-
PDF
- Split View
-
Views
-
Cite
Cite
Fangfang Zhou, Qun Luo, Lailiang Wang, Lina Han, Diagnostic value of neutrophil gelatinase-associated lipocalin for early diagnosis of cardiac surgery-associated acute kidney injury: a meta-analysis, European Journal of Cardio-Thoracic Surgery, Volume 49, Issue 3, March 2016, Pages 746–755, https://doi.org/10.1093/ejcts/ezv199
- Share Icon Share
Abstract
Neutrophil gelatinase-associated lipocalin (NGAL) has been identified as one of the most sensitive and specific biomarkers for predicting cardiac surgery-associated acute kidney injury (CSA-AKI); however, variable performance characteristics have been reported. We therefore performed a diagnostic meta-analysis to investigate the diagnostic accuracy of NGAL in early (within 12 h postoperatively) diagnosis of CSA-AKI using established guidelines. The search was carried out electronically with Medline (through PubMed interface), Embase, Cochrane library, ISI Web of Science, Scopus and ClinicalTrials.gov (up to 5 September 2014), and hand-searching was also done. Two reviewers conducted study inclusion, data extractions and quality assessment of the studies independently. The diagnostic capacity of NGAL for CSA-AKI was assessed using pooled sensitivity and specificity, diagnostic odds ratio (DOR), area under the summary receiver operating characteristic curve (AUC). Meta-Disc 1.4 and STATA 12.0 were used to investigate the source of heterogeneity and to perform the meta-analysis. Twenty-four studies (33 data sets of 4066 patients) were considered, which were all of good methodological quality. The overall pooled sensitivity of NGAL for the diagnosis of AKI was 0.68 [95% confidence interval (CI), 0.65–0.70], and specificity was 0.79 (95% CI, 0.77–0.80). The overall pooled positive likelihood ratio and negative likelihood ratio were 3.98 (95% CI, 3.05–5.20) and 0.33 (95% CI, 0.24–0.45), respectively, with a DOR of 13.05 (95% CI, 7.85–21.70). The receiver operating characteristic analysis showed an AUC [standard error (SE)] of 0.86 (0.02) and with a Q*-value (SE) of 0.79 (0.02). Subgroup analysis showed that NGAL had better predictive ability in neonates/children compared with adults (DOR, 19.37; AUC, 0.89 vs DOR, 8.98; AUC, 0.83), and adults without pre-existing renal insufficiency (PRI) had higher diagnostic value than adults without PRI to predict CSA-AKI (DOR, 15.43; AUC, 0.87 vs DOR, 6.56; AUC, 0.81). Both plasma/serum and urine NGAL had the highly predictive nature for early diagnosis of CSA-AKI (DOR, 13.09; AUC, 0.85 vs DOR, 13.20; AUC, 0.88), while lower DOR and AUC values were shown (DOR, 8.31; AUC, 0.81) when measured using standardized clinical platforms, compared with research-based assays (DOR, 19.22; AUC, 0.89). I2-values showed substantial heterogeneity, which mainly stems from characteristics of patient population (P = 0.037). NGAL diagnostic accuracy in early detection of CSA-AKI is high, especially in neonates/children or adults with normal baseline renal function.
INTRODUCTION
Despite improvements in perioperative health care, cardiac surgery-associated acute kidney injury (CSA-AKI) continues to be one of the most common aetiologies of hospital-acquired acute kidney injury (AKI). The incidence rate of CSA-AKI is reported to range from 3 to 30% (depending on the definition of AKI). And ∼1–5% of cardiac surgery cohorts require renal replacement therapy (RRT), which is associated with increased mortality, longer hospital length of stay and greater resource utilization [1–7].
Emerging data have revealed that even very small changes in serum creatinine (SCr) were associated with worse survival following cardiac surgery [8]. Early detection and intervention of CSA-AKI may improve outcomes. Although new definitions of AKI have been proposed recently, the diagnosis of AKI is still based on an increase in SCr and a decrease in urine output [9–11]. So far, these parameters have shown to be delayed and inadequate indicators of AKI. In the setting of cardiac surgery, SCr levels may be transiently decreased by less production when patients become hypothermic during cardiopulmonary bypass surgery or by perioperative haemodilution, and increase significantly only when substantial renal injury has already occurred, while urine output may be affected by the use of ultra-filtration or the administration of diuretics postoperatively. Thus, more reliable markers of postoperative kidney dysfunction are urgently needed.
Fortunately, the application of innovative technologies such as functional genomics and proteomics has accelerated the rate of novel biomarker discovery [12, 13]. Neutrophil gelatinase-associated lipocalin (NGAL) has been identified as one of the most sensitive and specific biomarkers for predicting AKI after cardiac surgery [14]. However, with accumulating evidence, a wide range of variability in NGAL's diagnostic performance for CSA-AKI has been reported [15–18]. We therefore conducted the meta-analysis to fully understand the diagnostic accuracy of NGAL as a predictive marker for early diagnosis of CSA-AKI.
METHODS
Data sources and search strategy
The search was carried out with Medline (through PubMed interface), Embase, Cochrane library, ISI Web of Science, Scopus and ClinicalTrials.gov (http://clinicaltrials.gov/) (up to 5 September 2014). The search terms used in Medline were: (((heart OR cardiac OR aort* OR valv* OR thoracic) AND surg*) OR ‘valve replacement*’ OR ‘bypass*’ OR ‘CABG’ OR ‘extracorporeal circulation’ OR ‘on pump’ OR ‘Cardiac Surgical Procedures’ [Mesh]) AND (‘neutrophil gelatinase-associated lipocalin’ OR NGAL OR ‘LCN2 protein, human’ [Supplementary Concept]). In Embase, Cochrane library, ISI Web of Science, Scopus and ClinicalTrials.gov, the same strategy was used. In addition, we searched the abstracts from meetings and reference lists of eligible papers or related reviews manually to identify additional relevant studies.
Study selection
The inclusion criteria used for this meta-analysis were as follows: (i) studies that evaluated NGAL in the early diagnosis of AKI after cardiac surgery (within 12 h after surgery); (ii) studies with mandatory data from which true-positive (TP), false-positive (FP), false-negative (FN) and true-negative (TN) could be found or calculated; and (iii) studies published in English. The exclusion criteria were as follows: (i) studies with duplicate data reported in other studies; (ii) studies that were review, animal research, letters, editorials, case reports or case series. Initially, two authors (Fangfang Zhou and Qun Luo) independently reviewed the titles and abstracts of all citations to judge study eligibility. For citations that could not be evaluated through the titles and abstracts, full texts were retrieved for detailed evaluation.
Data extraction and quality assessment
Two authors (Fangfang Zhou and Qun Luo) independently extracted data from all the eligible studies. The following information was collected using a standardized data extraction form: (i) basic characteristics of studies: name of the first author, year of publication, country of origin, study design, sample size, inclusion/exclusion criteria for enrolment and diagnosis criteria of CSA-AKI; (ii) characteristics of patient enrolled: mean/median age, number of male patients, baseline SCr level and number of patients who developed AKI; (iii) measurement of NGAL: specimen type, the measurement method and the timing of the measurement (within 12 h after surgery); (iv) outcomes of studies: TP/FP/FN/TN values which were mandatory. In addition, sensitivities, specificities; positive (PPV) and negative predictive values (NPV) [PPV = TP/(TP + FP); NPV = TN/(TN + FN)]; area under the receiver operating characteristic curve, and the optimal cut-off thresholds, which were optional. If a study lacked the mandatory data, we calculated the TP/FP/FN/TN according to the following formula: sensitive = TP/(TP + FN), specificity = TN/(FP + TN), AKI + non-AKI = TP + FP + TN + FN and filled in the 2 × 2 table. The sensitivity, specificity and number of AKI patients and non-AKI patients were obtained from the enrolled articles.
Subsequently, the two independent authors (Fangfang Zhou and Qun Luo) assessed the quality of the studies by the Quality Assessment of Diagnostic Accuracy Studies (QUADAS) tool [19, 20], a widely accepted and validated tool to evaluate the bias and variation for the quality assessment of diagnostic accuracy studies in systematic reviews. Fourteen items were included, each of which was scored as yes, no or unclear (yes = 1, no = 0, unclear = 0.5).
Any discrepancies that arose from study selection, data extraction and quality assessment were resolved by discussion to reach a final consensus.
Statistical analysis
The random-effects model (DerSimonian Laird methods) was conducted to calculate the summary sensitivities, specificities, positive and negative likelihood ratio (NLR) and DORs of the studies if heterogeneity existed; otherwise, the fixed-effect model (Mantel–Haenszel method) was chosen. The accuracy indexes were presented in the form of forest plots. Moreover, as the most appropriate representation of the diagnostic accuracy, the area under the summary receiver operating curves (AUC) and the Cochrane indices (Q*) were calculated [21]. An AUC ≥0.70 defines a useful risk predictor [22].
The inter-study heterogeneity was calculated by the χ2-based Q test and the inconsistency index I2. A significant Q test (P < 0.05) or I2 >50% indicated heterogeneity [23], which was investigated by strategies as follows: (i) to detect threshold effects, the summary receiver operating curve (SROC) was constructed to assess if the points in the plots had a curvilinear (shoulder arm) pattern or not. A typical ‘shoulder arm’ pattern would suggest the presence of the threshold effect. As a further assessment of the threshold effect, the relationship between sensitivity and specificity was evaluated by the Spearman correlation coefficient; (ii) to detect non-threshold effects, pre-planned subgroups were defined based on: specimen type (urine or plasma/serum), characteristics of the patient population (neonates/children, adults, adults with or without PRI) and the measurement method (research-based assay or standardized clinical laboratory platforms). Furthermore, a meta-regression was used according to the characteristics of the included studies.
Finally, we performed sensitivity analysis to examine the stability of our meta-analysis. Since publication bias is of concern for meta-analysis of diagnostic studies, we tested for the potential presence of this bias using Begg's funnel plots, Egger's linear regression test [24, 25] and the Trim and Fill method. Data analyses were performed using Meta-Disc [26] version 1.4 (Universidad Complutense, Madrid, Spain) and STATA version 12.0 (Stata Corporation, College Station, TX, USA). In every test, a two-sided P-value of <0.05 was considered statistically significant.
RESULT
Search results
The initial search yielded 1606 articles from variable databases. By excluding doubles, 762 articles remained. After screening of title and abstract, 716 articles were excluded. The remaining 46 articles were evaluated in detail. Finally, 24 studies were accepted according to the inclusive criteria. Screening of title and abstract of references and related articles did not result in additional relevant articles [15–18, 27–46]. A flow chart detailing the process of study identification and selection is shown in Fig. 1.
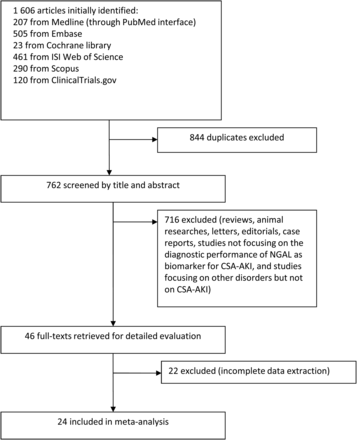
Flow chart of search results, with reasons for exclusion and the total number of studies included . NGAL: neutrophil gelatinase-associated lipocalin; CSA-AKI: cardiac surgery-associated acute kidney injury.
Study characteristics
Characteristics of the individual studies are listed in Table 1. There were 22 prospective cohort studies and 2 retrospective cohort studies adopted in our meta-analysis. The eligible studies were conducted in different continents, varying from North America, Europe, Asia and Australia, with the publication years ranging from 2005 to 2014. The sample sizes varied between 29 and 879 patients, including neonates, children and adults. A total of 4066 subjects were enrolled in these studies, of whom 1070 subjects (26.3%) were diagnosed as AKI after cardiac surgeries. Urine and plasma/serum NGALs were almost equally measured among the studies. Generally, commercial enzyme-linked immune sorbent assays (ELISAs) were more frequently used for measurement of NGAL; however, standardized clinical laboratory platforms such as the Triage kit, for plasma NGAL and the ARCHITECT platform for urine NGAL have been widely used since 2009.
