-
PDF
- Split View
-
Views
-
Cite
Cite
Pedro E. Antunes, José Ferrão de Oliveira, Manuel J. Antunes, Risk-prediction for postoperative major morbidity in coronary surgery, European Journal of Cardio-Thoracic Surgery, Volume 35, Issue 5, May 2009, Pages 760–768, https://doi.org/10.1016/j.ejcts.2008.10.046
- Share Icon Share
Abstract
Objective: Analysis of major perioperative morbidity has become an important factor in assessment of quality of patient care. We have conducted a prospective study of a large population of patients undergoing coronary artery bypass surgery (CABG), to identify preoperative risk factors and to develop and validate risk-prediction models for peri- and postoperative morbidity. Methods: Data on 4567 patients who underwent isolated CABG surgery over a 10-year period were extracted from our clinical database. Five postoperative major morbidity complications (cerebrovascular accident, mediastinitis, acute renal failure, cardiovascular failure and respiratory failure) were analysed. A composite morbidity outcome (presence of two or more major morbidities) was also analysed. For each one of these endpoints a risk model was developed and validated by logistic regression and bootstrap analysis. Discrimination and calibration were assessed using the under the receiver operating characteristic (ROC) curve area and the Hosmer–Lemeshow (H–L) test, respectively. Results: Hospital mortality and major composite morbidity were 1.0% and 9.0%, respectively. Specific major morbidity rates were: cerebrovascular accident (2.5%), mediastinitis (1.2%), acute renal failure (5.6%), cardiovascular failure (5.6%) and respiratory failure (0.9%). The risk models developed have acceptable discriminatory power (under the ROC curve area for cerebrovascular accident [0.715], mediastinitis [0.696], acute renal failure [0.778], cardiovascular failure [0.710], respiratory failure [0.787] and composite morbidity [0.701]). The results of the H–L test showed that these models predict accurately, both on average and across the ranges of patient deciles of risk. Conclusions: We developed a set of risk-prediction models that can be used as an instrument to provide information to clinicians and patients about the risk of postoperative major morbidity in our patient population undergoing isolated CABG.
1 Introduction
Evaluation of patient outcomes has become increasingly accepted as one important step to assess and improve quality of patient care. Because differences in outcomes may result from disease severity, effectiveness of treatment, or chance [1,2], and most outcome studies are observational rather than randomised, risk adjustment is necessary to account for case-mix. In this context, risk-prediction models play an important role in current cardiac surgical practice where they may be used to assess the impact of specific predictors on outcome, to aid in patient counselling and treatment selection, to profile provider quality, and to serve as the basis for continuous quality improvement [3].
Risk models for mortality after cardiac surgery are widely used, but the application to populations other than those for which they were developed may not be adequate. In a previously published study, we developed and validated a risk model for in-hospital mortality in patients submitted to isolated coronary artery bypass graft (CABG) procedures in our institution [4]. But although operative mortality is obviously the most important clinical endpoint, mortality alone is no longer considered sufficient to assess patient outcomes. It is clearly recognised that other non-fatal postoperative complications can significantly impact patients’ functional status and quality of life [5]. Therefore, identification of factors and calculation of risk-adjusted morbidity rates for CABG procedures may provide valuable insights on areas to focus for improved quality of care.
In this work, we aimed at identifying the preoperative risk factors for perioperative morbidity in patients submitted to isolated CABG, using a detailed dataset with information on a large group of patients undergoing this procedure at our institution, and to develop and validate risk-prediction models for the most important postoperative morbidity complications.
2 Materials and methods
2.1 Study design and population
We performed a retrospective cohort study of patients undergoing CABG during a 10-year period, from January 1992 through December 2001. Only patients undergoing isolated first operations and reoperations were included. Patients undergoing CABG combined with heart valve repair or replacement, resection of a ventricular aneurysm, or other surgical procedures were excluded. After exclusions, the material for this study’s risk modelling analyses consisted of 4567 patient records. There were 4030 men (88.2%) and 537 women and the mean age was 60.6 ± 9.2 years (median 62 years). The mean number of grafts per patient was 2.8 ± 0.8 and the mean cardiopulmonary bypass time was 63.3 ± 22.9 min. The left internal mammary artery (IMA) alone and double AMI were used in 99.5% and 23.4% of the study population, respectively. All operations were performed without cardioplegia, under hypothermic ventricular fibrillation or empty beating heart, a technique described in detail in previous reports from our institution [6,7]. The mean hospital stay was 7.9 ± 6.4 days (minimum, 0 days; maximum, 204 days; 25% quartile, 6 days; median, 7 days; 75% quartile, 7 days), and, in general, survivors were discharged to their home. No use was made of aftercare institutions.
