-
PDF
- Split View
-
Views
-
Cite
Cite
Sara M Sauer, Carole D Mitnick, Uzma Khan, Catherine Hewison, Mathieu Bastard, David Holtzman, Stephanie Law, Munira Khan, Shrivani Padayachee, Saman Ahmed, Afshan K Isani, Aga Krisnanda, Stalz Charles Vilbrun, Sagit Bektasov, Andargachew Kumsa, Wisney Docteur, Karen Tintaya, Mark McNicol, Hakob Atshemyan, Tatiana Voynilo, Thin Thin Thwe, Kwonjune Seung, Michael Rich, Helena Huerga, Palwasha Khan, Molly Franke, Estimating Post-treatment Recurrence After Multidrug-Resistant Tuberculosis Treatment Among Patients With and Without Human Immunodeficiency Virus: The Impact of Assumptions About Death and Missing Follow-up, Clinical Infectious Diseases, Volume 78, Issue 1, 15 January 2024, Pages 164–171, https://doi.org/10.1093/cid/ciad589
- Share Icon Share
Abstract
Quantification of recurrence risk following successful treatment is crucial to evaluating regimens for multidrug- or rifampicin-resistant (MDR/RR) tuberculosis (TB). However, such analyses are complicated when some patients die or become lost during post-treatment follow-up.
We analyzed data on 1991 patients who successfully completed a longer MDR/RR-TB regimen containing bedaquiline and/or delamanid between 2015 and 2018 in 16 countries. Using 5 approaches for handling post-treatment deaths, we estimated 6-month post-treatment TB recurrence risk overall and by HIV status. We used inverse-probability weighting to account for patients with missing follow-up and investigated the impact of potential bias from excluding these patients without applying inverse-probability weights.
The estimated TB recurrence risk was 7.4/1000 (95% credible interval: 3.3–12.8) when deaths were handled as non-recurrences and 7.6/1000 (3.3–13.0) when deaths were censored and inverse-probability weights were applied to account for the excluded deaths. The estimated risks of composite recurrence outcomes were 25.5 (15.3–38.1), 11.7 (6.4–18.2), and 8.6 (4.1–14.4) per 1000 for recurrence or (1) any death, (2) death with unknown or TB-related cause, or (3) TB-related death, respectively. Corresponding relative risks for HIV status varied in direction and magnitude. Exclusion of patients with missing follow-up without inverse-probability weighting had a small impact on estimates.
The estimated 6-month TB recurrence risk was low, and the association with HIV status was inconclusive due to few recurrence events. Estimation of post-treatment recurrence will be enhanced by explicit assumptions about deaths and appropriate adjustment for missing follow-up data.
Quantifying post-treatment tuberculosis (TB) recurrence is critical to evaluating the effectiveness of all multidrug- and rifampicin-resistant (MDR/RR) TB regimens during routine care. Estimating the risk of recurrence is complicated, however, when some patients die or are missing data during the post-treatment follow-up (FU) period.
Post-treatment deaths unrelated to TB preclude recurrence and constitute competing [1–3] or intercurrent [4, 5] events. Conversely, if deaths occur due to undetected TB, the risk of recurrence can be underestimated. In analyses of recurrence, assumptions are made regarding post-treatment deaths; different assumptions alter the target of estimation (ie, estimand), the appropriate analytic method, and the resulting interpretation [1]. Prior studies have typically (1) excluded patients who died [6–9], which will bias the estimated risk of recurrence in the likely scenario that people who do and do not die have different underlying risks of TB recurrence; (2) effectively assumed that none of the post-treatment deaths were due to recurrent TB [10, 11], which is not supported by the literature [12–14]; or (3) omitted details on how deaths were analyzed [15–19], which prevents a clear interpretation of the resulting estimate.
Among patients without post-treatment FU data, it is unknown whether recurrent TB occurred. Most prior studies that estimate TB recurrence have excluded individuals with missing post-treatment FU [6–8]. Valid estimates under this approach, however, require that individuals who do and do not have post-treatment FU have a similar underlying risk of recurrent TB. If there are common causes of recurrence and lack of post-treatment FU, this assumption does not hold, and simply excluding individuals who lack FU data may lead to selection bias [20, 21].
In this paper we estimated the overall risk of TB recurrence within 6 months of successful MDR/RR-TB treatment with a longer regimen containing bedaquiline (BDQ) and/or delamanid (DLM) and compared this risk by human immunodeficiency virus (HIV) status. Compared with people without HIV, those with HIV face a greater risk of unfavorable treatment outcomes, including post-treatment recurrence and death [13, 22–24]. In our analyses, we examined multiple approaches for handling post-treatment deaths and investigated the impact of selection bias from exclusion of patients without FU data.
METHODS
Data Source and Study Population
We used data from the endTB observational study, a prospective cohort of individuals who initiated a longer individualized MDR/RR-TB regimen containing BDQ and/or DLM between 2015 and 2018 in 1 of 17 countries [25]. For this analysis, we included patients' first treatment in endTB, excluded those with confirmed sensitivity to rifampicin, and restricted to patients with end-of-treatment success, defined as cure or treatment completion (Figure 1). We additionally excluded all individuals from North Korea, where post-treatment FU was not conducted.
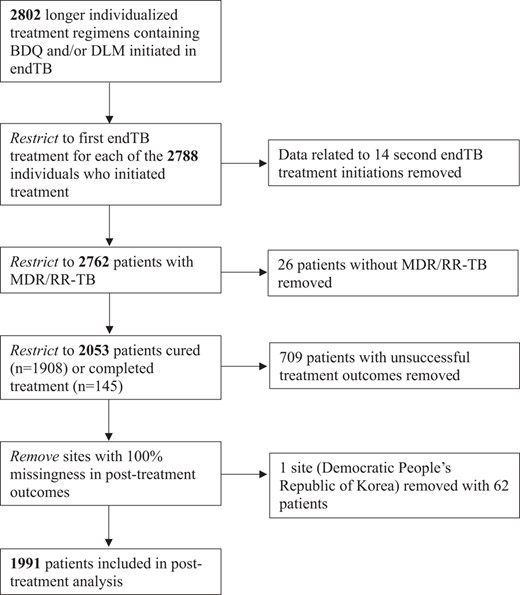
Inclusion/exclusion criteria for the post-treatment analysis. Abbreviations: BDQ, bedaquiline; DLM, delamanid; MDR/RR-TB, multidrug- or rifampicin-resistant tuberculosis.