Study (year) . | Country . | Design . | Population type . | Age (years) . | Males . | Baseline SCr (mg/dl) . | Sample size . | Patients with CSA-AKI . | Definition of CSA-AKI . |
---|---|---|---|---|---|---|---|---|---|
Mishra (2005) [27] | USA | PC | Children | 3.0 | 45 | 0.45 | 71 | 20 | SCr ≥50% increase from baseline |
Wagener (2006) [28] | USA | PC | Adults | 64.7 | 53 | 1.1 | 81 | 16 | A peak postoperative increase in SCr ≥50% compared with preoperative |
Dent (2007) [29] | USA | PC | Children | 4.2 | 63 | 0.5 | 123 | 45 | ≥50% increase in SCr from baseline |
Bennett (2008) [30] | USA | PC | Children | 4.0 | 105 | 0.39 | 196 | 99 | SCr ≥50% increase from baseline |
Koyner (2008) [15] | USA | PC | Adults | 61.3 | 51 | 1.25 | 72 | 18 | ≥25% increase in SCr from the preoperative baseline or the need for RRT within the first 3 postoperative days |
Wagener (2008) [16] | USA | PC | Adults | 63.2 | 282 | 1.08 | 426 | 85 | An increase in SCr level by ≥50% or ≥0.3 mg/dl (compared with preoperative values) during the first 48 h after surgery |
Haase-Fielitz (2009) [31] | Australia | PC | Adults | 69.5 | 61 | 1.04 | 100 | 23 | ≥50% increase in SCr from baseline to peak value within the first 5 postoperative days |
Haase (2009) [32] | Australia | PC | Adults | 65.6 | 61 | NR | 100 | 46 | AKIN criteria |
Tuladhar (2009) [33] | UK | PC | Adults | 66.7 | 35 | 1.1 | 50 | 9 | An increase in SCr in the postoperative period by more than 0.5 mg/dl from baseline |
Che (2010) [34] | China | RC | Adults | 59.5 | 20 | 0.8 | 29 | 14 | SCr ≥50% increase from baseline |
Tjörvi (2010) [36] | USA | RC | Adults | 65 | 705 | 1.1 | 879 | 75 | ≥50% increase from baseline in SCr during the first 4 postoperative days |
Prakhu (2010) [35] | India | PC | Adults | 54 | NR | NR | 30 | 8 | SCr ≥50% increase from baseline |
Heise (2011) [37] | Germany | PC | Adults | 69.5 | 35 | NR | 47 | 38 | AKIN criteria |
Krawczeski (2011) [38] | USA | PC | Neonates/children | 1.99 | 172 | 0.40 | 373 | 112 | Neonate: a ≥0.3 mg/dl absolute increase in SCr from baseline within 48 h of surgery Non-neonate: a ≥50% increase in SCr from preoperative baseline within 48 h of surgery |
Parikh (2011) [17] | USA | Multicentre PC | Children | 3.8 | 171 | NR | 311 | 53 | Receipt of acute dialysis during the entire hospital stay or a doubling in SCr from the baseline preoperative value |
Cantinotti (2012) [39] | Italy | PC | Neonates/children | 0.58 | 78 | 0.24 | 135 | 52 | An increase of ≥1.5-fold of SCr from preoperative baseline levels |
Ricci (2012) [40] | Italy | PC | Neonates/children | 0.37 | 88 | NR | 160 | 90 | pRIFLE criteria |
Doi (2013) [41] | Japan | PC | Adults | 68.8 | 92 | 0.97 | 146 | 53 | AKIN criteria |
Liu (2013) [42] | China | PC | Adults | 63 | 72 | 0.8 | 109 | 26 | An increase in SCr of ≥0.3 mg/dl or ≥50% of baseline |
Seitz (2013) [18] | Germany | PC | Children | 0.9 | 76 | 0.38 | 139 | 55 | pRIFLE criteria |
Zheng (2013) [43] | China | PC | Children | 0.72 | 39 | 0.37 | 58 | 29 | AKIN criteria |
Alcaraz (2014) [44] | Spain | PC | Children | 2.08 | 62 | 0.34 | 106 | 36 | ≥50% increase in SCr from preoperative baseline values |
Kidher (2014) [45] | UK | PC | Adults | 71 | 37 | 0.9 | 53 | 16 | RIFLE criteria |
Zeng (2014) [46] | China | PC | Adults | 52.2 | 109 | 1 | 199 | 37 | AKIN criteria |
Study (year) . | Country . | Design . | Population type . | Age (years) . | Males . | Baseline SCr (mg/dl) . | Sample size . | Patients with CSA-AKI . | Definition of CSA-AKI . |
---|---|---|---|---|---|---|---|---|---|
Mishra (2005) [27] | USA | PC | Children | 3.0 | 45 | 0.45 | 71 | 20 | SCr ≥50% increase from baseline |
Wagener (2006) [28] | USA | PC | Adults | 64.7 | 53 | 1.1 | 81 | 16 | A peak postoperative increase in SCr ≥50% compared with preoperative |
Dent (2007) [29] | USA | PC | Children | 4.2 | 63 | 0.5 | 123 | 45 | ≥50% increase in SCr from baseline |
Bennett (2008) [30] | USA | PC | Children | 4.0 | 105 | 0.39 | 196 | 99 | SCr ≥50% increase from baseline |
Koyner (2008) [15] | USA | PC | Adults | 61.3 | 51 | 1.25 | 72 | 18 | ≥25% increase in SCr from the preoperative baseline or the need for RRT within the first 3 postoperative days |
Wagener (2008) [16] | USA | PC | Adults | 63.2 | 282 | 1.08 | 426 | 85 | An increase in SCr level by ≥50% or ≥0.3 mg/dl (compared with preoperative values) during the first 48 h after surgery |
Haase-Fielitz (2009) [31] | Australia | PC | Adults | 69.5 | 61 | 1.04 | 100 | 23 | ≥50% increase in SCr from baseline to peak value within the first 5 postoperative days |
Haase (2009) [32] | Australia | PC | Adults | 65.6 | 61 | NR | 100 | 46 | AKIN criteria |
Tuladhar (2009) [33] | UK | PC | Adults | 66.7 | 35 | 1.1 | 50 | 9 | An increase in SCr in the postoperative period by more than 0.5 mg/dl from baseline |
Che (2010) [34] | China | RC | Adults | 59.5 | 20 | 0.8 | 29 | 14 | SCr ≥50% increase from baseline |
Tjörvi (2010) [36] | USA | RC | Adults | 65 | 705 | 1.1 | 879 | 75 | ≥50% increase from baseline in SCr during the first 4 postoperative days |
Prakhu (2010) [35] | India | PC | Adults | 54 | NR | NR | 30 | 8 | SCr ≥50% increase from baseline |
Heise (2011) [37] | Germany | PC | Adults | 69.5 | 35 | NR | 47 | 38 | AKIN criteria |
Krawczeski (2011) [38] | USA | PC | Neonates/children | 1.99 | 172 | 0.40 | 373 | 112 | Neonate: a ≥0.3 mg/dl absolute increase in SCr from baseline within 48 h of surgery Non-neonate: a ≥50% increase in SCr from preoperative baseline within 48 h of surgery |
Parikh (2011) [17] | USA | Multicentre PC | Children | 3.8 | 171 | NR | 311 | 53 | Receipt of acute dialysis during the entire hospital stay or a doubling in SCr from the baseline preoperative value |
Cantinotti (2012) [39] | Italy | PC | Neonates/children | 0.58 | 78 | 0.24 | 135 | 52 | An increase of ≥1.5-fold of SCr from preoperative baseline levels |
Ricci (2012) [40] | Italy | PC | Neonates/children | 0.37 | 88 | NR | 160 | 90 | pRIFLE criteria |
Doi (2013) [41] | Japan | PC | Adults | 68.8 | 92 | 0.97 | 146 | 53 | AKIN criteria |
Liu (2013) [42] | China | PC | Adults | 63 | 72 | 0.8 | 109 | 26 | An increase in SCr of ≥0.3 mg/dl or ≥50% of baseline |
Seitz (2013) [18] | Germany | PC | Children | 0.9 | 76 | 0.38 | 139 | 55 | pRIFLE criteria |
Zheng (2013) [43] | China | PC | Children | 0.72 | 39 | 0.37 | 58 | 29 | AKIN criteria |
Alcaraz (2014) [44] | Spain | PC | Children | 2.08 | 62 | 0.34 | 106 | 36 | ≥50% increase in SCr from preoperative baseline values |
Kidher (2014) [45] | UK | PC | Adults | 71 | 37 | 0.9 | 53 | 16 | RIFLE criteria |
Zeng (2014) [46] | China | PC | Adults | 52.2 | 109 | 1 | 199 | 37 | AKIN criteria |
PC: prospective cohort study; RC: retrospective cohort study; SCr: serum creatinine; CSA-AKI: cardiac surgery-associated acute kidney injury; NR: not reported; AKIN criteria: Acute Kidney Injury Network criteria, which was defined as an absolute increase in serum creatinine of 0.3 mg/dl (26.4 µmol/l) or more, a percentage increase in serum creatinine of 50% or more and/or urine output reduced <0.5 ml/kg/h for more than 6 h; RIFLE criteria: risk, injury, failure, lost, end-stage renal disease, in which AKI was defined as a rise in serum creatinine of greater than or equal to 50% from its baseline value and/or a fall in the glomerular filtration rate by greater than or equal to 25%, and/or urine output reduced <0.5 ml/kg/h for 6 h or more; pRIFLE, paediatric RIFLE: in which AKI was defined as ‘estimated glomerular filtration rate’ using the original Schwartz equation decrease by greater than or equal to 25% or 50–99% or serum creatinine rise from baseline within 7 days (1.50–1.99 × baseline), and/or urine output reduced <0.5 ml/kg/h for more than 8 h.
