-
PDF
- Split View
-
Views
-
Cite
Cite
Stuart J. Head, Ruben L.J. Osnabrugge, Neil J. Howell, Nick Freemantle, Ben Bridgewater, Domenico Pagano, A. Pieter Kappetein, A systematic review of risk prediction in adult cardiac surgery: considerations for future model development, European Journal of Cardio-Thoracic Surgery, Volume 43, Issue 5, May 2013, Pages e121–e129, https://doi.org/10.1093/ejcts/ezt044
- Share Icon Share
Abstract
Risk prediction in adult patients undergoing cardiac surgery remains inaccurate and should be further improved. Therefore, we aimed to identify risk factors that are predictive of mortality, stroke, renal failure and/or length of stay after adult cardiac surgery in contemporary practice.
We searched the Medline database for English-language original contributions from January 2000 to December 2011 to identify preoperative independent risk factors of one of the following outcomes after adult cardiac surgery: death, stroke, renal failure and/or length of stay. Two investigators independently screened the studies. Inclusion criteria were (i) the study described an adult cardiac patient population; (ii) the study was an original contribution; (iii) multivariable analyses were performed to identify independent predictors; (iv) ≥1 of the predefined outcomes was analysed; (v) at least one variable was an independent predictor, or a variable was included in a risk model that was developed.
The search yielded 5768 studies. After the initial title screening, a second screening of the full texts of 1234 studies was performed. Ultimately, 844 studies were included in the systematic review. In these studies, we identified a large number of independent predictors of mortality, stroke, renal failure and length of stay, which could be categorized into variables related to: disease pathology, planned surgical procedure, patient demographics, patient history, patient comorbidities, patient status, blood values, urine values, medication use and gene mutations. Many of these variables are frequently not considered as predictive of outcomes.
Risk estimates of mortality, stroke, renal failure and length of stay may be improved by the inclusion of additional (non-traditional) innovative risk factors. Current and future databases should consider collecting these variables.
INTRODUCTION
Predicting procedural mortality in adult cardiac surgery is critical for decision-making purposes, particularly when there are different treatments options available, as well as for benchmarking and outcome evaluation both at institutional and surgeon levels. Several prediction models have been developed with the main goal of estimating the risk of operative mortality for patients undergoing coronary artery bypass grafting (CABG), aortic valve replacement (AVR) or cardiac surgery in general [1–4]. Despite their usefulness, it remains challenging to develop a risk model that performs accurately across the spectrum of low-, intermediate- and high-risk patients evaluated for cardiac surgery. Although the recently developed EuroSCORE II may be associated with improvements when compared with the original additive and logistic EuroSCOREs [5], risk prediction remains a challenge in European patients [6–8]. The Society of Thoracic Surgeons (STS) score has shown to outperform the EuroSCORE [9–11], but still a number of studies have demonstrated poor model performance in certain patient subgroups [12–14]. Particularly in high-risk patients, risk models have been shown to be poorly calibrated and to over-predict mortality.
The reasons for suboptimal model performance are multifactorial. While conventional cardiovascular risk factors (e.g. renal failure, diabetes) are considered for inclusion in a model, less obvious factors may be valuable as well. Many risk models are developed through standard statistical approaches, not taking into account risk factor interactions or procedure-specific weightings [15]. A mismatch is frequently present between the model development patient cohort and the patient cohort that it is used for in practice; some patient subgroups are continuously under-represented. Considering these arguments, it is important to (i) clarify the purpose of a model, (ii) develop a model that is useful and (iii) define the limits of that usefulness. Any model should be based on the available literature and clinical intuition to define the appropriate dataset for model development.
The European Association for Cardio-Thoracic Surgery (EACTS) is establishing a quality improvement programme for adult cardiac surgery with an international database as an important component, aiming to bring forward an EACTS risk model. This score can be used to evaluate adult cardiac surgery practice in Europe. We performed a systematic review of the literature to identify which variables may need to be collected to be able to develop a better risk-prediction model.
METHODS
Search strategy
We systematically searched the MEDLINE database for English language original contributions from January 2000 to December 2011 to identify preoperative independent risk factors of one of the following outcomes after adult cardiac surgery: death, stroke, renal failure and/or length of stay. Our search entry consisted of outcome keywords: ‘mortality’ OR ‘death’ OR ‘stroke’ OR ‘cerebrovascular event’ OR ‘renal failure’ OR ‘length of stay’ OR ‘LOS’; subject keywords: ‘cardiac surgery’ OR ‘heart surgery’ OR ‘heart valve surgery’ OR ‘valve replacement’ OR ‘AVR' OR ‘MVR’ OR ‘valve repair’ OR ‘MVP’ OR ‘coronary artery bypass grafting’ OR ‘CABG’; and analysis keywords: ‘risk model’ OR ‘risk score’ OR ‘risk factor’ OR ‘independent’ OR ‘multivariate’ OR ‘multivariable’ OR ‘c-index’ OR ‘c-statistic’ OR ‘area under the curve’ OR ‘AUC’.
Study inclusion
Two investigators (S.J.H. and R.L.J.O) independently screened the studies identified by the search. During the first round of screening, all titles were judged for their relevance. Studies evaluating non-cardiac surgery, percutaneous or transcatheter therapies or diagnostic modalities were excluded. Many risk models have been developed for CABG surgery and/or valvular surgery, therefore to be homogeneous but also comprehensive, we excluded studies that focused on paediatrics, congenital cases, aortic arch or root surgery or heart transplants. Studies that were inconclusive with respect to the performed procedures and reported outcomes of a non-defined group, for example ‘patients that underwent cardiac surgery’, were included.
After identifying potentially relevant studies, the full-length articles were screened using the following criteria: (i) the study indeed described an adult cardiac patient population; (ii) the study was an original contribution; (iii) multivariable analyses were performed to identify independent predictors; (iv) the outcome of mortality, stroke, renal failure and/or length of stay was assessed and (v) at least one variable was an independent predictor, or a variable was included in a risk model that was developed.
Data extraction
For each end-point, independent predictors were extracted from the included studies.
The terminology of predictors differed significantly among studies. For example ‘aortic calcification’ was also reported as ‘extend of atherosclerotic ascending aorta disease’, ‘thoracic aorta total plaque-burden’ or ‘severe atheromatous aortic disease’. Risk factors were measured and reported according to different indexes; for example renal function was indicated with serum creatinine, creatinine clearance or estimated glomerular filtration rate. Such variations were merged into a single variable to avoid repetition.
RESULTS
The search yielded 5768 results (Fig. 1). After excluding non-relevant studies from an initial title screening, a second screening of the full texts of 1234 studies was performed. Another 351 studies were found to be irrelevant because the patient population did not meet the criteria, the end-point used was not death, stroke, renal failure or length of stay or no independent predictors were identified. The full texts of 78 studies could not be retrieved, so the abstracts were screened for their relevance. Ultimately, 844 studies were included in the systematic review.