2.2 Data collection
Data had been collected prospectively from each patient on a standardised written form by the respective surgeon, and validated and inputted in a computerised database by one of us (PEA). Supervision for data consistency was performed by the project co-ordinator (MJA) and aggregate outputs were periodically cross-checked against an independent clinical database. The data collection instruments included questions regarding demographic characteristics, preoperative risk factors, previous interventions, preoperative cardiac status, cardiac catheterisation results, intraoperative management and postoperative complications.
2.3 Morbidity endpoints
Five major postoperative morbidity complications, considered either life threatening or potentially resulting in permanent functional disability, were selected. They appear to be the most uniformly reported by other investigators: cerebrovascular accident, mediastinitis, acute renal failure, cardiovascular failure and respiratory failure. A composite morbidity outcome (presence of more than one major morbidity) was also considered an endpoint for this study. Definitions of these variables are presented in Appendix B. All morbidity complications were analysed as events occurring during hospital stay, unlimited in time, with exception of mediastinitis which was analysed as a 30-day event.
2.4 Methods of analysis
More than 50 preoperative patient variables were available in the database, of which 23 potential risk factors were chosen, based on univariate screening, clinical knowledge and previous research. The risk factors selected for analysis are listed and defined in Appendix C and include: age, gender, body mass index, body surface area, hypertension, diabetes mellitus, recent smoking, peripheral vascular disease, cerebrovascular disease, renal failure, serum creatinine level, anaemia, chronic obstructive pulmonary disease (COPD), cardiomegaly, recent myocardial infarction, unstable angina, Canadian Cardiovascular Society (CCS) class II/III, non-elective surgery, previous cardiac surgery, left ventricular dysfunction, left main disease, three-vessel disease and need for intra-aortic balloon pumping.
The entire database was initially used to develop the predictive logistic models. Univariate screening of all model-eligible risk factors was performed using unpaired Student‘s t test or the Mann–Whitney test for numeric variables, and the χ2 test or Fisher’s exact test for categorical variables. Multi-collinearity among variables was obviated by using only one of a set of variables with a correlation coefficient greater than 0.5 in the regression analysis. Variables with a p value lower than 0.2 by univariate analysis were used as independent variables for further analysis.
A multivariate stepwise logistic regression analysis was then performed for each of the six dependent morbidity groups: (1) cerebrovascular accident (2) mediastinitis (3) acute renal failure (4) respiratory failure (5) cardiovascular failure and (6) the composite morbidity. Because of the relatively small effective sample size of some events (respiratory failure and mediastinitis), a p value less than 0.1 was selected for variable retention in the final regression model. Bootstrap analysis was used in combination with logistic regression analysis to select the final set of risk factors included in the model. In the bootstrap procedure, 200 samples of 4567 patients (the same number of observations as the original database) were sampled with replacement. A stepwise logistic regression analysis was applied to every bootstrap sample. If the predictors occurred in more than 50% of the bootstrap models, they were judged to be reliable and were retained in the final model. Unreliable variables, if present, were removed from the final model. Thus, the risk factors were not only identified as statistically significant by traditional analysis, but also occurred the most frequently in the bootstrap analysis. The tables of risk factors include frequency of occurrence from multivariable bootstrap modelling, as well as conventional magnitude and certainty of association.
Finally, we validated the risk-prediction model internally by randomly drawing 200 samples, each containing 100% of the total number of subjects. The risk-prediction model was applied to each sample to calculate an individual sample area under the ROC curve and then the mean and standard error of the mean, with 95% confidence intervals (95% CI), for all 200 ROC values.