Study (year) . | Country . | Design . | Population type . | Age (years) . | Males . | Baseline SCr (mg/dl) . | Sample size . | Patients with CSA-AKI . | Definition of CSA-AKI . |
---|---|---|---|---|---|---|---|---|---|
Mishra (2005) [27] | USA | PC | Children | 3.0 | 45 | 0.45 | 71 | 20 | SCr ≥50% increase from baseline |
Wagener (2006) [28] | USA | PC | Adults | 64.7 | 53 | 1.1 | 81 | 16 | A peak postoperative increase in SCr ≥50% compared with preoperative |
Dent (2007) [29] | USA | PC | Children | 4.2 | 63 | 0.5 | 123 | 45 | ≥50% increase in SCr from baseline |
Bennett (2008) [30] | USA | PC | Children | 4.0 | 105 | 0.39 | 196 | 99 | SCr ≥50% increase from baseline |
Koyner (2008) [15] | USA | PC | Adults | 61.3 | 51 | 1.25 | 72 | 18 | ≥25% increase in SCr from the preoperative baseline or the need for RRT within the first 3 postoperative days |
Wagener (2008) [16] | USA | PC | Adults | 63.2 | 282 | 1.08 | 426 | 85 | An increase in SCr level by ≥50% or ≥0.3 mg/dl (compared with preoperative values) during the first 48 h after surgery |
Haase-Fielitz (2009) [31] | Australia | PC | Adults | 69.5 | 61 | 1.04 | 100 | 23 | ≥50% increase in SCr from baseline to peak value within the first 5 postoperative days |
Haase (2009) [32] | Australia | PC | Adults | 65.6 | 61 | NR | 100 | 46 | AKIN criteria |
Tuladhar (2009) [33] | UK | PC | Adults | 66.7 | 35 | 1.1 | 50 | 9 | An increase in SCr in the postoperative period by more than 0.5 mg/dl from baseline |
Che (2010) [34] | China | RC | Adults | 59.5 | 20 | 0.8 | 29 | 14 | SCr ≥50% increase from baseline |
Tjörvi (2010) [36] | USA | RC | Adults | 65 | 705 | 1.1 | 879 | 75 | ≥50% increase from baseline in SCr during the first 4 postoperative days |
Prakhu (2010) [35] | India | PC | Adults | 54 | NR | NR | 30 | 8 | SCr ≥50% increase from baseline |
Heise (2011) [37] | Germany | PC | Adults | 69.5 | 35 | NR | 47 | 38 | AKIN criteria |
Krawczeski (2011) [38] | USA | PC | Neonates/children | 1.99 | 172 | 0.40 | 373 | 112 | Neonate: a ≥0.3 mg/dl absolute increase in SCr from baseline within 48 h of surgery Non-neonate: a ≥50% increase in SCr from preoperative baseline within 48 h of surgery |
Parikh (2011) [17] | USA | Multicentre PC | Children | 3.8 | 171 | NR | 311 | 53 | Receipt of acute dialysis during the entire hospital stay or a doubling in SCr from the baseline preoperative value |
Cantinotti (2012) [39] | Italy | PC | Neonates/children | 0.58 | 78 | 0.24 | 135 | 52 | An increase of ≥1.5-fold of SCr from preoperative baseline levels |
Ricci (2012) [40] | Italy | PC | Neonates/children | 0.37 | 88 | NR | 160 | 90 | pRIFLE criteria |
Doi (2013) [41] | Japan | PC | Adults | 68.8 | 92 | 0.97 | 146 | 53 | AKIN criteria |
Liu (2013) [42] | China | PC | Adults | 63 | 72 | 0.8 | 109 | 26 | An increase in SCr of ≥0.3 mg/dl or ≥50% of baseline |
Seitz (2013) [18] | Germany | PC | Children | 0.9 | 76 | 0.38 | 139 | 55 | pRIFLE criteria |
Zheng (2013) [43] | China | PC | Children | 0.72 | 39 | 0.37 | 58 | 29 | AKIN criteria |
Alcaraz (2014) [44] | Spain | PC | Children | 2.08 | 62 | 0.34 | 106 | 36 | ≥50% increase in SCr from preoperative baseline values |
Kidher (2014) [45] | UK | PC | Adults | 71 | 37 | 0.9 | 53 | 16 | RIFLE criteria |
Zeng (2014) [46] | China | PC | Adults | 52.2 | 109 | 1 | 199 | 37 | AKIN criteria |
Study (year) . | Country . | Design . | Population type . | Age (years) . | Males . | Baseline SCr (mg/dl) . | Sample size . | Patients with CSA-AKI . | Definition of CSA-AKI . |
---|---|---|---|---|---|---|---|---|---|
Mishra (2005) [27] | USA | PC | Children | 3.0 | 45 | 0.45 | 71 | 20 | SCr ≥50% increase from baseline |
Wagener (2006) [28] | USA | PC | Adults | 64.7 | 53 | 1.1 | 81 | 16 | A peak postoperative increase in SCr ≥50% compared with preoperative |
Dent (2007) [29] | USA | PC | Children | 4.2 | 63 | 0.5 | 123 | 45 | ≥50% increase in SCr from baseline |
Bennett (2008) [30] | USA | PC | Children | 4.0 | 105 | 0.39 | 196 | 99 | SCr ≥50% increase from baseline |
Koyner (2008) [15] | USA | PC | Adults | 61.3 | 51 | 1.25 | 72 | 18 | ≥25% increase in SCr from the preoperative baseline or the need for RRT within the first 3 postoperative days |
Wagener (2008) [16] | USA | PC | Adults | 63.2 | 282 | 1.08 | 426 | 85 | An increase in SCr level by ≥50% or ≥0.3 mg/dl (compared with preoperative values) during the first 48 h after surgery |
Haase-Fielitz (2009) [31] | Australia | PC | Adults | 69.5 | 61 | 1.04 | 100 | 23 | ≥50% increase in SCr from baseline to peak value within the first 5 postoperative days |
Haase (2009) [32] | Australia | PC | Adults | 65.6 | 61 | NR | 100 | 46 | AKIN criteria |
Tuladhar (2009) [33] | UK | PC | Adults | 66.7 | 35 | 1.1 | 50 | 9 | An increase in SCr in the postoperative period by more than 0.5 mg/dl from baseline |
Che (2010) [34] | China | RC | Adults | 59.5 | 20 | 0.8 | 29 | 14 | SCr ≥50% increase from baseline |
Tjörvi (2010) [36] | USA | RC | Adults | 65 | 705 | 1.1 | 879 | 75 | ≥50% increase from baseline in SCr during the first 4 postoperative days |
Prakhu (2010) [35] | India | PC | Adults | 54 | NR | NR | 30 | 8 | SCr ≥50% increase from baseline |
Heise (2011) [37] | Germany | PC | Adults | 69.5 | 35 | NR | 47 | 38 | AKIN criteria |
Krawczeski (2011) [38] | USA | PC | Neonates/children | 1.99 | 172 | 0.40 | 373 | 112 | Neonate: a ≥0.3 mg/dl absolute increase in SCr from baseline within 48 h of surgery Non-neonate: a ≥50% increase in SCr from preoperative baseline within 48 h of surgery |
Parikh (2011) [17] | USA | Multicentre PC | Children | 3.8 | 171 | NR | 311 | 53 | Receipt of acute dialysis during the entire hospital stay or a doubling in SCr from the baseline preoperative value |
Cantinotti (2012) [39] | Italy | PC | Neonates/children | 0.58 | 78 | 0.24 | 135 | 52 | An increase of ≥1.5-fold of SCr from preoperative baseline levels |
Ricci (2012) [40] | Italy | PC | Neonates/children | 0.37 | 88 | NR | 160 | 90 | pRIFLE criteria |
Doi (2013) [41] | Japan | PC | Adults | 68.8 | 92 | 0.97 | 146 | 53 | AKIN criteria |
Liu (2013) [42] | China | PC | Adults | 63 | 72 | 0.8 | 109 | 26 | An increase in SCr of ≥0.3 mg/dl or ≥50% of baseline |
Seitz (2013) [18] | Germany | PC | Children | 0.9 | 76 | 0.38 | 139 | 55 | pRIFLE criteria |
Zheng (2013) [43] | China | PC | Children | 0.72 | 39 | 0.37 | 58 | 29 | AKIN criteria |
Alcaraz (2014) [44] | Spain | PC | Children | 2.08 | 62 | 0.34 | 106 | 36 | ≥50% increase in SCr from preoperative baseline values |
Kidher (2014) [45] | UK | PC | Adults | 71 | 37 | 0.9 | 53 | 16 | RIFLE criteria |
Zeng (2014) [46] | China | PC | Adults | 52.2 | 109 | 1 | 199 | 37 | AKIN criteria |
PC: prospective cohort study; RC: retrospective cohort study; SCr: serum creatinine; CSA-AKI: cardiac surgery-associated acute kidney injury; NR: not reported; AKIN criteria: Acute Kidney Injury Network criteria, which was defined as an absolute increase in serum creatinine of 0.3 mg/dl (26.4 µmol/l) or more, a percentage increase in serum creatinine of 50% or more and/or urine output reduced <0.5 ml/kg/h for more than 6 h; RIFLE criteria: risk, injury, failure, lost, end-stage renal disease, in which AKI was defined as a rise in serum creatinine of greater than or equal to 50% from its baseline value and/or a fall in the glomerular filtration rate by greater than or equal to 25%, and/or urine output reduced <0.5 ml/kg/h for 6 h or more; pRIFLE, paediatric RIFLE: in which AKI was defined as ‘estimated glomerular filtration rate’ using the original Schwartz equation decrease by greater than or equal to 25% or 50–99% or serum creatinine rise from baseline within 7 days (1.50–1.99 × baseline), and/or urine output reduced <0.5 ml/kg/h for more than 8 h.
Quality assessment
All studies had high QUADAS scores (≥10) (Supplementary Table 1). All of them clearly defined eligibility criteria and reasons for patient exclusion. The spectrum of patients was representative of patients who might receive the test in practice in all studies except one which had a too-strict exclusion criterion. None of the studies included health volunteers as a control. Variable diagnostic criteria, which, however, were made all based on elevations of SCr as the ‘gold standards’, were adopted in the individual studies. No patient underwent renal biopsy or scintigraphy for verification of AKI diagnosis. All studies showed acceptable delay (within days) between NGAL and SCr tests. However, among the 24 studies, 8 did not mention the detection range or the coefficient of variation for the measurement of NGAL [18, 34, 36, 37, 39, 41–43], and 6 did not give clear indication of the measurement of SCr [17, 33, 37, 40, 41, 45]. Blinding of investigators was documented in 13 studies [15, 17, 18, 27, 29, 30, 32, 34, 38, 39, 42, 44, 45] with this not known in 11 articles [16, 28, 31, 33, 35, 37, 40, 41, 43, 46]. About 75% of the studies reported unclearly on the uninterpretable results [15–17, 27–34, 36–38, 42, 43, 45, 46] (Fig. 2).
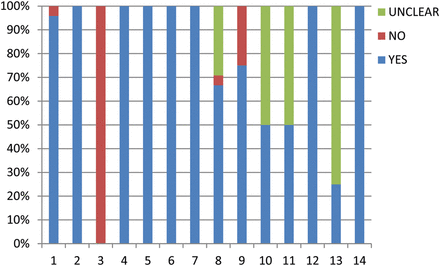
Quality assessment of the 24 enrolled studies using QUADAS-tool. QUADAS-tool: 1: spectrum composition; 2: selection criteria; 3: reference standard; 4: disease progression bias; 5: partial verification; 6: differential verification 7: incorporation bias; 8: index test execution; 9: reference standard execution; 10: test review bias; 11: reference standard review bias; 12: clinical review bias; 13: uninterruptible test results; 14: withdrawals.
Publication bias
The shape of the funnel plot of the pooled DOR of NGAL for the diagnosis of CSA-AKI showed symmetry (P = 0.134) (Fig. 3A), while the Egger's test showed a statistically significant value (P = 0.007), indicating that there was potential publication bias (Fig. 3B). Despite the existence of publication bias, analysis of the Trim and Fill method continued to show NGAL as a reliable early predictor of CSA-AKI (Supplementary Fig. 1).
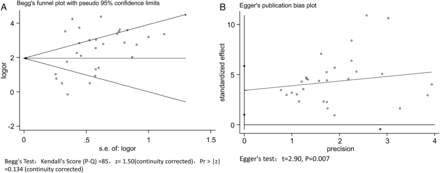
Begg's funnel chart (A) and Egger's regression chart (B) for publication bias analysis.
Data synthesis
A total of 33 sets of data from 24 eligible studies were extracted and are presented in Table 2, including TP/FP/FN/TN values; sensitivities; specificities; PPV; NPV; AUC; various optimal cut-off values for different sample types of NGAL; the measurement method and timing of measurement for the diagnosis of AKI.
