-
PDF
- Split View
-
Views
-
Cite
Cite
Suparna Das, Adam Allston, Jenevieve Opoku, Michael Kharfen, A Spatial Approach for Ending the Human Immunodeficiency Virus Epidemic for the United States—A DC Model, Clinical Infectious Diseases, Volume 73, Issue 5, 1 September 2021, Pages e1080–e1088, https://doi.org/10.1093/cid/ciaa1761
- Share Icon Share
Abstract
Mode of transmission–based hotspots is a smart approach to HIV mitigation, yet remains poorly evaluated and implemented in the United States. The primary aim was to identifying mode of transmission–based hotspots and populations at risk of lower viral suppression to assist in targeted planning and implementation of programs.
We implemented spatial statistics to identify global-local hotspots and regression analysis to find populations at risk of lower viral suppression within hotspots. Data were obtained from the District of Columbia’s (DC’s) active surveillance system, which were geocoded based on current residence address.
The analysis identified 6001 HIV-positive men who have sex with men (MSM) and 6077 HIV-positive non-MSM (N = 12 078) living in DC at the end of 2018. The hotspots for MSM were central DC and non-MSM in south DC. Trends of viral suppression within MSM hotspots showed plateauing and, among non-MSM, showed decline. Regression analysis showed MSM aged 21–25 (RR: 3.199; 95% CI: 1.832–5.586) and not linked to care (8.592; 2.907–25.398) were at higher risk of being virally unsuppressed within the hotspots. For non-MSM we found those aged 12–18 (9.025; 3.314–2.581) and with unknown linkages (6.087; 3.346–13.848) were at higher risk of being virally unsuppressed within the hotspots.
Our analysis provides a model that may be used by other jurisdictions to identify areas of priority and plan treatment-adherence programs using surveillance data. Attaining viral suppression is crucial in reducing new diagnoses; a spatial approach can be an important tool in Ending the HIV Epidemic.
People living with human immunodeficiency virus (PLHIV) should be receiving HIV medications known as antiretroviral therapy (ART). Adherence to ART reduces the amount of HIV in the body (viral load), significantly enabling proper functioning of the immune system and thus averting illness [1]. This is called viral suppression. Antiretroviral therapy can make the viral load undetectable by test, which is called undetectable viral load. An overwhelming amount of clinical evidence has recognized that, for HIV, undetectable = untransmittable [2]. Thus, it becomes imperative for health departments, in collaboration with the community, to help sustain the undetectable viral load in PLHIV.
Studies have shown that risk-behavior information may play a crucial role in viral suppression of HIV in PLHIV [3–5]. In addition, risk-behavior information is essential for allocating resources and developing effective HIV-prevention-intervention strategies [6]. The absence of risk-behavior information has been a constant barrier in implementing HIV mitigating strategies. In the absence of sufficient behavioral data, mode of transmission provides a basic statistical snapshot to inform health department strategies to attain viral suppression. Evidence has shown disparities in the health outcomes of PLHIV [7, 8]; thus, it is imperative to adopt a spatial approach to viral suppression strategy by jurisdictions across United States.
Spatial analysis offers a rigorous approach to detect spatial hotspots [9]. The identification of hotspots with unusually high disease occurrence helps to evaluate healthcare availability and healthcare operations [10, 11]. Hotspots may be defined as a collection of neighboring entities that are more alike one another compared with objects external to the cluster [12]. Specifically, hotspot analysis is applied to determine places of statistically significant high- and low-value clusters of an occurrence by assessing each feature (eg, census tract) within the context of neighboring features and against all features in the dataset [13]. Implementing prevention planning strategies based on core groups can be challenging because of a lack of standard operational definitions [11]. The primary aim of the analysis was to identify mode of HIV transmission–based hotspots and find populations at risk of lower viral suppression within the hotspots. We also identified the trends of viral suppression within hotspots over years to evaluate if current interventions are effective within the hotspots. Administrations across the United States may use a similar approach to plan for Ending the HIV Epidemic—A Plan for America (EHE), as EHE recommends focusing on geographic and demographic hotspots to plan for HIV mitigation [14].
METHODS
The District of Columbia (DC) law mandates reporting HIV diagnoses by providers and laboratories to the Department of Health (DOH). Reports are received from a variety of sources, including hospitals, private physicians’ offices, community-based organizations, clinics, and laboratories. Data on HIV cases are entered into the enhanced HIV/AIDS Reporting System (eHARS). The data on mode of transmission were collected from the transmission category variable and the definition was used following the Centers for Disease Control and Prevention (CDC) guidelines [15]. We used data for all people living in DC until December 2018 and their concurrent viral suppression status.
We divided PLHIV into 2 groups based on mode of transmission reported to DOH: (1) MSM (includes MSM and MSM who also inject drugs) and (2) non-MSM (all other modes of transmission). We coded the primary outcome variables for the spatial analysis as “MSM” = 1 and “non-MSM” = 2. There are 179 census tracts and 8 wards in DC (Supplementary Figure 1). The analysis was conducted by census tracts, but for visualization, ease of interpretation, and assistance in policymaking, the census tract map was overlaid with the ward shape files. We acquired data from US Census Bureau’s American Community Survey 2018 for the supplementary figures to evaluate the racial, economic, and social disparities at the household-aggregated level in percentages. We obtained the school data from DC Office of the Chief Technology Officer available for public use. (See Supplementary Methodology for details.)
Spatial Statistical Methods
In spatial data analysis, it is often necessary to determine whether or not identifiable spatial patterns exist. We used global Moran’s I and Getis and Ord G* () statistics to identify the global and local patterns of “hotspots” and “cold spots” of the rates in the census tracts of DC (see Supplementary Methodology for details). statistics are used to evaluate the local measures of spatial dependence, which is a focused test used to find a “concentrated area” that is hypothesized to be associated with a disease [16–18]. This well-known spatial method can detect local “pockets” of dependence that may not show up when using global statistics [13, 17, 19]. We used ArcGIS 10.5.1 to identify and calculate the Moran’s I and Getis and Ord G* statistics.
Multinomial Logistic Regression
We opted for multinomial logistic regression to understand the possible factors that may have an association with the viral suppression within and outside the hotspots for MSM and non-MSM (see Supplementary Methodology for details).
Outcome Variables
The outcome variable for the regression model was the viral suppression status of each MSM and non-MSM PLHIV. Viral suppression was defined as less than 200 copies of HIV per milliliter of blood as per the revised CDC guidelines [20]. The viral suppression status was recoded into 4 unordered categories for MSM and non-MSM: (1) virally unsuppressed and located within the hotspots, (2) virally suppressed and located within the hotspots, (3) virally unsuppressed and located outside the identified hotspots, and (4) virally suppressed and located outside the hotspots (base outcome).