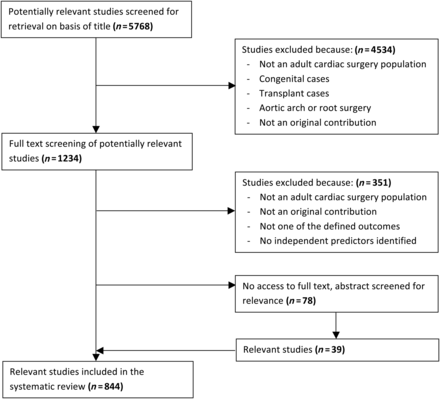
The diagnosed disease pathology and planned surgical procedure are essential elements in a risk model and always need to be documented (Table 1). The independent predictors of death, stroke, renal failure and length of stay are listed in Tables 2–5. The predictors were categorized as patient demographics, patient history, patient co-morbidities, patient status, blood values, urine values, medication use and gene mutations.
Disease pathology . | Planned surgical procedure . |
---|---|
Number of coronary vessel disease | Coronary artery bypass grafting |
Significant left main stenosis | Aortic valve replacement |
Coronary artery disease complexity (e.g. SYNTAX score) | Aortic valve repair |
Aortic valve stenosis | Aortic root surgery |
Aortic valve regurgitation | Mitral valve replacement |
Mitral valve stenosis | Mitral valve repair |
Mitral valve regurgitation | Tricuspid valve replacement |
Tricuspid valve regurgitation | Tricuspid valve repair |
Persistent atrial fibrillation | Aortic surgery |
Ascending aorta aneurysm | Maze |
Aortic arch aneurysm |
Disease pathology . | Planned surgical procedure . |
---|---|
Number of coronary vessel disease | Coronary artery bypass grafting |
Significant left main stenosis | Aortic valve replacement |
Coronary artery disease complexity (e.g. SYNTAX score) | Aortic valve repair |
Aortic valve stenosis | Aortic root surgery |
Aortic valve regurgitation | Mitral valve replacement |
Mitral valve stenosis | Mitral valve repair |
Mitral valve regurgitation | Tricuspid valve replacement |
Tricuspid valve regurgitation | Tricuspid valve repair |
Persistent atrial fibrillation | Aortic surgery |
Ascending aorta aneurysm | Maze |
Aortic arch aneurysm |
Disease pathology . | Planned surgical procedure . |
---|---|
Number of coronary vessel disease | Coronary artery bypass grafting |
Significant left main stenosis | Aortic valve replacement |
Coronary artery disease complexity (e.g. SYNTAX score) | Aortic valve repair |
Aortic valve stenosis | Aortic root surgery |
Aortic valve regurgitation | Mitral valve replacement |
Mitral valve stenosis | Mitral valve repair |
Mitral valve regurgitation | Tricuspid valve replacement |
Tricuspid valve regurgitation | Tricuspid valve repair |
Persistent atrial fibrillation | Aortic surgery |
Ascending aorta aneurysm | Maze |
Aortic arch aneurysm |
Disease pathology . | Planned surgical procedure . |
---|---|
Number of coronary vessel disease | Coronary artery bypass grafting |
Significant left main stenosis | Aortic valve replacement |
Coronary artery disease complexity (e.g. SYNTAX score) | Aortic valve repair |
Aortic valve stenosis | Aortic root surgery |
Aortic valve regurgitation | Mitral valve replacement |
Mitral valve stenosis | Mitral valve repair |
Mitral valve regurgitation | Tricuspid valve replacement |
Tricuspid valve regurgitation | Tricuspid valve repair |
Persistent atrial fibrillation | Aortic surgery |
Ascending aorta aneurysm | Maze |
Aortic arch aneurysm |
Patient characteristics . | |||
---|---|---|---|
Demographics | Carotid artery disease | On intubation/ventilation | Lactate dehydrogenase |
Age | Peripheral vascular disease | Sepsis | INR group |
Gender | (Severity of) atherosclerotic aortic disease | Active endocarditis | PTT |
Race | Atrial fibrillation | Vegetations size (endocarditis) | Antithrombin 3 |
Weight | Type of arrhythmia | Prosthetic valve endocarditis | HPF4 antibodies |
Height | Hypertension | Staphylococcus endocarditis infection | Thrombocytes |
Body surface area | Pulmonary function/disease (e.g. COPD) | Pulmonary oedema | Lymphocyte |
Geographic region (city, rural) | Pulmonary hypertension | Ventilator-associated pneumonia | Neutrophil |
Social economic status | Renal function/failure | Multiorgan failure | Total cholesterol |
Employment status (unemployed) | Liver function/disease | Ventricular assist device | Non-HDL cholesterol |
Type of personality | Malignancy | Resuscitation | Cholesterol esters |
Family history | Peptic ulcer disease | Postinfarct septal rupture | Triglycerides |
Primary payer | Status | Unstable/shock | Urine values |
Current smoker | Frailty | Intra-aortic balloon pump | Proteinuria |
Alcohol abuse | Energy level | Urgency of surgery | Medications |
History | Problems with self-care | ASA score | Aspirin |
Pack-years smoking | Non-ambulatory state | Pulse pressure | Warfarin or coumadin |
Previous hospitalization for heart failure | Mental component score (SF-36) | Blood values | Other anticoagulant |
Timing and number of previous PCI | Physical component score (SF-36) | Haemoglobin | Thrombolysis |
Timing of congestive heart failure | Health status (EQ-5D) | Haematocrit | Nitroglycerin |
Timing and location of previous MIa | CCS classification | Homocysteine | Statin |
Timing of dialysis | NYHA classification | Creatinine | β-Blocker |
Timing of previous TIA/CVA | Left ventricular ejection fraction | HbA1c | Catecholamine |
Timing of previous angina | LV end-systolic diameter/volume | Glucose | Digoxin |
History of hematological disorder/coagulopathy | LV hypertrophy | CRP | Digitalis |
Previous surgery for thrombosis | LV end-diastolic pressure/diameter | BNP | Antidepressant (SSRI) |
History of thyroid disease | Restrictive LV filling | NT-proBNP | Inotropic support |
Immune deficiency | LV posterior wall thickness | Interleukin 6 | Immunosuppressive therapy |
Connective tissue disease | LV mass index | Endotoxin core antibody | Gene mutations |
Pathological weight-loss | Lack of contractile reserve | Sodium | C677T mutation in MTHFR gene |
Pacemaker implantation | Left atrial diameter | Magnesium | VEGF +405 GG |
Number and type of reoperations | Small annulus | Protein | rs10116277 (2 allele) − Chromosome 9p21 |
Comorbidities | Right ventricular end-diastolic area | Albumin | rs1042579 recessive |
Diabetes | Right atrial pressure | Bilirubin | |
Metabolic syndrome | Cardiothoracic ratio | Aspartate aminotransferase | |
Cerebrovascular disease | Heart rate | Uric acid level | |
Neurological disorder | Conduction defect | CK-MB | |
Depression | Corrected QT interval | High-sensitive Troponin T | |
Anxiety | Amount of ST-segment depression | Troponin T | |
Psychoses | Preoperative intensive care unit stay | Troponin I |
Patient characteristics . | |||
---|---|---|---|
Demographics | Carotid artery disease | On intubation/ventilation | Lactate dehydrogenase |
Age | Peripheral vascular disease | Sepsis | INR group |
Gender | (Severity of) atherosclerotic aortic disease | Active endocarditis | PTT |