Study (year) . | Sample type . | Measurement method . | TP . | FP . | FN . | TN . | Timing of measurement (h) . | NGAL cut-off . | Sensitivity (95% CI) . | Specificity (95% CI) . | PPV . | NPV . | AUC (95% CI) . |
---|---|---|---|---|---|---|---|---|---|---|---|---|---|
Mishra (2005) [27] | Urine | ELISAa | 20 | 1 | 0 | 50 | 2 | 50 ng/ml | 1.0 (0.8–1.0) | 0.98 (0.882–0.999) | 0.952 | 1 | 0.998 (NR) |
Mishra (2005) [27] | Serum | ELISAa | 14 | 3 | 6 | 48 | 2 | 25 ng/ml | 0.7 (0.457–0.872) | 0.941 (0.828–0.985) | 0.824 | 0.889 | 0.906 (NR) |
Wagener (2006) [28] | Urine | Immunoblot | 11 | 23 | 5 | 42 | 3 | 400 ng/ml | 0.688 (0.415–0.879) | 0.646 (0.517–0.758) | 0.324 | 0.857 | 0.737 (0.504–0.97) |
Dent (2007) [29] | Plasma | Triage Meter | 38 | 5 | 7 | 73 | 2 | 150 ng/ml | 0.844 (0.699–0.93) | 0.936 (0.85–0.976) | 0.884 | 0.913 | 0.96 (0.94–0.99) |
Bennett (2008) [30] | Urine | ELISAa | 78 | 8 | 21 | 89 | 2 | 100 ng/ml | 0.788 (0.692–0.861) | 0.918 (0.839–0.961) | 0.907 | 0.809 | 0.93 (NR) |
Koyner (2008) [15] | Urine | ELISAa | 12 | 19 | 6 | 35 | 0–6 | 550 ng/ml | 0.667 (0.412–0.856) | 0.648 (0.506–0.77) | 0.387 | 0.854 | 0.691 (0.567–0.815) |
Koyner (2008) [15] | Plasma | ELISAa | 8 | 13 | 10 | 41 | 0–6 | 280 ng/ml | 0.444 (0.224–0.687) | 0.759 (0.621–0.861) | 0.381 | 0.803 | 0.536 (0.399–0.672) |
Wagener (2008) [16] | Urine | ELISAa | 32 | 75 | 53 | 266 | 3 | 18.1 ng/ml | 0.377 (0.323–0.434) | 0.781 (0.669–0.869) | 0.3 | 0.834 | 0.603 (0.533–0.674) |
Haase-Fielitz (2009) [31] | Plasma | Triage Meter | 18 | 17 | 5 | 60 | 6 | 150 ng/ml | 0.783 (0.558–0.917) | 0.779 (0.668–0.863) | 0.514 | 0.923 | 0.80 (0.63–0.96) |
Haase-Fielitz (2009) [31] | Plasmab | Triage Meter | 11 | 5 | 4 | 53 | 6 | 150 ng/ml | 0.73 (NR) | 0.91 (NR) | 0.69 | 0.93 | 0.80 (0.58–0.99) |
Haase (2009) [32] | Plasma | Triage Meter | 34 | 14 | 12 | 40 | 0–6 | 150 ng/ml | 0.73 (NR) | 0.74 (NR) | 0.708 | 0.769 | 0.77 (0.63–0.91) |
Tuladhar (2009) [33] | Urine | ELISAa | 8 | 9 | 1 | 32 | 2 | 390 ng/ml | 0.889 (0.507–0.994) | 0.781 (0.62–0.889) | 0.47 | 0.97 | 0.96 (0.90–1.0) |
Tuladhar (2009) [33] | Plasma | ELISAa | 7 | 13 | 2 | 28 | 2 | 420 ng/ml | 0.778 (0.402–0.961) | 0.683 (0.518–0.814) | 0.35 | 0.93 | 0.85 (0.73–0.97) |
Che (2010) [34] | Urine | ELISAd | 12 | 3 | 2 | 12 | 0 | 49.15 ng/mg | 0.86 (NR) | 0.8 (NR) | 0.8 | 0.86 | 0.85 (0.70–1.00) |
Tjörvi (2010) [36] | Plasma | Triage Meter | 29 | 149 | 46 | 655 | 0 | 353.5 ng/ml | 0.387 (NR) | 0.815 (NR) | 0.163 | 0.934 | 0.641 (0.58–0.71) |
Prakhu (2010) [35] | Plasma | ELISAa | 8 | 2 | 0 | 20 | 4 | 229 ng/ml | 1.0 (NR) | 0.909 (NR) | 0.8 | 1 | 0.98 (0.95–1.0) |
Heise (2011) [37] | Urine | ELISAa | 31 | 2 | 7 | 7 | 0–6 | 16.8 ng/ml | 0.816 (NR) | 0.778 (NR) | 0.94 | 0.5 | 0.773 (0.628–0.882) |
Krawczeski (2011) [38] | Urine | ELISAa | 88 | 33 | 16 | 201 | 2 | 48 ng/ml | 0.85 (NR) | 0.86 (NR) | 0.73 | 0.93 | 0.92 (NR) |
Krawczeski (2011) [38] | Plasma | ELISAa | 94 | 28 | 10 | 206 | 2 | 45 ng/ml | 0.9 (NR) | 0.88 (NR) | 0.77 | 0.95 | 0.94 (NR) |
Krawczeski (2011) [38] | Urinec | ELISAa | 8 | 2 | 0 | 25 | 2 | 185 ng/ml | 1.0 (NR) | 0.93 (NR) | 0.8 | 1 | 0.95 (NR) |
Krawczeski (2011) [38] | Plasmac | ELISAa | 7 | 2 | 1 | 25 | 2 | 95 ng/ml | 0.88 (NR) | 0.93 (NR) | 0.78 | 0.96 | 0.95 (NR) |
Parikh (2011) [17] | Urine | ARCHITECT® assay | 40 | 90 | 13 | 168 | 0–6 | 17 ng/ml | 0.75 (NR) | 0.65 (NR) | 0.3 | 0.93 | 0.71 (NR) |
Parikh (2011) [17] | Plasma | Triage Meter | 31 | 119 | 22 | 139 | 0–6 | 154 ng/ml | 0.59 (NR) | 0.54 (NR) | 0.2 | 0.86 | 0.56 (NR) |
Cantinotti (2012) [39] | Urine | ARCHITECT® assay | 41 | 15 | 11 | 68 | 2 | 49.95 ng/ml | 0.78 (NR) | 0.82 (NR) | 0.73 | 0.86 | 0.85 (NR) |
Ricci (2012) [40] | Whole blood | Triage Meter | 12 | 6 | 78 | 64 | 0–1 | 150 ng/ml | 0.13 (NR) | 0.91 (NR) | 0.66 | 0.45 | NR |
Doi (2013) [41] | Plasma | Triage Meter | 13 | 6 | 7 | 52 | 0 | 102 ng/ml | 0.65 (NR) | 0.9 (NR) | 0.684 | 0.881 | 0.76 (0.60–0.87) |
Doi (2013) [41] | Plasmab | Triage Meter | 17 | 2 | 16 | 33 | 2 | 233 ng/ml | 0.52 (NR) | 0.94 (NR) | 0.895 | 0.673 | 0.75 (0.61–0.85) |
Liu (2013) [42] | Urine | ELISAd | 21 | 14 | 5 | 69 | 2 | 33.73 ng/mg | 0.808 | 0.831 | 0.6 | 0.932 | 0.871 (0.775–0.967) |
Seitz (2013) [18] | Urine | ARCHITECT® assay | 30 | 49 | 25 | 35 | 2 | 27.6 ng/ml | 0.545 (NR) | 0.42 (NR) | 0.38 | 0.58 | 0.56 (NR) |
Zheng (2013) [43] | Urine | ELISAd | 23 | 3 | 6 | 26 | 4 | 54 ng/ml | 0.793 (NR) | 0.897 (NR) | 0.885 | 0.812 | 0.857 (0.753–0.961) |
Alcaraz (2013) [44] | Urine | ARCHITECT® assay | 29 | 12 | 7 | 58 | 3 | 75 ng/ml | 0.79 | 0.83 | 0.68 | 0.9 | 0.86 (0.78–0.95) |
Kidher (2014) [45] | Plasma | Triage Meter | 13 | 4 | 3 | 33 | 3 | 150 ng/ml | 0.8 (NR) | 0.89 (NR) | 0.76 | 0.92 | 0.83 (0.7–0.95) |
Zeng (2014) [46] | Urine | ELISAd | 28 | 40 | 9 | 122 | 12 | 86 ng/mg | 0.757 (NR) | 0.753 (NR) | 0.412 | 0.931 | 0.83 (0.74–0.91) |
Study (year) . | Sample type . | Measurement method . | TP . | FP . | FN . | TN . | Timing of measurement (h) . | NGAL cut-off . | Sensitivity (95% CI) . | Specificity (95% CI) . | PPV . | NPV . | AUC (95% CI) . |
---|---|---|---|---|---|---|---|---|---|---|---|---|---|
Mishra (2005) [27] | Urine | ELISAa | 20 | 1 | 0 | 50 | 2 | 50 ng/ml | 1.0 (0.8–1.0) | 0.98 (0.882–0.999) | 0.952 | 1 | 0.998 (NR) |
Mishra (2005) [27] | Serum | ELISAa | 14 | 3 | 6 | 48 | 2 | 25 ng/ml | 0.7 (0.457–0.872) | 0.941 (0.828–0.985) | 0.824 | 0.889 | 0.906 (NR) |
Wagener (2006) [28] | Urine | Immunoblot | 11 | 23 | 5 | 42 | 3 | 400 ng/ml | 0.688 (0.415–0.879) | 0.646 (0.517–0.758) | 0.324 | 0.857 | 0.737 (0.504–0.97) |
Dent (2007) [29] | Plasma | Triage Meter | 38 | 5 | 7 | 73 | 2 | 150 ng/ml | 0.844 (0.699–0.93) | 0.936 (0.85–0.976) | 0.884 | 0.913 | 0.96 (0.94–0.99) |
Bennett (2008) [30] | Urine | ELISAa | 78 | 8 | 21 | 89 | 2 | 100 ng/ml | 0.788 (0.692–0.861) | 0.918 (0.839–0.961) | 0.907 | 0.809 | 0.93 (NR) |
Koyner (2008) [15] | Urine | ELISAa | 12 | 19 | 6 | 35 | 0–6 | 550 ng/ml | 0.667 (0.412–0.856) | 0.648 (0.506–0.77) | 0.387 | 0.854 | 0.691 (0.567–0.815) |
Koyner (2008) [15] | Plasma | ELISAa | 8 | 13 | 10 | 41 | 0–6 | 280 ng/ml | 0.444 (0.224–0.687) | 0.759 (0.621–0.861) | 0.381 | 0.803 | 0.536 (0.399–0.672) |
Wagener (2008) [16] | Urine | ELISAa | 32 | 75 | 53 | 266 | 3 | 18.1 ng/ml | 0.377 (0.323–0.434) | 0.781 (0.669–0.869) | 0.3 | 0.834 | 0.603 (0.533–0.674) |
Haase-Fielitz (2009) [31] | Plasma | Triage Meter | 18 | 17 | 5 | 60 | 6 | 150 ng/ml | 0.783 (0.558–0.917) | 0.779 (0.668–0.863) | 0.514 | 0.923 | 0.80 (0.63–0.96) |
Haase-Fielitz (2009) [31] | Plasmab | Triage Meter | 11 | 5 | 4 | 53 | 6 | 150 ng/ml | 0.73 (NR) | 0.91 (NR) | 0.69 | 0.93 | 0.80 (0.58–0.99) |
Haase (2009) [32] | Plasma | Triage Meter | 34 | 14 | 12 | 40 | 0–6 | 150 ng/ml | 0.73 (NR) | 0.74 (NR) | 0.708 | 0.769 | 0.77 (0.63–0.91) |
Tuladhar (2009) [33] | Urine | ELISAa | 8 | 9 | 1 | 32 | 2 | 390 ng/ml | 0.889 (0.507–0.994) | 0.781 (0.62–0.889) | 0.47 | 0.97 | 0.96 (0.90–1.0) |
Tuladhar (2009) [33] | Plasma | ELISAa | 7 | 13 | 2 | 28 | 2 | 420 ng/ml | 0.778 (0.402–0.961) | 0.683 (0.518–0.814) | 0.35 | 0.93 | 0.85 (0.73–0.97) |
Che (2010) [34] | Urine | ELISAd | 12 | 3 | 2 | 12 | 0 | 49.15 ng/mg | 0.86 (NR) | 0.8 (NR) | 0.8 | 0.86 | 0.85 (0.70–1.00) |
Tjörvi (2010) [36] | Plasma | Triage Meter | 29 | 149 | 46 | 655 | 0 | 353.5 ng/ml | 0.387 (NR) | 0.815 (NR) | 0.163 | 0.934 | 0.641 (0.58–0.71) |
Prakhu (2010) [35] | Plasma | ELISAa | 8 | 2 | 0 | 20 | 4 | 229 ng/ml | 1.0 (NR) | 0.909 (NR) | 0.8 | 1 | 0.98 (0.95–1.0) |
Heise (2011) [37] | Urine | ELISAa | 31 | 2 | 7 | 7 | 0–6 | 16.8 ng/ml | 0.816 (NR) | 0.778 (NR) | 0.94 | 0.5 | 0.773 (0.628–0.882) |
Krawczeski (2011) [38] | Urine | ELISAa | 88 | 33 | 16 | 201 | 2 | 48 ng/ml | 0.85 (NR) | 0.86 (NR) | 0.73 | 0.93 | 0.92 (NR) |
Krawczeski (2011) [38] | Plasma | ELISAa | 94 | 28 | 10 | 206 | 2 | 45 ng/ml | 0.9 (NR) | 0.88 (NR) | 0.77 | 0.95 | 0.94 (NR) |
Krawczeski (2011) [38] | Urinec | ELISAa | 8 | 2 | 0 | 25 | 2 | 185 ng/ml | 1.0 (NR) | 0.93 (NR) | 0.8 | 1 | 0.95 (NR) |
Krawczeski (2011) [38] | Plasmac | ELISAa | 7 | 2 | 1 | 25 | 2 | 95 ng/ml | 0.88 (NR) | 0.93 (NR) | 0.78 | 0.96 | 0.95 (NR) |
Parikh (2011) [17] | Urine | ARCHITECT® assay | 40 | 90 | 13 | 168 | 0–6 | 17 ng/ml | 0.75 (NR) | 0.65 (NR) | 0.3 | 0.93 | 0.71 (NR) |
Parikh (2011) [17] | Plasma | Triage Meter | 31 | 119 | 22 | 139 | 0–6 | 154 ng/ml | 0.59 (NR) | 0.54 (NR) | 0.2 | 0.86 | 0.56 (NR) |
Cantinotti (2012) [39] | Urine | ARCHITECT® assay | 41 | 15 | 11 | 68 | 2 | 49.95 ng/ml | 0.78 (NR) | 0.82 (NR) | 0.73 | 0.86 | 0.85 (NR) |
Ricci (2012) [40] | Whole blood | Triage Meter | 12 | 6 | 78 | 64 | 0–1 | 150 ng/ml | 0.13 (NR) | 0.91 (NR) | 0.66 | 0.45 | NR |
Doi (2013) [41] | Plasma | Triage Meter | 13 | 6 | 7 | 52 | 0 | 102 ng/ml | 0.65 (NR) | 0.9 (NR) | 0.684 | 0.881 | 0.76 (0.60–0.87) |
Doi (2013) [41] | Plasmab | Triage Meter | 17 | 2 | 16 | 33 | 2 | 233 ng/ml | 0.52 (NR) | 0.94 (NR) | 0.895 | 0.673 | 0.75 (0.61–0.85) |
Liu (2013) [42] | Urine | ELISAd | 21 | 14 | 5 | 69 | 2 | 33.73 ng/mg | 0.808 | 0.831 | 0.6 | 0.932 | 0.871 (0.775–0.967) |
Seitz (2013) [18] | Urine | ARCHITECT® assay | 30 | 49 | 25 | 35 | 2 | 27.6 ng/ml | 0.545 (NR) | 0.42 (NR) | 0.38 | 0.58 | 0.56 (NR) |
Zheng (2013) [43] | Urine | ELISAd | 23 | 3 | 6 | 26 | 4 | 54 ng/ml | 0.793 (NR) | 0.897 (NR) | 0.885 | 0.812 | 0.857 (0.753–0.961) |
Alcaraz (2013) [44] | Urine | ARCHITECT® assay | 29 | 12 | 7 | 58 | 3 | 75 ng/ml | 0.79 | 0.83 | 0.68 | 0.9 | 0.86 (0.78–0.95) |
Kidher (2014) [45] | Plasma | Triage Meter | 13 | 4 | 3 | 33 | 3 | 150 ng/ml | 0.8 (NR) | 0.89 (NR) | 0.76 | 0.92 | 0.83 (0.7–0.95) |
Zeng (2014) [46] | Urine | ELISAd | 28 | 40 | 9 | 122 | 12 | 86 ng/mg | 0.757 (NR) | 0.753 (NR) | 0.412 | 0.931 | 0.83 (0.74–0.91) |
FN: false negative; FP: false positive; TP: true positive; TN: true negative; NGAL: neutrophil gelatinase-associated lipocalin; CIs: confidence intervals; PPV: positive predictive value; NPV: negative predictive value; AUC: area under the receiver operating characteristic curve; ELISA: enzyme-linked immunosorbent assay; NR: not reported.
