-
PDF
- Split View
-
Views
-
Cite
Cite
Alessandro Brunelli, Richard G. Berrisford, Gaetano Rocco, Gonzalo Varela, on behalf of the European Society of Thoracic Surgeons Database Committee, The European Thoracic Database project: composite performance score to measure quality of care after major lung resection, European Journal of Cardio-Thoracic Surgery, Volume 35, Issue 5, May 2009, Pages 769–774, https://doi.org/10.1016/j.ejcts.2009.01.037
- Share Icon Share
Abstract
Background: Performance measurement is an essential element of quality improvement initiatives. The objective of this study was to develop a composite performance score (CPS) incorporating processes and outcomes measures available in the European Society of Thoracic Surgeons (ESTS) Database and apply it to stratify performance of participating units. Methods: A total of 1656 major lung resections for malignant primary neoplastic disease were collected in the ESTS database from 2001 through 2003 and were analyzed. For the purpose of this study only data collected from units contributing more than 50 consecutive cases were included. Three quality domains were selected: preoperative care, operative care, and postoperative outcome. According to best available evidence the following measures were selected for each domain: preoperative care (% of predicted postoperative carbon monoxide lung diffusion capacity (ppoDLCO) measurement in patients with predicted postoperative forced expiratory volume in one second (ppoFEV1) <40%), operative care (% of systematic lymph node dissection), and outcomes (risk-adjusted cardiopulmonary morbidity and mortality rates). Morbidity and mortality risk models were developed by hierarchical logistic regression and validated by bootstrap analyses. Individual processes and outcomes scores were rescaled according to their standard deviations and summed to generate the CPS, which was used to rate units. Results: CPS ranged from −4.4 to 3.7. Individual scores were poorly correlated with each other. Two units were negative outliers and two positive outliers (outside 95% confidence limits). Compared to the rating obtained by using the risk-adjusted mortality rates, all units changed their positions when ranked by CPS. Conclusions: The composite performance score methodology may support future peer-based organizational quality benchmarking initiatives and may be used for regulatory and credentialing purposes.
1 Introduction
Performance measurement is an essential element of quality improvement initiatives and health care reform.
Until recently, quality improvement has focused on short-term outcomes (i.e. operative mortality and morbidity). However, it is recognized that such clinical outcomes are only one measure of overall health care quality [1,2]. More comprehensive quality indicators include intraoperative and perioperative processes of care that impact hospital outcomes, secondary prevention, and long-term health.
Like intelligence or musical ability, quality is an abstract construct that cannot be measured directly and it is characterized by one or more latent (unobserved) variables or traits [3]. To quantify abstract constructs we typically rely upon some combination of measurable surrogates that are thought to be associated with or contribute to that underlying trait.
The European Society of Thoracic Surgeons (ESTS) Database Committee and Audit and Clinical Excellence ad hoc Committee were appointed to devise and implement instruments of performance monitoring across European thoracic surgery units. A European Thoracic Database was used to collect thoracic procedures from European units to provide a platform for quality management initiatives. Although a risk model for in-hospital mortality after lung resection (ESOS) was developed, validated and applied to this purpose [2,4], mortality provided only limited information about individual providers’ performance and more comprehensive and reliable quality indicators were needed.
A recent document from the STS quality measurement task force elegantly explained the conceptual framework and the statistical consideration in the development of composite performance scores (CPSs) in cardiac surgery [3,5].
Based on that methodology, we have developed a composite performance score for lung surgery. The objective of this analysis was to generate a composite score from the data available in the European Thoracic Database to provide an instrument for performance monitoring for quality improvement, regulation and credentialing.
2 Patients and methods
Data was prospectively captured from the European thoracic surgery database (version 1) on thoracic surgical procedures from units across Europe. The database was designed to contain a minimum set of core variables and endpoints. Twenty-seven units from 14 European countries consistently submitted data from January 2001 through December 2003 without external local data audit. Data was scrutinized for possible inconsistencies and missing values to improve quality of data in the context of a voluntary database.
One thousand, six hundred and fifty six patients submitted to major lung resections (1281 lobectomies and 375 pneumonectomies) for malignant primary neoplastic disease in the 10 units contributing more than 50 consecutive cases to the European database (period 2001–2003) were analyzed. These cases represent approximately 70% of the total major lung resection in the database.
We selected three quality domains assessing preoperative care (process indicator), operative care (process indicator), postoperative care (outcome indicator). Based on the best available evidence (the integration of the best research evidence with clinical expertise and patient values [6]) the following indicators were selected.
2.1 Process indicators
Based on published guidelines,
The proportion of patients with predicted postoperative carbon monoxide lung diffusion capacity (ppoDLCO) estimated in the eligible group with a low predicted postoperative forced expiratory volume in one second (ppoFEV1) (defined as ppoFEV1 <40%) [7,8].
