-
PDF
- Split View
-
Views
-
Cite
Cite
Armin Rashidi, Jonathan U Peled, Maryam Ebadi, Tauseef Ur Rehman, Heba Elhusseini, LeeAnn T Marcello, Hossam Halaweish, Thomas Kaiser, Shernan G Holtan, Alexander Khoruts, Daniel J Weisdorf, Christopher Staley, Protective Effect of Intestinal Blautia Against Neutropenic Fever in Allogeneic Transplant Recipients, Clinical Infectious Diseases, Volume 75, Issue 11, 1 December 2022, Pages 1912–1920, https://doi.org/10.1093/cid/ciac299
- Share Icon Share
Abstract
Neutropenic fever (NF) occurs in >70% of hematopoietic cell transplant (HCT) recipients, without a documented cause in most cases. Antibiotics used to prevent and treat NF disrupt the gut microbiota; these disruptions predict a higher posttransplantation mortality rate. We hypothesized that specific features in the gut microbial community may mediate the risk of NF.
We searched a large gut microbiota database in allogeneic HCT recipients (12 546 stool samples; 1278 patients) to find pairs with NF (cases) versus without NF (controls) on the same day relative to transplantation and with a stool sample on the previous day. A total of 179 such pairs were matched as to the underlying disease and graft source. Several other important clinical variables were similar between the groups.
The gut microbiota of cases on the day before NF occurrence had a lower abundance of Blautia than their matched controls on the same day after transplantation, suggesting a protective role for Blautia. Microbiota network analysis did not find any differences in community structure between the groups, suggesting a single-taxon effect. To identify putative mechanisms, we searched a gut microbiome and serum metabolome database of patients with acute leukemia receiving chemotherapy and identified 139 serum samples collected within 24 hours after a stool sample from the same patient. Greater Blautia abundances predicted higher levels of next-day citrulline, a biomarker of total enterocyte mass.
These findings support a model in which Blautia protects against NF by improving intestinal health. Therapeutic restoration of Blautia may help prevent NF, thus reducing antibiotic exposures and transplantation-related deaths.
In most patients undergoing allogeneic hematopoietic cell transplantation (HCT), neutropenic fever (NF) develops in the early posttransplantation period [1, 2], resulting in prolonged and costly hospitalization, heavy antibiotic exposure, and compromised quality of life. The multitude of antibiotics used to prevent and treat NF in these patients disrupts gut microbial communities [3, 4]; reduced gut microbial diversity has been associated with increased posttransplantation mortality rates [5]. Translocation of bacteria from the gut in the setting of transplant conditioning–related gut barrier damage is a known cause of bacteremia and NF [6–9]. However, because blood cultures and other infectious workup usually fail to find a culprit, the cause of NF remains unknown in most cases [1]. A better understanding of NF pathogenesis could help identify novel targets for prevention and treatment, leading to improved outcomes after HCT. We have found preliminary evidence for a more complex interaction between the gut microbiota and the host as a mechanism for NF. Specific bacteria can influence the integrity and function of the intestinal epithelium, thus altering the risk of translocation of other microbes and also modulating metabolic cross-talk between the host and microbiota [10, 11].
The study of the gut microbiota in HCT recipients is challenging owing to the high-risk nature of most experimental interventions in this patient population and also the large interindividual variability of clinical factors that may influence both the microbiota and transplant outcomes. In observational studies, the patient cohort should ideally be homogeneous in relevant covariates. Unfortunately, most HCT microbiota databases are not large enough to permit selection of a homogenous cohort with an adequate sample size for powerful analysis. We used a large database of fecal microbiota profiles of allogeneic HCT recipients (12 546 longitudinal stool samples from 1278 patients) to characterize gut microbiota composition within 24 hours before NF. The large size of this database allowed us to identify 179 matched pairs with versus without NF on the same day after transplantation.
Although the observational design of this study can only be used to define correlations and not causal relationships, we selected a short interval between the sample and subsequent NF to minimize relevant interim events, thus maximizing the chances that any observed associations reflect a directional causal link. First, we found a significant decrease in Blautia before NF, without major changes in network characteristics of the gut microbiota. To identify putative mechanisms for this single-taxon effect, we used an independent biorepository of stool and serum samples in patients with acute myeloid leukemia (AML) receiving induction chemotherapy. We found 139 paired samples with serum collected within 24 hours after a stool sample from the same patient. The analysis of stool microbiome and serum metabolome in these sample pairs showed that higher Blautia abundances in the gut were associated with higher serum levels of citrulline, a biomarker of intestinal epithelial mass [12, 13], in the next 24 hours.
