-
PDF
- Split View
-
Views
-
Cite
Cite
Yael Goldstein-Marcusohn, Rahaf Asaad, Leen Asaad, Erez Freud, The large-scale organization of shape processing in the ventral and dorsal pathways is dissociable from attention, Cerebral Cortex, Volume 34, Issue 6, June 2024, bhae221, https://doi.org/10.1093/cercor/bhae221
- Share Icon Share
Abstract
The two visual pathways model posits that visual information is processed through two distinct cortical systems: The ventral pathway promotes visual recognition, while the dorsal pathway supports visuomotor control. Recent evidence suggests the dorsal pathway is also involved in shape processing and may contribute to object perception, but it remains unclear whether this sensitivity is independent of attentional mechanisms that were localized to overlapping cortical regions. To address this question, we conducted two fMRI experiments that utilized different parametric scrambling manipulations in which human participants viewed novel objects in different levels of scrambling and were instructed to attend to either the object or to another aspect of the image (e.g. color of the background). Univariate and multivariate analyses revealed that the large-scale organization of shape selectivity along the dorsal and ventral pathways was preserved regardless of the focus of attention. Attention did modulate shape sensitivity, but these effects were similar across the two pathways. These findings support the idea that shape processing is at least partially dissociable from attentional processes and relies on a distributed set of cortical regions across the visual pathways.
Introduction
The two visual pathways hypothesis is a predominant model of the neural processing of visual information. According to this model, visual information is processed through two distinct cortical systems—the ventral pathway (i.e. the “what” pathway) which promotes visual recognition, and the dorsal pathway (i.e. the “where/how pathway”) which promotes visually guided actions (Ungerleider and Mishkin 1982; Goodale et al. 1991). However, emerging research, both in humans and primates, suggests that the dorsal pathway is also involved in processing of object properties fundamental for perception even when visuomotor responses are not required (see Farivar 2009; Freud et al. 2016, 2020; Xu 2018).
Recent neuroimaging studies have demonstrated that shape representations in the dorsal pathway appear to be robust to variations in local object features, thereby supporting the processing of global shape. The representations are at least somewhat invariant to modulations in size and orientation (Ayzenberg and Behrmann, 2022a; Konen and Kastner, 2008; Vaziri-Pashkam and Xu 2019). Specifically, the posterior parietal cortex (PPC), a key subsection of the dorsal pathway, computes object properties relevant for recognition. For example, the PPC exhibits robust sensitivity to shape information (Georgieva et al. 2008; Bracci and Op De Beeck 2016; Freud et al. 2017a; Ayzenberg and Behrmann 2022b), akin to the ventral object regions such as the lateral occipital complex (Grill-Spector et al. 2001; Kourtzi and Kanwisher 2001). Moreover, these representations seem to be relatively abstract, as the multivariate responses in the PPC correspond to perceived semantic similarity among objects, even after controlling for low-level visual properties (Bracci and Op De Beeck 2016; Jeong and Xu 2016).
One technique that has provided valuable insights into the neural mechanisms of shape processing along the two pathways is parametric scrambling. In this technique, shape information is systematically removed by progressively distorting object images. In the past this method was used to explore shape processing along the posterior–anterior axis of the ventral pathway (Lerner et al. 2001). More recently, this approach was utilized to explore shape processing mechanisms along the two pathways. Freud et al. (2017a) found an increase in shape sensitivity from early visual cortex to extrastriate cortex in both the dorsal and ventral pathways that decreased in anterior regions of these pathways. This large-scale organization of shape processing along both ventral and dorsal pathways was also exhibited in children (Freud et al. 2019). Lastly, in a patient with visual agnosia, following a circumscribed right hemisphere ventral lesion, a reduction of shape sensitivity slopes were found along the right ventral pathway, but also in the contralesional left ventral pathway, and in posterior parts of the dorsal pathway bilaterally (Freud and Behrmann 2020).
However, previous studies have indicated that the parietal cortex is also engaged in attentional processes (e.g. Colby and Goldberg 1999; Behrmann et al. 2004; Husain and Nachev 2007), and an increasing body of evidence suggests that attentional demands can alter activation of regions dedicated to the processing of visual information. For example, prior studies have demonstrated the influence of task requirements on representations derived by the dorsal pathway (Bracci et al. 2017; Jeong and Xu 2016; T. Liu et al. 2011; Shomstein and Gottlieb 2016). Vaziri-Pashkam and Xu (2017) conducted a functional magnetic resonance imaging (fMRI) study to investigate how goal-directed visual processing affects category representations. In three experiments participants viewed colored images from different categories and were requested to detect repetitions of either the same object or the same color presented consecutively. The study found that attention to object repetition significantly enhanced classification accuracy of object category, with a greater effect in regions along the dorsal pathway. Taking into consideration these findings, it is unclear whether the sensitivity of the dorsal pathway to shape information can be attributed to perceptual processes or alternatively might be mainly explained by attentional, task-related, processes (Xu 2018, 2023).