Race | Atrial fibrillation | Vegetations size (endocarditis) | Antithrombin 3 |
Weight | Type of arrhythmia | Prosthetic valve endocarditis | HPF4 antibodies |
Height | Hypertension | Staphylococcus endocarditis infection | Thrombocytes |
Body surface area | Pulmonary function/disease (e.g. COPD) | Pulmonary oedema | Lymphocyte |
Geographic region (city, rural) | Pulmonary hypertension | Ventilator-associated pneumonia | Neutrophil |
Social economic status | Renal function/failure | Multiorgan failure | Total cholesterol |
Employment status (unemployed) | Liver function/disease | Ventricular assist device | Non-HDL cholesterol |
Type of personality | Malignancy | Resuscitation | Cholesterol esters |
Family history | Peptic ulcer disease | Postinfarct septal rupture | Triglycerides |
Primary payer | Status | Unstable/shock | Urine values |
Current smoker | Frailty | Intra-aortic balloon pump | Proteinuria |
Alcohol abuse | Energy level | Urgency of surgery | Medications |
History | Problems with self-care | ASA score | Aspirin |
Pack-years smoking | Non-ambulatory state | Pulse pressure | Warfarin or coumadin |
Previous hospitalization for heart failure | Mental component score (SF-36) | Blood values | Other anticoagulant |
Timing and number of previous PCI | Physical component score (SF-36) | Haemoglobin | Thrombolysis |
Timing of congestive heart failure | Health status (EQ-5D) | Haematocrit | Nitroglycerin |
Timing and location of previous MIa | CCS classification | Homocysteine | Statin |
Timing of dialysis | NYHA classification | Creatinine | β-Blocker |
Timing of previous TIA/CVA | Left ventricular ejection fraction | HbA1c | Catecholamine |
Timing of previous angina | LV end-systolic diameter/volume | Glucose | Digoxin |
History of hematological disorder/coagulopathy | LV hypertrophy | CRP | Digitalis |
Previous surgery for thrombosis | LV end-diastolic pressure/diameter | BNP | Antidepressant (SSRI) |
History of thyroid disease | Restrictive LV filling | NT-proBNP | Inotropic support |
Immune deficiency | LV posterior wall thickness | Interleukin 6 | Immunosuppressive therapy |
Connective tissue disease | LV mass index | Endotoxin core antibody | Gene mutations |
Pathological weight-loss | Lack of contractile reserve | Sodium | C677T mutation in MTHFR gene |
Pacemaker implantation | Left atrial diameter | Magnesium | VEGF +405 GG |
Number and type of reoperations | Small annulus | Protein | rs10116277 (2 allele) − Chromosome 9p21 |
Comorbidities | Right ventricular end-diastolic area | Albumin | rs1042579 recessive |
Diabetes | Right atrial pressure | Bilirubin | |
Metabolic syndrome | Cardiothoracic ratio | Aspartate aminotransferase | |
Cerebrovascular disease | Heart rate | Uric acid level | |
Neurological disorder | Conduction defect | CK-MB | |
Depression | Corrected QT interval | High-sensitive Troponin T | |
Anxiety | Amount of ST-segment depression | Troponin T | |
Psychoses | Preoperative intensive care unit stay | Troponin I |
ACE: angiotensin-converting enzyme; ASA: American Society of Anaesthesiologists; BNP: brain natriuretic peptide; CCS: Canadian Cardiovascular Society; CK-MB: creatine kinase myocardial band; COPD: chronic obstructive pulmonary disease; CRP: c-reactive protein; CVA: cerebrovascular accident; HDL: high-density lipoprotein; HPF4: heparin-platelet factor 4; ICU: intensive care unit; INR: international normalized ratio; MI: myocardial infarction; NT-proBNP: N-terminal-pro-brain natriuretic peptide; NYHA: New York Heart Association; LV: left ventricular; PCI: percutaneous coronary intervention; PTT: partial thromboplastin time; SSRI: selective serotonin reuptake inhibitor; TIA: transient ischaemic attack.
aInferior/anterior myocardial infarction.
Patient characteristics . | |||
---|---|---|---|
Demographics | Carotid artery disease | On intubation/ventilation | Lactate dehydrogenase |
Age | Peripheral vascular disease | Sepsis | INR group |
Gender | (Severity of) atherosclerotic aortic disease | Active endocarditis | PTT |
Race | Atrial fibrillation | Vegetations size (endocarditis) | Antithrombin 3 |
Weight | Type of arrhythmia | Prosthetic valve endocarditis | HPF4 antibodies |
Height | Hypertension | Staphylococcus endocarditis infection | Thrombocytes |
Body surface area | Pulmonary function/disease (e.g. COPD) | Pulmonary oedema | Lymphocyte |
Geographic region (city, rural) | Pulmonary hypertension | Ventilator-associated pneumonia | Neutrophil |
Social economic status | Renal function/failure | Multiorgan failure | Total cholesterol |
Employment status (unemployed) | Liver function/disease | Ventricular assist device | Non-HDL cholesterol |
Type of personality | Malignancy | Resuscitation | Cholesterol esters |
Family history | Peptic ulcer disease | Postinfarct septal rupture | Triglycerides |
Primary payer | Status | Unstable/shock | Urine values |
Current smoker | Frailty | Intra-aortic balloon pump | Proteinuria |
Alcohol abuse | Energy level | Urgency of surgery | Medications |
History | Problems with self-care | ASA score | Aspirin |
Pack-years smoking | Non-ambulatory state | Pulse pressure | Warfarin or coumadin |
Previous hospitalization for heart failure | Mental component score (SF-36) | Blood values | Other anticoagulant |
Timing and number of previous PCI | Physical component score (SF-36) | Haemoglobin | Thrombolysis |
Timing of congestive heart failure | Health status (EQ-5D) | Haematocrit | Nitroglycerin |
Timing and location of previous MIa | CCS classification | Homocysteine | Statin |
Timing of dialysis | NYHA classification | Creatinine | β-Blocker |
Timing of previous TIA/CVA | Left ventricular ejection fraction | HbA1c | Catecholamine |
Timing of previous angina | LV end-systolic diameter/volume | Glucose | Digoxin |
History of hematological disorder/coagulopathy | LV hypertrophy | CRP | Digitalis |
Previous surgery for thrombosis | LV end-diastolic pressure/diameter | BNP | Antidepressant (SSRI) |
History of thyroid disease | Restrictive LV filling | NT-proBNP | Inotropic support |
Immune deficiency | LV posterior wall thickness | Interleukin 6 | Immunosuppressive therapy |
Connective tissue disease | LV mass index | Endotoxin core antibody | Gene mutations |
Pathological weight-loss | Lack of contractile reserve | Sodium | C677T mutation in MTHFR gene |
Pacemaker implantation | Left atrial diameter | Magnesium | VEGF +405 GG |
Number and type of reoperations | Small annulus | Protein | rs10116277 (2 allele) − Chromosome 9p21 |
Comorbidities | Right ventricular end-diastolic area | Albumin | rs1042579 recessive |