Six-Month Post-treatment Outcomes
Post-treatment outcomes, including culture results, were recorded 6 months post-treatment and classified into the following categories: no change, post-treatment death, recurrent TB, lost to follow-up (LTFU), and not evaluated. We grouped individuals with the latter 2 outcomes, or for whom there was no post-treatment outcome record, into a single “missing FU” category. Clinicians indicated cause of post-treatment death as follows: (1) “TB immediate cause of death”, (2) “TB contributing to death”, (3) “Surgery related death”, (4) “Cause other than TB”, (5) “Cause related to TB treatment”, or (6) “Unknown”.
Defining Estimands in the Presence of Post-treatment Deaths and Missing Follow-up
We considered 5 strategies from the competing/intercurrent events literature for handling post-treatment deaths (Table 1, Figure 2), each targeting a different research question. First, in what we term the “observed recurrence” strategy, we included patients who died but did not count them as recurrences, thereby assuming that patients who died did not experience recurrent TB. In this scenario, we estimate (1) recurrence risk in the presence of deaths and (2) the association of HIV infection with TB recurrence (whether by a direct effect of HIV on recurrence or an indirect effect of HIV through death, which makes recurrence impossible) (Figure 2).

Graphical representation of the association between HIV and recurrent TB at 6 months post-treatment. In the diagram, post-treatment death temporally precedes TB recurrence; occurrence of death makes subsequent TB recurrence impossible, and the arrow from death to TB recurrence represents this deterministic relationship. The “observed recurrence” strategy targets recurrence risk and association with HIV status in the observed scenario where post-treatment deaths are not eliminated, and estimates the association between HIV status and TB recurrence agnostic of pathway (ie, estimates both paths a and b-c); the “hypothetical recurrence” strategy targets recurrence risk and association with HIV status in the hypothetical scenario where post-treatment deaths are eliminated, and estimates the association between HIV status and TB recurrence only through the direct path, a, adjusting for common factors of death and recurrent TB (L1) to eliminate bias through the b-d-e path; adjustment for the joint predictors of missing FU and recurrent TB (L2) accounts for potential selection bias through the f-g-h path; adjustment for common causes of HIV infection and recurrent TB (L3) addresses potential confounding bias by blocking the i-j path. Abbreviations: FU, follow-up; HIV, human immunodeficiency virus; TB, tuberculosis.
Approach . | Operationalization . | Assumptions . | Corresponding Strategy in ICE Literaturea . | Corresponding Causal Effectsb . |
---|---|---|---|---|
Observed recurrence | Include individuals who died in the analysis and treat as non-recurrencesc | Patients who died did not experience recurrent TB | Composite/favorable strategyd | Total effect |
Hypothetical recurrence | Censor (exclude) individuals who died, account for common causes of death and recurrence with inverse-probability weights | All common causes of death and recurrence have been accounted for | Hypothetical strategy | Controlled direct effect |
Observed composite/any | Define a composite outcome of recurrence or death from any cause | … | Composite/unfavorable strategye | Total effect on composite outcome |
Observed composite/probable | Define a composite outcome of recurrence or death with either (1) unknown cause or (2) cause related to TB; treat deaths with cause unrelated to TB as non-recurrencesc | Patients with deaths unrelated to TB did not experience recurrent TB | Composite/unfavorable strategye | Total effect on composite outcome |
Observed composite/explicit | Define a composite outcome of recurrence or death with cause related to TB, treat deaths with (1) unknown cause and (2) cause unrelated to TB as non-recurrencesc | Patients with deaths of unknown cause or cause unrelated to TB did not experience recurrent TB | Composite/unfavorable strategye | Total effect on composite outcome |
Approach . | Operationalization . | Assumptions . | Corresponding Strategy in ICE Literaturea . | Corresponding Causal Effectsb . |
---|---|---|---|---|
Observed recurrence | Include individuals who died in the analysis and treat as non-recurrencesc | Patients who died did not experience recurrent TB | Composite/favorable strategyd | Total effect |
Hypothetical recurrence | Censor (exclude) individuals who died, account for common causes of death and recurrence with inverse-probability weights | All common causes of death and recurrence have been accounted for | Hypothetical strategy | Controlled direct effect |
Observed composite/any | Define a composite outcome of recurrence or death from any cause | … | Composite/unfavorable strategye | Total effect on composite outcome |
Observed composite/probable | Define a composite outcome of recurrence or death with either (1) unknown cause or (2) cause related to TB; treat deaths with cause unrelated to TB as non-recurrencesc | Patients with deaths unrelated to TB did not experience recurrent TB | Composite/unfavorable strategye | Total effect on composite outcome |
Observed composite/explicit | Define a composite outcome of recurrence or death with cause related to TB, treat deaths with (1) unknown cause and (2) cause unrelated to TB as non-recurrencesc | Patients with deaths of unknown cause or cause unrelated to TB did not experience recurrent TB | Composite/unfavorable strategye | Total effect on composite outcome |
Abbreviations: ICE, intercurrent event; TB, tuberculosis.
bYoung et al [1]. Although the associations reported here do not necessarily represent causal effects, the strategies for handling post-treatment deaths are related to strategies used to estimate causal effects in the presence of competing events.
cRecurrent TB outcome indicator set to 0 for all patients handled as non-recurrences.