The proportion of patients with a systematic lymph nodes dissection according to ESTS published guidelines [9].
2.2 Outcome indicators
In-hospital mortality.
In-hospital cardiopulmonary morbidity (pneumonia, atelectasis requiring bronchoscopy, adult respiratory distress syndrome, mechanical ventilation longer than 24 h, pulmonary edema, pulmonary embolism, myocardial ischemia, cardiac failure, arrhythmia, stroke, acute renal insufficiency).
These two outcome indicators were risk-modeled as follows. An initial screening of preoperative and operative variables (age, gender, predicted postoperative forced expiratory volume in one second in percent of predicted normal values – ppoFEV1, type of operation – (lobectomy or pneumonectomy), presence of extended resection (lung resection associated with resection of chest wall, diaphragm, pericardium, or other mediastinal structures)) was performed by univariate analyses using unpaired Student’s t-test or Mann–Whitney test for numerical variables (with or without normal distribution, respectively) and the chi-square test or Fisher’s exact test whenever appropriate for categorical variables. Significant variables (p < 0.1 at univariate analysis) were then used as independent predictors in hierarchical regression models. Hierarchical or multilevel random-effect multivariate regression analysis takes into account the between-provider and within-provider variations. Provider-specific estimates are shrunken towards the mean for all providers and this methodology has been shown to provide a more reliable estimate of a single provider performance [10,11] by better reflecting the true mortality rate of low volume providers and accounting for clustering (non-random allocation) of observations within providers [12,13]. The regression models were further validated by bootstrap analyses with 1000 samples. In the bootstrap procedure, repeated samples of the same number of observations as the original database (1656) were selected with replacement from the original set observations. For each sample, hierarchical multivariate regression was performed entering the variables with p < 0.1 at univariate analysis. The stability of the final model can be assessed by identifying the variables that enter most frequently in the repeated bootstrap models and comparing those variables with the variables in the final model. If the final model variables occur in a majority (>50%) of the bootstrap models, these are judged to be stable [14–16].
Finally, risk-adjusted morbidity and mortality rates were calculated for each unit by dividing the observed to the predicted outcome multiplied by the mean observed outcome rate in the total population. Risk-adjusted outcome rates (morbidity or mortality) are regarded as the outcome rates a unit would have if its case-mix would be similar to the average case-mix in the entire population.
2.2.1 Determination of final composite score
Final composite score combined the two process measures (percent of ppoDLCO in patients with ppoFEV1 <40%, and percent of systematic lymph nodes dissection in patients with primary lung cancer) with the two risk-adjusted outcome measures (mortality and cardiopulmonary complications rates) into a single comprehensive quality score.
To assure consistent directionality (increasingly positive values reflecting better performance), mortality rates were converted to survival rates (risk-standardized survival rate = 100 − risk-standardized mortality rate), and morbidity rates were converted to ‘freedom from morbidity’ rates (risk-standardized freedom from morbidity rate = 100 − risk-standardized morbidity rate).
Account was taken of the differing measurement scales of the domain-specific scores. Theoretically, each measure has the same scale, 0–100%, in reality, measurement scales differ dramatically. Process adherence rates are widely dispersed and range from close to 0% to almost 100% (a range of 100%). In contrast, risk-standardized survival rates are tightly clustered in a narrow interval ranging from about 95% to 99% (a range of 4%). To account for these differences, the scales of measurement were standardized by the reciprocal of their standard deviations before the individual domain scores were combined into an overall composite score (rescaled score = original score − average score of the entire population/standard deviation of the entire population). This rescaling method was applied to all the outcome and process indicators before summarizing the final composite score of each unit.
All data were at least 95% complete. All patients with postoperative events had complete data. Missing data were imputed by averaging the non-missing values for numerical variables and by taking the most frequent category for categorical variables. All the statistical tests were two-tailed and a significance level of 0.05 was accepted. The analysis was performed by using the STATA 8.2 (Stata Corp., College Station, TX) statistical software.
3 Results
The characteristics of patients operated on in the 10 units analyzed in this study are shown in Table 1 along with the individual morbidity and mortality rates.


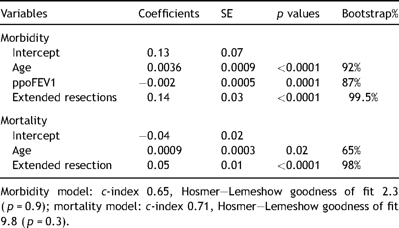
Results of the multilevel regression analyses (dependent variables: in-hospital morbidity and mortality). Parsimonious models.