Diabetes | Right atrial pressure | Bilirubin | |
Metabolic syndrome | Cardiothoracic ratio | Aspartate aminotransferase | |
Cerebrovascular disease | Heart rate | Uric acid level | |
Neurological disorder | Conduction defect | CK-MB | |
Depression | Corrected QT interval | High-sensitive Troponin T | |
Anxiety | Amount of ST-segment depression | Troponin T | |
Psychoses | Preoperative intensive care unit stay | Troponin I |
Patient characteristics . | |||
---|---|---|---|
Demographics | Carotid artery disease | On intubation/ventilation | Lactate dehydrogenase |
Age | Peripheral vascular disease | Sepsis | INR group |
Gender | (Severity of) atherosclerotic aortic disease | Active endocarditis | PTT |
Race | Atrial fibrillation | Vegetations size (endocarditis) | Antithrombin 3 |
Weight | Type of arrhythmia | Prosthetic valve endocarditis | HPF4 antibodies |
Height | Hypertension | Staphylococcus endocarditis infection | Thrombocytes |
Body surface area | Pulmonary function/disease (e.g. COPD) | Pulmonary oedema | Lymphocyte |
Geographic region (city, rural) | Pulmonary hypertension | Ventilator-associated pneumonia | Neutrophil |
Social economic status | Renal function/failure | Multiorgan failure | Total cholesterol |
Employment status (unemployed) | Liver function/disease | Ventricular assist device | Non-HDL cholesterol |
Type of personality | Malignancy | Resuscitation | Cholesterol esters |
Family history | Peptic ulcer disease | Postinfarct septal rupture | Triglycerides |
Primary payer | Status | Unstable/shock | Urine values |
Current smoker | Frailty | Intra-aortic balloon pump | Proteinuria |
Alcohol abuse | Energy level | Urgency of surgery | Medications |
History | Problems with self-care | ASA score | Aspirin |
Pack-years smoking | Non-ambulatory state | Pulse pressure | Warfarin or coumadin |
Previous hospitalization for heart failure | Mental component score (SF-36) | Blood values | Other anticoagulant |
Timing and number of previous PCI | Physical component score (SF-36) | Haemoglobin | Thrombolysis |
Timing of congestive heart failure | Health status (EQ-5D) | Haematocrit | Nitroglycerin |
Timing and location of previous MIa | CCS classification | Homocysteine | Statin |
Timing of dialysis | NYHA classification | Creatinine | β-Blocker |
Timing of previous TIA/CVA | Left ventricular ejection fraction | HbA1c | Catecholamine |
Timing of previous angina | LV end-systolic diameter/volume | Glucose | Digoxin |
History of hematological disorder/coagulopathy | LV hypertrophy | CRP | Digitalis |
Previous surgery for thrombosis | LV end-diastolic pressure/diameter | BNP | Antidepressant (SSRI) |
History of thyroid disease | Restrictive LV filling | NT-proBNP | Inotropic support |
Immune deficiency | LV posterior wall thickness | Interleukin 6 | Immunosuppressive therapy |
Connective tissue disease | LV mass index | Endotoxin core antibody | Gene mutations |
Pathological weight-loss | Lack of contractile reserve | Sodium | C677T mutation in MTHFR gene |
Pacemaker implantation | Left atrial diameter | Magnesium | VEGF +405 GG |
Number and type of reoperations | Small annulus | Protein | rs10116277 (2 allele) − Chromosome 9p21 |
Comorbidities | Right ventricular end-diastolic area | Albumin | rs1042579 recessive |
Diabetes | Right atrial pressure | Bilirubin | |
Metabolic syndrome | Cardiothoracic ratio | Aspartate aminotransferase | |
Cerebrovascular disease | Heart rate | Uric acid level | |
Neurological disorder | Conduction defect | CK-MB | |
Depression | Corrected QT interval | High-sensitive Troponin T | |
Anxiety | Amount of ST-segment depression | Troponin T | |
Psychoses | Preoperative intensive care unit stay | Troponin I |
ACE: angiotensin-converting enzyme; ASA: American Society of Anaesthesiologists; BNP: brain natriuretic peptide; CCS: Canadian Cardiovascular Society; CK-MB: creatine kinase myocardial band; COPD: chronic obstructive pulmonary disease; CRP: c-reactive protein; CVA: cerebrovascular accident; HDL: high-density lipoprotein; HPF4: heparin-platelet factor 4; ICU: intensive care unit; INR: international normalized ratio; MI: myocardial infarction; NT-proBNP: N-terminal-pro-brain natriuretic peptide; NYHA: New York Heart Association; LV: left ventricular; PCI: percutaneous coronary intervention; PTT: partial thromboplastin time; SSRI: selective serotonin reuptake inhibitor; TIA: transient ischaemic attack.
aInferior/anterior myocardial infarction.
Patient characteristics . | |
---|---|
Demographics | Status |
Age | Left ventricular ejection fraction |
Gender | Active infection |
Race | Active endocarditis |
Body surface area | Intra-aortic balloon pump |
Current smoker | Unstable/shock |
History | Urgency of surgery |
Timing of smoking | Pulse pressure |
Timing of previous TIA/CVA | Blood values |
Timing of previous MI | Haemoglobin |
Previous deep vein thrombosis | Creatinine |
Number of reoperations | INR group |
Dialysis | Medications |
Comorbidities | Aspirin |
Diabetes | Statin |
Cerebrovascular disease | ACE inhibitor |
Neurological status (e.g. deficit, dementia) | β-Blocker |
Carotid artery disease | Inotropic support |
Peripheral vascular disease | Gene mutations |
(Severity of) Atherosclerotic aortic disease | Interleukin 6 (-174G/C) |
Atrial fibrillation | CRP 3′UTR1846C/T |
Hypertension | |
Hypercholesterolaemia/lipidaemia | |
Renal function/failure | |
Pulmonary hypertension | |
Left ventricular hypertrophy |
Patient characteristics . | |
---|---|
Demographics | Status |
Age | Left ventricular ejection fraction |
Gender | Active infection |
Race | Active endocarditis |
Body surface area | Intra-aortic balloon pump |
Current smoker | Unstable/shock |
History | Urgency of surgery |
Timing of smoking | Pulse pressure |
Timing of previous TIA/CVA | Blood values |
Timing of previous MI | Haemoglobin |
Previous deep vein thrombosis | Creatinine |
Number of reoperations | INR group |
Dialysis | Medications |
Comorbidities | Aspirin |
Diabetes | Statin |
Cerebrovascular disease | ACE inhibitor |
Neurological status (e.g. deficit, dementia) | β-Blocker |
Carotid artery disease | Inotropic support |
Peripheral vascular disease | Gene mutations |
(Severity of) Atherosclerotic aortic disease | Interleukin 6 (-174G/C) |
Atrial fibrillation | CRP 3′UTR1846C/T |
Hypertension | |
Hypercholesterolaemia/lipidaemia | |
Renal function/failure | |
Pulmonary hypertension | |
Left ventricular hypertrophy |
ACE: angiotensin-converting enzyme; CVA: cerebrovascular accident; INR: international normalized ratio; MI: myocardial infarction; TIA: transient ischaemic attack.