2.5 Model performance
Two different properties were used to evaluate the predictive accuracy of the models: calibration and discrimination. Calibration, which measures the ability of the model to assign the appropriate risk, was evaluated by the Hosmer–Lemeshow (H–L) goodness-of-fit method. The H–L χ2 statistics measures the differences between expected and observed outcomes over deciles of risk. A statistically non-significant result (p value ≥0.05) suggests that the model predicts accurately on average [8]. In order to get more insight into the model performance across the ranges of patient deciles of risk, we have plotted the observed and expected events rates in these risk groups. Accurate predictions of events within each of these risk groups would suggest that the risk model is suitable for patient advice for all (low- to high-risk) patients.
Discrimination, which measures the model’s ability to differentiate among those who have or have not suffered an event, was evaluated by analysis of the area under the ROC curve [9]. If the area is greater than 0.7, it can be concluded that the model has an acceptable discriminatory power and, consequently, may be used to rank patients into treatment groups to facilitate management [10].
3 Results
3.1 Risk profile for study population and outcomes
This generally male CABG population (11.8% female) was predominantly noted to have triple-vessel disease (75.3%). The risk profile for the CABG study population is shown in Table 1 .
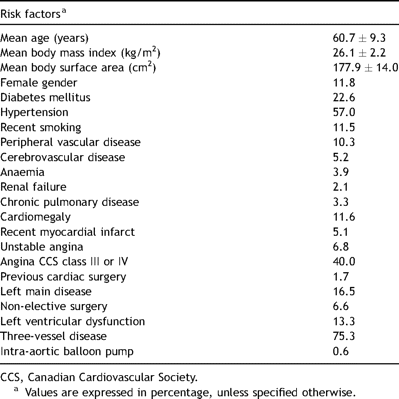
The study population had hospital mortality and major composite morbidity rates of 1.0% and 9.0%, respectively. The specific major morbidity rates were: cerebrovascular accident, 2.5%; mediastinitis, 1.2%; acute renal failure, 5.6%; cardiovascular failure 5.6%; and respiratory failure, 0.9%. The composite morbidity rate was 9.0%.
3.2 Model results
Six different risk models were developed, one for each of the five morbid events and one for the composite morbidity, and the final logistic model results are presented in Table 2 . This table summarises, for each one of the constructed models, the variables used and their frequency of occurrence (%) in bootstrap analyses, regression coefficients, odds ratio and associated p values. All the risk factors included in each model occurred in more than 50% of the bootstrap samples, indicating reliability.
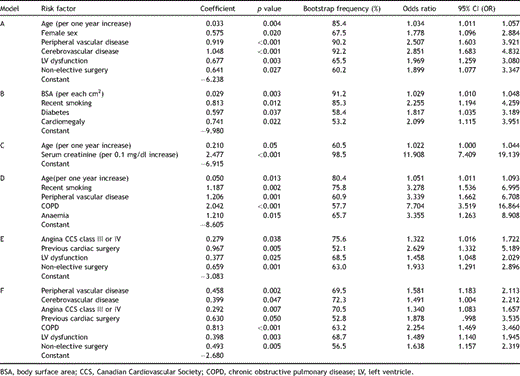
Logistic regression risk models for CABG postoperative major morbidity. (A) Cerebrovascular accident, (B) mediastinitis, (C) renal failure, (D) respiratory failure, (E) cardiovascular failure and (F) composite morbidity.
The various preoperative risk factors influenced differently each of the five morbid events. The variables found to have the most significant impact in the occurrence of morbidity were: for cerebrovascular accident: age (OR = 1.034 per one year increase), female sex (OR = 1.778), peripheral vascular disease (OR = 2.507), cerebrovascular disease (OR = 2.851), LV dysfunction (OR = 1.969) and non-elective surgery (OR = 1.899); for mediastinitis: BSA (OR = 1.029 per each cm2 increase), recent smoking (OR = 2.255), diabetes (OR = 1.817) and cardiomegaly (OR = 2.099); for acute renal failure: age (OR = 1.022 per one year increase) and serum creatinine (OR = 11.908 per 0.1 mg/dl increase); for respiratory failure were: age (OR = 1.051 per one year increase), recent smoking (OR = 3.278), peripheral vascular disease (OR = 3.339), COPD (OR = 7.704) and anaemia (OR = 3.355); and for cardiovascular failure: angina CCS class III/IV (OR = 1.322), previous cardiac surgery (OR = 2.629), LV dysfunction (OR = 1.458) and non-elective surgery (OR = 1.933).