Accordingly, the objective of the current study was to determine if shape sensitivity in the dorsal pathway can be dissociated from attentional processes. To this aim, we conducted two complementary fMRI experiments using parametric scrambling techniques. Across both experiments, task requirements were manipulated to direct participants’ attention towards the shape in one experimental condition, and away from the shape in another condition (similar to Vaziri-Pashkam and Xu 2017).
In the Experiment 1, we used box-scrambling to eliminate shape information. This approach has been successfully used in the last few decades to investigate neural mechanisms of shape processing (e.g. Grill-Spector et al. 1998; Lerner et al. 2001). Notwithstanding, the box-scrambling technique has some disadvantages. Specifically, in addition to eliminating shape information it modifies low-level visual properties; it increases edge numbers, availability of high-spatial frequencies and hinders figure-ground information (Grill-Spector et al. 1998; Lerner et al. 2001). Additionally, it disrupts crucial Gestalt attributes such as good continuation and figure-ground distinction, resulting in the removal of stimulus identity information (Read et al. 1997; Qiu and von der Heydt 2005). Therefore, to validate our findings, we have conducted a complementary second experiment, in which diffeomorphic scrambling was employed. In this approach, shape information is progressively distorted while retaining some low-level properties (Stojanoski and Cusack 2014). Furthermore, while in Experiment 1, the box scrambling manipulation enforced us to slightly modify Vaziri-Pashkam and Xu (2017) task, the usage of the diffeomorphic manipulation allowed us to directly replicate the task used by this previous study.
In both studies, the primary hypothesis posited that, although attentional demands might influence shape sensitivity in various brain regions, the overall organization of shape processing along the ventral and dorsal pathways would remain consistent across tasks. We used a combination of univariate and multivariate analyses to map the topographical organization and functional properties of shape processing mechanisms along the two visual pathways.
Experiment 1—Box scrambling
Materials and methods
Data sharing
All raw data, protocols, and stimuli are available on https://osf.io/cbvjs/.
Participants
A total of 12 participants (eight females; mean age = 27, SD = 2.8). All participants had normal or corrected-to-normal vision and were financially compensated for their participation. Informed consent was obtained prior to the experiment. All experimental procedures were approved by the York University Human Participants Review Committee. The number of participants was predefined based on previous studies that utilized a similar approach (Freud et al. 2017a; Vaziri-Pashkam and Xu 2019).
Stimuli
The stimuli consisted of 40 images of 3D novel shapes sourced from Freud et al. (2015). Each image featured a colored stimulus in one color and a contrasting color for the background. The colors for the stimuli were red, green, blue, cyan, magenta, and yellow. The images were then divided into 16, 64, and 256 squares that were randomly rearranged, resulting in four levels of scrambling (Fig. 1B). Each version of each stimulus was presented twice in the experiment.
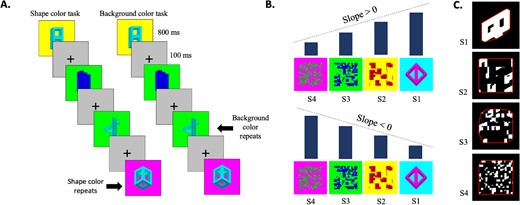
Experimental stimuli and paradigm of the box-scrambling experiment. A) The experimental paradigm. Participants performed a one-back repetition detection task on either the background color or the shape color. B) Examples for the different levels of scrambling and the manner in which slope was calculated. A positive slope of a particular voxel suggests that activation increases as scrambling decreases, indicating a greater sensitivity to shape. Conversely, a negative slope suggests that activation decreases as scrambling decreases. C) Image analysis was conducted with respect to the scrambling level. For each image, we computed the minimum distance between the shape edges and the image’s convex hull (represented by the frame), which was then normalized relative to the original, unaltered image. The analysis exhibits that the manipulation led to a reduction in shape information.
To evaluate the “goodness of shape” characteristics of the stimuli, we employed two algorithms. Initially, we calculated image entropy, which serves as an indicator of the overall irregularity within the image, where higher values correspond to greater entropy. Analysis of the entropy revealed significant difference between levels of scrambling, F(3,498) = 1774.705, P < 0.001, η2p = 0.914, with entropy gradually increasing with each level. For the second method, we delineated the edges of each image by identifying all pixels adjacent to a background pixel. Subsequently, we computed the convex hull, representing the smallest convex set that contains a given image. We then proceeded to calculate the distance from each edge pixel to the nearest convex hull pixel. The aggregate of these distances for each image was normalized by dividing it by the sum of distances for the original, unaltered version of the image. Consequently, high values in the normalized distance signify heightened shape complexity relative to the intact image (Fig. 1C). Analysis of the normalized distance has also shown significant difference between levels of scrambling, F(3,498) = 490.204, P < 0.001, η2p = 0.747, with normalized distance increasing with each level. Therefore, both measures reveal progressive increase in irregularity and complexity with higher levels of scrambling.