Patient characteristics . | |
---|---|
Demographics | Status |
Age | Left ventricular ejection fraction |
Gender | Active infection |
Race | Active endocarditis |
Body surface area | Intra-aortic balloon pump |
Current smoker | Unstable/shock |
History | Urgency of surgery |
Timing of smoking | Pulse pressure |
Timing of previous TIA/CVA | Blood values |
Timing of previous MI | Haemoglobin |
Previous deep vein thrombosis | Creatinine |
Number of reoperations | INR group |
Dialysis | Medications |
Comorbidities | Aspirin |
Diabetes | Statin |
Cerebrovascular disease | ACE inhibitor |
Neurological status (e.g. deficit, dementia) | β-Blocker |
Carotid artery disease | Inotropic support |
Peripheral vascular disease | Gene mutations |
(Severity of) Atherosclerotic aortic disease | Interleukin 6 (-174G/C) |
Atrial fibrillation | CRP 3′UTR1846C/T |
Hypertension | |
Hypercholesterolaemia/lipidaemia | |
Renal function/failure | |
Pulmonary hypertension | |
Left ventricular hypertrophy |
Patient characteristics . | |
---|---|
Demographics | Status |
Age | Left ventricular ejection fraction |
Gender | Active infection |
Race | Active endocarditis |
Body surface area | Intra-aortic balloon pump |
Current smoker | Unstable/shock |
History | Urgency of surgery |
Timing of smoking | Pulse pressure |
Timing of previous TIA/CVA | Blood values |
Timing of previous MI | Haemoglobin |
Previous deep vein thrombosis | Creatinine |
Number of reoperations | INR group |
Dialysis | Medications |
Comorbidities | Aspirin |
Diabetes | Statin |
Cerebrovascular disease | ACE inhibitor |
Neurological status (e.g. deficit, dementia) | β-Blocker |
Carotid artery disease | Inotropic support |
Peripheral vascular disease | Gene mutations |
(Severity of) Atherosclerotic aortic disease | Interleukin 6 (-174G/C) |
Atrial fibrillation | CRP 3′UTR1846C/T |
Hypertension | |
Hypercholesterolaemia/lipidaemia | |
Renal function/failure | |
Pulmonary hypertension | |
Left ventricular hypertrophy |
ACE: angiotensin-converting enzyme; CVA: cerebrovascular accident; INR: international normalized ratio; MI: myocardial infarction; TIA: transient ischaemic attack.
Patient characteristics . | |
---|---|
Demographics | Blood values |
Age | Haemoglobin |
Gender | Haematocrit |
Race | Creatinine |
Height | Platelet count |
Weight | HbA1c |
Body surface area | Hyperuricemia |
History | Urea nitrogen |
Timing of previous MI | Bicarbonate |
Timing of recent cardiac catheterization | Sodium |
Timing of previous PCI | Albumin |
Dialysis | Bilirubin |
Congestive heart failure | Urine values |
Number or reoperations | Albumin to creatinine ratio |
Comorbidities | Proteinuria |
Diabetes | Medications |
Metabolic syndrome | Statin |
Cerebrovascular disease | Calcium channel blocker |
Carotid artery disease | ACE inhibitor |
Peripheral vascular disease | Renin-angiotensin system inhibitor |
Atrial fibrillation | Diuretic |
Hypertension | Immunosuppressive therapy |
Renal function/failure | Gene mutations |
Pulmonary disease (e.g. COPD) | Catechol-O-methyltransferase LL |
Pulmonary hypertension | |
Charlson comorbidity index | |
Status | |
CCS classification | |
NYHA classification | |
Left ventricular ejection fraction | |
Sepsis | |
Active endocarditis | |
Intra-aortic balloon pump | |
Unstable/shock | |
Urgency of surgery | |
ASA physical status |
Patient characteristics . | |
---|---|
Demographics | Blood values |
Age | Haemoglobin |
Gender | Haematocrit |
Race | Creatinine |
Height | Platelet count |
Weight | HbA1c |
Body surface area | Hyperuricemia |
History | Urea nitrogen |
Timing of previous MI | Bicarbonate |
Timing of recent cardiac catheterization | Sodium |
Timing of previous PCI | Albumin |
Dialysis | Bilirubin |
Congestive heart failure | Urine values |
Number or reoperations | Albumin to creatinine ratio |
Comorbidities | Proteinuria |
Diabetes | Medications |
Metabolic syndrome | Statin |
Cerebrovascular disease | Calcium channel blocker |
Carotid artery disease | ACE inhibitor |
Peripheral vascular disease | Renin-angiotensin system inhibitor |
Atrial fibrillation | Diuretic |
Hypertension | Immunosuppressive therapy |
Renal function/failure | Gene mutations |
Pulmonary disease (e.g. COPD) | Catechol-O-methyltransferase LL |
Pulmonary hypertension | |
Charlson comorbidity index | |
Status | |
CCS classification | |
NYHA classification | |
Left ventricular ejection fraction | |
Sepsis | |
Active endocarditis | |
Intra-aortic balloon pump | |
Unstable/shock | |
Urgency of surgery | |
ASA physical status |
ACE: angiotensin-converting enzyme; ASA: American Society of Anaesthesiologists; CCS: Canadian Cardiovascular Society; COPD: chronic obstructive pulmonary disease; MI: myocardial infarction; NYHA: New York Heart Association; PCI: percutaneous coronary intervention.