In the final composite morbidity outcome, peripheral and cerebral vascular disease, age, angina CCS class III/IV, previous cardiac surgery, COPD, LV dysfunction and non-elective surgery were the variables which were found to have the most impact.
For all the risk models, the H–L goodness-of-fit test was not statistically significant (p ≥ 0.05). Fig. 1A–F demonstrates the calibration of the models, i.e., how well the predicted event rates match the observed rates among patient decile risk groups. As noted by the close agreement between these results, these models appear to be relatively accurate across the ranges of patient risk subgroups. These results indicate that the models predict accurately, both on average and across the ranges of patient deciles of risk, hence it is suitable for use in all (low- to high-risk) patients. The risk models developed demonstrate an acceptable discriminatory power (area under the ROC curve for cerebrovascular accident [0.715], mediastinitis [0.696], acute renal failure [0.778], cardiovascular failure [0.710], respiratory failure [0.787] and composite morbidity outcome [0.701]). The details of model performance metrics are provided in Table 3 .
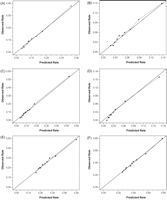
Model calibrations (A) cerebrovascular accident (B) mediastinitis (C) renal failure (D) respiratory failure (E) cardiovascular failure and (F) composite morbidity.

4 Discussion
For many years, operative mortality was the sole criterion used for evaluation of patient outcomes, and many published studies have analysed the mortality of cardiac operations, but studies concentrated on the analysis of perioperative morbidity and its influence on global early and late results are much fewer. Although operative mortality is obviously the most deleterious clinical endpoint when analysing surgical results, this is no longer considered sufficient to assess surgical/patient outcomes. It is clearly recognised that other non-fatal postoperative complications can significantly impact not only the perioperative period but also the patient’s quality of life, and may often constitute serious threats to the longer-term survival, functional capability, and overall well-being after CABG or any other cardiac surgical procedure. Therefore, identification of risk factors for increased perioperative morbidity and analysis of risk-adjusted morbidity for CABG procedures may provide valuable information which may subsequently be used to improve quality of care.
The Society of Thoracic Surgeons (STS) Quality Measurement Task Force has recently identified a group of measures to serve as the basis for comprehensive assessment of the quality of adult cardiac surgery [5]. Eleven individual measures of coronary artery bypass grafting quality were selected within four domains: (1) perioperative medical care, (2) operative care, (3) risk-adjusted operative mortality and (4) postoperative risk-adjusted major morbidity, the latter defined as the risk-adjusted occurrence of any of the following: renal failure, deep sternal wound infection, re-exploration, stroke, or prolonged ventilation/intubation. We could identify only one other study, published by Shroyer and colleagues, conducted to develop separate risk-prediction models for major morbidity events [11]. Using part of the large national experience captured in the STS database, these authors examined five postoperative CABG complications (stroke, renal failure, reoperation within 24 h after CABG, prolonged postoperative ventilation, and mediastinitis) and, for each of these, developed risk-prediction models.
However, risk models cannot be uniformly applied to different population groups, as the experience with the Parsonnet, EuroSCORE and the STS models for operative mortality has demonstrated. In a previously published study, we described the development of our own local risk-prediction model for mortality after CABG in our population, which predicted outcomes better than those widely used models [4]. In the current study, complementary outcome measures for risk-adjusted major morbid postoperative complications were developed. Five morbid events, either life threatening or potentially resulting in permanent functional disability, which appeared to be some of the most uniformly reported complications [12–16], were analysed: cerebrovascular accident, mediastinitis, renal failure, respiratory failure and cardiovascular failure. A model for composite morbidity (association of two or more major morbidity events) was also developed. Except for mediastinitis, which was evaluated as a 30-day event, the morbidity complications were analysed as events occurring during hospital stay, unlimited in time. Although it represents one of the most widely reported metrics to assess postoperative complications after CABG, it may be a too short interval for the evaluation of the true early risk. Nevertheless, and in the context of the present study, we believe that the more important issue is the ability to measure and validate it conveniently and accurately.