METHODS
The overall study schema is shown in Figure 1A.
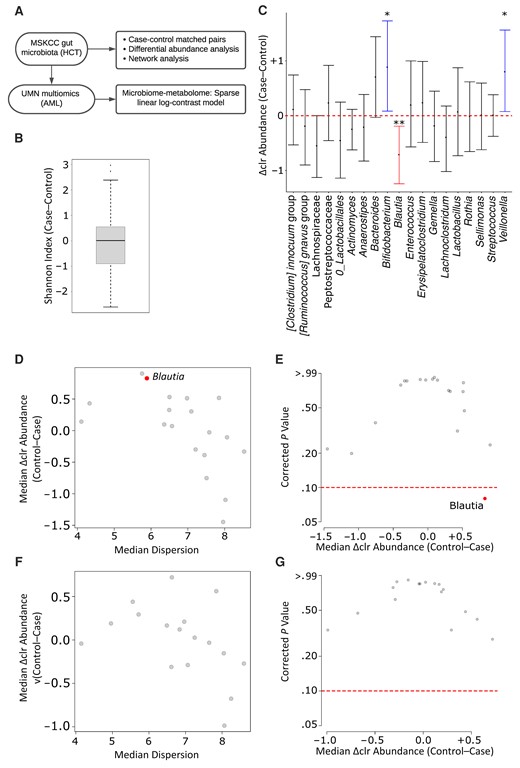
Gut microbiota in cases and controls. A, Overall study schema. Abbreviations: AML, acute myeloid leukemia; HCT, hematopoietic cell transplantation; MSKCC, Memorial Sloan Kettering Cancer Center; UMN, University of Minnesota. B, Alpha diversity. as measured by the Shannon index. The difference between each case and their matched control was used to generate the plot. The horizontal line in the middle represents the median and the box the interquartile range; the whiskers extend to the last data value that is still within 1.5 times the interquartile range from the lower or upper quartile. C, Genus-level difference in centered log-ratio (Δclr) abundance between cases and their matched controls. The y-axis shows median values (with interquartile ranges). Red and blue represent genera that were significantly more abundant in controls or cases, respectively. Significance is shown by one (P < .05) or two (P < .01) asterisks. No formal differential abundance analysis was performed in this plot. D–G, ALDEx2 results with a 1-day (D, E) versus 2-day (F, G) window interval between the stool sample and subsequent neutropenic fever (cases) or no neutropenic fever (controls). Differentially abundant genera (q < 0.10 in aldex.ttest) are shown in red.
Memorial Sloan Kettering Cancer Center Database
The Memorial Sloan Kettering Cancer Center (MSKCC) HCT data set [14] includes 12 546 longitudinal stool samples collected from 1278 allogeneic HCT recipients. Samples were prospectively collected, most commonly weekly, during the hospitalization for HCT, although some samples were collected before admission and sometimes samples were collected more frequently. In addition to 16S ribosomal RNA amplicon sequences, we used the published “hospitalome” associated with this data set [14] to find patient pairs with NF (cases) and without NF (controls) on the same day relative to transplantation and with a stool sample on the previous day. With the day of transplantation defined as day 0, we explored the database between days −7 and 28 for the occurrence of a first NF episode for each patient. The choice of day −7 was made to capture the beginning of hospitalization in all patients. NF was defined as an oral temperature ≥100.4°F combined with an absolute neutrophil count ≤0.5 × 109/L [15]. We excluded samples with a depth of <5000 reads. This threshold was chosen according to the lower 10% quantile among all samples (4927 reads).