Patient characteristics . | |
---|---|
Demographics | Blood values |
Age | Haemoglobin |
Gender | Haematocrit |
Race | Creatinine |
Height | Platelet count |
Weight | HbA1c |
Body surface area | Hyperuricemia |
History | Urea nitrogen |
Timing of previous MI | Bicarbonate |
Timing of recent cardiac catheterization | Sodium |
Timing of previous PCI | Albumin |
Dialysis | Bilirubin |
Congestive heart failure | Urine values |
Number or reoperations | Albumin to creatinine ratio |
Comorbidities | Proteinuria |
Diabetes | Medications |
Metabolic syndrome | Statin |
Cerebrovascular disease | Calcium channel blocker |
Carotid artery disease | ACE inhibitor |
Peripheral vascular disease | Renin-angiotensin system inhibitor |
Atrial fibrillation | Diuretic |
Hypertension | Immunosuppressive therapy |
Renal function/failure | Gene mutations |
Pulmonary disease (e.g. COPD) | Catechol-O-methyltransferase LL |
Pulmonary hypertension | |
Charlson comorbidity index | |
Status | |
CCS classification | |
NYHA classification | |
Left ventricular ejection fraction | |
Sepsis | |
Active endocarditis | |
Intra-aortic balloon pump | |
Unstable/shock | |
Urgency of surgery | |
ASA physical status |
Patient characteristics . | |
---|---|
Demographics | Blood values |
Age | Haemoglobin |
Gender | Haematocrit |
Race | Creatinine |
Height | Platelet count |
Weight | HbA1c |
Body surface area | Hyperuricemia |
History | Urea nitrogen |
Timing of previous MI | Bicarbonate |
Timing of recent cardiac catheterization | Sodium |
Timing of previous PCI | Albumin |
Dialysis | Bilirubin |
Congestive heart failure | Urine values |
Number or reoperations | Albumin to creatinine ratio |
Comorbidities | Proteinuria |
Diabetes | Medications |
Metabolic syndrome | Statin |
Cerebrovascular disease | Calcium channel blocker |
Carotid artery disease | ACE inhibitor |
Peripheral vascular disease | Renin-angiotensin system inhibitor |
Atrial fibrillation | Diuretic |
Hypertension | Immunosuppressive therapy |
Renal function/failure | Gene mutations |
Pulmonary disease (e.g. COPD) | Catechol-O-methyltransferase LL |
Pulmonary hypertension | |
Charlson comorbidity index | |
Status | |
CCS classification | |
NYHA classification | |
Left ventricular ejection fraction | |
Sepsis | |
Active endocarditis | |
Intra-aortic balloon pump | |
Unstable/shock | |
Urgency of surgery | |
ASA physical status |
ACE: angiotensin-converting enzyme; ASA: American Society of Anaesthesiologists; CCS: Canadian Cardiovascular Society; COPD: chronic obstructive pulmonary disease; MI: myocardial infarction; NYHA: New York Heart Association; PCI: percutaneous coronary intervention.
Patient characteristics . | |
---|---|
Demographics | Status |
Age | SF-36 quality of life |
Gender | CCS classification |
Race | NYHA classification |
Height | Left ventricular ejection fraction |
Weight | Diastolic dysfunction |
Body surface area | Right ventricular end-systolic diameter |
Geographic region (e.g. rural area) | Cardiothoracic ratio |
Social status | Frailty |
History | Immunosuppressive therapy |
Previous TIA/CVA | Rheumatic fever |
Previous embolism | Active infection |
Timing of MI | Active endocarditis |
Timing of PCI | Large endocarditis vegetations (15 mm) |
(Duration of preceding) Hypertension | Unstable/Shock |
Previous arrhythmia treatment | Intra-aortic balloon pump |
Dialysis | Urgency of surgery |
Previous endocarditis | Blood values |
Congestive heart failure | Haemoglobin |
Number of reoperations | NT-pro-BNP |
Comorbidities | BNP |
Diabetes | Creatinine |
Cerebrovascular disease | Medications |
Peripheral vascular disease | β-Blocker |
Atherosclerotic aortic disease | Non-aspirin platelet inhibitor |
Atrial fibrillation | Inotropic support |
Arrhythmia | Gene mutations |
Hypertension | Il-8-251AA |
Pulmonary function/disease (e.g. COPD) | Catechol-O-methyltransferase LL |
Pulmonary hypertension | |
Renal function/failure | |
Post-traumatic stress disorder | |
Depression | |
Liver function/failure | |
Malignancy | |
Dyslipidaemia/hypercholesterolaemia | |
Hyperglycaemia |
Patient characteristics . | |
---|---|
Demographics | Status |
Age | SF-36 quality of life |
Gender | CCS classification |
Race | NYHA classification |
Height | Left ventricular ejection fraction |
Weight | Diastolic dysfunction |
Body surface area | Right ventricular end-systolic diameter |
Geographic region (e.g. rural area) | Cardiothoracic ratio |
Social status | Frailty |
History | Immunosuppressive therapy |
Previous TIA/CVA | Rheumatic fever |
Previous embolism | Active infection |
Timing of MI | Active endocarditis |
Timing of PCI | Large endocarditis vegetations (15 mm) |
(Duration of preceding) Hypertension | Unstable/Shock |
Previous arrhythmia treatment | Intra-aortic balloon pump |
Dialysis | Urgency of surgery |
Previous endocarditis | Blood values |
Congestive heart failure | Haemoglobin |
Number of reoperations | NT-pro-BNP |
Comorbidities | BNP |
Diabetes | Creatinine |
Cerebrovascular disease | Medications |
Peripheral vascular disease | β-Blocker |
Atherosclerotic aortic disease | Non-aspirin platelet inhibitor |
Atrial fibrillation | Inotropic support |
Arrhythmia | Gene mutations |
Hypertension | Il-8-251AA |
Pulmonary function/disease (e.g. COPD) | Catechol-O-methyltransferase LL |
Pulmonary hypertension | |
Renal function/failure | |
Post-traumatic stress disorder | |
Depression | |
Liver function/failure | |
Malignancy | |
Dyslipidaemia/hypercholesterolaemia | |
Hyperglycaemia |
BNP: brain natriuretic peptide; CCS: Canadian Cardiovascular Society; COPD: chronic obstructive pulmonary disease; CVA: cerebrovascular accident; MI: myocardial infarction; NT-proBNP: N-terminal-pro-brain natriuretic peptide; NYHA: New York Heart Association; PCI: percutaneous coronary intervention; TIA: transient ischaemic attack.