Predictor Variables
We classified binary categories of races and current ages of PLHIV. We used current age of PLHIV instead of age at diagnosis for the analysis. The clinical outcomes were grouped into binary categories of (1) link to HIV care within 12+ months, (2) those who were either not linked to care or had missing linkage information, (3) linked within 3 months of diagnosis, (4) linked within 3–6 months of diagnosis, and (5) not retained in any care after linkage to care (poor retention).
RESULTS
Descriptive Statistics of Persons Living With HIV in DC
Table 1 shows that of the 12 078 PLHIV through 2018 in DC, 6001 reported as being MSM and 6077 were categorized as non-MSM based on their mode of transmission. Out of 6077 non-MSM, 87.12% were Black, 4.06% were White, and 4.43% were Hispanic, while 27.86 % of the MSM were Black, 55.94 % were White, and 10.61% were Hispanic. Among the non-MSM and MSM, 32.04% and 29.37% were diagnosed between the ages 30 and 39 years, respectively. Approximately 31.04% of non-MSM and 37.16% of MSM were linked to care after 12 months after diagnosis, while a large percentage of non-MSM (58.04%) and MSM (52.94%) were linked to care within 3 months of their diagnosis. Approximately 78% of non-MSM and 77% of MSM living in DC were retained in care through the end of 2018. The latest viral suppression status of 2018 showed that approximately 65% of non-MSM and 67% of MSM were virally suppressed. We tested global Moran’s I for the aggregated MSM (0.533) and non-MSM (0.417), which indicated spatial autocorrelation with a P value less than .001 (Table 1). Supplementary Figure 2 shows the global clustering of Moran’s I of MSM and non-MSM in DC (Supplementary Figure 2). Based on the distribution map we found the highest rates of MSM diagnoses per 1000 in all wards except for ward 3. Non-MSM were found to be distributed in all wards except for wards 3 and 1. These maps provide a basic visualization without any statistical inference, thus cannot be designated as high-risk hotspots (Figure 1). The distribution maps without statistical inference are not recommended for targeted policy implementation.
Characteristics . | Non-MSM . | MSM . |
---|---|---|
Years of diagnosis | ||
Years before 1990 | 187 (3.08) | 408 (6.80) |
Years between 1991 and 2000 | 1395 (22.96) | 1208 (20.13) |
Years between 2000 and 2010 | 2926 (48.15) | 2737 (45.61) |
Years after 2011 | 1569 (25.82) | 1648 (27.46) |
Race* | ||
White | 247 (4.06) | 1672 (27.86) |
Black | 5294 (87.12) | 3357 (55.94) |
Hispanic | 269 (4.43) | 637 (10.61) |
Other | 267 (4.39) | 335 (5.58) |
Age at diagnosis (years) | ||
Pediatric | 142 (2.34) | 0 (0) |
13–19 | 249 (4.10) | 277 (4.62) |
20–24 | 572 (9.41) | 1019 (16.98) |
25–29 | 722 (11.88) | 1195 (19.91) |
30–39 | 1785 (29.37) | 1923 (32.04) |
40–49 | 1606 (26.43) | 1151 (19.18) |
50–59 | 713 (11.73) | 351 (5.85) |
≥60 | 262 (4.31) | 77 (1.28) |
Missing | 26 (0.43) | 8 (0.13) |
Linkage to care | ||
Linked within 3 months | 3527 (58.04) | 3180 (52.94) |
Linked 3–6 months | 248 (4.08) | 238 (3.96) |
Linked 6–12 months | 275 (4.53) | 289 (4.81) |
Linked after 12 months | 1886 (31.04) | 2232 (37.16) |
Missing linkage information or not linked to care | 141 (2.32) | 62 (1.03) |
Retention status | ||
Not retained | 1328 (21.85) | 1369 (22.81) |
Retained | 4749 (78.15) | 4632 (77.19) |
Latest viral suppression status of PLHIV | ||
Not suppressed | 698 (11.49) | 536 (8.93) |
Suppressed | 3942 (64.87) | 4008 (66.79) |
Unknown | 1437 (23.65) | 1457 (24.28) |
Global Moran’s I*** | 0.533 | 0.417 |
Characteristics . | Non-MSM . | MSM . |
---|---|---|
Years of diagnosis | ||
Years before 1990 | 187 (3.08) | 408 (6.80) |
Years between 1991 and 2000 | 1395 (22.96) | 1208 (20.13) |
Years between 2000 and 2010 | 2926 (48.15) | 2737 (45.61) |
Years after 2011 | 1569 (25.82) | 1648 (27.46) |
Race* | ||
White | 247 (4.06) | 1672 (27.86) |
Black | 5294 (87.12) | 3357 (55.94) |
Hispanic | 269 (4.43) | 637 (10.61) |
Other | 267 (4.39) | 335 (5.58) |
Age at diagnosis (years) | ||
Pediatric | 142 (2.34) | 0 (0) |
13–19 | 249 (4.10) | 277 (4.62) |
20–24 | 572 (9.41) | 1019 (16.98) |
25–29 | 722 (11.88) | 1195 (19.91) |
30–39 | 1785 (29.37) | 1923 (32.04) |
40–49 | 1606 (26.43) | 1151 (19.18) |
50–59 | 713 (11.73) | 351 (5.85) |
≥60 | 262 (4.31) | 77 (1.28) |
Missing | 26 (0.43) | 8 (0.13) |
Linkage to care | ||
Linked within 3 months | 3527 (58.04) | 3180 (52.94) |
Linked 3–6 months | 248 (4.08) | 238 (3.96) |
Linked 6–12 months | 275 (4.53) | 289 (4.81) |
Linked after 12 months | 1886 (31.04) | 2232 (37.16) |
Missing linkage information or not linked to care | 141 (2.32) | 62 (1.03) |
Retention status | ||
Not retained | 1328 (21.85) | 1369 (22.81) |
Retained | 4749 (78.15) | 4632 (77.19) |
Latest viral suppression status of PLHIV | ||
Not suppressed | 698 (11.49) | 536 (8.93) |
Suppressed | 3942 (64.87) | 4008 (66.79) |
Unknown | 1437 (23.65) | 1457 (24.28) |
Global Moran’s I*** | 0.533 | 0.417 |
Data are presented as n (%) unless otherwise indicated. Abbreviations: DC, District of Columbia; HIV, human immunodeficiency virus; MSM, men who have sex with men; PLHIV, people living with human immunodeficiency virus.
