clinical and methodological experts were appointed by the Clinical Practice Guidelines Committee, with the first tasked with updating the LOE grading system for therapy and prevention, and the second responsible for developing an LOE grading system for diagnosis and prediction. The updated system for therapy and prevention presented here maintains the three-level grading structure but uses revised definitions. Level of evidence A represents conclusive evidence usually from ≥2 adequately powered randomized controlled trials (RCTs) free from major bias, with substantial evidence against the play of chance when combined in a meta-analysis (e.g. P < .005 for superiority). Additional criteria are specified to define substantial evidence against the play of chance in the case of non-inferiority, equivalence, and harm. Level of evidence B is now subdivided into B1 and B2. Level of evidence B1 represents suggestive evidence usually from ≥1 adequately powered RCT free from major bias, or a meta-analysis of such RCTs, with some evidence against the play of chance (e.g. P < .05 for superiority). Level of evidence B2 represents limited evidence from ≥2 adequately powered non-randomized studies with careful control of major sources of bias or from a meta-analysis of small, underpowered RCTs. Level of evidence C represents preliminary evidence from either non-randomized studies without careful control of major sources of bias, a single small, underpowered RCT, or expert consensus (Figure 1).
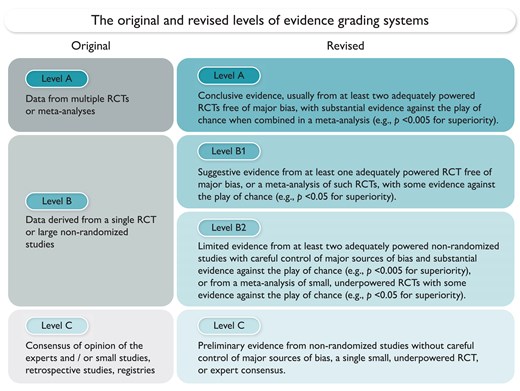
The original and revised level of evidence grading systems. RCT, randomized controlled trial.9
The second Special Article entitled ‘2024 Revision of the level of evidence grading system for ESC clinical practice guideline recommendations II: diagnostic tests and prediction models’ is by Emanuele Di Angelantonio from the University of Cambridge, UK, and colleagues.10 This report develops a new system for diagnostic tests and prediction models which maintains the three-level grading structure to classify the quality of the evidence but introduces new definitions specific for diagnosis and prediction. For diagnostic tests, LOE A represents conclusive evidence of adequate diagnostic ability from at least two high-quality studies. Level of evidence B represents suggestive evidence from one high-quality or at least two moderate-quality studies. Level of evidence C represents preliminary evidence not classified as A or B, including evidence from fewer than two moderate-quality studies, or from expert consensus. For prediction models, LOE A represents conclusive evidence of adequate predictive ability from at least one high-quality derivation and two or more external validation studies of at least moderate quality. Level of evidence B represents suggestive evidence in one or more derivation study and one or more external validation studies of at least moderate quality. Level of evidence C represents preliminary evidence not classified as A or B, including evidence from a derivation study of at least moderate quality, but with low quality or no external validation, or a derivation study of low quality.
The Issue continues with a focus on digital health and innovation and congenital heart disease. With the advent of artificial intelligence (AI), novel opportunities arise to revolutionize healthcare delivery and improve population health.11–16 In a State of the Art Review article entitled ‘Artificial intelligence to improve cardiovascular population health’, Benjamin Meder from the University of Heidelberg, Germany, and colleagues provides a state-of-the-art overview of recent advancements in AI technologies and their applications in enhancing cardiovascular health at the population level.17 From predictive analytics to personalized interventions, AI-driven approaches are increasingly being utilized to analyse vast amounts of healthcare data, uncover disease patterns, and optimize resource allocation. Furthermore, AI-enabled technologies such as wearable devices and remote monitoring systems facilitate continuous cardiac monitoring and early detection of diseases, and promise more timely interventions. Additionally, AI-powered systems aid healthcare professionals in clinical decision-making processes, thereby improving accuracy and treatment effectiveness. By using AI systems to augment existing data sources, such as registries and biobanks, completely new research questions can be addressed to identify novel mechanisms and pharmaceutical targets. Despite this remarkable potential of AI in enhancing population health, challenges related to legal issues, data privacy, algorithm bias, and ethical considerations must be addressed to ensure equitable access and improved outcomes for all individuals.