aBioporto, Gentofte, Denmark.
bPre-existing renal insufficiency.
cNeonates.
dR&D Systems, Minneapolis, MN; ARCHITECT® assay, Abbott Diagnostics, Abbott Park, IL; Triage Meter, Biosite, San Diego, CA, USA.
Study (year) . | Sample type . | Measurement method . | TP . | FP . | FN . | TN . | Timing of measurement (h) . | NGAL cut-off . | Sensitivity (95% CI) . | Specificity (95% CI) . | PPV . | NPV . | AUC (95% CI) . |
---|---|---|---|---|---|---|---|---|---|---|---|---|---|
Mishra (2005) [27] | Urine | ELISAa | 20 | 1 | 0 | 50 | 2 | 50 ng/ml | 1.0 (0.8–1.0) | 0.98 (0.882–0.999) | 0.952 | 1 | 0.998 (NR) |
Mishra (2005) [27] | Serum | ELISAa | 14 | 3 | 6 | 48 | 2 | 25 ng/ml | 0.7 (0.457–0.872) | 0.941 (0.828–0.985) | 0.824 | 0.889 | 0.906 (NR) |
Wagener (2006) [28] | Urine | Immunoblot | 11 | 23 | 5 | 42 | 3 | 400 ng/ml | 0.688 (0.415–0.879) | 0.646 (0.517–0.758) | 0.324 | 0.857 | 0.737 (0.504–0.97) |
Dent (2007) [29] | Plasma | Triage Meter | 38 | 5 | 7 | 73 | 2 | 150 ng/ml | 0.844 (0.699–0.93) | 0.936 (0.85–0.976) | 0.884 | 0.913 | 0.96 (0.94–0.99) |
Bennett (2008) [30] | Urine | ELISAa | 78 | 8 | 21 | 89 | 2 | 100 ng/ml | 0.788 (0.692–0.861) | 0.918 (0.839–0.961) | 0.907 | 0.809 | 0.93 (NR) |
Koyner (2008) [15] | Urine | ELISAa | 12 | 19 | 6 | 35 | 0–6 | 550 ng/ml | 0.667 (0.412–0.856) | 0.648 (0.506–0.77) | 0.387 | 0.854 | 0.691 (0.567–0.815) |
Koyner (2008) [15] | Plasma | ELISAa | 8 | 13 | 10 | 41 | 0–6 | 280 ng/ml | 0.444 (0.224–0.687) | 0.759 (0.621–0.861) | 0.381 | 0.803 | 0.536 (0.399–0.672) |
Wagener (2008) [16] | Urine | ELISAa | 32 | 75 | 53 | 266 | 3 | 18.1 ng/ml | 0.377 (0.323–0.434) | 0.781 (0.669–0.869) | 0.3 | 0.834 | 0.603 (0.533–0.674) |
Haase-Fielitz (2009) [31] | Plasma | Triage Meter | 18 | 17 | 5 | 60 | 6 | 150 ng/ml | 0.783 (0.558–0.917) | 0.779 (0.668–0.863) | 0.514 | 0.923 | 0.80 (0.63–0.96) |
Haase-Fielitz (2009) [31] | Plasmab | Triage Meter | 11 | 5 | 4 | 53 | 6 | 150 ng/ml | 0.73 (NR) | 0.91 (NR) | 0.69 | 0.93 | 0.80 (0.58–0.99) |
Haase (2009) [32] | Plasma | Triage Meter | 34 | 14 | 12 | 40 | 0–6 | 150 ng/ml | 0.73 (NR) | 0.74 (NR) | 0.708 | 0.769 | 0.77 (0.63–0.91) |
Tuladhar (2009) [33] | Urine | ELISAa | 8 | 9 | 1 | 32 | 2 | 390 ng/ml | 0.889 (0.507–0.994) | 0.781 (0.62–0.889) | 0.47 | 0.97 | 0.96 (0.90–1.0) |
Tuladhar (2009) [33] | Plasma | ELISAa | 7 | 13 | 2 | 28 | 2 | 420 ng/ml | 0.778 (0.402–0.961) | 0.683 (0.518–0.814) | 0.35 | 0.93 | 0.85 (0.73–0.97) |
Che (2010) [34] | Urine | ELISAd | 12 | 3 | 2 | 12 | 0 | 49.15 ng/mg | 0.86 (NR) | 0.8 (NR) | 0.8 | 0.86 | 0.85 (0.70–1.00) |
Tjörvi (2010) [36] | Plasma | Triage Meter | 29 | 149 | 46 | 655 | 0 | 353.5 ng/ml | 0.387 (NR) | 0.815 (NR) | 0.163 | 0.934 | 0.641 (0.58–0.71) |
Prakhu (2010) [35] | Plasma | ELISAa | 8 | 2 | 0 | 20 | 4 | 229 ng/ml | 1.0 (NR) | 0.909 (NR) | 0.8 | 1 | 0.98 (0.95–1.0) |
Heise (2011) [37] | Urine | ELISAa | 31 | 2 | 7 | 7 | 0–6 | 16.8 ng/ml | 0.816 (NR) | 0.778 (NR) | 0.94 | 0.5 | 0.773 (0.628–0.882) |
Krawczeski (2011) [38] | Urine | ELISAa | 88 | 33 | 16 | 201 | 2 | 48 ng/ml | 0.85 (NR) | 0.86 (NR) | 0.73 | 0.93 | 0.92 (NR) |
Krawczeski (2011) [38] | Plasma | ELISAa | 94 | 28 | 10 | 206 | 2 | 45 ng/ml | 0.9 (NR) | 0.88 (NR) | 0.77 | 0.95 | 0.94 (NR) |
Krawczeski (2011) [38] | Urinec | ELISAa | 8 | 2 | 0 | 25 | 2 | 185 ng/ml | 1.0 (NR) | 0.93 (NR) | 0.8 | 1 | 0.95 (NR) |
Krawczeski (2011) [38] | Plasmac | ELISAa | 7 | 2 | 1 | 25 | 2 | 95 ng/ml | 0.88 (NR) | 0.93 (NR) | 0.78 | 0.96 | 0.95 (NR) |
Parikh (2011) [17] | Urine | ARCHITECT® assay | 40 | 90 | 13 | 168 | 0–6 | 17 ng/ml | 0.75 (NR) | 0.65 (NR) | 0.3 | 0.93 | 0.71 (NR) |
Parikh (2011) [17] | Plasma | Triage Meter | 31 | 119 | 22 | 139 | 0–6 | 154 ng/ml | 0.59 (NR) | 0.54 (NR) | 0.2 | 0.86 | 0.56 (NR) |
Cantinotti (2012) [39] | Urine | ARCHITECT® assay | 41 | 15 | 11 | 68 | 2 | 49.95 ng/ml | 0.78 (NR) | 0.82 (NR) | 0.73 | 0.86 | 0.85 (NR) |
Ricci (2012) [40] | Whole blood | Triage Meter | 12 | 6 | 78 | 64 | 0–1 | 150 ng/ml | 0.13 (NR) | 0.91 (NR) | 0.66 | 0.45 | NR |
Doi (2013) [41] | Plasma | Triage Meter | 13 | 6 | 7 | 52 | 0 | 102 ng/ml | 0.65 (NR) | 0.9 (NR) | 0.684 | 0.881 | 0.76 (0.60–0.87) |
Doi (2013) [41] | Plasmab | Triage Meter | 17 | 2 | 16 | 33 | 2 | 233 ng/ml | 0.52 (NR) | 0.94 (NR) | 0.895 | 0.673 | 0.75 (0.61–0.85) |
Liu (2013) [42] | Urine | ELISAd | 21 | 14 | 5 | 69 | 2 | 33.73 ng/mg | 0.808 | 0.831 | 0.6 | 0.932 | 0.871 (0.775–0.967) |
Seitz (2013) [18] | Urine | ARCHITECT® assay | 30 | 49 | 25 | 35 | 2 | 27.6 ng/ml | 0.545 (NR) | 0.42 (NR) | 0.38 | 0.58 | 0.56 (NR) |
Zheng (2013) [43] | Urine | ELISAd | 23 | 3 | 6 | 26 | 4 | 54 ng/ml | 0.793 (NR) | 0.897 (NR) | 0.885 | 0.812 | 0.857 (0.753–0.961) |
Alcaraz (2013) [44] | Urine | ARCHITECT® assay | 29 | 12 | 7 | 58 | 3 | 75 ng/ml | 0.79 | 0.83 | 0.68 | 0.9 | 0.86 (0.78–0.95) |
Kidher (2014) [45] | Plasma | Triage Meter | 13 | 4 | 3 | 33 | 3 | 150 ng/ml | 0.8 (NR) | 0.89 (NR) | 0.76 | 0.92 | 0.83 (0.7–0.95) |
Zeng (2014) [46] | Urine | ELISAd | 28 | 40 | 9 | 122 | 12 | 86 ng/mg | 0.757 (NR) | 0.753 (NR) | 0.412 | 0.931 | 0.83 (0.74–0.91) |
Study (year) . | Sample type . | Measurement method . | TP . | FP . | FN . | TN . | Timing of measurement (h) . | NGAL cut-off . | Sensitivity (95% CI) . | Specificity (95% CI) . | PPV . | NPV . | AUC (95% CI) . |
---|---|---|---|---|---|---|---|---|---|---|---|---|---|
Mishra (2005) [27] | Urine | ELISAa | 20 | 1 | 0 | 50 | 2 | 50 ng/ml | 1.0 (0.8–1.0) | 0.98 (0.882–0.999) | 0.952 | 1 | 0.998 (NR) |
Mishra (2005) [27] | Serum | ELISAa | 14 | 3 | 6 | 48 | 2 | 25 ng/ml | 0.7 (0.457–0.872) | 0.941 (0.828–0.985) | 0.824 | 0.889 | 0.906 (NR) |
Wagener (2006) [28] | Urine | Immunoblot | 11 | 23 | 5 | 42 | 3 | 400 ng/ml | 0.688 (0.415–0.879) | 0.646 (0.517–0.758) | 0.324 | 0.857 | 0.737 (0.504–0.97) |
Dent (2007) [29] | Plasma | Triage Meter | 38 | 5 | 7 | 73 | 2 | 150 ng/ml | 0.844 (0.699–0.93) | 0.936 (0.85–0.976) | 0.884 | 0.913 | 0.96 (0.94–0.99) |
Bennett (2008) [30] | Urine | ELISAa | 78 | 8 | 21 | 89 | 2 | 100 ng/ml | 0.788 (0.692–0.861) | 0.918 (0.839–0.961) | 0.907 | 0.809 | 0.93 (NR) |
Koyner (2008) [15] | Urine | ELISAa | 12 | 19 | 6 | 35 | 0–6 | 550 ng/ml | 0.667 (0.412–0.856) | 0.648 (0.506–0.77) | 0.387 | 0.854 | 0.691 (0.567–0.815) |
Koyner (2008) [15] | Plasma | ELISAa | 8 | 13 | 10 | 41 | 0–6 | 280 ng/ml | 0.444 (0.224–0.687) | 0.759 (0.621–0.861) | 0.381 | 0.803 | 0.536 (0.399–0.672) |