Characteristics . | Non-MSM . | MSM . |
---|---|---|
Years of diagnosis | ||
Years before 1990 | 187 (3.08) | 408 (6.80) |
Years between 1991 and 2000 | 1395 (22.96) | 1208 (20.13) |
Years between 2000 and 2010 | 2926 (48.15) | 2737 (45.61) |
Years after 2011 | 1569 (25.82) | 1648 (27.46) |
Race* | ||
White | 247 (4.06) | 1672 (27.86) |
Black | 5294 (87.12) | 3357 (55.94) |
Hispanic | 269 (4.43) | 637 (10.61) |
Other | 267 (4.39) | 335 (5.58) |
Age at diagnosis (years) | ||
Pediatric | 142 (2.34) | 0 (0) |
13–19 | 249 (4.10) | 277 (4.62) |
20–24 | 572 (9.41) | 1019 (16.98) |
25–29 | 722 (11.88) | 1195 (19.91) |
30–39 | 1785 (29.37) | 1923 (32.04) |
40–49 | 1606 (26.43) | 1151 (19.18) |
50–59 | 713 (11.73) | 351 (5.85) |
≥60 | 262 (4.31) | 77 (1.28) |
Missing | 26 (0.43) | 8 (0.13) |
Linkage to care | ||
Linked within 3 months | 3527 (58.04) | 3180 (52.94) |
Linked 3–6 months | 248 (4.08) | 238 (3.96) |
Linked 6–12 months | 275 (4.53) | 289 (4.81) |
Linked after 12 months | 1886 (31.04) | 2232 (37.16) |
Missing linkage information or not linked to care | 141 (2.32) | 62 (1.03) |
Retention status | ||
Not retained | 1328 (21.85) | 1369 (22.81) |
Retained | 4749 (78.15) | 4632 (77.19) |
Latest viral suppression status of PLHIV | ||
Not suppressed | 698 (11.49) | 536 (8.93) |
Suppressed | 3942 (64.87) | 4008 (66.79) |
Unknown | 1437 (23.65) | 1457 (24.28) |
Global Moran’s I*** | 0.533 | 0.417 |
Characteristics . | Non-MSM . | MSM . |
---|---|---|
Years of diagnosis | ||
Years before 1990 | 187 (3.08) | 408 (6.80) |
Years between 1991 and 2000 | 1395 (22.96) | 1208 (20.13) |
Years between 2000 and 2010 | 2926 (48.15) | 2737 (45.61) |
Years after 2011 | 1569 (25.82) | 1648 (27.46) |
Race* | ||
White | 247 (4.06) | 1672 (27.86) |
Black | 5294 (87.12) | 3357 (55.94) |
Hispanic | 269 (4.43) | 637 (10.61) |
Other | 267 (4.39) | 335 (5.58) |
Age at diagnosis (years) | ||
Pediatric | 142 (2.34) | 0 (0) |
13–19 | 249 (4.10) | 277 (4.62) |
20–24 | 572 (9.41) | 1019 (16.98) |
25–29 | 722 (11.88) | 1195 (19.91) |
30–39 | 1785 (29.37) | 1923 (32.04) |
40–49 | 1606 (26.43) | 1151 (19.18) |
50–59 | 713 (11.73) | 351 (5.85) |
≥60 | 262 (4.31) | 77 (1.28) |
Missing | 26 (0.43) | 8 (0.13) |
Linkage to care | ||
Linked within 3 months | 3527 (58.04) | 3180 (52.94) |
Linked 3–6 months | 248 (4.08) | 238 (3.96) |
Linked 6–12 months | 275 (4.53) | 289 (4.81) |
Linked after 12 months | 1886 (31.04) | 2232 (37.16) |
Missing linkage information or not linked to care | 141 (2.32) | 62 (1.03) |
Retention status | ||
Not retained | 1328 (21.85) | 1369 (22.81) |
Retained | 4749 (78.15) | 4632 (77.19) |
Latest viral suppression status of PLHIV | ||
Not suppressed | 698 (11.49) | 536 (8.93) |
Suppressed | 3942 (64.87) | 4008 (66.79) |
Unknown | 1437 (23.65) | 1457 (24.28) |
Global Moran’s I*** | 0.533 | 0.417 |
Data are presented as n (%) unless otherwise indicated. Abbreviations: DC, District of Columbia; HIV, human immunodeficiency virus; MSM, men who have sex with men; PLHIV, people living with human immunodeficiency virus.
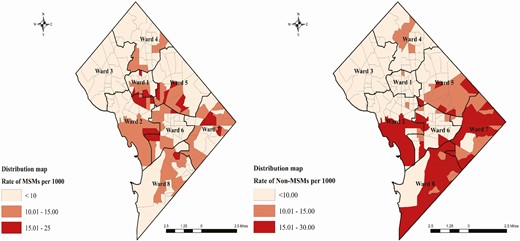
Distribution of MSM and non-MSM with HIV in DC. Abbreviations: DC, District of Columbia; HIV, human immunodeficiency virus; MSM, men who have sex with men.
Geographic Hotspots Using Spatial Statistics
Figure 2 shows the local hotspots and cold spots detected for MSM and non-MSM in the tracts of DC. For statistically significant positive z-scores, the higher the z-score, the stronger the clustering of high values (hotspot). For statistically significant negative z-scores, the lesser the z-score is, the stronger the concentration of low values (cold spot). Tracts of central DC covering adjoining parts of wards 1, 2, and 5 were identified as MSM hotspots at 99% confidence with a corresponding z-score of more than 2.38, while tracts in ward 3 and parts of ward 4 show the cold spots at a 99% confidence interval. Non-MSM hotspots were located in south and southeast DC covering tracts primarily of wards 7 and 8 at a confidence of 99%, with a corresponding z-score of more than 2.38. Cold spots for non-MSM were found in north and parts of central DC covering adjacent tracts of wards 1, 2, and 3 at 99% confidence. For our purposes, we were particularly interested in hotspots to plan for geographically targeted EHE (Figure 2).

Geographic hotspots of MSM and non-MSM with HIV in DC through 2018. Abbreviations: DC, District of Columbia; HIV, human immunodeficiency virus; MSM, men who have sex with men.
Temporal Trends of Viral Suppression Within Geographic Hotspots
We calculated the percentages of viral suppression status and plotted them to identify trends within and outside the hotspots over years. Figure 3 shows that, within non-MSM hotspots, the percentage of virally unsuppressed PLHIV trends upward in recent years. Among MSM, the trend of poor outcomes of viral suppression was found to be plateauing both within and outside the hotspots. (Figure 3). Both of these trends indicate that intervention strategies are probably either not optimized or we need to evaluate the efficacy of the current strategies.