Approach . | Operationalization . | Assumptions . | Corresponding Strategy in ICE Literaturea . | Corresponding Causal Effectsb . |
---|---|---|---|---|
Observed recurrence | Include individuals who died in the analysis and treat as non-recurrencesc | Patients who died did not experience recurrent TB | Composite/favorable strategyd | Total effect |
Hypothetical recurrence | Censor (exclude) individuals who died, account for common causes of death and recurrence with inverse-probability weights | All common causes of death and recurrence have been accounted for | Hypothetical strategy | Controlled direct effect |
Observed composite/any | Define a composite outcome of recurrence or death from any cause | … | Composite/unfavorable strategye | Total effect on composite outcome |
Observed composite/probable | Define a composite outcome of recurrence or death with either (1) unknown cause or (2) cause related to TB; treat deaths with cause unrelated to TB as non-recurrencesc | Patients with deaths unrelated to TB did not experience recurrent TB | Composite/unfavorable strategye | Total effect on composite outcome |
Observed composite/explicit | Define a composite outcome of recurrence or death with cause related to TB, treat deaths with (1) unknown cause and (2) cause unrelated to TB as non-recurrencesc | Patients with deaths of unknown cause or cause unrelated to TB did not experience recurrent TB | Composite/unfavorable strategye | Total effect on composite outcome |
Approach . | Operationalization . | Assumptions . | Corresponding Strategy in ICE Literaturea . | Corresponding Causal Effectsb . |
---|---|---|---|---|
Observed recurrence | Include individuals who died in the analysis and treat as non-recurrencesc | Patients who died did not experience recurrent TB | Composite/favorable strategyd | Total effect |
Hypothetical recurrence | Censor (exclude) individuals who died, account for common causes of death and recurrence with inverse-probability weights | All common causes of death and recurrence have been accounted for | Hypothetical strategy | Controlled direct effect |
Observed composite/any | Define a composite outcome of recurrence or death from any cause | … | Composite/unfavorable strategye | Total effect on composite outcome |
Observed composite/probable | Define a composite outcome of recurrence or death with either (1) unknown cause or (2) cause related to TB; treat deaths with cause unrelated to TB as non-recurrencesc | Patients with deaths unrelated to TB did not experience recurrent TB | Composite/unfavorable strategye | Total effect on composite outcome |
Observed composite/explicit | Define a composite outcome of recurrence or death with cause related to TB, treat deaths with (1) unknown cause and (2) cause unrelated to TB as non-recurrencesc | Patients with deaths of unknown cause or cause unrelated to TB did not experience recurrent TB | Composite/unfavorable strategye | Total effect on composite outcome |
Abbreviations: ICE, intercurrent event; TB, tuberculosis.
bYoung et al [1]. Although the associations reported here do not necessarily represent causal effects, the strategies for handling post-treatment deaths are related to strategies used to estimate causal effects in the presence of competing events.
cRecurrent TB outcome indicator set to 0 for all patients handled as non-recurrences.
Second, in the “hypothetical recurrence” strategy [1–5], we excluded patients who died. In this scenario, we estimate (1) recurrence risk in a world where deaths were eliminated (ie, one in which TB recurrence was observed in all patients vs only in those who lived) and (2) the association representing any direct effect between HIV infection and recurrence (Figure 2).
In strategies 3 through 5, we defined composite outcomes of recurrence or death. In the “observed composite/any” strategy, we included recurrent TB and death from any cause in the outcome, whereas in the “observed composite/probable” strategy, we included recurrent TB and death with unknown or TB-related cause. In the “observed composite/explicit” strategy, we included recurrent TB and only death due to a TB-related cause in the outcome. In the latter 2 strategies, we classified deaths not counted in the outcome as non-recurrences, thereby assuming that these patients did not experience recurrent TB. By including deaths in the outcome, composite strategies answer a different research question.
In all strategies, we treated missing FU as a missing data problem and excluded these patients. In the following section we describe the statistical adjustments used to ensure valid estimation of the target quantities.
Aligning Analytic Methods With Assumptions
Post-treatment Deaths
Excluding patients who died from analyses assumes that they have the same underlying risk of recurrent TB as those who lived. For the “hypothetical” strategy, we therefore used inverse-probability (IP) weights to account for potentially different underlying risks of recurrent TB among excluded versus included patients. HIV status and other factors associated with both post-treatment death and recurrence were used to construct IP weights: age, CD4 count, cavitary disease, alcohol use, drug use, smoking, incarceration, diabetes, stoppage of group A drugs due to adverse events, and body mass index (BMI) (see Supplementary Information for details).
Missing Post-treatment Follow-up Data
We utilized another set of IP weights to overcome the potential selection bias induced by excluding patients who lack follow-up data [20, 21]. We constructed the weight denominators by estimating the probabilities of having an observed 6-month post-treatment outcome conditional on HIV status and joint predictors of missing FU and recurrent TB: cavitary disease; BMI; time to culture conversion; use, duration, and likely effectiveness of group A drugs; stoppage of group A drugs due to an adverse event; country; and diabetes (see Supplementary Information for details).
Estimating Post-treatment Recurrence
Under each strategy for handling post-treatment deaths, we estimated overall risk by computing a weighted average of the corresponding outcome. Weights to account for missing FU were included in all analyses, whereas weights to account for common causes of death and recurrent TB were only used in the “hypothetical recurrence” analysis, in which new weights were constructed by multiplying together the 2 sets of weights. We estimated the relative risk of the outcome by HIV status by first fitting an IP-weighted logistic regression model with the HIV status indicator as the only covariate. The IP weights were calculated by multiplying the weights used in the overall risk estimation with an additional set of IP weights constructed to adjust for potential confounding by factors associated with HIV and recurrent TB (age, country, hepatitis C, alcohol use, drug use, smoking, incarceration; see Supplementary Information). Risk ratios were computed using predicted probabilities from logistic regression output [26]. We constructed 95% credible intervals for all estimates with a bootstrapping approach [27] using the boot package in R (R Core Team 2020).
Exploring the Impact of Selection Bias
Sensitivity Analysis
We repeated the analysis described in the previous section, this time without applying IP weights for missing FU, thereby imposing the assumption that people with and without FU are similar, on average, with regard to factors that influence recurrence. This approach does not account for the potential selection bias induced by excluding patients who lack FU data.
Simulation Study
Finally, to investigate the impact of potential selection bias in a different data setting, we conducted a simulation study (see Supplementary Information). Briefly, we generated an underlying population in which treatment site is strongly associated with having missing FU, TB recurrence, and death. We then computed and compared estimates of overall TB recurrence risk with and without weights to account for joint predictors of missing FU and TB recurrence. We explored the impact on results of varying different characteristics of the baseline simulation scenario (Supplementary Table 3), such as the underlying risk of TB recurrence.
RESULTS
Of 1991 patients analyzed, 17 (0.9%) died within 6 months post-treatment (2, TB contributing to death; 11, cause other than TB; 4, unknown cause), 746 (37.5%) lacked post-treatment follow-up data, and 1228 (61.7%) had an observed 6-month post-treatment outcome of recurrence or no change.
Table 2 shows the characteristics of the 1991 patients, overall and by 6-month post-treatment outcome. Overall, patients with HIV represented 11.9% of the cohort, but were overrepresented among those who lacked 6-month FU data (21.4%). Relative to those missing FU data and those observed at 6 months, patients who died were more likely to be aged 60 years or older. Compared with others, patients who lacked FU data were more likely to have a BMI of less than 18.5 kg/m2 and were less likely to have diabetes.