Patient characteristics . | |
---|---|
Demographics | Status |
Age | SF-36 quality of life |
Gender | CCS classification |
Race | NYHA classification |
Height | Left ventricular ejection fraction |
Weight | Diastolic dysfunction |
Body surface area | Right ventricular end-systolic diameter |
Geographic region (e.g. rural area) | Cardiothoracic ratio |
Social status | Frailty |
History | Immunosuppressive therapy |
Previous TIA/CVA | Rheumatic fever |
Previous embolism | Active infection |
Timing of MI | Active endocarditis |
Timing of PCI | Large endocarditis vegetations (15 mm) |
(Duration of preceding) Hypertension | Unstable/Shock |
Previous arrhythmia treatment | Intra-aortic balloon pump |
Dialysis | Urgency of surgery |
Previous endocarditis | Blood values |
Congestive heart failure | Haemoglobin |
Number of reoperations | NT-pro-BNP |
Comorbidities | BNP |
Diabetes | Creatinine |
Cerebrovascular disease | Medications |
Peripheral vascular disease | β-Blocker |
Atherosclerotic aortic disease | Non-aspirin platelet inhibitor |
Atrial fibrillation | Inotropic support |
Arrhythmia | Gene mutations |
Hypertension | Il-8-251AA |
Pulmonary function/disease (e.g. COPD) | Catechol-O-methyltransferase LL |
Pulmonary hypertension | |
Renal function/failure | |
Post-traumatic stress disorder | |
Depression | |
Liver function/failure | |
Malignancy | |
Dyslipidaemia/hypercholesterolaemia | |
Hyperglycaemia |
Patient characteristics . | |
---|---|
Demographics | Status |
Age | SF-36 quality of life |
Gender | CCS classification |
Race | NYHA classification |
Height | Left ventricular ejection fraction |
Weight | Diastolic dysfunction |
Body surface area | Right ventricular end-systolic diameter |
Geographic region (e.g. rural area) | Cardiothoracic ratio |
Social status | Frailty |
History | Immunosuppressive therapy |
Previous TIA/CVA | Rheumatic fever |
Previous embolism | Active infection |
Timing of MI | Active endocarditis |
Timing of PCI | Large endocarditis vegetations (15 mm) |
(Duration of preceding) Hypertension | Unstable/Shock |
Previous arrhythmia treatment | Intra-aortic balloon pump |
Dialysis | Urgency of surgery |
Previous endocarditis | Blood values |
Congestive heart failure | Haemoglobin |
Number of reoperations | NT-pro-BNP |
Comorbidities | BNP |
Diabetes | Creatinine |
Cerebrovascular disease | Medications |
Peripheral vascular disease | β-Blocker |
Atherosclerotic aortic disease | Non-aspirin platelet inhibitor |
Atrial fibrillation | Inotropic support |
Arrhythmia | Gene mutations |
Hypertension | Il-8-251AA |
Pulmonary function/disease (e.g. COPD) | Catechol-O-methyltransferase LL |
Pulmonary hypertension | |
Renal function/failure | |
Post-traumatic stress disorder | |
Depression | |
Liver function/failure | |
Malignancy | |
Dyslipidaemia/hypercholesterolaemia | |
Hyperglycaemia |
BNP: brain natriuretic peptide; CCS: Canadian Cardiovascular Society; COPD: chronic obstructive pulmonary disease; CVA: cerebrovascular accident; MI: myocardial infarction; NT-proBNP: N-terminal-pro-brain natriuretic peptide; NYHA: New York Heart Association; PCI: percutaneous coronary intervention; TIA: transient ischaemic attack.
DISCUSSION
In this systematic review, we screened 5768 studies and included 844 studies in which we identified relevant independent predictors of death, stroke, renal failure and length of stay after adult cardiac surgical procedures. This study was the first to identify systematically all predictors of adverse events after CABG and/or valvular surgery in adults. Many risk factors with a significant impact are frequently not considered when evaluating patients for major invasive procedures. Decision-making may be improved by taking into account these neglected yet predictive risk factors. Beside demographics (e.g. age, gender), disease complexity (e.g. coronary and/or valve lesions) and comorbidities (e.g. renal failure), other factors such as medication intake and the patient's psychiatric, mental and social-economic status have also been shown to have a predictive power [16, 17].
Over the last decade(s) there has been a growing interest in risk-prediction models both for monitoring innovations and benchmarking outcomes as well as for clinical use to multidisciplinary shared-decision making. The latter is especially true in an era of expanding multimodality therapy for coronary artery and aortic valve disease when risk prediction plays an important role in determining which patients would benefit most from surgery or interventional therapy [18].
The inaccuracy of risk models may in part be due to the selection of variables [18]. As shown by previous studies, risk models are inconsistent in including variables and are missing several different yet important risk factors [19, 20], although until now it has been unclear which factors need to be considered. Furthermore, different definitions are used for some of the risk factors, resulting in a different weighting of that factor between models. Collection of the variables identified in this study may help to improve future risk models, and standardize the risk factor definitions best suitable for inclusion.
A number of studies have identified genetic variations or mutations that carry an increased risk of adverse events after cardiac surgery. Indeed, collection of these variables in a large database could potentially provide insights into the understanding of the patient's risk, but it might be too optimistic to apply genetic profiling to a large international database. Costs of sequencing technologies are decreasing, but genetic profiling is still not widely used. It will be interesting to see whether genetic phenotyping might be more suitable to identify patients at higher risk of adverse events [21], although little evidence is available at this time to use this technique for risk stratification in cardiac surgery. Some of the laboratory values or echocardiographic measures that have shown to be independent predictors may be too costly to collect. Quality of life assessments are time-consuming activities that will need to be performed by educated research nurses. Therefore, a model will always be lacking some variables that could potentially increase its performance.
The balance between the number of variables and model performance should be carefully considered when developing a risk model. Although many variables may be predictive (Tables 2–5), they cannot all be included because this will decrease the user-friendliness of the model [22]. Furthermore, a great number of variables will likely result in missing data that will have a negative impact on the accuracy of a newly developed risk model. On the other hand, ignoring some of these variables may produce a model with modest performance at best. It is recommended to exclude only variables with little impact on the predictive value of the model. Factors must be relatively present in the population, and enough adverse events must occur in a frequent manner to be able to have adequate power for each risk factor to weight it in a multivariate model. Factors that are only present in a very small minority (<1%) of patients may not be relevant to collect, although their relative weight may be high. Ideally, the impact of the identified risk factors would be used to select which factors are more important to collect than others. However, to obtain an accurate estimate of the impact on the model, a broad range of risk factors need to be collected—including (non-)conventional factors—in a large database. Only then can unnecessary risk factors be excluded. Collection of these factors will furthermore identify specific factors with international variation in prevalence or dynamic effect weights, which might result in a different or a changing impact of factors on short- and/or long-term risks [23].
It is unrealistic to collect, for each patient, the hundreds of variables that were identified in this study. It might be appropriate to start data collection with a small selection of centres as a feasibility project. This helps to determine the relative impact of certain variables and whether it is necessary and possible to collect these on a larger scale. Nevertheless, even in a feasibility design, there are variables that may need to be prioritized over others. This study provides a framework for future model development, from which certain variables can be chosen depending on the prevalence of a risk factor, its relative impact, the patient population, the type of model (e.g. short- or long-term), the end-points for which the model is developed and the cost and resources available.
Risk models that have been developed on a cohort of patients undergoing specific procedures may have limited value when applied to other population groups, as the impact of any one variable can have a very different weighting when applied to a cohort of patients undergoing another procedure. This may also be one of the reasons why risk models fail to predict accurately outcomes of low- to high-risk patient cohorts. This is clearly evident when examining the predictive power of the original EuroSCORE. It was developed on relatively low-risk patients undergoing CABG [24] but subsequently has been widely used with limited value for high-risk AVR, probably because such patients were hardly represented in the EuroSCORE database.