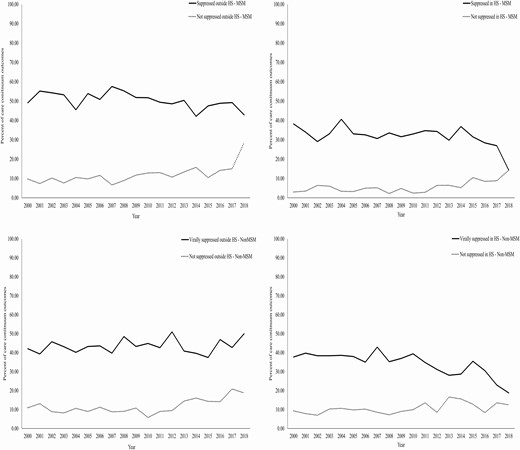
Trends of viral suppression within and outside the hotspots. Abbreviations: HS, hotspots; MSM, men who have sex with men.
Regression Analysis to Identify Populations at Risk Within Geographic Hotspots
Tables 2 and 3 show the results of the regression analysis. It was interesting to note that MSM ages 21–25 (young MSM) (RR: 3.199; 95% confidence interval [CI]: 1.832–5.586) were at the highest risk of being virally unsuppressed within the hotspots. Youth aged 19–20 (RR: 5.018; 95% CI: 1.295–19.436) were found to be at higher risk outside of the geographic hotspots compared with those within the hotspots. It was also interesting to find that, among MSM, whites (RR: 2.209; 95% CI: 1.685–2.896) showed a higher likelihood of being virally suppressed outside the hotspots. MSM with unknown linkage status outside the hotspots (RR: 17.716; 95% CI: 6.572–47.756) and inside the hotspots (RR: 8.592; 95% CI: 2.907–25.398) were both at higher risk of being virally unsuppressed (Table 2).
Results From Regression Analysis of Viral Suppression Status Among MSM in DC
. | Viral Suppression Status Among MSM . | ||||||||
---|---|---|---|---|---|---|---|---|---|
. | Not Suppressed Within Hotspots . | Not Suppressed Outside Hotspots . | Suppressed in Hotspots . | ||||||
. | RR . | Std Error . | 95% CI . | RR . | Std Error . | 95% CI . | RR . | Std Error . | 95% CI . |
White | .742 | .207 | .429–1.283 | .375*** | .091 | .232–.605 | 2.209*** | .305 | 1.685–2.896 |
Hispanic | .693 | .213 | .379–1.267 | .665 | .164 | .410–1.080 | 1.309 | .201 | .969–1.769 |
Black | .773 | .194 | .472–1.264 | 1.142 | .225 | .775–1.681 | .575*** | .077 | .442–.749 |
Current age (years) | |||||||||
12–18 | .000007 | .002 | .000–.020 | 2.934 | 2.510 | .549–15.693 | .546 | .599 | .064–4.686 |
19–20 | 3.284 | 3.626 | .377–28.602 | 5.018** | 3.467 | 1.296–19.437 | .909 | .658 | .220–3.759 |
21–25 | 3.199*** | .910 | 1.832–5.586 | 2.668*** | .573 | 1.751–4.063 | .854 | .165 | .585–1.247 |
26–30 | 2.182*** | .429 | 1.483–3.209 | 2.667*** | .353 | 2.057–3.457 | .732** | .083 | .586–0.915 |
31–40 | 2.337*** | .348 | 1.745–3.129 | 1.768*** | .203 | 1.412–2.215 | 1.032 | .078 | .889–1.198 |
Unknown linkage information | 8.592*** | 4.751 | 2.907–25.398 | 17.716*** | 8.963 | 6.572–47.756 | .609 | .446 | .146–2.555 |
Linkage to care 3 months after diagnosis | .612 | .158 | .369–1.016 | .955 | .214 | .615–1.482 | 1.056 | .155 | .792–1.407 |
Linkage to care 3–6 months after diagnosis | .532 | .226 | .232–1.223 | 1.246 | .373 | .693–2.240 | 1.054 | .220 | .701–1.588 |
Linkage to care 12 months after diagnosis | .734 | .195 | .436–1.236 | 1.168 | .267 | .746–1.829 | 1.003 | .150 | .748–1.345 |
Not retained in care | .335*** | .046 | .254–.436 | .386*** | .041 | .314–.476 | .854* | .064 | .737–.990 |
Constant | .252*** | .090 | .125–.506 | .272*** | .081 | .152–.488 | .699 | .139 | .474–1.034 |
AIC | 11 733.1 | ||||||||
BIC | 12 013.58 | ||||||||
Log likelihood | −5824.5476 |
. | Viral Suppression Status Among MSM . | ||||||||
---|---|---|---|---|---|---|---|---|---|
. | Not Suppressed Within Hotspots . | Not Suppressed Outside Hotspots . | Suppressed in Hotspots . | ||||||
. | RR . | Std Error . | 95% CI . | RR . | Std Error . | 95% CI . | RR . | Std Error . | 95% CI . |
White | .742 | .207 | .429–1.283 | .375*** | .091 | .232–.605 | 2.209*** | .305 | 1.685–2.896 |
Hispanic | .693 | .213 | .379–1.267 | .665 | .164 | .410–1.080 | 1.309 | .201 | .969–1.769 |
Black | .773 | .194 | .472–1.264 | 1.142 | .225 | .775–1.681 | .575*** | .077 | .442–.749 |
Current age (years) | |||||||||
12–18 | .000007 | .002 | .000–.020 | 2.934 | 2.510 | .549–15.693 | .546 | .599 | .064–4.686 |
19–20 | 3.284 | 3.626 | .377–28.602 | 5.018** | 3.467 | 1.296–19.437 | .909 | .658 | .220–3.759 |
21–25 | 3.199*** | .910 | 1.832–5.586 | 2.668*** | .573 | 1.751–4.063 | .854 | .165 | .585–1.247 |
26–30 | 2.182*** | .429 | 1.483–3.209 | 2.667*** | .353 | 2.057–3.457 | .732** | .083 | .586–0.915 |
31–40 | 2.337*** | .348 | 1.745–3.129 | 1.768*** | .203 | 1.412–2.215 | 1.032 | .078 | .889–1.198 |
Unknown linkage information | 8.592*** | 4.751 | 2.907–25.398 | 17.716*** | 8.963 | 6.572–47.756 | .609 | .446 | .146–2.555 |
Linkage to care 3 months after diagnosis | .612 | .158 | .369–1.016 | .955 | .214 | .615–1.482 | 1.056 | .155 | .792–1.407 |
Linkage to care 3–6 months after diagnosis | .532 | .226 | .232–1.223 | 1.246 | .373 | .693–2.240 | 1.054 | .220 | .701–1.588 |
Linkage to care 12 months after diagnosis | .734 | .195 | .436–1.236 | 1.168 | .267 | .746–1.829 | 1.003 | .150 | .748–1.345 |
Not retained in care | .335*** | .046 | .254–.436 | .386*** | .041 | .314–.476 | .854* | .064 | .737–.990 |
Constant | .252*** | .090 | .125–.506 | .272*** | .081 | .152–.488 | .699 | .139 | .474–1.034 |
AIC | 11 733.1 | ||||||||
BIC | 12 013.58 | ||||||||
Log likelihood | −5824.5476 |
Abbreviations: AIC, Akaike Information Criterion; BIC, Bayesian Information Criterion CI, confidence interval; DC, District of Columbia; MSM, men who have sex with men; RR, Relative Risk; Std, standard.