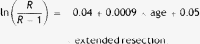
Table 3 summarizes the process and outcome rates in each unit in the study. The average rates of DLCO measurement (in patients with ppoFEV1 <40%), systematic lymph nodes dissection, probability of non-complication and probability of survival in the entire population were 16.3% (SD 31.6), 56.7% (SD 37.8), 80.6% (SD 10.9), 97.5% (SD 2.1), respectively. From a quality management perspective, the different indicators are associated with each other in such a ratio that a 1% decrease in mortality corresponds to 5% decrease in complications, 19% increase in systematic nodal dissection and 16% increase in DLCO measured in pts with marginal ppoFEV1.
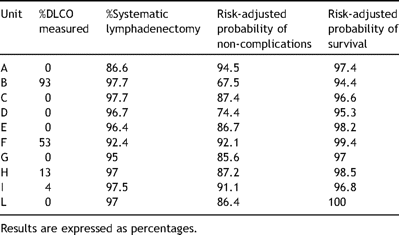
Process and risk-adjusted outcome measures in each unit under analysis.
Composite scores were generated by adding the rescaled individual process and outcome scores of each unit, and they ranged from −4.4 to 3.7 (Table 4 ). The correlation coefficients between the individual four rescaled scores (processes and outcomes) were all below 0.5, with the exception of non-complication score and survival score (0.68).
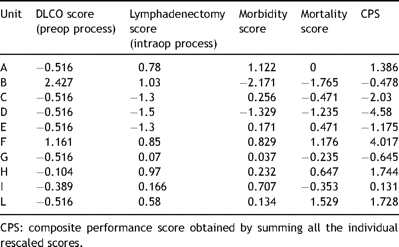
Summary of the individual rescaled process and outcome scores in each unit.
Fig. 1 depicts the distribution of the units rated by their CPS. When the 95% confidence limits threshold was used as rating method, two units were negative outliers and two were positive outliers. By using the CPS all units changed their position compared to the rank obtained by using the mortality alone (Table 5 ). One unit moved from the lower half to the upper half of the rating panel and one from the upper half to the lower half. Forty percent of units moved two or more positions.

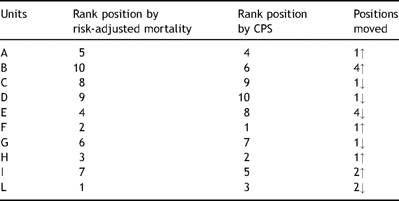
Rating of units by their composite score and, by reference, their risk-adjusted mortality rates.
4 Discussion
The current climate of public accountability, financial restraints, pay-for-performance and ranking culture demands that performance of health care providers be monitored through reliable instruments. In our specialty outcome endpoints are still the most widely used quality indicators [2,4,17–21], however these may be quite insensitive to quality, require long-term evaluation to aggregate the sufficient number of events for a reliable statistics and fail to provide immediately actionable information to providers [1].
More comprehensive quality indicators are needed that may capture most aspects of the entire process of care and for which providers may be reliably made accountable.
The ESTS thoracic database was designed to provide a tool for a fair evaluation of performance across different European thoracic surgery units. Several reports have already been published on the development and use of a risk-adjusted mortality model derived from data collected in the database [2,4]. More recently the ESTS database committee in cooperation with the ESTS Audit and Clinical Excellence Committee and in response to the growing demand for a more reliable instrument of performance rating that may be used as a tool for credentialing and regulatory purposes decided to build a comprehensive performance score from data available in the version 1 of the European database.
Several principles underpinned the development of a comprehensive quality rating system for lung surgery:
Quality assessment should be at the level of the program or hospital rather than the individual surgeon.
Quality scores should consider structure, process, and outcomes [22].
Quality scores should assess three temporal domains: preoperative, operative, and postoperative [3].
Quality scores should be interpretable and actionable by providers.
Initial quality reports should focus on lung resection as the most representative operation in our specialty
All quality measures should be available as data elements within the European thoracic surgery database.
The use of a composite score for measuring quality of care is appealing in light of the abstract nature of the quality itself, which cannot be directly measured. Although quality improvement requires attention to each individual aspect of quality, there are many settings in which the users of quality measures are most interested in the bottom line. Thus, composite indicators seem particularly useful for summarizing and comparing the quality of care delivered by healthcare providers. This is particularly true in many surgical areas where the small sample size and the low rate of adverse events diminish the statistical utility of outcomes comparisons [23]. Thus, composite indicators may provide a quantitative basis for clinicians, organizations, and planners aiming to achieve improvement in care and the process by which patient care is provided. They may serve many purposes: document the quality of care, make comparisons (benchmarking) over time between providers or within the same provider, make judgements and set priorities (i.e. choosing a hospital or surgery; organizing medical care), support accountability, regulation, and accreditation, support quality improvement, and support patient choice of providers.
It was noteworthy that results obtained applying the CPS differed substantially from those obtained by applying a more traditional endpoint such as risk-adjusted mortality. In fact, all units changed their position in the ranking and 40% moved two or more positions. This may have important administrative and managerial implications.