Characteristic . | Overall (N = 1991), n (%) . | Observed (n = 1228), n (%) . | Missing FU (n = 746), n (%) . | Died (n = 17), n (%) . |
---|---|---|---|---|
HIV | ||||
Yes | 237 (11.9) | 75 (6.1) | 160 (21.4) | 2 (11.8) |
No | 1754 (88.1) | 1153 (93.9) | 586 (78.6) | 15 (88.2) |
Age | ||||
0–19 y | 79 (4.0) | 37 (3.0) | 42 (5.6) | 0 (0.0) |
20–44 y | 1292 (64.9) | 806 (65.6) | 478 (64.1) | 8 (47.1) |
45–59 y | 486 (24.4) | 308 (25.1) | 174 (23.3) | 4 (23.5) |
60+ y | 134 (6.7) | 77 (6.3) | 52 (7.0) | 5 (29.4) |
BMI <18.5 kg/m2 | ||||
Yes | 423 (21.2) | 215 (17.5) | 197 (26.4) | 11 (64.7) |
No | 1568 (78.8) | 1013 (82.5) | 549 (73.6) | 6 (35.3) |
Diabetes mellitus | ||||
Yes | 295 (14.8) | 189 (15.4) | 103 (13.8) | 3 (17.6) |
No | 1669 (83.8) | 1031 (84.0) | 624 (83.6) | 14 (82.4) |
Missing | 27 (1.4) | 8 (0.7) | 19 (2.5) | 0 (0.0) |
Hepatitis C | ||||
Yes | 185 (9.3) | 130 (10.6) | 53 (7.1) | 2 (11.8) |
No | 1806 (90.7) | 1098 (89.4) | 693 (92.9) | 15 (88.2) |
Cavitary disease | ||||
Yes | 722 (36.3) | 469 (38.2) | 246 (33.0) | 7 (41.2) |
No | 1156 (58.1) | 722 (58.8) | 425 (57.0) | 9 (52.9) |
Missing | 113 (5.7) | 37 (3.0) | 75 (10.1) | 1 (5.9) |
BDQ ever used | ||||
Yes | 1567 (78.7) | 993 (80.9) | 561 (75.2) | 13 (76.5) |
No | 424 (21.3) | 235 (19.1) | 185 (24.8) | 4 (23.5) |
FQ ever used | ||||
Yes | 1341(67.4) | 819 (66.7) | 510 (68.4) | 12 (70.6) |
No | 650 (32.6) | 409 (33.3) | 236 (31.6) | 5 (29.4) |
LZD ever used | ||||
Yes | 1787 (89.8) | 1149 (93.6) | 622 (83.4) | 16 (94.1) |
No | 204 (10.2) | 79 (6.4) | 124 (16.6) | 1 (5.9) |
Region | ||||
Central Asia | 583 (29.3) | 373 (30.4) | 206 (27.6) | 4 (23.5) |
East or Southeast Asia | 94 (4.7) | 27 (2.2) | 64 (8.6) | 3 (17.6) |
South Asia | 455 (22.9) | 280 (22.8) | 170 (22.8) | 5 (29.4) |
Western Asia | 276 (13.9) | 217 (17.7) | 56 (7.5) | 3 (17.6) |
Europe | 82 (4.1) | 65 (5.3) | 17 (2.3) | 0 (0.0) |
Sub-Saharan Africa | 264 (13.3) | 54 (4.4) | 208 (27.9) | 2 (11.8) |
South America or Caribbean | 237 (11.9) | 212 (17.3) | 25 (3.4) | 0 (0.0) |
Characteristic . | Overall (N = 1991), n (%) . | Observed (n = 1228), n (%) . | Missing FU (n = 746), n (%) . | Died (n = 17), n (%) . |
---|---|---|---|---|
HIV | ||||
Yes | 237 (11.9) | 75 (6.1) | 160 (21.4) | 2 (11.8) |
No | 1754 (88.1) | 1153 (93.9) | 586 (78.6) | 15 (88.2) |
Age | ||||
0–19 y | 79 (4.0) | 37 (3.0) | 42 (5.6) | 0 (0.0) |
20–44 y | 1292 (64.9) | 806 (65.6) | 478 (64.1) | 8 (47.1) |
45–59 y | 486 (24.4) | 308 (25.1) | 174 (23.3) | 4 (23.5) |
60+ y | 134 (6.7) | 77 (6.3) | 52 (7.0) | 5 (29.4) |
BMI <18.5 kg/m2 | ||||
Yes | 423 (21.2) | 215 (17.5) | 197 (26.4) | 11 (64.7) |
No | 1568 (78.8) | 1013 (82.5) | 549 (73.6) | 6 (35.3) |
Diabetes mellitus | ||||
Yes | 295 (14.8) | 189 (15.4) | 103 (13.8) | 3 (17.6) |
No | 1669 (83.8) | 1031 (84.0) | 624 (83.6) | 14 (82.4) |
Missing | 27 (1.4) | 8 (0.7) | 19 (2.5) | 0 (0.0) |
Hepatitis C | ||||
Yes | 185 (9.3) | 130 (10.6) | 53 (7.1) | 2 (11.8) |
No | 1806 (90.7) | 1098 (89.4) | 693 (92.9) | 15 (88.2) |
Cavitary disease | ||||
Yes | 722 (36.3) | 469 (38.2) | 246 (33.0) | 7 (41.2) |
No | 1156 (58.1) | 722 (58.8) | 425 (57.0) | 9 (52.9) |
Missing | 113 (5.7) | 37 (3.0) | 75 (10.1) | 1 (5.9) |
BDQ ever used | ||||
Yes | 1567 (78.7) | 993 (80.9) | 561 (75.2) | 13 (76.5) |
No | 424 (21.3) | 235 (19.1) | 185 (24.8) | 4 (23.5) |
FQ ever used | ||||
Yes | 1341(67.4) | 819 (66.7) | 510 (68.4) | 12 (70.6) |
No | 650 (32.6) | 409 (33.3) | 236 (31.6) | 5 (29.4) |
LZD ever used | ||||
Yes | 1787 (89.8) | 1149 (93.6) | 622 (83.4) | 16 (94.1) |
No | 204 (10.2) | 79 (6.4) | 124 (16.6) | 1 (5.9) |
Region | ||||
Central Asia | 583 (29.3) | 373 (30.4) | 206 (27.6) | 4 (23.5) |
East or Southeast Asia | 94 (4.7) | 27 (2.2) | 64 (8.6) | 3 (17.6) |
South Asia | 455 (22.9) | 280 (22.8) | 170 (22.8) | 5 (29.4) |
Western Asia | 276 (13.9) | 217 (17.7) | 56 (7.5) | 3 (17.6) |
Europe | 82 (4.1) | 65 (5.3) | 17 (2.3) | 0 (0.0) |
Sub-Saharan Africa | 264 (13.3) | 54 (4.4) | 208 (27.9) | 2 (11.8) |
South America or Caribbean | 237 (11.9) | 212 (17.3) | 25 (3.4) | 0 (0.0) |
Abbreviations: BDQ, bedaquiline; BMI, body mass index; FQ, fluoroquinolone; HIV, human immunodeficiency virus; FU, follow-up; LZD, linezolid.