Wagener (2008) [16] | Urine | ELISAa | 32 | 75 | 53 | 266 | 3 | 18.1 ng/ml | 0.377 (0.323–0.434) | 0.781 (0.669–0.869) | 0.3 | 0.834 | 0.603 (0.533–0.674) |
Haase-Fielitz (2009) [31] | Plasma | Triage Meter | 18 | 17 | 5 | 60 | 6 | 150 ng/ml | 0.783 (0.558–0.917) | 0.779 (0.668–0.863) | 0.514 | 0.923 | 0.80 (0.63–0.96) |
Haase-Fielitz (2009) [31] | Plasmab | Triage Meter | 11 | 5 | 4 | 53 | 6 | 150 ng/ml | 0.73 (NR) | 0.91 (NR) | 0.69 | 0.93 | 0.80 (0.58–0.99) |
Haase (2009) [32] | Plasma | Triage Meter | 34 | 14 | 12 | 40 | 0–6 | 150 ng/ml | 0.73 (NR) | 0.74 (NR) | 0.708 | 0.769 | 0.77 (0.63–0.91) |
Tuladhar (2009) [33] | Urine | ELISAa | 8 | 9 | 1 | 32 | 2 | 390 ng/ml | 0.889 (0.507–0.994) | 0.781 (0.62–0.889) | 0.47 | 0.97 | 0.96 (0.90–1.0) |
Tuladhar (2009) [33] | Plasma | ELISAa | 7 | 13 | 2 | 28 | 2 | 420 ng/ml | 0.778 (0.402–0.961) | 0.683 (0.518–0.814) | 0.35 | 0.93 | 0.85 (0.73–0.97) |
Che (2010) [34] | Urine | ELISAd | 12 | 3 | 2 | 12 | 0 | 49.15 ng/mg | 0.86 (NR) | 0.8 (NR) | 0.8 | 0.86 | 0.85 (0.70–1.00) |
Tjörvi (2010) [36] | Plasma | Triage Meter | 29 | 149 | 46 | 655 | 0 | 353.5 ng/ml | 0.387 (NR) | 0.815 (NR) | 0.163 | 0.934 | 0.641 (0.58–0.71) |
Prakhu (2010) [35] | Plasma | ELISAa | 8 | 2 | 0 | 20 | 4 | 229 ng/ml | 1.0 (NR) | 0.909 (NR) | 0.8 | 1 | 0.98 (0.95–1.0) |
Heise (2011) [37] | Urine | ELISAa | 31 | 2 | 7 | 7 | 0–6 | 16.8 ng/ml | 0.816 (NR) | 0.778 (NR) | 0.94 | 0.5 | 0.773 (0.628–0.882) |
Krawczeski (2011) [38] | Urine | ELISAa | 88 | 33 | 16 | 201 | 2 | 48 ng/ml | 0.85 (NR) | 0.86 (NR) | 0.73 | 0.93 | 0.92 (NR) |
Krawczeski (2011) [38] | Plasma | ELISAa | 94 | 28 | 10 | 206 | 2 | 45 ng/ml | 0.9 (NR) | 0.88 (NR) | 0.77 | 0.95 | 0.94 (NR) |
Krawczeski (2011) [38] | Urinec | ELISAa | 8 | 2 | 0 | 25 | 2 | 185 ng/ml | 1.0 (NR) | 0.93 (NR) | 0.8 | 1 | 0.95 (NR) |
Krawczeski (2011) [38] | Plasmac | ELISAa | 7 | 2 | 1 | 25 | 2 | 95 ng/ml | 0.88 (NR) | 0.93 (NR) | 0.78 | 0.96 | 0.95 (NR) |
Parikh (2011) [17] | Urine | ARCHITECT® assay | 40 | 90 | 13 | 168 | 0–6 | 17 ng/ml | 0.75 (NR) | 0.65 (NR) | 0.3 | 0.93 | 0.71 (NR) |
Parikh (2011) [17] | Plasma | Triage Meter | 31 | 119 | 22 | 139 | 0–6 | 154 ng/ml | 0.59 (NR) | 0.54 (NR) | 0.2 | 0.86 | 0.56 (NR) |
Cantinotti (2012) [39] | Urine | ARCHITECT® assay | 41 | 15 | 11 | 68 | 2 | 49.95 ng/ml | 0.78 (NR) | 0.82 (NR) | 0.73 | 0.86 | 0.85 (NR) |
Ricci (2012) [40] | Whole blood | Triage Meter | 12 | 6 | 78 | 64 | 0–1 | 150 ng/ml | 0.13 (NR) | 0.91 (NR) | 0.66 | 0.45 | NR |
Doi (2013) [41] | Plasma | Triage Meter | 13 | 6 | 7 | 52 | 0 | 102 ng/ml | 0.65 (NR) | 0.9 (NR) | 0.684 | 0.881 | 0.76 (0.60–0.87) |
Doi (2013) [41] | Plasmab | Triage Meter | 17 | 2 | 16 | 33 | 2 | 233 ng/ml | 0.52 (NR) | 0.94 (NR) | 0.895 | 0.673 | 0.75 (0.61–0.85) |
Liu (2013) [42] | Urine | ELISAd | 21 | 14 | 5 | 69 | 2 | 33.73 ng/mg | 0.808 | 0.831 | 0.6 | 0.932 | 0.871 (0.775–0.967) |
Seitz (2013) [18] | Urine | ARCHITECT® assay | 30 | 49 | 25 | 35 | 2 | 27.6 ng/ml | 0.545 (NR) | 0.42 (NR) | 0.38 | 0.58 | 0.56 (NR) |
Zheng (2013) [43] | Urine | ELISAd | 23 | 3 | 6 | 26 | 4 | 54 ng/ml | 0.793 (NR) | 0.897 (NR) | 0.885 | 0.812 | 0.857 (0.753–0.961) |
Alcaraz (2013) [44] | Urine | ARCHITECT® assay | 29 | 12 | 7 | 58 | 3 | 75 ng/ml | 0.79 | 0.83 | 0.68 | 0.9 | 0.86 (0.78–0.95) |
Kidher (2014) [45] | Plasma | Triage Meter | 13 | 4 | 3 | 33 | 3 | 150 ng/ml | 0.8 (NR) | 0.89 (NR) | 0.76 | 0.92 | 0.83 (0.7–0.95) |
Zeng (2014) [46] | Urine | ELISAd | 28 | 40 | 9 | 122 | 12 | 86 ng/mg | 0.757 (NR) | 0.753 (NR) | 0.412 | 0.931 | 0.83 (0.74–0.91) |
FN: false negative; FP: false positive; TP: true positive; TN: true negative; NGAL: neutrophil gelatinase-associated lipocalin; CIs: confidence intervals; PPV: positive predictive value; NPV: negative predictive value; AUC: area under the receiver operating characteristic curve; ELISA: enzyme-linked immunosorbent assay; NR: not reported.
aBioporto, Gentofte, Denmark.
bPre-existing renal insufficiency.
cNeonates.
dR&D Systems, Minneapolis, MN; ARCHITECT® assay, Abbott Diagnostics, Abbott Park, IL; Triage Meter, Biosite, San Diego, CA, USA.
Heterogeneity was detected in all pooled indices, identified with the following results: the pooled sensitivity (χ2 = 301.22, I2 = 89.4%, P < 0.0001), specificity (χ2 = 296.72, I2 = 89.2%, P < 0.0001), positive likelihood ratio (PLR) (χ2 = 272.00, I2 = 88.2%, P < 0.0001), NLR (χ2 = 461.79, I2 = 93.1%, P < 0.0001) and DOR (χ2 = 252.73, I2 = 87.3%, P < 0.0001). All the results indicated a significant heterogeneity between studies. Therefore, the random-effects model was used for calculating pooled sensitivity, specificity, PLR, NLR and DOR in the meta-analysis.
For all data sets, the overall pooled sensitivity of NGAL for the diagnosis of AKI was 0.68 (95% CI, 0.65–0.70), and specificity was 0.79 (95% CI, 0.77–0.80). The overall pooled PLR and NLR were 3.98 (95% CI, 3.05–5.20) and 0.33 (95% CI, 0.24–0.45), respectively, with a DOR of 13.05 (95% CI, 7.85–21.70) (Fig. 4 and Supplementary Fig. 2). The graph of the SROC curve for NGAL showing sensitivity versus 1-specificity from individual studies is shown in Fig. 5. It depicted an AUC (SE) of 0.86 (0.02) and with a Q*-value (SE) of 0.79 (0.02), suggesting that the efficiency of NGAL for AKI diagnosis was considerable.
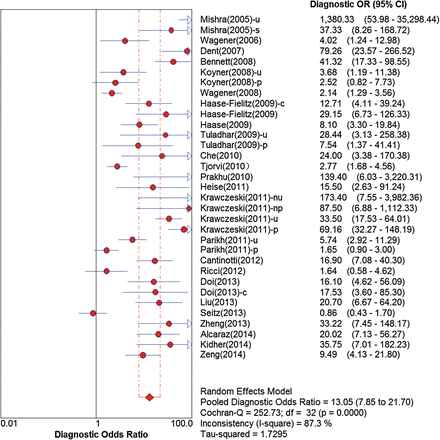
Forest plots of the diagnostic OR (E) of NGAL in predicting CSA-AKI across all studies. u: urine; s: serum; p: plasma; nu: urine from neonates; np: plasma from neonates; c: pre-existing renal insufficiency; OR: odds ratio; CI: confidence interval; NGAL: neutrophil gelatinase-associated lipocalin; CSA-AKI: cardiac surgery-associated acute kidney injury.
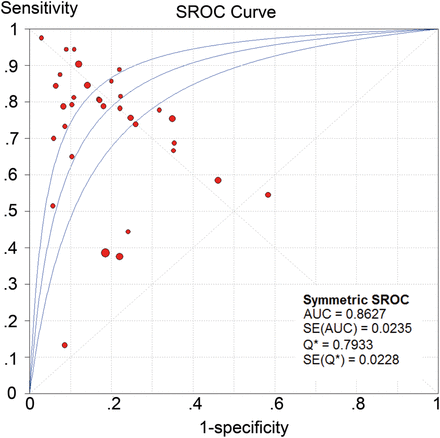
SROC plots of NGAL in predicting CSA-AKI across all studies. SROC: summary receiver operating characteristics; NGAL: neutrophil gelatinase-associated lipocalin; CSA-AKI: cardiac surgery-associated acute kidney injury.
Sensitivity analysis was conducted in terms of sample size, study design, sampling times and diagnostic criteria of CSA-AKI. The results were similar to the original, producing no obvious changes, when we excluded the small-sized studies (N ≤ 50), the retrospective studies, a study with sampling >6 h postoperatively or a study whose diagnostic criterion was ‘severe AKI’ [consistent with RIFLE stage I or the Acute Kidney Injury Network (AKIN) stage 2], respectively. In addition, the results showed overlapping confidence intervals (CIs), enhancing the reliability of our results (Supplementary Table 2).