The main goal of our study was to identify the preoperative risk factors and to develop and validate risk-prediction models which could be used as instruments to provide information to clinicians and patients about the risk of major postoperative morbidity in our patient population undergoing isolated CABG surgery. To ensure the accuracy and usefulness of such model, many factors are essential, including selection of an appropriate clinical database, inclusion of critical variables, and proper model development and validation. The data used for this study were taken from our clinical database, which was created and is in use since the beginning of the surgical activity in this department, in 1988. In our database, some of the variables selected for analysis (ejection fraction, haematocrit, cardiothoracic ratio) were codified as categorical instead of continuous variables, which may constitute one limitation to the model building process. The risk models were developed by means of logistic regression and bootstrap analyses, which are the techniques most commonly used for risk modelling.
However, the resultant models are useful only if they reliably predict outcomes for patients by determining significant risk factors associated with the particular outcome. A problem might arise from this dependence on risk factor analysis. Different investigators evaluating the same predictors through regression analyses may obtain heterogeneous results because of methodological discrepancies and inadvertent biases introduced in the statistical elaboration [17]. Bootstrap analysis is a simulation method for statistical inference, which, if applied to regression analysis, can provide variables that have a high degree of reproducibility and reliability as risk-factors for a given outcome. We also used bootstrap analysis to internally validate the model. This methodology was recently proposed as a breakthrough method for internal validation of surgical regression models [18]. The main advantage of this technique is that the entire dataset can be used for building a more robust model, especially in moderate-size databases and for rare outcomes [19].
However, as discussed above, the risk model created may not be used in other patient populations. Local models need to be developed for use in each particular population.
Overall, the set of risk models developed in this study performed acceptably. The Hosmer–Lemeshow test was not statistically significant for all the models and demonstrated good calibration across the ranges of patient risk subgroups. These results indicate that the models predict accurately, both on average and across the ranges of patient deciles of risk, hence, are suitable for use in all (low- to high-risk) patients. The risk models developed also demonstrate an acceptable discriminatory power and, consequently, may be used to rank patients into treatment groups to facilitate management (under the ROC curve areas ≥0.7). However, the demographic and clinical characteristics presented in Table 1 may place the study population in a low-risk profile, which means that any inference of the models, namely the validity of the stability over the spectrum of risk, must be reduced to the centre where it was developed, possibly limiting the applicability to other centres.
The recognition of specific risk factors for each particular morbid event and for global morbidity permits preoperative modulation of these factors, aimed at decreasing perioperative morbidity. As our knowledge of these correlations in our specific conditions increased, we have applied measures to improve on preoperative risk factors and observed a significant decrease of morbidity rates in our patient population, which may have been a form of practical validation of the model created but have also contributed to decrease the level of morbidity.
This may be one of the weaknesses of this study. As it evolved, it influenced its own results. One other weakness is that formal external auditing was not available for our data. However, systematic supervision and periodical cross-checking, as described, captured (and corrected) a number of erroneous data inputs, however small enough to render the whole dataset satisfactorily reliable. Additionally, although we have performed a prospectively designed study, the data were collected from a single-centre database, which carries the risk that any inference may be reduced to the centre where it was developed, possibly limiting the applicability to others, as discussed above.
In conclusion, we developed a set of risk-prediction models that can be used as instruments to provide information to clinicians and patients about the risk of postoperative major morbidity in our patient population awaiting CABG surgery. It has also served to apply corrective measures which permitted improvement of our own results. Naturally, it is for our own use and is not intended for use in other patient populations. But it may facilitate comparisons with the results of other centres. Other groups are encouraged to create their own models using the methodologies applied here.
Presented at the 22nd Annual Meeting of the European Association for Cardio-thoracic Surgery, Lisbon, Portugal, September 14–17, 2008.