We chose the underlying disease and graft source as criteria for 1:1 matching of cases and controls because they are associated with other important factors that influence the microbiota and NF. As an example, patients with acute leukemia usually receive higher-intensity chemotherapy than patients with lymphoma or myeloma, spend more time in the hospital, and experience a higher antibiotic exposure. Similarly, the immunosuppressive medications used early after HCT are frequently determined based on the graft source. Therefore, this “bundling” feature is expected to yield a reasonable match for several additional clinical variables. We did not consider antibiotic exposures because although they are a powerful cause of microbiota disruptions, our question concerned the connection between microbiota changes (regardless of their cause) and subsequent NF. After finding 179 pairs of case and control samples matched 1:1 for the underlying disease and graft source in their corresponding patients, we compared cases and controls in aggregate for age, sex, conditioning intensity, and HCT-specific comorbidity index [16]. Supplementary Data 1 provides sample identifiers (IDs) for cases and controls, which may be used to retrieve additional linked data from a prior publication [14].
University of Minnesota Database
The University of Minnesota (UMN) has a large longitudinal gut microbiota data set in patients with AML receiving inpatient induction chemotherapy (National Center for Biotechnology Information sequence read archive no. SRP141394) [10]. This database includes 410 stool samples from 52 patients. A total of 260 serum samples from these patients have been analyzed for metabolomics [10]. We and others have shown that the pattern of microbiota injury in these patients is largely similar to that of allogeneic HCT recipients [4, 17]. Thus, we used this unique microbiome/metabolome database to find potential circulating metabolites that may explain the mechanism of the protective effect of Blautia against NF in the MSKCC database. Sample collection started with hospital admission and continued twice weekly (Monday/Thursday ±2 days) until day 28 of chemotherapy or discharge (whichever occurred first). Serum samples were collected twice weekly (Monday/Thursday; preprandial) between 6 and 8 Am in standard red-top tubes, split in 250-µL aliquots, and stored at −80°C within 2 hours of collection. Stool samples were collected in 95% ethanol-filled sterile tubes and stored at −80°C. We identified 139 same-patient pairs of serum and stool samples, with the stool sample collected within 24 hours before the serum sample. This interval mirrored that used in the analysis of MSKCC data. The short interval also minimized potential intervening confounders and accounted for the short half-life of many circulating metabolites.
Gut Microbiome and Serum Metabolome Profiling
The experimental procedures to generate 16S ribosomal RNA gene amplicon sequences in MSKCC and UMN databases have been described in detail elsewhere [14, 18]. The UMN serum metabolome was profiled using untargeted, ultra-high-performance liquid chromatography tandem mass spectroscopy (Supplementary Methods, Supplementary Tables 1–4, and Supplementary Figure 1).
Statistical Analysis
Methodological details are provided in Supplementary Methods. A paired Wilcoxon test was used to compare MSKCC cases and controls for alpha diversity. Differential abundance analysis using ALDEx2 [19] was performed to identify overrepresented and underrepresented taxa in each group. The MSKCC gut microbiota network was compared between the groups using the 100 most abundant genera (NetCoMi package [20]). To evaluate the potential mechanisms by which differentially abundant taxa may protect against or promote NF, a multiomics analysis of the UMN gut microbiome and serum metabolome database was performed, using sparse linear log-contrast modeling [21].
RESULTS
A total of 179 samples from 179 unique case and their matched paired 179 samples from 117 unique controls in the MSKCC database were analyzed (Table 1). Case samples were individually matched to control samples for the underlying disease and graft source. The underlying disease distribution included leukemia in 98 pairs(55%), myeloproliferative neoplasm or myelodysplastic syndrome in 41 (23%), lymphoma in 24 (13%), and multiple myeloma in 16 (9%). Graft source distribution included bone marrow in 23 pairs (13%), peripheral blood in 47 (26%), cord blood in 18 (10%), and T-deplete grafts in 91 (51%). When the included patients were considered for other important clinical variables in aggregate, there were no significant differences between the groups in sex, conditioning intensity, and HCT-specific comorbidity index. Controls were significantly older than cases (mean age, 56 vs 53 years, respectively; P = .04), but this difference did not seem clinically meaningful. Infection workup at the time of NF in cases revealed Escherichia coli in 8 patients, vancomycin-resistant enterococci in 2, and Enterobacter species in 2. The median (range) number of reads per sample was 28 428 (range, 5021–703 665). Alpha diversity was similar in both groups (median, 2.60 in cases vs 2.54 in controls, respectively; median difference, 0.009; paired Wilcoxon P = .35; Figure 1B).