Subgroup analysis
We performed subgroup analysis, results of which are shown in Table 3. Based on the comparisons of DOR and AUC, NGAL showed some variability in diagnostic value in CSA-AKI. The value of the NGAL level to predict CSA-AKI in neonates/children was substantially higher compared with that in adults (DOR, 19.37; AUC, 0.89 vs DOR, 8.98; AUC, 0.83). Across all studies of adults, we investigated the diagnostic accuracies of NGAL with and without PRI. It showed that the latter had a higher value to predict CSA-AKI (DOR, 15.43; AUC, 0.87 vs DOR, 6.56; AUC, 0.81). The DOR and AUC of the urine NGAL level were similar to those of the plasma/serum NGAL level in the prediction of CSA-AKI (DOR, 13.09; AUC, 0.85 vs DOR, 13.20; AUC, 0.88). However, when the studies that did head-to-head comparison of both ways to measure NGAL were included for further sub-subgroup analysis, the result was not the same as we expected. It showed that urine NGAL performed better for CSA-AKI prediction than plasma/serum NGAL (DOR, 23.05; AUC, 0.91 vs DOR, 12.66; AUC, 0.84) (Supplementary Table 3). And when measured using standardized clinical platforms, lower DOR and AUC values were shown (DOR, 8.31; AUC, 0.81), compared with research-based assays (DOR, 19.22; AUC, 0.89). We also did the subgroup analysis of 12 data sets from 8 studies in which multivariable analyses were provided. The results showed that the DOR was 32.09 (95% CI, 13.63–75.57) and an AUC (SE) was 0.93 (0.02), which were much higher than the overall value (DOR, 13.05; AUC, 0.86). It would be more reasonable to believe NGAL as an independent predictor of CSA-AKI.
. | N . | Sen (95% CI) . | I2 (%) . | Spe (95% CI) . | I2 (%) . | PLR (95% CI) . | I2 (%) . | NLR (95% CI) . | I2 (%) . | DOR (95% CI) . | I2 (%) . | AUC . |
---|---|---|---|---|---|---|---|---|---|---|---|---|
Sample type | ||||||||||||
Urine | 17 | 0.73 (0.70–0.77) | 82.4 | 0.77 (0.75–0.79) | 88.1 | 3.68 (2.58–5.25) | 89 | 0.30 (0.20–0.46) | 88.8 | 13.09 (6.44–26.6) | 89.0 | 0.85 |
Plasma/serum | 16 | 0.61 (0.57–0.65) | 92.0 | 0.80 (0.78–0.82) | 90.3 | 4.40 (2.86–6.77) | 88 | 0.36 (0.23–0.56) | 94.3 | 13.20 (6.10–28.59) | 87.6 | 0.88 |
Characteristics of patient population | ||||||||||||
Neonates/children | 15 | 0.71 (0.68–0.74) | 93.3 | 0.77 (0.75–0.79) | 94.4 | 5.05 (3.03–8.41) | 94 | 0.27 (0.14–0.52) | 97 | 19.37 (7.76–48.34) | 92.3 | 0.89 |
Adults | 18 | 0.62 (0.58–0.66) | 79.0 | 0.80 (0.78–0.81) | 61.5 | 3.17 (2.53–3.98) | 63 | 90.40 (0.31–0.53) | 76.5 | 8.98 (5.47–14.73) | 70.6 | 0.83 |
Adults without PRI | 6 | 0.78 (0.70–0.85) | 27.1 | 0.82 (0.78–0.86) | 60.9 | 4.32 (3.25–5.73) | 42.5 | 0.29 (0.21–0.40) | 0 | 15.43 (9.03–26.38) | 0 | 0.87 |
Adults with PRI | 12 | 0.56 (0.51–0.61) | 79.4 | 0.79 (0.77–0.81) | 63.1 | 2.73 (2.13–3.48) | 61.5 | 0.48 (0.37–0.63) | 74.1 | 6.56 (3.79–11.34) | 70.4 | 0.81 |
Measurement method | ||||||||||||
Research-based assay | 19 | 0.76 (0.73–0.79) | 83.5 | 0.82 (0.80–0.84) | 77.1 | 4.52 (3.24–6.31) | 82.8 | 0.26 (0.16–0.41) | 89.2 | 19.22 (9.44–39.11) | 84.9 | 0.89 |
Standardized platforms | 14 | 0.58 (0.54–0.62) | 91.0 | 0.76 (0.74–0.78) | 93.3 | 3.31 (2.27–4.83) | 89.0 | 0.43 (0.30–0.61) | 92.8 | 8.31 (4.20–16.43) | 87.5 | 0.81 |
Studies with multivariable analyses provided | 12 | 0.78 (0.74–0.81) | 79.0 | 0.85 (0.83–0.87) | 41.37 | 6.39 (4.25–9.60) | 64.2 | 0.22 (0.12–0.39) | 137.5 | 32.09 (13.63–75.57) | 93.9 |
. | N . | Sen (95% CI) . | I2 (%) . | Spe (95% CI) . | I2 (%) . | PLR (95% CI) . | I2 (%) . | NLR (95% CI) . | I2 (%) . | DOR (95% CI) . | I2 (%) . | AUC . |
---|---|---|---|---|---|---|---|---|---|---|---|---|
Sample type | ||||||||||||
Urine | 17 | 0.73 (0.70–0.77) | 82.4 | 0.77 (0.75–0.79) | 88.1 | 3.68 (2.58–5.25) | 89 | 0.30 (0.20–0.46) | 88.8 | 13.09 (6.44–26.6) | 89.0 | 0.85 |
Plasma/serum | 16 | 0.61 (0.57–0.65) | 92.0 | 0.80 (0.78–0.82) | 90.3 | 4.40 (2.86–6.77) | 88 | 0.36 (0.23–0.56) | 94.3 | 13.20 (6.10–28.59) | 87.6 | 0.88 |
Characteristics of patient population | ||||||||||||
Neonates/children | 15 | 0.71 (0.68–0.74) | 93.3 | 0.77 (0.75–0.79) | 94.4 | 5.05 (3.03–8.41) | 94 | 0.27 (0.14–0.52) | 97 | 19.37 (7.76–48.34) | 92.3 | 0.89 |
Adults | 18 | 0.62 (0.58–0.66) | 79.0 | 0.80 (0.78–0.81) | 61.5 | 3.17 (2.53–3.98) | 63 | 90.40 (0.31–0.53) | 76.5 | 8.98 (5.47–14.73) | 70.6 | 0.83 |
Adults without PRI | 6 | 0.78 (0.70–0.85) | 27.1 | 0.82 (0.78–0.86) | 60.9 | 4.32 (3.25–5.73) | 42.5 | 0.29 (0.21–0.40) | 0 | 15.43 (9.03–26.38) | 0 | 0.87 |
Adults with PRI | 12 | 0.56 (0.51–0.61) | 79.4 | 0.79 (0.77–0.81) | 63.1 | 2.73 (2.13–3.48) | 61.5 | 0.48 (0.37–0.63) | 74.1 | 6.56 (3.79–11.34) | 70.4 | 0.81 |
Measurement method | ||||||||||||
Research-based assay | 19 | 0.76 (0.73–0.79) | 83.5 | 0.82 (0.80–0.84) | 77.1 | 4.52 (3.24–6.31) | 82.8 | 0.26 (0.16–0.41) | 89.2 | 19.22 (9.44–39.11) | 84.9 | 0.89 |
Standardized platforms | 14 | 0.58 (0.54–0.62) | 91.0 | 0.76 (0.74–0.78) | 93.3 | 3.31 (2.27–4.83) | 89.0 | 0.43 (0.30–0.61) | 92.8 | 8.31 (4.20–16.43) | 87.5 | 0.81 |
Studies with multivariable analyses provided | 12 | 0.78 (0.74–0.81) | 79.0 | 0.85 (0.83–0.87) | 41.37 | 6.39 (4.25–9.60) | 64.2 | 0.22 (0.12–0.39) | 137.5 | 32.09 (13.63–75.57) | 93.9 |
Sen: sensitivity; CIs: confidence intervals; Spe: specificity; PLR: positive likelihood ratio; NLR: negative likelihood ratio; DOR: diagnostic odds ratio; AUC: area under the receiver operating characteristic curve; PRI: pre-existing renal insufficiency.
. | N . | Sen (95% CI) . | I2 (%) . | Spe (95% CI) . | I2 (%) . | PLR (95% CI) . | I2 (%) . | NLR (95% CI) . | I2 (%) . | DOR (95% CI) . | I2 (%) . | AUC . |
---|---|---|---|---|---|---|---|---|---|---|---|---|
Sample type | ||||||||||||
Urine | 17 | 0.73 (0.70–0.77) | 82.4 | 0.77 (0.75–0.79) | 88.1 | 3.68 (2.58–5.25) | 89 | 0.30 (0.20–0.46) | 88.8 | 13.09 (6.44–26.6) | 89.0 | 0.85 |
Plasma/serum | 16 | 0.61 (0.57–0.65) | 92.0 | 0.80 (0.78–0.82) | 90.3 | 4.40 (2.86–6.77) | 88 | 0.36 (0.23–0.56) | 94.3 | 13.20 (6.10–28.59) | 87.6 | 0.88 |
Characteristics of patient population | ||||||||||||
Neonates/children | 15 | 0.71 (0.68–0.74) | 93.3 | 0.77 (0.75–0.79) | 94.4 | 5.05 (3.03–8.41) | 94 | 0.27 (0.14–0.52) | 97 | 19.37 (7.76–48.34) | 92.3 | 0.89 |
Adults | 18 | 0.62 (0.58–0.66) | 79.0 | 0.80 (0.78–0.81) | 61.5 | 3.17 (2.53–3.98) | 63 | 90.40 (0.31–0.53) | 76.5 | 8.98 (5.47–14.73) | 70.6 | 0.83 |
Adults without PRI | 6 | 0.78 (0.70–0.85) | 27.1 | 0.82 (0.78–0.86) | 60.9 | 4.32 (3.25–5.73) | 42.5 | 0.29 (0.21–0.40) | 0 | 15.43 (9.03–26.38) | 0 | 0.87 |
Adults with PRI | 12 | 0.56 (0.51–0.61) | 79.4 | 0.79 (0.77–0.81) | 63.1 | 2.73 (2.13–3.48) | 61.5 | 0.48 (0.37–0.63) | 74.1 | 6.56 (3.79–11.34) | 70.4 | 0.81 |
Measurement method | ||||||||||||
Research-based assay | 19 | 0.76 (0.73–0.79) | 83.5 | 0.82 (0.80–0.84) | 77.1 | 4.52 (3.24–6.31) | 82.8 | 0.26 (0.16–0.41) | 89.2 | 19.22 (9.44–39.11) | 84.9 | 0.89 |
Standardized platforms | 14 | 0.58 (0.54–0.62) | 91.0 | 0.76 (0.74–0.78) | 93.3 | 3.31 (2.27–4.83) | 89.0 | 0.43 (0.30–0.61) | 92.8 | 8.31 (4.20–16.43) | 87.5 | 0.81 |
Studies with multivariable analyses provided | 12 | 0.78 (0.74–0.81) | 79.0 | 0.85 (0.83–0.87) | 41.37 | 6.39 (4.25–9.60) | 64.2 | 0.22 (0.12–0.39) | 137.5 | 32.09 (13.63–75.57) | 93.9 |
. | N . | Sen (95% CI) . | I2 (%) . | Spe (95% CI) . | I2 (%) . | PLR (95% CI) . | I2 (%) . | NLR (95% CI) . | I2 (%) . | DOR (95% CI) . | I2 (%) . | AUC . |
---|---|---|---|---|---|---|---|---|---|---|---|---|
Sample type | ||||||||||||
Urine | 17 | 0.73 (0.70–0.77) | 82.4 | 0.77 (0.75–0.79) | 88.1 | 3.68 (2.58–5.25) | 89 | 0.30 (0.20–0.46) | 88.8 | 13.09 (6.44–26.6) | 89.0 | 0.85 |
Plasma/serum | 16 | 0.61 (0.57–0.65) | 92.0 | 0.80 (0.78–0.82) | 90.3 | 4.40 (2.86–6.77) | 88 | 0.36 (0.23–0.56) | 94.3 | 13.20 (6.10–28.59) | 87.6 | 0.88 |
Characteristics of patient population | ||||||||||||
Neonates/children | 15 | 0.71 (0.68–0.74) | 93.3 | 0.77 (0.75–0.79) | 94.4 | 5.05 (3.03–8.41) | 94 | 0.27 (0.14–0.52) | 97 | 19.37 (7.76–48.34) | 92.3 | 0.89 |
Adults | 18 | 0.62 (0.58–0.66) | 79.0 | 0.80 (0.78–0.81) | 61.5 | 3.17 (2.53–3.98) | 63 | 90.40 (0.31–0.53) | 76.5 | 8.98 (5.47–14.73) | 70.6 | 0.83 |
Adults without PRI | 6 | 0.78 (0.70–0.85) | 27.1 | 0.82 (0.78–0.86) | 60.9 | 4.32 (3.25–5.73) | 42.5 | 0.29 (0.21–0.40) | 0 | 15.43 (9.03–26.38) | 0 | 0.87 |
Adults with PRI | 12 | 0.56 (0.51–0.61) | 79.4 | 0.79 (0.77–0.81) | 63.1 | 2.73 (2.13–3.48) | 61.5 | 0.48 (0.37–0.63) | 74.1 | 6.56 (3.79–11.34) | 70.4 | 0.81 |
Measurement method | ||||||||||||
Research-based assay | 19 | 0.76 (0.73–0.79) | 83.5 | 0.82 (0.80–0.84) | 77.1 | 4.52 (3.24–6.31) | 82.8 | 0.26 (0.16–0.41) | 89.2 | 19.22 (9.44–39.11) | 84.9 | 0.89 |
Standardized platforms | 14 | 0.58 (0.54–0.62) | 91.0 | 0.76 (0.74–0.78) | 93.3 | 3.31 (2.27–4.83) | 89.0 | 0.43 (0.30–0.61) | 92.8 | 8.31 (4.20–16.43) | 87.5 | 0.81 |
Studies with multivariable analyses provided | 12 | 0.78 (0.74–0.81) | 79.0 | 0.85 (0.83–0.87) | 41.37 | 6.39 (4.25–9.60) | 64.2 | 0.22 (0.12–0.39) | 137.5 | 32.09 (13.63–75.57) | 93.9 |
Sen: sensitivity; CIs: confidence intervals; Spe: specificity; PLR: positive likelihood ratio; NLR: negative likelihood ratio; DOR: diagnostic odds ratio; AUC: area under the receiver operating characteristic curve; PRI: pre-existing renal insufficiency.