Characteristic . | Overall (N = 1991), n (%) . | Observed (n = 1228), n (%) . | Missing FU (n = 746), n (%) . | Died (n = 17), n (%) . |
---|---|---|---|---|
HIV | ||||
Yes | 237 (11.9) | 75 (6.1) | 160 (21.4) | 2 (11.8) |
No | 1754 (88.1) | 1153 (93.9) | 586 (78.6) | 15 (88.2) |
Age | ||||
0–19 y | 79 (4.0) | 37 (3.0) | 42 (5.6) | 0 (0.0) |
20–44 y | 1292 (64.9) | 806 (65.6) | 478 (64.1) | 8 (47.1) |
45–59 y | 486 (24.4) | 308 (25.1) | 174 (23.3) | 4 (23.5) |
60+ y | 134 (6.7) | 77 (6.3) | 52 (7.0) | 5 (29.4) |
BMI <18.5 kg/m2 | ||||
Yes | 423 (21.2) | 215 (17.5) | 197 (26.4) | 11 (64.7) |
No | 1568 (78.8) | 1013 (82.5) | 549 (73.6) | 6 (35.3) |
Diabetes mellitus | ||||
Yes | 295 (14.8) | 189 (15.4) | 103 (13.8) | 3 (17.6) |
No | 1669 (83.8) | 1031 (84.0) | 624 (83.6) | 14 (82.4) |
Missing | 27 (1.4) | 8 (0.7) | 19 (2.5) | 0 (0.0) |
Hepatitis C | ||||
Yes | 185 (9.3) | 130 (10.6) | 53 (7.1) | 2 (11.8) |
No | 1806 (90.7) | 1098 (89.4) | 693 (92.9) | 15 (88.2) |
Cavitary disease | ||||
Yes | 722 (36.3) | 469 (38.2) | 246 (33.0) | 7 (41.2) |
No | 1156 (58.1) | 722 (58.8) | 425 (57.0) | 9 (52.9) |
Missing | 113 (5.7) | 37 (3.0) | 75 (10.1) | 1 (5.9) |
BDQ ever used | ||||
Yes | 1567 (78.7) | 993 (80.9) | 561 (75.2) | 13 (76.5) |
No | 424 (21.3) | 235 (19.1) | 185 (24.8) | 4 (23.5) |
FQ ever used | ||||
Yes | 1341(67.4) | 819 (66.7) | 510 (68.4) | 12 (70.6) |
No | 650 (32.6) | 409 (33.3) | 236 (31.6) | 5 (29.4) |
LZD ever used | ||||
Yes | 1787 (89.8) | 1149 (93.6) | 622 (83.4) | 16 (94.1) |
No | 204 (10.2) | 79 (6.4) | 124 (16.6) | 1 (5.9) |
Region | ||||
Central Asia | 583 (29.3) | 373 (30.4) | 206 (27.6) | 4 (23.5) |
East or Southeast Asia | 94 (4.7) | 27 (2.2) | 64 (8.6) | 3 (17.6) |
South Asia | 455 (22.9) | 280 (22.8) | 170 (22.8) | 5 (29.4) |
Western Asia | 276 (13.9) | 217 (17.7) | 56 (7.5) | 3 (17.6) |
Europe | 82 (4.1) | 65 (5.3) | 17 (2.3) | 0 (0.0) |
Sub-Saharan Africa | 264 (13.3) | 54 (4.4) | 208 (27.9) | 2 (11.8) |
South America or Caribbean | 237 (11.9) | 212 (17.3) | 25 (3.4) | 0 (0.0) |
Characteristic . | Overall (N = 1991), n (%) . | Observed (n = 1228), n (%) . | Missing FU (n = 746), n (%) . | Died (n = 17), n (%) . |
---|---|---|---|---|
HIV | ||||
Yes | 237 (11.9) | 75 (6.1) | 160 (21.4) | 2 (11.8) |
No | 1754 (88.1) | 1153 (93.9) | 586 (78.6) | 15 (88.2) |
Age | ||||
0–19 y | 79 (4.0) | 37 (3.0) | 42 (5.6) | 0 (0.0) |
20–44 y | 1292 (64.9) | 806 (65.6) | 478 (64.1) | 8 (47.1) |
45–59 y | 486 (24.4) | 308 (25.1) | 174 (23.3) | 4 (23.5) |
60+ y | 134 (6.7) | 77 (6.3) | 52 (7.0) | 5 (29.4) |
BMI <18.5 kg/m2 | ||||
Yes | 423 (21.2) | 215 (17.5) | 197 (26.4) | 11 (64.7) |
No | 1568 (78.8) | 1013 (82.5) | 549 (73.6) | 6 (35.3) |
Diabetes mellitus | ||||
Yes | 295 (14.8) | 189 (15.4) | 103 (13.8) | 3 (17.6) |
No | 1669 (83.8) | 1031 (84.0) | 624 (83.6) | 14 (82.4) |
Missing | 27 (1.4) | 8 (0.7) | 19 (2.5) | 0 (0.0) |
Hepatitis C | ||||
Yes | 185 (9.3) | 130 (10.6) | 53 (7.1) | 2 (11.8) |
No | 1806 (90.7) | 1098 (89.4) | 693 (92.9) | 15 (88.2) |
Cavitary disease | ||||
Yes | 722 (36.3) | 469 (38.2) | 246 (33.0) | 7 (41.2) |
No | 1156 (58.1) | 722 (58.8) | 425 (57.0) | 9 (52.9) |
Missing | 113 (5.7) | 37 (3.0) | 75 (10.1) | 1 (5.9) |
BDQ ever used | ||||
Yes | 1567 (78.7) | 993 (80.9) | 561 (75.2) | 13 (76.5) |
No | 424 (21.3) | 235 (19.1) | 185 (24.8) | 4 (23.5) |