When microbiota composition in the 2 groups was compared using a simple analysis of pairwise differences in genus-level abundances, Blautia was significantly more abundant in controls (paired Wilcoxon P = .007), while Bifidobacterium and Veillonella (both P = .03) were more abundant in cases (Figure 1C). Next, we proceeded to formal differential abundance analysis using the 19 postfiltering genera (Supplementary Data 2). Blautia was the only differentially abundant genus, significantly more abundant in controls (corrected paired Wilcoxon P = .08; Figure 1D and 1E). In exploratory analysis with a 2-day interval allowed between the stool sample and fever and 294 eligible pairs of matched case and control samples, no differentially abundant genera were found (Figure 1F and 1G), indicating that the apparent protective effect of Blautia occurs on a fast time scale.
Next, we asked whether the relative depletion of Blautia before NF represents a single-taxon effect or an indicator of a broader, community-level change in the gut microbiota. In comparative network analysis of the microbiota in cases and controls, we found no significant differences between the groups in any of the studied measures (Figure 2 and Supplementary Data 3). The Blautia cluster was highly similar in the 2 groups and included obligate anaerobic commensals in the Lachnospiraceae (Lachnoclostridium [Ruminococcus] gnavus group, [Ruminococcus] torques group, Sellimonas, Anaerostipes) and Oscillospiraceae (UBA1819, Ruminiclostridium 5) families (Figure 2). The strongest association of Blautia in both groups was with Lachnoclostridium (Figure 2). These findings suggested that the negative association between Blautia and NF was a single-taxon effect rather than a reflection of a broader community-level change in the gut microbiota.
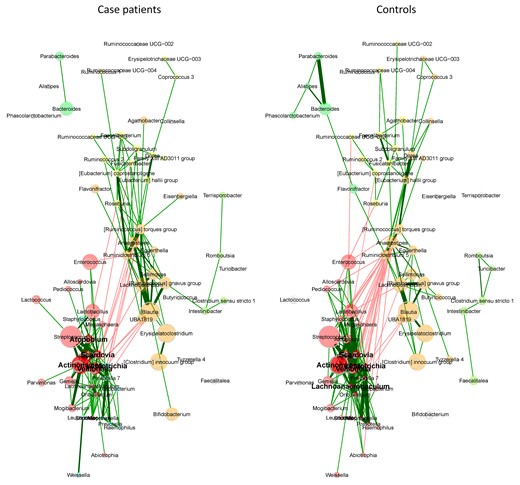
Network analysis of the gut microbiota. Two clusters have the same color in both groups if they have ≥2 nodes in common. Node sizes are scaled to the column sums of centered log-ratio-transformed data. Edge weights are (nonnegative) similarities, with red and green representing negative and positive associations, respectively.
We then evaluated antibiotic exposures as a potential cause for Blautia loss. We defined each sample as Blautia low or high based on its relative abundance of Blautia compared with the median Blautia abundance in all samples (12.6%). We considered all antibacterial antibiotic exposures before NF in cases and before the same day relative to HCT in their matched controls. We found antibiotic exposures among Blautia-low samples to be consistently heavier than in Blautia-high samples (Figure 3). This suggests that antibiotic exposure was a major cause of Blautia loss.
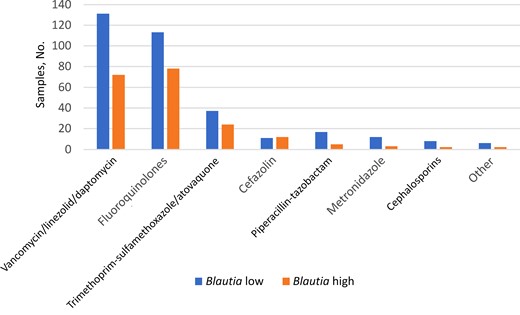
Antibacterial antibiotic exposures before samples with low versus high Blautia abundance. Low and high Blautia abundance were defined based on whether the relative abundance of Blautia in the sample was lower or higher than the median among all samples. Exposure was defined binarily. Cephalosporins were third generation or higher.