Note: Suppressed outside the hotspots (Base outcome).
***P value <.001.
Results From Regression Analysis of Viral Suppression Status Among MSM in DC
. | Viral Suppression Status Among MSM . | ||||||||
---|---|---|---|---|---|---|---|---|---|
. | Not Suppressed Within Hotspots . | Not Suppressed Outside Hotspots . | Suppressed in Hotspots . | ||||||
. | RR . | Std Error . | 95% CI . | RR . | Std Error . | 95% CI . | RR . | Std Error . | 95% CI . |
White | .742 | .207 | .429–1.283 | .375*** | .091 | .232–.605 | 2.209*** | .305 | 1.685–2.896 |
Hispanic | .693 | .213 | .379–1.267 | .665 | .164 | .410–1.080 | 1.309 | .201 | .969–1.769 |
Black | .773 | .194 | .472–1.264 | 1.142 | .225 | .775–1.681 | .575*** | .077 | .442–.749 |
Current age (years) | |||||||||
12–18 | .000007 | .002 | .000–.020 | 2.934 | 2.510 | .549–15.693 | .546 | .599 | .064–4.686 |
19–20 | 3.284 | 3.626 | .377–28.602 | 5.018** | 3.467 | 1.296–19.437 | .909 | .658 | .220–3.759 |
21–25 | 3.199*** | .910 | 1.832–5.586 | 2.668*** | .573 | 1.751–4.063 | .854 | .165 | .585–1.247 |
26–30 | 2.182*** | .429 | 1.483–3.209 | 2.667*** | .353 | 2.057–3.457 | .732** | .083 | .586–0.915 |
31–40 | 2.337*** | .348 | 1.745–3.129 | 1.768*** | .203 | 1.412–2.215 | 1.032 | .078 | .889–1.198 |
Unknown linkage information | 8.592*** | 4.751 | 2.907–25.398 | 17.716*** | 8.963 | 6.572–47.756 | .609 | .446 | .146–2.555 |
Linkage to care 3 months after diagnosis | .612 | .158 | .369–1.016 | .955 | .214 | .615–1.482 | 1.056 | .155 | .792–1.407 |
Linkage to care 3–6 months after diagnosis | .532 | .226 | .232–1.223 | 1.246 | .373 | .693–2.240 | 1.054 | .220 | .701–1.588 |
Linkage to care 12 months after diagnosis | .734 | .195 | .436–1.236 | 1.168 | .267 | .746–1.829 | 1.003 | .150 | .748–1.345 |
Not retained in care | .335*** | .046 | .254–.436 | .386*** | .041 | .314–.476 | .854* | .064 | .737–.990 |
Constant | .252*** | .090 | .125–.506 | .272*** | .081 | .152–.488 | .699 | .139 | .474–1.034 |
AIC | 11 733.1 | ||||||||
BIC | 12 013.58 | ||||||||
Log likelihood | −5824.5476 |
. | Viral Suppression Status Among MSM . | ||||||||
---|---|---|---|---|---|---|---|---|---|
. | Not Suppressed Within Hotspots . | Not Suppressed Outside Hotspots . | Suppressed in Hotspots . | ||||||
. | RR . | Std Error . | 95% CI . | RR . | Std Error . | 95% CI . | RR . | Std Error . | 95% CI . |
White | .742 | .207 | .429–1.283 | .375*** | .091 | .232–.605 | 2.209*** | .305 | 1.685–2.896 |
Hispanic | .693 | .213 | .379–1.267 | .665 | .164 | .410–1.080 | 1.309 | .201 | .969–1.769 |
Black | .773 | .194 | .472–1.264 | 1.142 | .225 | .775–1.681 | .575*** | .077 | .442–.749 |
Current age (years) | |||||||||
12–18 | .000007 | .002 | .000–.020 | 2.934 | 2.510 | .549–15.693 | .546 | .599 | .064–4.686 |
19–20 | 3.284 | 3.626 | .377–28.602 | 5.018** | 3.467 | 1.296–19.437 | .909 | .658 | .220–3.759 |
21–25 | 3.199*** | .910 | 1.832–5.586 | 2.668*** | .573 | 1.751–4.063 | .854 | .165 | .585–1.247 |
26–30 | 2.182*** | .429 | 1.483–3.209 | 2.667*** | .353 | 2.057–3.457 | .732** | .083 | .586–0.915 |
31–40 | 2.337*** | .348 | 1.745–3.129 | 1.768*** | .203 | 1.412–2.215 | 1.032 | .078 | .889–1.198 |
Unknown linkage information | 8.592*** | 4.751 | 2.907–25.398 | 17.716*** | 8.963 | 6.572–47.756 | .609 | .446 | .146–2.555 |
Linkage to care 3 months after diagnosis | .612 | .158 | .369–1.016 | .955 | .214 | .615–1.482 | 1.056 | .155 | .792–1.407 |
Linkage to care 3–6 months after diagnosis | .532 | .226 | .232–1.223 | 1.246 | .373 | .693–2.240 | 1.054 | .220 | .701–1.588 |
Linkage to care 12 months after diagnosis | .734 | .195 | .436–1.236 | 1.168 | .267 | .746–1.829 | 1.003 | .150 | .748–1.345 |
Not retained in care | .335*** | .046 | .254–.436 | .386*** | .041 | .314–.476 | .854* | .064 | .737–.990 |
Constant | .252*** | .090 | .125–.506 | .272*** | .081 | .152–.488 | .699 | .139 | .474–1.034 |
AIC | 11 733.1 | ||||||||
BIC | 12 013.58 | ||||||||
Log likelihood | −5824.5476 |
Abbreviations: AIC, Akaike Information Criterion; BIC, Bayesian Information Criterion CI, confidence interval; DC, District of Columbia; MSM, men who have sex with men; RR, Relative Risk; Std, standard.
Note: Suppressed outside the hotspots (Base outcome).