FQ ever used | ||||
Yes | 1341(67.4) | 819 (66.7) | 510 (68.4) | 12 (70.6) |
No | 650 (32.6) | 409 (33.3) | 236 (31.6) | 5 (29.4) |
LZD ever used | ||||
Yes | 1787 (89.8) | 1149 (93.6) | 622 (83.4) | 16 (94.1) |
No | 204 (10.2) | 79 (6.4) | 124 (16.6) | 1 (5.9) |
Region | ||||
Central Asia | 583 (29.3) | 373 (30.4) | 206 (27.6) | 4 (23.5) |
East or Southeast Asia | 94 (4.7) | 27 (2.2) | 64 (8.6) | 3 (17.6) |
South Asia | 455 (22.9) | 280 (22.8) | 170 (22.8) | 5 (29.4) |
Western Asia | 276 (13.9) | 217 (17.7) | 56 (7.5) | 3 (17.6) |
Europe | 82 (4.1) | 65 (5.3) | 17 (2.3) | 0 (0.0) |
Sub-Saharan Africa | 264 (13.3) | 54 (4.4) | 208 (27.9) | 2 (11.8) |
South America or Caribbean | 237 (11.9) | 212 (17.3) | 25 (3.4) | 0 (0.0) |
Abbreviations: BDQ, bedaquiline; BMI, body mass index; FQ, fluoroquinolone; HIV, human immunodeficiency virus; FU, follow-up; LZD, linezolid.
Six-Month Risk of Tuberculosis Recurrence
Ten patients (0.5%) experienced recurrent TB within 6 months. Table 3 shows the estimated six-month risk of recurrent TB under the 5 strategies for handling post-treatment deaths. The risk of recurrent TB was 7.4 (95% credible interval [CI]: 3.3–12.8) per 1000 individuals under the “observed recurrence” strategy and 7.6 (95% CI: 3.3–13.0) per 1000 under the “hypothetical recurrence” strategy. Estimated frequencies of a composite outcome under the “observed composite/any”, “observed composite/probable”, and “observed composite/explicit” strategies were 25.5 (95% CI: 15.3–38.1), 11.7 (95% CI: 6.4–18.2), and 8.6 (95% CI: 4.1–14.4) per 1000, respectively.
Estimated Overall Risk of Recurrence and Relative Risk by HIV Status Under 5 Strategies for Handling Post-treatment Deaths
. | Adjusted for Missing FU . | Not Adjusted for Missing FU . | ||
---|---|---|---|---|
Strategy . | Overall Risk per 1000a . | Relative Riska,b . | Overall Risk Per 1000a . | Relative Riska,b . |
Observed recurrence | 7.4 (3.3–12.8) | 1.2 (0.0–5.1) | 8.0 (3.3–13.6) | 1.3 (0.0–5.1) |
Hypothetical recurrence | 7.6 (3.3–13.0) | 1.2 (0.0–4.8) | 8.1 (3.3–13.8) | 1.3 (0.0–4.8) |
Observed composite/any | 25.5 (15.3–38.1) | 0.6 (0.1–1.7) | 21.7 (14.2–29.9) | 0.8 (0.1–2.1) |
Observed composite/probable | 11.7 (6.4–18.2) | 0.7 (0.0–2.7) | 12.9 (7.1–19.6) | 0.7 (0.0–2.6) |
Observed composite/explicit | 8.6 (4.1–14.4) | 1.0 (0.0–3.8) | 9.6 (4.8–15.8) | 1.0 (0.0–3.7) |
. | Adjusted for Missing FU . | Not Adjusted for Missing FU . | ||
---|---|---|---|---|
Strategy . | Overall Risk per 1000a . | Relative Riska,b . | Overall Risk Per 1000a . | Relative Riska,b . |
Observed recurrence | 7.4 (3.3–12.8) | 1.2 (0.0–5.1) | 8.0 (3.3–13.6) | 1.3 (0.0–5.1) |
Hypothetical recurrence | 7.6 (3.3–13.0) | 1.2 (0.0–4.8) | 8.1 (3.3–13.8) | 1.3 (0.0–4.8) |
Observed composite/any | 25.5 (15.3–38.1) | 0.6 (0.1–1.7) | 21.7 (14.2–29.9) | 0.8 (0.1–2.1) |
Observed composite/probable | 11.7 (6.4–18.2) | 0.7 (0.0–2.7) | 12.9 (7.1–19.6) | 0.7 (0.0–2.6) |
Observed composite/explicit | 8.6 (4.1–14.4) | 1.0 (0.0–3.8) | 9.6 (4.8–15.8) | 1.0 (0.0–3.7) |
Abbreviations: FU, follow-up; HIV, human immunodeficiency virus.
a95% credible intervals are constructed as the 2.5th and 97.5th percentiles of the 1000 bootstrap sample estimates.
bRelative risk is comparing the risk of outcome among people with HIV (numerator) and this risk among people without HIV (denominator).