To explore potential mechanisms by which Blautia may protect against NF, we investigated the relationship between the gut microbiota and serum metabolome in an independent multiomics database from the UMN. Although the patients in this database were enrolled at the time of induction chemotherapy, their patterns of gut microbiota changes largely resemble those in allogeneic HCT recipients [4]. The 2 populations have many microbiome-relevant similarities, including mucositis due to cytotoxic chemotherapy, nutritional compromise, prolonged hospital stay, and extensive healthcare and antibiotic exposure. However, there are also some important differences between the 2 patient populations. Most HCT recipients have previously experienced microbiome damage due to their prior treatments, whereas many patients with AML have new diagnoses, without a significant prior history. The presence of an allogeneic immune system and the use of total body irradiation are other unique features of HCT recipients. We included data from patients with AML with the goal of finding novel insight into a plausible mechanism for the observed association in HCT recipients, while understanding that confident extrapolation may not be possible given the differences between the 2 patient populations.
Patient Characteristics in the Memorial Sloan Kettering Cancer Center Cohort
Characteristic . | Patients, No. (%)a . | P-value . | |
---|---|---|---|
Cases (n = 179) . | Controls (n = 117) . | ||
Age, mean (SD), y | 53 (13) | 56 (12) | .04 |
Sex | |||
Male | 120 (67) | 70 (60) | .25 |
Female | 59 (33) | 47 (40) | |
HCT-CI | |||
0–1 | 54 (31) | 36 (32) | >.99 |
≥2 | 119 (69) | 78 (68) | |
Conditioning intensity | |||
Myeloablative | 124 (69) | 69 (59) | .11 |
Reduced intensity | 11 (6) | 6 (5) | |
Nonmyeloablative | 44 (25) | 42 (36) |
Characteristic . | Patients, No. (%)a . | P-value . | |
---|---|---|---|
Cases (n = 179) . | Controls (n = 117) . | ||
Age, mean (SD), y | 53 (13) | 56 (12) | .04 |
Sex | |||
Male | 120 (67) | 70 (60) | .25 |
Female | 59 (33) | 47 (40) | |
HCT-CI | |||
0–1 | 54 (31) | 36 (32) | >.99 |
≥2 | 119 (69) | 78 (68) | |
Conditioning intensity | |||
Myeloablative | 124 (69) | 69 (59) | .11 |
Reduced intensity | 11 (6) | 6 (5) | |
Nonmyeloablative | 44 (25) | 42 (36) |
Abbreviation: HCT-CT, HCT-specific comorbidity index; SD, standard deviation.
Data represent no. (%) of patients unless otherwise specified.
Patient Characteristics in the Memorial Sloan Kettering Cancer Center Cohort
Characteristic . | Patients, No. (%)a . | P-value . | |
---|---|---|---|
Cases (n = 179) . | Controls (n = 117) . | ||
Age, mean (SD), y | 53 (13) | 56 (12) | .04 |
Sex | |||
Male | 120 (67) | 70 (60) | .25 |
Female | 59 (33) | 47 (40) | |
HCT-CI | |||
0–1 | 54 (31) | 36 (32) | >.99 |
≥2 | 119 (69) | 78 (68) | |
Conditioning intensity | |||
Myeloablative | 124 (69) | 69 (59) | .11 |
Reduced intensity | 11 (6) | 6 (5) | |
Nonmyeloablative | 44 (25) | 42 (36) |
Characteristic . | Patients, No. (%)a . | P-value . | |
---|---|---|---|
Cases (n = 179) . | Controls (n = 117) . | ||
Age, mean (SD), y | 53 (13) | 56 (12) | .04 |
Sex | |||
Male | 120 (67) | 70 (60) | .25 |
Female | 59 (33) | 47 (40) | |
HCT-CI | |||
0–1 | 54 (31) | 36 (32) | >.99 |
≥2 | 119 (69) | 78 (68) | |
Conditioning intensity | |||
Myeloablative | 124 (69) | 69 (59) | .11 |
Reduced intensity | 11 (6) | 6 (5) | |
Nonmyeloablative | 44 (25) | 42 (36) |
Abbreviation: HCT-CT, HCT-specific comorbidity index; SD, standard deviation.
Data represent no. (%) of patients unless otherwise specified.
The gut microbiota is known to regulate and significantly contribute to the circulating metabolome [22–24]. Thus, we used this unique multiomics database to address the question of interest in the present study. In all, 139 same-patient stool-serum sample pairs from 51 patients were identified and analyzed by means of sparse linear log-contrast modeling. Each stool sample was collected within 24 hours before its corresponding serum sample. Table 2 summarizes patient characteristics. We detected 945 circulating metabolites, 872 of which were detectable in at least half of the samples (Supplementary Data 4). The 10 postfiltering genera were included in the model as potential predictors of the 872 metabolites.