Emerging evidence supports AI-enhanced electrocardiograms (AI-ECGs) for detecting acute myocardial infarction (AMI), but real-world validation is needed.18 In a Clinical Research article entitled ‘Artificial intelligence applied to electrocardiogram to rule out acute myocardial infarction: the ROMIAE multicentre study’, Min Sung Lee from Sejong Hospital, Republic of Korea, and colleagues performed a study to evaluate the performance of AI-ECG in detecting AMI in the emergency department (ED). The Rule-Out acute Myocardial Infarction using Artificial intelligence Electrocardiogram analysis (ROMIAE) study is a prospective cohort study conducted in the Republic of Korea from March 2022 to October 2023, involving 18 university-level teaching hospitals. Adult patients presenting to the ED within 24 h of symptom onset with suspected AMI were assessed. Exposure included AI-ECG score, HEART score, GRACE 2.0 score, high-sensitivity troponin level, and Physician AMI score. The primary outcome was diagnosis of AMI during index admission, and the secondary outcome was 30 day major adverse cardiovascular events (MACE). The study population comprised 8493 adults, of whom 19% were diagnosed with AMI. The area under the receiver operating characteristic curve (AUC) for AI-ECG was 0.878, comparable with the HEART score (0.877) and superior to the GRACE 2.0 score, high-sensitivity troponin level, and Physician AMI score. For predicting 30 day MACE, AI-ECG (AUC 0.866) performed comparably with the HEART score (0.858). The integration of the AI-ECG improved risk stratification and AMI discrimination, with a net reclassification improvement of 20% and a C-index of 0.926, compared with the HEART score alone.
The authors conclude that in this multicentre prospective study, AI-ECG demonstrates diagnostic accuracy and predictive power for AMI and 30 day MACE, similar to or better than that of traditional risk stratification methods. The manuscript is accompanied by an Editorial by I-Min Chiu and David Ouyang from Cedars-Sinai Medical Center, Los Angeles, CA, USA.19 The authors point out that the ROMIAE study highlights AI-ECG as a promising innovation for managing AMI, but its full potential in clinical practice requires further evaluation and investigation. Randomized controlled trials are essential to evaluate the real-world impact of these advancements on patient outcomes and healthcare efficiency. Further refinement of AI-ECG algorithms is critical to addressing diagnostic challenges. Integrating AI-ECG with existing clinical scoring systems, such as the HEART score, could enhance its acceptance among clinicians by improving interpretability and trust. This approach leverages the strengths of AI while aligning it with established decision-making frameworks, ensuring a smoother integration into clinical workflows. Indeed, AI-ECG has the potential to transform cardiovascular diagnostics and care by improving workflows and enabling timely, effective interventions. However, successful implementation requires addressing key challenges, such as infrastructure demands, clinician training, and equitable access. By focusing on these areas and generating robust evidence to support its utility, AI-ECG can evolve into a transformative tool that not only enhances diagnostic precision but also advances precision medicine and equitable healthcare delivery.
Adults with congenital heart disease who underwent a Fontan palliation are at increased risk of premature death. In a Clinical Research article entitled ‘The Fontan Adult Brompton clinical score and mortality risk’, Claudia Montanaro from Imperial College London, UK, and colleagues aimed to identify a risk score based on widely available clinical variables at the first adult visit and facilitate personalized care.20 A single tertiary centre retrospective study included patients with a Fontan circulation during their first outpatient visit as an adult with congenital heart disease. Anatomic and peri-procedural data, clinical, laboratory, and echocardiographic parameters at the first visit, and mortality or heart transplantation data were examined. A stepwise backward conditional approach was used to build a multivariate prognostic model, validated in an external retrospective cohort. Overall, 230 patients (50% female), with a median age of 21.7 years, were included. Within 10.2 years of follow-up, 44 patients died and two received heart transplantation. A score including age at the first visit, history of atrial tachyarrhythmia, heart failure, New York Heart Association class, systolic blood pressure, and resting arterial oxygen saturation was derived, discriminating patients into low, intermediate, and high risk of death or transplantation (C-index: 0.71). Discriminative ability was excellent (C-index 0.92) when externally validated in a cohort of 80 patients (41% female, median age 18.4 years) (Figure 2).