***P value <.001.
Results from Regression Analysis of Viral Suppression Status Among Non-MSMs in DC
. | Viral Suppression Status Among Non-MSM . | |||||||||
---|---|---|---|---|---|---|---|---|---|---|
. | Not Suppressed Within Hotspots . | Not Suppressed Outside Hotspots . | Suppressed in Hotspots . | Suppressed Outside Hotspots (Base Outcome) . | ||||||
. | RR . | Std Error . | 95% CI . | RR . | Std Error . | 95% CI . | RR . | Std Error . | 95% CI . | . |
White | .132*** | .063 | .052–.334 | .485* | .170 | .244–.963 | .125*** | .035 | .072–.216 | |
Hispanic | .254*** | .098 | .119–.539 | 1.250 | .376 | .694–2.252 | .318*** | .071 | .206–.493 | |
Black | 1.179 | .262 | .763–1.821 | 1.403 | .344 | .868–2.269 | 1.327 | .187 | 1.007–1.749 | |
Current age (years) | ||||||||||
12–18 | 9.025*** | 4.614 | 3.314–24.581 | 6.863*** | 3.715 | 2.375–19.827 | 2.013 | .964 | .787–5.146 | |
19–20 | 2.739 | 1.420 | .992–7.566 | 1.338 | .794 | .419–4.279 | 1.455 | .594 | .653–3.240 | |
21–25 | 3.858*** | .858 | 2.495–5.964 | 1.525 | .423 | .886–2.625 | 1.413 | .269 | .973–2.052 | |
26–30 | 2.966*** | .548 | 2.066–4.260 | 1.907*** | .387 | 1.281–2.838 | 1.418** | .202 | 1.073–1.874 | |
31–40 | 2.099*** | .257 | 1.651–2.669 | 2.043*** | .242 | 1.619–2.577 | 1.282** | .109 | 1.085–1.515 | |
Unknown linkage information | 6.807*** | 2.467 | 3.346–13.848 | 8.436*** | 3.061 | 4.143–17.181 | .813 | .363 | .339–1.949 | |
Linkage to care 3 months after diagnosis | .595** | .125 | .394–.898 | .840 | .193 | .536–1.318 | .906 | .131 | .682–1.204 | |
Linkage to care 3–6 months after diagnosis | .884 | .260 | .497–1.571 | .845 | .278 | .443–1.610 | 1.044 | .210 | .703–1.548 | |
Linkage to care 12 months after diagnosis | .763 | .166 | .498–1.169 | 1.079 | .255 | .679–1.714 | .988 | .148 | .737–1.324 | |
Not retained in care | .554*** | .060 | .448–.686 | .370*** | .038 | .303–.452 | 1.264** | .100 | 1.082–1.477 | |
Constant | .358*** | .111 | .195–.656 | .301*** | .101 | .156–.581 | .594** | .124 | .395–.894 | |
AIC | 13 703.14 | |||||||||
BIC | 13 985.05 | |||||||||
Log likelihood | −6809.5697 |
. | Viral Suppression Status Among Non-MSM . | |||||||||
---|---|---|---|---|---|---|---|---|---|---|
. | Not Suppressed Within Hotspots . | Not Suppressed Outside Hotspots . | Suppressed in Hotspots . | Suppressed Outside Hotspots (Base Outcome) . | ||||||
. | RR . | Std Error . | 95% CI . | RR . | Std Error . | 95% CI . | RR . | Std Error . | 95% CI . | . |
White | .132*** | .063 | .052–.334 | .485* | .170 | .244–.963 | .125*** | .035 | .072–.216 | |
Hispanic | .254*** | .098 | .119–.539 | 1.250 | .376 | .694–2.252 | .318*** | .071 | .206–.493 | |
Black | 1.179 | .262 | .763–1.821 | 1.403 | .344 | .868–2.269 | 1.327 | .187 | 1.007–1.749 | |
Current age (years) | ||||||||||
12–18 | 9.025*** | 4.614 | 3.314–24.581 | 6.863*** | 3.715 | 2.375–19.827 | 2.013 | .964 | .787–5.146 | |
19–20 | 2.739 | 1.420 | .992–7.566 | 1.338 | .794 | .419–4.279 | 1.455 | .594 | .653–3.240 | |
21–25 | 3.858*** | .858 | 2.495–5.964 | 1.525 | .423 | .886–2.625 | 1.413 | .269 | .973–2.052 | |
26–30 | 2.966*** | .548 | 2.066–4.260 | 1.907*** | .387 | 1.281–2.838 | 1.418** | .202 | 1.073–1.874 | |
31–40 | 2.099*** | .257 | 1.651–2.669 | 2.043*** | .242 | 1.619–2.577 | 1.282** | .109 | 1.085–1.515 | |
Unknown linkage information | 6.807*** | 2.467 | 3.346–13.848 | 8.436*** | 3.061 | 4.143–17.181 | .813 | .363 | .339–1.949 | |
Linkage to care 3 months after diagnosis | .595** | .125 | .394–.898 | .840 | .193 | .536–1.318 | .906 | .131 | .682–1.204 | |
Linkage to care 3–6 months after diagnosis | .884 | .260 | .497–1.571 | .845 | .278 | .443–1.610 | 1.044 | .210 | .703–1.548 | |
Linkage to care 12 months after diagnosis | .763 | .166 | .498–1.169 | 1.079 | .255 | .679–1.714 | .988 | .148 | .737–1.324 | |
Not retained in care | .554*** | .060 | .448–.686 | .370*** | .038 | .303–.452 | 1.264** | .100 | 1.082–1.477 | |
Constant | .358*** | .111 | .195–.656 | .301*** | .101 | .156–.581 | .594** | .124 | .395–.894 | |
AIC | 13 703.14 | |||||||||
BIC | 13 985.05 | |||||||||
Log likelihood | −6809.5697 |
Abbreviations: AIC, ; BIC, ; CI, confidence interval; DC, District of Columbia; MSM, men who have sex with men; RR, ; Std, standard.