Characteristic . | Patients, No. (%)a (n = 51) . |
---|---|
Age, mean (SD), y | 57 (15) |
Sex | |
Male | 30 (59) |
Female | 21 (41) |
Disease phase | |
Newly diagnosed | 44 (86) |
Relapsed | 7 (14) |
Chemotherapy regimen | |
7 + 3 (Conventional or liposomal) | 35 (69) |
Clofarabine + cytarabine | 9 (18) |
Venetoclax + hypomethylator | 3 (6) |
Other | 4 (8) |
Characteristic . | Patients, No. (%)a (n = 51) . |
---|---|
Age, mean (SD), y | 57 (15) |
Sex | |
Male | 30 (59) |
Female | 21 (41) |
Disease phase | |
Newly diagnosed | 44 (86) |
Relapsed | 7 (14) |
Chemotherapy regimen | |
7 + 3 (Conventional or liposomal) | 35 (69) |
Clofarabine + cytarabine | 9 (18) |
Venetoclax + hypomethylator | 3 (6) |
Other | 4 (8) |
Data represent no. (%) of patients unless otherwise specified.
Characteristic . | Patients, No. (%)a (n = 51) . |
---|---|
Age, mean (SD), y | 57 (15) |
Sex | |
Male | 30 (59) |
Female | 21 (41) |
Disease phase | |
Newly diagnosed | 44 (86) |
Relapsed | 7 (14) |
Chemotherapy regimen | |
7 + 3 (Conventional or liposomal) | 35 (69) |
Clofarabine + cytarabine | 9 (18) |
Venetoclax + hypomethylator | 3 (6) |
Other | 4 (8) |
Characteristic . | Patients, No. (%)a (n = 51) . |
---|---|
Age, mean (SD), y | 57 (15) |
Sex | |
Male | 30 (59) |
Female | 21 (41) |
Disease phase | |
Newly diagnosed | 44 (86) |
Relapsed | 7 (14) |
Chemotherapy regimen | |
7 + 3 (Conventional or liposomal) | 35 (69) |
Clofarabine + cytarabine | 9 (18) |
Venetoclax + hypomethylator | 3 (6) |
Other | 4 (8) |
Data represent no. (%) of patients unless otherwise specified.
Although each genus was associated with several metabolites (Figure 4 and Supplementary Data 5), Blautia was the only genus that showed exclusively positive associations (Supplementary Data 6), suggesting its unique metabolic relevance. Citrulline was one of 21 associated metabolites associated with Blautia. To exclude the possibility of this relationship being driven primarily by patient-level factors owing to the longitudinal nature of the database, we built a mixed-effects model (lme4 package) in the form of Blautia ∼ patient ID + citrulline, where patient ID was considered a random effect. After controlling for patient ID, Blautia remained a significant predictor of citrulline (regression coefficient, 6.01 [95% confidence interval, .16–10.90]; P = .04). A conventional univariate correlation analysis showed a significant (P = .04) but weak (Pearson R = 0.17) relationship between Blautia and citrulline, indicating the presence of additional regulators of enterocyte mass.
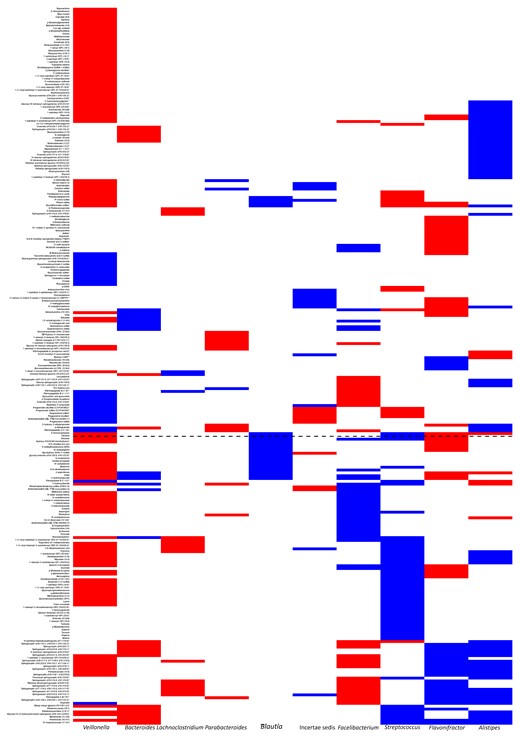
Gut microbiome predictors of next-day metabolome. Results of sparse linear log-contrast modeling using the gut microbiota as predictors of next-day serum metabolome in patients with acute myeloid leukemia. The genera along the x-axis are those that remained in >90 of the 100 bootstraps and showed a uniformly positive or negative association with ≥1 metabolite. Red and blue represent negative and positive associations, respectively. Associations with <90% consistency across the bootstraps are not colored. Blautia is shown in a larger font, and the dashed horizontal line indicates citrulline. This plot is provided in tabular format in Supplementary Data 4 for better visibility of metabolite names.