Estimated Overall Risk of Recurrence and Relative Risk by HIV Status Under 5 Strategies for Handling Post-treatment Deaths
. | Adjusted for Missing FU . | Not Adjusted for Missing FU . | ||
---|---|---|---|---|
Strategy . | Overall Risk per 1000a . | Relative Riska,b . | Overall Risk Per 1000a . | Relative Riska,b . |
Observed recurrence | 7.4 (3.3–12.8) | 1.2 (0.0–5.1) | 8.0 (3.3–13.6) | 1.3 (0.0–5.1) |
Hypothetical recurrence | 7.6 (3.3–13.0) | 1.2 (0.0–4.8) | 8.1 (3.3–13.8) | 1.3 (0.0–4.8) |
Observed composite/any | 25.5 (15.3–38.1) | 0.6 (0.1–1.7) | 21.7 (14.2–29.9) | 0.8 (0.1–2.1) |
Observed composite/probable | 11.7 (6.4–18.2) | 0.7 (0.0–2.7) | 12.9 (7.1–19.6) | 0.7 (0.0–2.6) |
Observed composite/explicit | 8.6 (4.1–14.4) | 1.0 (0.0–3.8) | 9.6 (4.8–15.8) | 1.0 (0.0–3.7) |
. | Adjusted for Missing FU . | Not Adjusted for Missing FU . | ||
---|---|---|---|---|
Strategy . | Overall Risk per 1000a . | Relative Riska,b . | Overall Risk Per 1000a . | Relative Riska,b . |
Observed recurrence | 7.4 (3.3–12.8) | 1.2 (0.0–5.1) | 8.0 (3.3–13.6) | 1.3 (0.0–5.1) |
Hypothetical recurrence | 7.6 (3.3–13.0) | 1.2 (0.0–4.8) | 8.1 (3.3–13.8) | 1.3 (0.0–4.8) |
Observed composite/any | 25.5 (15.3–38.1) | 0.6 (0.1–1.7) | 21.7 (14.2–29.9) | 0.8 (0.1–2.1) |
Observed composite/probable | 11.7 (6.4–18.2) | 0.7 (0.0–2.7) | 12.9 (7.1–19.6) | 0.7 (0.0–2.6) |
Observed composite/explicit | 8.6 (4.1–14.4) | 1.0 (0.0–3.8) | 9.6 (4.8–15.8) | 1.0 (0.0–3.7) |
Abbreviations: FU, follow-up; HIV, human immunodeficiency virus.
a95% credible intervals are constructed as the 2.5th and 97.5th percentiles of the 1000 bootstrap sample estimates.
bRelative risk is comparing the risk of outcome among people with HIV (numerator) and this risk among people without HIV (denominator).
Risk of Tuberculosis Recurrence by HIV Status
The relative risks of recurrent TB in patients with and without HIV under the “observed recurrence” and “hypothetical recurrence” strategies were 1.2 (95% CI: 0.0–5.1) and 1.2 (95% CI: 0.0–4.8), respectively (Table 3). The corresponding risk ratios for the 3 composite outcomes were 0.6 (95% CI: 0.1–1.7), 0.7 (95% CI: 0.0–2.7), and 1.0 (95% CI: 0.0–3.8) for the “observed composite/any”, “observed composite/probable”, and “observed composite/explicit” strategies, respectively.
Sensitivity Analysis
When patients missing post-treatment follow-up were excluded from the analysis without adjustment for potential selection bias, the estimated overall risk of recurrent TB was 8.0 per 1000 (95% CI: 3.3–13.6) under the “observed recurrence” strategy, 8.1 per 1000 (95% CI: 3.3–13.8) under the “hypothetical recurrence” strategy, and 21.7 (95% CI: 14.2–29.9), 12.9 (95% CI: 7.1–19.6), and 9.6 (95% CI: 4.8–15.8) per 1000 under the 3 composite strategies (Table 3). Unadjusted relative risk estimates differed in magnitude from the adjusted estimates under the “observed composite/any” strategy, but were similar in the other 2 composite strategies (Table 3).
Simulation Study
The results of the simulation study are shown in Supplementary Tables 4–9 in the Supplementary Information. The variation in results across simulation scenarios demonstrates that there are several factors that increase the impact of selection bias, such as a stronger relationship between the joint predictor(s) of missing FU and recurrence and (1) a higher frequency of missing FU or (2) a higher incidence of recurrence.
DISCUSSION
The overall risk of TB recurrence and TB recurrence or death was small, substantiating the effectiveness of longer MDR-TB regimens containing BDQ and/or DLM. This was true irrespective of method used to handle post-treatment deaths. The association between HIV and TB recurrence was inconclusive due to the small number of recurrences and deaths, which resulted in wide credible intervals across all strategies.
Estimates of TB recurrence and relative risk comparing patients with and without HIV were similar whether derived under the assumption that patients who died post-treatment did not experience recurrent TB (“observed recurrence”) or in a hypothetical scenario where all patient deaths were eliminated (“hypothetical recurrence”). There are other scenarios in which the difference between these 2 strategies might be larger; for example, if post-treatment deaths were more common and patients who died had a much higher risk of recurrent TB compared with others, the “hypothetical recurrence” estimate would be markedly higher than the “observed recurrence” estimate.
As expected, estimates of the 6-month incidence of composite outcomes of TB recurrence or death were higher than the estimates for recurrence alone. Furthermore, estimates varied depending on which deaths were included in the composite outcome: the incidence of recurrent TB or death from any cause was almost 3 times that of recurrent TB or TB-related death, and the risk ratio estimates changed in magnitude depending on whether deaths of unknown cause were included in the outcome or assumed to be non-recurrences. The proportion of post-treatment deaths that are TB-related, and therefore the importance of incorporating cause of death into composite estimates, will vary by setting and highlights the importance of TB programs ascertaining such information.
The estimates from each strategy answer different questions and, in practice, researchers and decision-makers will need to select which one to report [1–5]. Intuitively, the “observed recurrence” strategy may be preferred to the “hypothetical recurrence” strategy, as the latter considers a scenario that is aspirational but not currently relevant to most settings (ie, one in which all post-treatment deaths could be prevented). Moreover, valid estimation under the “hypothetical recurrence” strategy requires complete data on common causes of death and recurrent TB, which may not be routinely collected by TB programs. On the other hand, the “observed recurrence” strategy may be inadequate in certain circumstances. For example, if patients with a certain characteristic were much more likely to die post-treatment compared with those without that characteristic, the “observed recurrence” estimate could indicate that patients with these characteristics are less likely to experience recurrent TB because earlier death largely prevented this group from developing recurrent TB (see Rojas-Saunero et al [2] for another example). A second scenario in which the “observed recurrence” strategy may not be ideal is when deaths due to undetected TB are common. In this case, one of the composite strategies might be preferable, particularly when complete information on cause of death is available, although this fundamentally alters the outcome.