This score is far from being perfect and future refinements in data collection and the inclusion of new outcome or process indicators are needed to improve its reliability and precision. A mechanism for independent validation of the completeness and accuracy of each center’s data was not present and should be implemented. It would be desirable to include multiple processes within each domain in order to assess the quality of care in a more comprehensive manner. Unfortunately, we were limited to those process indicators that were present in the first version of the European database. A second version of the database has been recently launched that could allow the inclusion of multiple evidence-based process indicators (https://www.thoracicdata.org).
For a composite score to be valid, there must be a strong link between processes and outcomes that can come from previously published evidence. This prior evidence supporting the link between process and outcomes may be weak or non-existent for many processes even when they are truly linked to outcomes.
We did not include structural indicators in the CPS. However, a structural indicator is very often regarded as a surrogate in the absence of valid risk-adjusted outcome endpoints. Furthermore all units under analysis participated to the database, which was considered per se a structural indicator common to all centers. Each individual indicator reflects a different aspect of quality. Therefore, some information is invariably lost when only a single summary score is reported, particularly when measures are poorly correlated and non-redundant.
We recognize that some important areas of performance may not be addressed or may be relatively undervalued, and aggregation may also obscure individual areas of strength or weakness. The ability to decompose the composite into its individual components is critical. This allows providers to analyze their performance in specific areas and to formulate improvement strategies [5]. The CPS as developed in this analysis can be easily decomposed and the units may be evaluated according to their individual scores related to preoperative, operative and postoperative domains.
Determining a set of valid exclusion criteria for process measures has proved challenging in many areas of medicine, and information on contraindications to various process measures is absent in the European database. As a result, some patients who are appropriately denied a practice owing to a contraindication will be misclassified as representing a process failure. The true overall percentage compliance with process measures will therefore be underestimated because some ineligible patients are included. Limitations also exist with respect to various outcome measures. Because certain outcomes (morbidity) are difficult to define precisely, it is possible that variation in coding practices could account for some of the observed differences between providers.
The encouraging results of this study lead us to consider future implementations in agreement with similar conclusions reached by the STS task force for quality measurement in cardiac surgery [5]:
construction of separate composite scores for processes and outcomes, assessing their degree of congruence, and studying each separately to determine their ability to discriminate overall performance,
consideration of new or revised measures that provide important incremental information about overall care,
ongoing assessment of the relationship between process measures and short- or long-term outcomes, with potential elimination of process measures that demonstrate limited clinical effectiveness,
monitoring provider-specific scores longitudinally to assess their consistency, and to study which domains and individual measures appear most predictive of subsequent provider performance.
if composite scores are to be used for a rating system, choices must be made regarding the use of an internal or external benchmark, the number of rating tiers, the cut points used to define each tier, and the statistical criteria used to assign providers to tiers.
research is needed to identify the optimum time window for estimating and reporting performance, and the methods for dealing with underperformance.
The availability of a reliable rating system may make possible to implement peer-based, provider-initiated, organizational quality initiatives [24,25], such as the creation of European thoracic quality benchmark program. Those units participating in the database will be subject to scoring and rating on a voluntary, non-coercive, non-punitive, co-operative way. Their performance will be reviewed annually during a benchmark meeting lead by ESTS database representatives. Rating will be confidentially revealed and an in-depth analysis of individual critical pathways of care reviewed in the outlier’s centers. Possible visits to individual centers of high performance may be scheduled and made available to representatives of units in the lower performance tiers in order to gain insights for improving their activity. The success of such an initiative may be evaluated by longitudinal review of performance on a yearly basis. The main objective of this program would be to globally improve the quality of the entire European thoracic surgery community and to apply clinical governance mechanisms that may protect our profession from the increasing public and forensic scrutiny.
It must be emphasized that the aim of the present project was to provide an example of methodology on how to construct and use a composite performance score in our specialty and in the context of multicenter database and not to provide a ready-made instrument for quality assessment.
In conclusion, based on data and variables from the European Thoracic Database, a composite performance score, incorporating process and outcome measures of quality, has been developed, with the ultimate intent to provide a methodological template for implementing instruments for a reliable provider profiling and performance monitoring. Future refinement is needed in order to apply this tool for international quality initiatives and regulatory and credentialing purposes.
Presented in part at the Postgraduate Course of the 16th European Conference on General Thoracic Surgery, Bologna, Italy, June 8–11, 2008.
References
- quality of care
- lung
- carbon monoxide
- forced expiratory volume function
- benchmarking
- credentialing
- expressed sequence tags
- preoperative care
- morbidity
- mortality
- neoplasms
- thoracic surgery specialty
- lung volume reduction
- lymph node dissection
- diffusion capacity
- quality improvement
- surgical outcome
- performance measurement