Heterogeneity analysis
The SROC was constructed and the points in the plots did not show a ‘shoulder arm’ pattern, suggesting no presence of the threshold effect. Furthermore, computation of the Spearman correction coefficient between the logit of sensitivity and logit of 1-specificity of NGAL was −0.325 (P = 0.065), also indicating no threshold effect.
To explore other possible reasons for the heterogeneity, meta-regression and subgroup analysis were performed (Table 3). The main source may be from characteristics of the patient population (P = 0.037) (Supplementary Table 4). The I2-values of diagnostic accuracies of adults without PRI were all significantly reduced. While the specimen type (urine or plasma/serum), the measurement method (research-based assay or standardized clinical laboratory platforms), the study design (prospective or retrospective), the sample size (n ≥ 50 or <50) and the study method (blinded or not) may be not the heterogeneity sources for NGAL.
DISCUSSION
In spite of progress in the development of strategies towards prevention, effective prevention of CSA-AKI has been still hampered by the delayed diagnosis of AKI with current markers (e.g. SCr). Given the shortcoming of the current markers, more reliable biomarkers, serving as ‘renal troponin’ should be considered, which may facilitate earlier recognition of tubular damage, rather than the declining function [12]. Subsequently, timely interventions may be triggered to help to potentially reverse renal injury and improve the patients' outcome.
Recently, NGAL has been investigated extensively and appeared to be one of the most promising early AKI biomarkers [47]. As an iron-transporting protein, NGAL may be expressed by the damaged tubule to induce re-epithelialization, playing a primary role in the renal injury and repair process [48]. It is found to be markedly up-regulated in the early postischaemic kidney, prior to the rise in SCr that might happen several days later [49]. And it had been suggested that using urine NGAL would be a cost-effective strategy for AKI [50], because it appeared to lead to lower expected costs as a result of quicker diagnosis, followed with earlier institution of preventive and salvage therapies.
A previous meta-analysis [51] published in 2009 had shown NGAL to be useful in early AKI diagnosis; to update and fully evaluate the predictive value of NGAL for the early diagnosis of CSA-AKI, which was one of the subgroups of the previous meta-analysis, we performed the present meta-analysis. To our knowledge, it might be a novel meta-analysis which assessed the diagnostic value of NGAL for CSA-AKI. Consistent with the result from the previous meta-analysis, we found NGAL to be a useful early (within 12 h postoperatively) predictor of CSA-AKI, with DOR of 13.05 and AUC of 0.86. Sensitivity analysis revealed that the findings were robust.
So far, NGAL is the only biomarker that has been studied in both plasma/serum and urine for the early diagnosis of AKI. We therefore assessed the predictive value of plasma/serum and urine NGAL, respectively, and further examined the studies that did head-to-head comparison of both ways for sub-subgroup analysis. With respect to plasma/serum NGAL, unsatisfactory results had been shown in some studies [15, 17, 36], and our sub-subgroup analysis also showed that plasma/serum NGAL might have inferior performance to urine NGAL. It is considered that plasma/serum NGAL is a closer reflection of systemic inflammation than the extent of renal injury inflicted. Distant organs (such as the lungs and liver), stress, activated neutrophils and macrophages may have a dramatic influence on the plasma/serum NGAL but not on urine, thereby the predictive ability of the former was reduced. On the other hand, urine is thought to be an ideal fluid for kidney biomarker discovery, with the non-invasive nature of sample collection and the reduced interfering proteins. The potential superiority of urinary NGAL was confirmed in our analysis. We suggest that before plasma/serum NGAL, urine NGAL could be taken into consideration. However, it may be difficult to obtain samples from patients with severe oliguria, which is not uncommon during cardiac surgery. In our meta-analysis, plasma/serum still had the highly predictive nature for early diagnosis of CSA-AKI (DOR, 12.66; AUC, 0.84), though inferior to urine. Plasma/serum NGAL may be an alternative, when urine is unobtainable in oliguric or anuric patients.
Our meta-analysis showed that the population settings were the main source of heterogeneity. Compared with the paediatric populations, an inferior predictive value of NGAL is found in adults, because almost all neonates/children enrolled had started with normal kidney function, and were less likely to exhibit a broad spectrum of comorbidities such as chronic heart failure, atherosclerosis, diabetes, nephrotoxin use (e.g. radiocontrast) and neutrophilic inflammation which might confound and hinder the NGAL's performance. It should be also noted that operative factors may be another plausible explanation for the result. Adult patients always had more complex surgery and longer bypass time, which lead to increased NGAL excretion independent of kidney injury. Further subgroup analysis was performed between patients with and without PRI in adults. It appeared that NGAL may be more discriminant in de novo AKI rather than AKI superimposed on chronic kidney disease (CKD). Several possible explanations may account for this result. Firstly, unlike the passive leakage of preformed troponin from an injured cardiomyocyte, NGAL expression is more complex, depending on the tubular cell function [48]. When it comes to kidneys with pre-existing injury, urine NGAL may fail to rapidly express in tubular epithelial cells and plasma NGAL may be inefficiently reabsorbed by the proximal renal tubule. Secondly, CKD is always accompanied with several comorbidities (e.g. atherosclerosis, diabetes etc.) as mentioned above, which may potentially influence the expression of NGAL. Combined, NGAL seemed to predict most accurately CSA-AKI in patients with limited comorbidities and normal baseline renal function. And it was suggested by McIlroy et al. [52] that the diagnostic performance of NGAL for postoperative AKI would be improved by stratified baseline renal function, allowing the use of different diagnostic cut-off points.
Previous studies evaluating NGAL as a predictor of AKI used cumbersome techniques (immunoblot, ELISA) that are somewhat difficult to implement in routine clinical practice. Recently, standardized clinical platforms for providing quantitative NGAL measurements in minutes have made this promising new biomarker more facile and deployable directly to the point of patient care. However, inconsistent with the previous meta-analysis [51], lower diagnostic accuracies were found in using standardized clinical platforms [17, 18, 29, 32, 36, 37, 39–41, 44, 45]. Several reasons may account for these results. Firstly, it has been indicated that the monomeric form of NGAL is the major form that is secreted by kidney epithelial cells and found in the urine of patients with AKI; hence, assays (such as western blots) that specifically detect the AKI-specific form may outperform platforms that detect multiple forms [53]. Secondly, though the architect assay detecting predominantly the monomeric form of NGAL in urine had given much more precise results [54, 55], the Triage kit for plasma/serum NGAL measurement had shown a high variation around the proposed cut-off in a recent study by Cavalier et al. [55]. And the author thought this variability could be even worse in the hands of many different inexperienced nurses in a crowded ward, because this high variation was observed when the analyses were performed under optimal conditions, with a well-trained technician. Last but not least, we further examined the studies that reported the poor performance of NGAL when standardized clinical platforms were used. One of them was retrospective [36], and one study's primary outcome was the development of severe AKI (consistent with RIFLE stage 1 or AKIN stage 2) [17]. Besides, most of them had included patients with older ages, or PRI, which might hinder the NGAL's performance. And as far as our knowledge goes, there were few studies that did head-to-head comparison of research-based assays and clinical platforms. Thus, prospective studies with multiple AKI settings or patient populations that perform head-to-head comparison of both methods may contribute to a more comprehensive evaluation of NGAL.
Our meta-analysis had several limitations. Firstly, using elevations in SCr as ‘gold standards’ in the diagnosis criteria of CSA-AKI might be inadequate [56], which would result in misinterpretations of the diagnostic performance of NGAL. A recently proposed consensus definition of AKI uses a small increase of ≥0.3 mg/dl or 50% in SCr over 7 days [11], which has increased the sensitivity for diagnosis of AKI; however, in AKI, significant renal disease can exist with minimal or no change in SCr. A meta-analysis of NGAL in AKI had shown that patients with increased NGAL values but no SCr elevations (NGAL-positive, creatinine-negative) had adverse clinical outcomes [57]. It has been suggested that a composite end-point by long-term events such as progressive CKD, eventual need for RRT and mortality, other than a short-term change in SCr, should be regarded to test novel tubular injury biomarkers [56]. Secondly, because of the multifactorial aetiologies, including ischaemia–reperfusion injury, oxidative stress and the systemic inflammatory response, and the multiple overlapping pathophysiological mechanisms contributing to CSA-AKI [58], it is very unlikely that one single biomarker such as NGAL gives sufficiently high sensitivity and specificity. Instead, a panel of strategically selected candidates may be more beneficial for detecting CSA-AKI efficiently and accurately. Six studies enrolled in our meta-analysis reported on the application of the NGAL and its combination with other novel biomarkers for early screening of CSA-AKI [15, 31, 34, 39, 42, 46]. Four of them documented enhanced predictive values, and the remaining two yielded limited improvement when NGAL was combined with cystatin C [15, 31], which appeared rather to be a marker of chronic renal impairment than of acute worsening renal function. Thirdly, different cut-offs were used in the enrolled studies. These discrepancies may be attributable to differences in experimental protocols (such as various sampling specimens, times and methods or clinical conditions of the enrolled patients). And some were corrected by urine creatinine [34, 42, 46], though there is no enough evidence to date to demonstrate urine NGAL levels corrected have superior diagnostic abilities to those of not corrected. So it was difficult for us to determine the optimized overall cut-off for CSA-AKI. Further investigations should be undertaken, according to a range of clinical settings of CSA-AKI. Fourthly, a certain degree of publication bias was found in the analysis. The exclusion of unpublished data, ongoing studies, conference abstracts, letters to editors and non-English language studies may have led to the publication bias, causing the diagnostic accuracies of NGAL in our meta-analysis to be overestimated.
CONCLUSION
In conclusion, our meta-analysis demonstrated that as early as within 6 h of measuring postoperatively, both urine and plasma/serum NGAL appeared to be promising biomarkers of CSA-AKI, especially in neonates/children or adults with limited comorbidities and normal baseline renal function. To optimize the predictive value of NGAL for CSA-AKI, We anticipate that further analysis could more definitively establish the thresholds for NGAL in different samples (urine and plasma) and different patient cohorts.
SUPPLEMENTARY MATERIAL
Supplementary material is available at EJCTS online.
Funding
This study was supported in part by a project from Medical Science Research Foundation of Zhejiang Province (Grant No. 201336474, to Qun Luo) and Ningbo city Natural Science Foundation (Grant No. 2013A610266, to Fangfang Zhou). The funders had no role in study design, data collection and analysis, decision to publish or preparation of the manuscript.
Conflict of interest: none declared.
REFERENCES