The preferred strategy may differ based on the clinical relevance of the questions that each answers [4], which is dependent on whose perspective is considered—for example, that of the patient, the clinician, or a national TB program (NTP). In the context of this study, the estimates from the “observed composite/any” strategy might be most relevant to a patient for whom TB recurrence and death from any cause constitute unfavorable outcomes, or to clinicians and NTPs who are interested in minimizing overall TB morbidity and mortality. On the other hand, for the purpose of evaluating a particular regimen's effectiveness, the “observed composite/probable” or “observed composite/explicit” strategies may be more relevant. In this latter scenario, yet another strategy may be preferred if, contrary to our setting, information on cause of death is complete and precise: a “hypothetical composite/explicit” strategy, in which the outcome is defined as a composite of recurrent TB and TB-related deaths, and TB-unrelated deaths are excluded. Given adjustment for common causes of TB-unrelated death and the composite outcome, this strategy estimates the risk of recurrence and TB-related death in the hypothetical scenario in which TB-unrelated deaths were eliminated, a scenario that might be plausible, if, for example, TB-unrelated deaths are mostly accidental.
Patients with a low BMI or HIV were more likely, and patients with diabetes were less likely, to have missing FU data in this study. Previous studies have linked each of these subgroups to an increased risk of recurrent TB [9, 11, 18], which underscores the importance of accounting for patients with missing FU data when estimating recurrent TB. In sensitivity analyses, estimates computed by excluding patients with missing FU data and without accounting for selection bias through IP weighting were similar to the IP-weighted estimates of overall recurrence. Although credible intervals were largely overlapping, the unadjusted overall risk estimates were typically slightly higher than the adjusted estimates, likely because patients from treatment sites with higher rates of missing FU and lower outcome rates were up-weighted in the adjusted analysis. Larger imbalances in the characteristics of patients with and without FU combined with a stronger association of these characteristics with TB recurrence risk would result in larger differences in the weighted and unweighted estimates. The simulation study in the Supplementary Information provides examples of several such settings.
This study has several limitations. First, although current evidence suggests 12 months of FU to capture most relapses [28, 29], we estimated 6-month post-treatment outcomes, as data were not consistently collected thereafter. Second, we did not conduct sequencing to distinguish between TB relapse and reinfection; however, relapse is likely to be the prevailing source of recurrent TB in the first 6 months post-treatment [30]. Finally, our results on HIV as a determinant of recurrence may be affected by collider bias [31, 32]. This could occur if there is a factor such as age that is associated with both HIV status and inclusion in the cohort. If a third factor, such as TB disease severity, is associated with cohort inclusion and recurrent TB risk, the relationship observed between HIV status and recurrent TB may be distorted. This is a challenge with all observational TB studies, as TB is more likely to go undetected among people with HIV [33]. This bias could be reduced through IP weighting if data were available on factors related to (1) HIV status and cohort inclusion and (2) cohort inclusion and TB recurrence. Unfortunately, this information is often unattainable in practice.
As MDR/RR-TB treatment guidelines evolve in response to emerging evidence [34–36] on the relative safety and effectiveness of all-oral shortened regimens compared with conventional longer treatments, an important question is whether this relative safety differs across important patient subgroups, such as people with HIV [28]. Rigorous analyses of post-TB treatment recurrence are needed to accurately understand whether certain groups require closer monitoring, given possible increased risk of recurrence [37–39] relative to longer regimens.
While methods for handling intercurrent events and potential selection bias due to missing FU are well established [4, 20, 21], their use in estimating TB recurrence is sparse. Careful handling of post-treatment deaths and missing post-treatment FU data together with accompanying sensitivity analyses will generate more robust and interpretable evidence, with the overall goal of improving treatment and care.
Supplementary Data
Supplementary materials are available at Clinical Infectious Diseases online. Consisting of data provided by the authors to benefit the reader, the posted materials are not copyedited and are the sole responsibility of the authors, so questions or comments should be addressed to the corresponding author.
Notes
Author Contributions. S. M. S., C. D. M., and M. F. led the conceptualization, data curation, methodology, analysis, and writing of the paper. C. D. M., M. F., M. R., K. S., U. K., P. K., M. B., C. H., and H. H. led the design of the endTB study. U. K., C. H., M. B., D. H., S. L., M. K., S. P., S. A., A. K. I., A. Krisnanda, S. C. V., S. B., A. Kumsa, W. D., K. T., M. M., H. A., T. V., T. T. T., K. S., M. R., H. H., and P. K. contributed to data collection and curation. All authors contributed to editing and review of the paper.
Acknowledgments. The authors thank the patients who participated in the endTB observational study and the clinicians and program staff of participating national tuberculosis programs. They also thank the endTB staff at Partners in Health, Doctors Without Borders, Epicentre, and Interactive Research and Development.
Disclaimer. The funders had no role in the conceptualization, analysis, or presentation of findings of this study. The contents of this paper are solely the responsibility of the authors and do not necessarily represent the official views of the NIH.
Financial support. The endTB observational study was funded by UNITAID. S. M. S. was supported by the National Institutes of Allergy and Infectious Diseases under award number T32 AI007433. M. F., C. D. M., K. S., and M. R. were supported by the National Institutes of Allergy and Infectious Diseases under award number R01AI46095. S. L. was supported by the Canadian Institutes of Health Research under award number TS1-170663.
Data availability statement. Some of the data included in this analysis are managed in countries governed by the European Union General Data Protection Regulation (GDPR). The data contain sensitive and potentially identifying information and cannot be sufficiently anonymized to meet GDPR standards and retain their utility. Pseudo-anonymized data will be made available upon request to an Médecins Sans Frontières Medical Director at [email protected], and execution of a data-sharing agreement or alternate means that allow assurance that principles of GDPR regulations will be met.
References
Author notes
H. H., P. K., and M. K. contributed equally.
Potential conflicts of interest. Bedaquiline donations made from Janssen to the Global Drug Facility were used for patients in the endTB observational study. Donations of delamanid from Otsuka were used for initial patients enrolled in the endTB observational study. The companies from which drug donations were received did not have any role on the study design, data analyses, data interpretation, or manuscript writing. C. D. M. has served as a board member of Otsuka Scientific Advisory Board. U. K. reports that the endTB Consortium coordinated donations of delamanid from Otsuka Pharmaceuticals to be used for treatment of some of the patients included in the endTB observational study. U. K. also reports that the endTB Consortium coordinated donations of bedaquiline from Janssen to be used for treatment of some of the patients included in the endTB observational study. P. K. reports honoraria for being part of an expert panel, payments made to the author, from Johns Hopkins University. All other authors report no potential conflicts.
All authors have submitted the ICMJE Form for Disclosure of Potential Conflicts of Interest. Conflicts that the editors consider relevant to the content of the manuscript have been disclosed.