Results from Regression Analysis of Viral Suppression Status Among Non-MSMs in DC
. | Viral Suppression Status Among Non-MSM . | |||||||||
---|---|---|---|---|---|---|---|---|---|---|
. | Not Suppressed Within Hotspots . | Not Suppressed Outside Hotspots . | Suppressed in Hotspots . | Suppressed Outside Hotspots (Base Outcome) . | ||||||
. | RR . | Std Error . | 95% CI . | RR . | Std Error . | 95% CI . | RR . | Std Error . | 95% CI . | . |
White | .132*** | .063 | .052–.334 | .485* | .170 | .244–.963 | .125*** | .035 | .072–.216 | |
Hispanic | .254*** | .098 | .119–.539 | 1.250 | .376 | .694–2.252 | .318*** | .071 | .206–.493 | |
Black | 1.179 | .262 | .763–1.821 | 1.403 | .344 | .868–2.269 | 1.327 | .187 | 1.007–1.749 | |
Current age (years) | ||||||||||
12–18 | 9.025*** | 4.614 | 3.314–24.581 | 6.863*** | 3.715 | 2.375–19.827 | 2.013 | .964 | .787–5.146 | |
19–20 | 2.739 | 1.420 | .992–7.566 | 1.338 | .794 | .419–4.279 | 1.455 | .594 | .653–3.240 | |
21–25 | 3.858*** | .858 | 2.495–5.964 | 1.525 | .423 | .886–2.625 | 1.413 | .269 | .973–2.052 | |
26–30 | 2.966*** | .548 | 2.066–4.260 | 1.907*** | .387 | 1.281–2.838 | 1.418** | .202 | 1.073–1.874 | |
31–40 | 2.099*** | .257 | 1.651–2.669 | 2.043*** | .242 | 1.619–2.577 | 1.282** | .109 | 1.085–1.515 | |
Unknown linkage information | 6.807*** | 2.467 | 3.346–13.848 | 8.436*** | 3.061 | 4.143–17.181 | .813 | .363 | .339–1.949 | |
Linkage to care 3 months after diagnosis | .595** | .125 | .394–.898 | .840 | .193 | .536–1.318 | .906 | .131 | .682–1.204 | |
Linkage to care 3–6 months after diagnosis | .884 | .260 | .497–1.571 | .845 | .278 | .443–1.610 | 1.044 | .210 | .703–1.548 | |
Linkage to care 12 months after diagnosis | .763 | .166 | .498–1.169 | 1.079 | .255 | .679–1.714 | .988 | .148 | .737–1.324 | |
Not retained in care | .554*** | .060 | .448–.686 | .370*** | .038 | .303–.452 | 1.264** | .100 | 1.082–1.477 | |
Constant | .358*** | .111 | .195–.656 | .301*** | .101 | .156–.581 | .594** | .124 | .395–.894 | |
AIC | 13 703.14 | |||||||||
BIC | 13 985.05 | |||||||||
Log likelihood | −6809.5697 |
. | Viral Suppression Status Among Non-MSM . | |||||||||
---|---|---|---|---|---|---|---|---|---|---|
. | Not Suppressed Within Hotspots . | Not Suppressed Outside Hotspots . | Suppressed in Hotspots . | Suppressed Outside Hotspots (Base Outcome) . | ||||||
. | RR . | Std Error . | 95% CI . | RR . | Std Error . | 95% CI . | RR . | Std Error . | 95% CI . | . |
White | .132*** | .063 | .052–.334 | .485* | .170 | .244–.963 | .125*** | .035 | .072–.216 | |
Hispanic | .254*** | .098 | .119–.539 | 1.250 | .376 | .694–2.252 | .318*** | .071 | .206–.493 | |
Black | 1.179 | .262 | .763–1.821 | 1.403 | .344 | .868–2.269 | 1.327 | .187 | 1.007–1.749 | |
Current age (years) | ||||||||||
12–18 | 9.025*** | 4.614 | 3.314–24.581 | 6.863*** | 3.715 | 2.375–19.827 | 2.013 | .964 | .787–5.146 | |
19–20 | 2.739 | 1.420 | .992–7.566 | 1.338 | .794 | .419–4.279 | 1.455 | .594 | .653–3.240 | |
21–25 | 3.858*** | .858 | 2.495–5.964 | 1.525 | .423 | .886–2.625 | 1.413 | .269 | .973–2.052 | |
26–30 | 2.966*** | .548 | 2.066–4.260 | 1.907*** | .387 | 1.281–2.838 | 1.418** | .202 | 1.073–1.874 | |
31–40 | 2.099*** | .257 | 1.651–2.669 | 2.043*** | .242 | 1.619–2.577 | 1.282** | .109 | 1.085–1.515 | |
Unknown linkage information | 6.807*** | 2.467 | 3.346–13.848 | 8.436*** | 3.061 | 4.143–17.181 | .813 | .363 | .339–1.949 | |
Linkage to care 3 months after diagnosis | .595** | .125 | .394–.898 | .840 | .193 | .536–1.318 | .906 | .131 | .682–1.204 | |
Linkage to care 3–6 months after diagnosis | .884 | .260 | .497–1.571 | .845 | .278 | .443–1.610 | 1.044 | .210 | .703–1.548 | |
Linkage to care 12 months after diagnosis | .763 | .166 | .498–1.169 | 1.079 | .255 | .679–1.714 | .988 | .148 | .737–1.324 | |
Not retained in care | .554*** | .060 | .448–.686 | .370*** | .038 | .303–.452 | 1.264** | .100 | 1.082–1.477 | |
Constant | .358*** | .111 | .195–.656 | .301*** | .101 | .156–.581 | .594** | .124 | .395–.894 | |
AIC | 13 703.14 | |||||||||
BIC | 13 985.05 | |||||||||
Log likelihood | −6809.5697 |
Abbreviations: AIC, ; BIC, ; CI, confidence interval; DC, District of Columbia; MSM, men who have sex with men; RR, ; Std, standard.
Table 3 shows the results for non-MSM. We found that those in the younger age group of 12–18 (RR: 9.025; 95% CI: 3.314–24.581) were at higher risk of being virally unsuppressed within the hotspots. PLHIV with unknown linkage post diagnosis (RR: 6.807; 95% CI: 3.345–13.848) were at higher risk of being virally unsuppressed within the hotspots. Unknown linkage to care outside the geographic hotspots (RR: 8.436; 95% CI: 4.142–13.848) also showed a higher risk of poor viral suppression outcomes.
Disparities Across Tracts of DC
Supplementary Figure 3 shows the percentage distribution of races, unemployment, school dropouts, and internet access across tracts of DC, which may provide context for a multilevel approach to HIV-prevention intervention. The non-MSM hotspots have a higher percentage of Black individuals, while MSM hotspots have larger percentages of White individuals. Few tracts of MSM hotspots also showed larger percentages of Hispanic individuals in ward 1, while tracts in ward 5 showed a larger percentage of Black individuals. Supplementary Figure 3B showed a higher percentage of poverty in ward 1 of MSM hotspots and ward 8 of non-MSM hotspots. Unemployment was found to be higher in the non-MSM hotspots. Supplementary Figure 3C also showed higher percentages of high-school dropouts in the non-MSM hotspots and shows the location of schools and colleges within the hotspots for MSM and non-MSM, which can be instrumental in policy implementation.
DISCUSSION
Detecting the mode of transmission–based hotspots is a smart approach for local HIV-prevention intervention where surveillance data may be available instead of using case-by-case investigation. We used DC data to model our analysis. These hotspots are strongly recommended to be investigated to understand the underlying etiology of the clustering. We also used individual demographical and care continuum variables to evaluate the association of viral suppression within these hotspots. We found that spatial hotspots of MSM were located in the tracts of central DC, while the analysis found non-MSM hotspots in south and southeast DC. Mode of transmission–based hotspot analysis lacks precedence, and our analysis fills an important gap that can be replicated by jurisdictions using local surveillance data.