DISCUSSION
Using a large HCT microbiota database with granular hospitalome and an independent, unique multiomics database in patients with AML, we found evidence for a single-taxon association between Blautia and NF, possibly through strengthening of the intestinal epithelium. Because HCT recipients are severely immunocompromised and at high risk for bleeding and infection early after HCT, mechanistic studies are usually infeasible, so we considered the approach taken here to be the next best alternative.
In one of the earliest microbiota studies in HCT recipients, intestinal Blautia was associated with a reduced mortality rate from acute graft-vs-host disease and improved overall survival [25]. This is consistent with the known anti-inflammatory effects of Blautia [26, 27]. Other metabolic activities of Blautia include bacteriocin production, which can modulate the gut microbiota and prevent pathogen colonization [28, 29]. Our network analysis did not find any community-level changes in the gut microbiota before NF, suggesting a single-taxon phenomenon. Our metabolomic analysis found a strong association between Blautia and circulating citrulline. Citrulline is an amino acid produced exclusively by intestinal epithelial cells [30], with circulating levels indicating total functioning enterocyte mass. Citrulline has been established as a biomarker for gut barrier integrity and function [30, 31], and its low levels in the blood are associated with bacteremia and inflammation [32].
Induction chemotherapy and conditioning regimens used for HCT cause cytotoxic damage to the intestinal epithelium, which may be partially mitigated by the protective microbiota, such as Blautia. Considering the primacy of gut barrier damage in the pathogenesis of acute intestinal graft-vs-host disease [33], this model provides an additional mechanism by which Blautia may protect against fatal intestinal graft-vs-host disease. Our group’s previous work suggested that the expansion of the mucolytic genus Akkermansia may increase the risk of NF through gut barrier modulation [10, 11]. However, while the association between Blautia abundance and freedom from NF was observable in immediately adjacent time points (within 24 hours), Akkermansia associations have been observed over a time span of 5–7 days, suggesting different modes of action on the epithelium. The lack of an association between Blautia and Akkermansia abundances in our network analysis does not support a functional relationship between the 2 genera, though this possibility cannot be excluded.
The current study has some limitations. Because amplicon data do not have reliable species-level resolution [34], we do not know which species of Blautia are involved. In addition, because network analysis was based on the most abundant taxa, subtle community structure differences involving less abundant taxa may have been present between the groups that could not be detected. Citrulline was only one of the metabolites associated with Blautia. We found several other metabolites that could protect against NF, including those involved in amino acid, secondary bile acid, and lipid metabolism. Short-chain fatty acids such as butyrate, produced from dietary fiber by several predominantly anaerobic members of the gut microbiome, have been associated with anti-inflammatory effects through an inhibitory effect on histone deacetylase-mediated pathways and, through metabolism to acetyl coenzyme A, as an enterocyte tricarboxylic acid cycle–mediated energy source, thereby contributing to enterocyte health and mucosal integrity [35]. Although we found no significant association between Blautia and short-chain fatty acids, this possibility is worth further exploration. Finally, the extent to which data from the AML cohort might be help explain the mechanisms implicated in the HCT cohort are unclear at this time, and any extrapolation should be treated with caution.