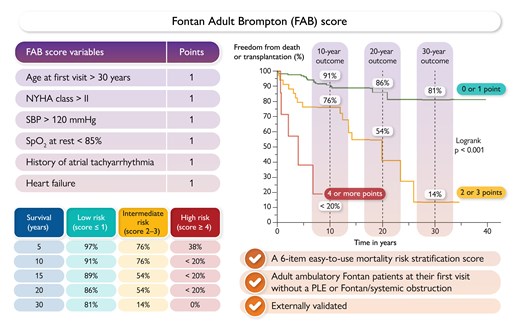
The Fontan Adult Brompton (FAB) score. NYHA, New York Heart Association; PLE, protein-losing enteropathy; SBP, systolic blood pressure; SpO2%, arterial oxygen saturation.20
The authors conclude that this novel, readily available, externally validated, clinical score identifies adult patients with a Fontan palliation at risk of mortality or transplantation and can be applied towards personalized care in this growing population with complex congenital heart disease.
In a Rapid Communication article entitled ‘Common and divergent cellular aetiologies underlying hypoplastic left heart syndrome and hypoplastic right heart syndrome’, Yang Yu from the Nationwide Children’s Hospital, Columbus, OH, USA, and colleagues, note that single ventricle heart defects (SVHDs) are the most severe forms of congenital heart defects (CHDs) and are classified based on the affected ventricles: hypoplastic left heart syndrome (HLHS) and hypoplastic right heart syndrome (HRHS).21 Cellular aetiologies underlying differential left and right ventricular hypoplasia are unknown and remain intractable without an experimental model to probe common and divergent cellular aetiologies between HLHS and HRHS. In this study, the authors leveraged patient-specific induced pluripotent stem cells (iPSCs) and single-cell transcriptomics to interrogate distinct aetiologies of HLHS and HRHS. Both HLHS and HRHS iPSC-derived cardiomyocytes (iPSC-CMs) exhibited a reduced proliferation capacity compared with sex-matched family controls under static and cyclic stretch conditions. Biological pathways related to cell cycle progression, DNA replication, and cell proliferation were down-regulated in both HLHS and HRHS iPSC-CMs, suggesting an intrinsic cardiac proliferation deficiency. Single-cell transcriptomics indicated that differentiation of cardiac mesoderm towards second heart field (SHF) progenitors was compromised in both HLHS and HRHS. However, differentiation of epicardial progenitors was enhanced at the expense of first heart field (FHF)/SHF progenitors in HLHS, whereas FHF progenitors were prevalent in HRHS. Trajectory inference analysis uncovers distinct cell lineage determination routes in FHF and SHF progenitors between HLHS and HRHS. Moreover, HLHS iPSC-CMs exhibit reduced mitochondrial activities, whereas HRHS iPSC-CMs show enhanced mitochondrial respiration and ATP production compared with controls.
The authors conclude that these common and divergent cellular aetiologies of HLHS and HRHS may underlie ventricular hypoplasia in the left and right side of the heart.
The issue is also complemented by a Discussion Forum. In a commentary entitled ‘Possible perspectives for cardiovascular events following coronavirus disease 2019 vaccination’, Xue-Yan Gong, James Cheng-Chung Wei, and Li-Yun Zhang from Tongji Shanxi Hospital, China and Chung Shan Medical University Hospital, China comment on the recent publication ‘Cardiovascular events following coronavirus disease 2019 vaccination in adults: a nationwide Swedish study’, by Yiyi Xu from the University of Gothenburg, Sweden, and colleagues.22
The editors hope that this issue of the European Heart Journal will be of interest to its readers.
Dr. Crea reports speaker fees from Abbott, Amgen, Astra Zeneca, BMS, Chiesi, Daiichi Sankyo, Menarini outside the submitted work.
With thanks to Lindsay Washington, Johanna Huggler, and Martin Meyer for help with compilation of this article.