Poor outcomes of viral suppression play a major role in sustaining HIV burden within any jurisdiction. Addressing the geographic and demographic hotspots of poor viral suppression may significantly improve HIV outcomes. While we identified the hotspots, we witnessed a disturbing upward trend of the percentage of virally unsuppressed PLHIV within the non-MSM hotspots, while viral suppression status plateaus in the MSM hotspots. In order to further progress towards achieving the goals of EHE, attention needs to be paid to these hotspots and the younger population within these hotspots. Our results indicate that teenagers aged 12–18 who are not linked to care were at higher risk of being virally unsuppressed within the non-MSM hotspots. Our results also identified that youth aged 21–24 were at higher risk of being virally unsuppressed within the MSM hotspots. Based on the DC Youth Risk Behavioral Survey (YRBS), a CDC study of health-risk behaviors conducted every 2 years, an increase in the percentage of middle-school students who had sex during their lifetime was reported [21]. The percentage of youth who have ever been sexually active and the percentage who have been tested for sexually transmitted diseases (STDs) or HIV infection were significantly higher among youth in higher grade levels. The YRBS also reports that female high-school youth were less likely (48.7%) to use condoms than their male counterparts (72.2%) [21].
Linkage to care plays a key role in the HIV care continuum: it is a necessary precursor to ART initiation and viral suppression. Delayed linkage to care is a major barrier to “treatment as prevention” to reduce HIV transmission rates in the United States. Thus, identifying persons with HIV and successfully linking them to care plays a key role in the overall HIV epidemic, both from a treatment and a prevention standpoint [22]. The analysis showed that, at a local level, unknown linkages increased the risk of poorer outcomes of viral suppression. In the following sections we evaluate the current strategies that have been implemented and make recommendations to optimize the strategies as well as recommend some new strategies to achieve better outcomes for viral suppression among PLHIV.
Current Policies Implemented to Address Youth HIV-Prevention Intervention in DC
Supplementary Figure 2 shows the HIV epi-curve of DC, which changed over time with the implementation of major programs in DC. As the jurisdiction aims to end the HIV epidemic, the analysis would be important for planning purposes. DC, so far, has implemented homogeneous policies on youth across the city. One of the primary efforts in addressing youth-based HIV prevention is DC’s Youth and HIV Prevention Initiative Plan in an effort to define and coordinate needed resources to address the HIV epidemic in DC. The highlights of the plan include the launch of the ShowOff social marketing campaign, aimed at promoting positive attitudes and awareness around sexual health. Based on our findings, we recommend that the hotspots be prioritized for the ShowOff social marketing campaign, particularly around educational institutions. Youth with historically low service utilization were reached through a variety of community-based organization (CBO) and government initiatives to increase education, outreach, and testing. We recommend that CBOs consider the hotspots as high-priority zones for their activities. DC successfully piloted 2 pop-up STD screening at 2 District of Columbia Public Schools (DCPS); based on our results we recommend expansion of HIV testing to schools located within the identified hotspots, which may also include, but are not limited to, mobile testing vans [23]. DC expanded condom-availability programs to include all District of Columbia Public Schools (DCPS) high schools and continues to expand programs at public charter schools; based on our results, we recommend that condoms be made available to middle schoolers in the non-MSM hotspot areas. Despite steep cuts in federal support for youth-based HIV-prevention activities, including reduction in the number of testing counsellors, DC has secured prevention funding to support youth peer education and capacity building initiatives at various CBOs. As the part of the youth plan DC aims to identify options for insurance coverage for those under 26 without parental connection or with a need for insurance outside of their parents’ policy; this would be instrumental as our results identified MSM youth aged 21–25 to be at higher risk of being virally unsuppressed, particularly within the hotspots.
Future Recommendations and Limitations
The analysis should be used for “data-to-care” planning activities. “Data-to-care” is a CDC-endorsed public health strategy that uses HIV surveillance data to identify HIV-diagnosed individuals not in care and link them to treatment that will encourage viral suppression as well as reduce HIV-related health disparities and health inequalities. The DC EHE 2017 progress report mentions the need to expand access to treatment and related services, targeting demographics and geographic areas where populations are at higher risk [24].
Outreach activities for treatment adherence engaging Hispanics and Black PLHIV within the MSM hotspots are strongly recommended. School-based teenagers were at higher risk of being virally suppressed, so we recommend that public schools and charter schools in DC support treatment adherence in school-aged PLHIV. The higher percentage of high school dropouts in non-MSM hotspots also indicates truancy, which increases risks of poor outcomes of viral suppression. The schools, colleges, and universities across DC located in the hotspots can play a major role in attaining viral suppression among PLHIV. Within the MSM hotspots, colleges and universities may play a major role in assisting treatment adherence in youth aged 21–26. As administrations across the United States move to text- and mobile-based treatment adherence strategies, especially during the current coronavirus disease 2019 (COVID-19) pandemic, we need to evaluate the digital divide, which may act as a major barrier. While we recommend internet-based treatment adherence strategies for MSM hotspots, this method may not be optimal in the non-MSM hotspots. We recommend partnering with local schools and parent-teacher organizations to increase treatment adherence and decrease truancy in the non-MSM hotspots.
The results of the regression analysis showed individual outcomes that need to be evaluated at the community level to plan community-based prevention interventions. Although there are various other methods of hotspot identification we chose the method based on the research question and the scope of the analysis. We are currently investigating the impact of household-aggregated variables on PLHIV in an ecological model to further understand disparities in health outcomes.
Despite these limitations our findings will be instrumental in driving policies for HIV mitigation as DC aims to release its update on the EHE. These results have the capacity to drive space-based interventions, such as linkage to care, ART adherence, and viral suppression, and increase testing along with culturally appropriate public health messaging.
Supplementary Data
Supplementary materials are available at Clinical Infectious Diseases online. Consisting of data provided by the authors to benefit the reader, the posted materials are not copyedited and are the sole responsibility of the authors, so questions or comments should be addressed to the corresponding author.
Notes
Acknowledgments. We thank Dr Rupali Doshi for proofreading the manuscript. The authors thank the HIV/AIDS, Hepatitis, STD, and TB Administration (HAHSTA) and the Strategic Information Division (SID) for their support. The analysis is based on surveillance data. We are exempt from institutional review board approval for the publication. The raw data cannot be made available to the reviewers as it has identifying information reported exclusively to the DC Department of Health. The aggregated or de-identified data can be made available through a data request application submitted to the co-author Dr Adam Allston, HAHSTA, District of Columbia, Department of Health. Excerpts of the manuscript were accepted at the International AIDS Conference-AIDS, 2020.
Potential conflicts of interest. The authors: No reported conflicts of interest. All authors have submitted the ICMJE Form for Disclosure of Potential Conflicts of Interest. Conflicts that the editors consider relevant to the content of the manuscript have been disclosed.