In conclusion, we propose that NF-relevant effects of the gut microbiota on the intestinal epithelium occur on 2 time scales. A protective effect, perhaps mediated by Blautia, might occur on the faster time scale, where Blautia or its products modulate the health of the epithelium. The absence of an association between Blautia and NF when the interval was increased from 1 to 2 days could indicate a short-lasting effect by a Blautia metabolite with a short half-life. In contrast, the detrimental effect mediated by Akkermansia occurs on the slower time scale where Akkermansia degrades the mucus and thins the intestinal barrier. As an obligate anaerobic commensal of the gut, Blautia depletion has been associated with the use of anti-anaerobic antibiotics and parenteral nutrition [25]. Similarly, dietary fiber deprivation in mice caused expansion of Akkermansia [36]. Therefore, restricted use of anti-anaerobic antibiotics and resumption of fiber-rich oral intake as early as possible could be effective strategies to reduce NF. Selective restoration of Blautia to sustain high levels during the periods of neutropenia in patients at high risk for depletion is another potential strategy for future trials. The precise mechanism by which Blautia may augment intestinal health is unknown and in need of further research.
Supplementary Data
Supplementary materials are available at Clinical Infectious Diseases online. Consisting of data provided by the authors to benefit the reader, the posted materials are not copyedited and are the sole responsibility of the authors, so questions or comments should be addressed to the corresponding author.
Notes
Author contributions. A. R., J. U. P., and C.S. conceived the study and performed the analyses. A. R., J. U. P., M. E., T. U. R., H. E., and L. T. M. collected metadata. H. H., T. K., and C.S. extracted and sequenced DNA for the University of Minnesota database. A. R. wrote the manuscript. J. U. P, M. E., S. G. H, A. K., D. J. W., and C. S. provided critical feedback on the manuscript.
Acknowledgments. Sequence data from University of Minnesota samples were analyzed using the resources of the Minnesota Supercomputing Institute. Serum samples were analyzed for metabolomics by Metabolon.
Disclaimer. The content is solely the responsibility of the authors and does not necessarily represent the official views of the National Institutes of Health’s National Center for Advancing Translational Sciences.
Financial support. This work was supported by the National Center for Advancing Translational Sciences, National Institutes of Health (NIH) (grants KL2TR002492 and UL1TR002494 to the University of Minnesota), NHLBI, NIH (grant K08HL143189 to J. U. P.), the National Cancer Institute, NIH (core grant NCI P30 CA008748 to Memorial Sloan Kettering Cancer Center [MSKCC]), and the Parker Institute for Cancer Immunotherapy at MSKCC (of which J. U. P. is a member).
References
Author notes
Potential conflicts of interest. J. U. P. reports research funding, intellectual property fees, and travel reimbursement from Seres Therapeutics and consulting fees from DaVolterra, CSL Behring, MaaT Pharma, Arjun Pai, and the French National Cancer Institute. He serves on an advisory board for and holds equity in Postbiotics Plus Research. He has filed intellectual property applications related to the microbiome (reference nos. 62/843,849, 62/977,908, and 15/756,845). MSKCC has financial interests relative to Seres Therapeutics. J. U. P. reports the grants or contracts from the following: Society for MSKCC (research grant), Adult BMT Service Translational Research Award (Pilot Grant), Seres Therapeutics (sponsored research), Starr Cancer Consortium, Cycle for Survival Foundation, the National Cancer Institute (2 P01 CA023766 39A1), and the Memorial Sloan Kettering/Hackensack Meridian Health Partnership Immunology Research Immunology Research Collaboration. J. U. P. also reports licensing fees from Seres Therapeutics; payment or honoraria for lectures, presentations, speakers bureaus, manuscript writing, or educational events from the University of Nebraska Medical Center, HayMatick Meetings & Events, the Japanese Society of Hematology, William Blair & Co, KongresKompagniet, the Clinical Immunology Society, the University of Pennsylvania, University Hospital Regensburg, and Hanson Wade; and support for attending meetings and/or travel from the Clinical Immunology Society, the University of Nebraska Medical Center, the University of Pennsylvania, and Parker Institute for Cancer Immunotherapy. J. U. P. asserts 2 patents planned, issued, or pending for β-lactamase compositions for treatment of graft-vs-host disease, and bacterial compositions and methods for cancer survival. Finally, J. U. P. holds stock or stock options with Postbiotics Plus Research. All other authors report no potential conflicts. All authors have submitted the ICMJE Form for Disclosure of Potential Conflicts of Interest. Conflicts that the editors consider relevant to the content of the manuscript have been disclosed.