The EuroSCORE II was developed with 22 381 patients of whom 46.7 and 46.3% underwent isolated CABG and valve procedures, respectively [5]. However, recent evidence suggests that this more-balanced inclusion of procedures was at the expense of decreased model performance in isolated CABG procedures [8]. Although generic risk models are useful in describing the risk profile of large patient populations included in randomized clinical trials or registries, procedure-specific models for CABG, AVR and mitral valve surgery are advocated to increase risk prediction for individual patients. Clearly, some of the risk factors we identified will more likely be included in a CABG risk model while others are more specific for an AVR model, such as the SYNTAX score or prosthetic valve endocarditis, respectively. The predictive power of some factors remains unclear when evaluating a cohort of patients undergoing a specific procedure, which is why there is a need to collect these factors in a generic database. This will furthermore provide the opportunity to examine whether useful generic models with procedure-related interaction terms can be constructed or whether only procedure-specific models are required for accurate risk prediction.
One major limitation of the widely used European risk scores remains that they have been developed to predict operative mortality, although this is not the only outcome of interest to patients, health care systems or policy makers. Many variables predictive of death will also be significant for other outcomes including renal failure, stroke and length of stay. However, the associated odds ratios might be different for specific outcomes. For example, in the STS model for isolated valve surgery, the OR of active infectious endocarditis for mortality is 1.95 (95% CI 1.68–2.27) but 2.79 (95% CI 2.51–3.09) for prolonged length of stay [4]. One of the goals of the forthcoming EACTS risk model will be to develop a model able to predict accurately multiple outcomes using outcome-specific ORs, similar to the STS risk model.
Although risk models can be improved, random events will always occur and a prediction model can therefore never be perfect. Thus, clinical guidelines recommend that clinical decision-making related to interventional and surgical interventions should be performed by a multidisciplinary Heart Team that consists of at least an interventional cardiologist and cardiovascular surgeon to interpret and weight risk models and additional information to come up with the most appropriate treatment recommendation for the individual patient [25].
Limitations
The focus of this study was adult patients undergoing CABG and/or valve surgery, because the available surgical risk models have predominantly been developed for these populations. Although there may indeed be significant overlap, the identified independent risk factors may not be applicable to other surgeries such as on the aortic root or aorta, congenital cases or heart transplantations.
CONCLUSION
This systematic review identified a significant number of independent predictors of adverse outcomes after adult coronary and valvular procedures, many of which are frequently not considered. These variables will be collected in a dedicated European database, and used for the development of the forthcoming EACTS risk model. However, the clinical value of these risk factors needs to be weighed against the cost and effort of collecting them.
Conflict of interest: none declared.
REFERENCES
APPENDIX. CONFERENCE DISCUSSION
Dr M. Mack(Dallas, TX, USA): So your hypothesis is that risk algorithms are inaccurate as currently constructed, and you have reviewed 884 articles for candidate variables. You are proposing to look at four outcomes, death, stroke, renal failure, and length of stay, and you have come up with over a hundred candidate variables in nine different domains.
Now, while I appreciate that you are trying to be comprehensive and casting a wide net to begin with, at some point you have to balance accuracy, comprehensiveness, and user friendliness. If you look at the current STS risk modelling for CABG, there were 45 candidate variables considered and 29 finally chosen. If you look at the valve risk algorithm, it varies between 10 covariates for sternal wound infection, up to 24 variables for major mortality and morbidity.
To be a candidate variable, as you have alluded to, first of all you have to collect it. So if you do not collect it, you cannot consider it. Secondly, you have to collect it completely, relatively accurately, and it has to be relatively current. And it has to occur with enough frequency in your sample so that it can be accurate. But at some point you have to balance completeness and accuracy with user friendliness, otherwise you are going to lose accuracy.
So my question is, how much more do you think you can improve on the current calibration by adding additional variables to what we are doing? And if you are going to add additional variables, what do you think would be the variables that occur frequently enough so that the impact would be enough for initial consideration? Would it be frailty? Would it be liver disease?
Dr Head: Well, first of all, I agree with what you say. It is a problem, of course, if you have 150 risk factors, that you need to take into account in collecting them and putting that into a database, and clearly there are a lot of costs involved with that as well.
Concerning the risk model, the problem with some of the variables that I have just mentioned is that they have never been collected very thoroughly in large databases. So once you have everything in the database, you can actually look at the relative impact they have against each other. So while you may at first think that some variables have a clear association with postoperative outcomes, they may not because of the interaction with other factors that you have collected. So if you have a large database and build the model, that is actually the first time that you can look at the factors relatively and include them step-wise into your model and see whether they actually improve the c-statistic, for instance, of a model. If you have not done that, you cannot be 100% sure whether variables need to be collected or not. I hope that answers your question.
Dr Mack: It does, but the more you collect, the less accurate it is going to be and the more burdensome it is going to be. So, for instance, for the STS CABG, there were 45 variables considered and 29 sorted out as being relevant. At what point do you say, you know what, it is so burdensome, and we really cannot expect to increase the accuracy of the calibration, that it is not worth doing? I realize that is not an answerable question, but that is kind of what you are setting out to do. But I think that one has to strike a balance in all of this.
Dr Head: Yes, that is true. And once you have a fairly good model and you add variables, that is probably not going to increase the value of the risk score. So at one point you will have to say, okay, this is the best you can do, and you have to exclude a lot of the variables. But again, once you have all variables, it is actually the only way to systematically determine which variables are important. This is not only important for risk scoring, but also if you look at a heart team. I mentioned that the guidelines recommend taking into account risk scores, but also other factors that are not in risk scores. So even though they may not be included in the risk model, there are factors that you may need to take into consideration when you are evaluating therapies and talking to patients about what therapy you think is best for them.
Dr Mack: At least today, because then you have to get into the concept of dynamic risk modelling because what may not matter today may matter tomorrow and vice versa. So it constantly has to be revisited and reanalysed and recalibrated.
Dr Head: And hopefully a database that is large enough with many variables can help with that. And that is what we are setting out to do through the Quality Improvement Programme of cardiac surgery in Europe as well.
Dr S. Siregar (Utrecht, Netherlands): So how feasible do you think it is to collect 140 variables for each patient when it is already difficult to collect, for example, EuroSCORE II variables or the STS variables?
Dr Head: Yes, that is one of the major issues, of course. But if you look at, for instance, the TVT registry in the United States that evaluates transcatheter aortic valve replacement, there is actually a CRF of seven pages long, and as far as I have heard, it is going well. And, of course, there are a lot of costs associated with collecting those variables as well. The problem with that is that maybe those variables you are collecting are not going to be included in the model and apparently may not be as predictive as you thought they would be. But again, once you have them in the database, you can finally conclude that. But there are not 150 centers that will be collecting 150 variables. Hopefully we will start with a small group and be able to grow from that and see how feasible it is and maybe improve the concept. We may already see which variables are obligatory to collect and focus on the ones that we think are actually necessary.
Author notes
Presented at the 26th Annual Meeting of the European Association for Cardio-Thoracic Surgery, Barcelona, Spain, 27–31 October 2012.