Procedure
Functional magnetic resonance imaging
Stimuli were presented in a pseudorandomized order using the PsychoPy (Peirce et al. 2019) and projected via PROPixx projector located at the back of the scanner bore, behind the subject’s head. Stimuli were presented within a square frame (visual angle of 4.5° X 4.5°) on a gray background. Participants viewed the stimuli through a tilted double-mirror setup mounted above their eyes on the head coil. Prior to scanning, they all completed a short training session with the experimental tasks and stimuli.
Magnetic resonance imaging setup
Participants were scanned in a Siemens MAGNESTOM Prisma fit 3-Tesla human MRI scanner with a 64-channels coil at York University. A structural scan was acquired using a T1-weighted protocol that included 192 sagittal slices (1 mm thickness, in-plane resolution = 1 mm, matrix = 256 256, repetition time = 2300 ms, echo time = 0.03 ms, inversion time = 900 ms, flip angle = 9°). Functional images based on the blood oxygenation level-dependent (BOLD) signal were acquired with a gradient-echo, echoplanar imaging sequence (TR = 1.5 s, TE = 30 ms, flip angle 78°). To achieve full coverage of both visual pathways, 76 axial slices (slice thickness = 2.20 mm, gap = 0 mm, in-plane resolution = 2.2 mm) were acquired in 12 runs of 200 volumes each (such that each run lasted 5 min).
Experiment procedure
The experimental procedure consisted of twelve runs, where participants viewed alternating images on the screen. These images were blocked by four levels of scrambling, namely S3, S2, S1, and Full. Each run began with an initial fixation period of 12 s. Subsequently, 10 blocks, comprising of 10 stimuli each, were presented on the screen. Each stimulus in each block was displayed for 800 ms, followed by a 100 ms fixation period (Fig. 1A). The blocks were separated by 9 s fixation periods. In half of the twelve runs, participants were instructed to focus on the color of the shape and press a button when two consecutive colors appeared. Conversely, in the remaining six runs, participants were given the same instruction, but for the background color. Importantly, the visual stream of information was identical across the two attentional tasks. The order of the runs was counterbalanced between participants.
Data analysis
Behavioral responses
Participants were equipped with a response box and behavioral responses were collected throughout the scan. In each run, we included 13 relevant repetitions (1–2 per block). Accuracy and response time data served as the dependent variables.
Functional magnetic resonance imaging analysis
MRI brain images were converted to NIFTI format using MRIcon, and then analyzed using FSL (Smith et al. 2004). Statistical analysis was performed using MATLAB (The MathWorks Inc. 2022), and R Development Core Team (2022). Preprocessing included 3D-motion correction, filtering of low temporal frequencies (cutoff frequency of 2 cycles per run), and 5 mm spatial smoothing. All scans were transformed to Montreal Neurological Institute (MNI) space (Fonov et al. 2011). Three primary analytical methods were utilized in the study. 1) A Regions of Interest (ROI) analysis, 2) a voxel-wise correlation analysis, 3) a multivariate representational similarity analysis (RSA; Kriegeskorte et al. 2008).
Regions of interest analysis
Regions of interests (ROIs) were defined using a probabilistic atlas (Wang et al. 2015) encompassing regions along the ventral and dorsal visual pathways. The maximum probability maps (volume space), which identify the most likely region for any given point, were employed. Note that ROIs such as IPS5, SPL, and TO2 consisted of only a few voxels and were consequently excluded from our analysis. Additionally, since the FEF is located in the frontal lobe and is not part either of the pathways, we did not analyze data from this region.
For each ROI, beta weights were computed for each of the four experimental conditions. To evaluate shape sensitivity, the slope of activation (beta values) was determined as a function of scrambling level for each voxel. A positive slope indicated increased activation with increasing scrambling (from S3 to intact), indicating higher shape sensitivity. Conversely, a negative slope indicated decreased activation with decreasing scrambling, potentially indicating enhanced sensitivity to local elements and edges, which are more prevalent in distorted images (Fig. 1B).
Voxel-wise analysis
A map of visually selective voxels along the two pathways was obtained from a previous study (Freud et al. 2017a). All voxels superior to the calcarine sulcus were considered part of the dorsal pathway, and all the voxels inferior to the calcarine sulcus were considered part of the ventral pathway.
Correlation analysis
To estimate the consistency of the spatial organization of shape selectivity across the different conditions, we conducted a correlation analysis. The dataset was divided into odd and even runs. We then extracted beta weights for the four scrambling levels and computed a linear slope across these weights to serve as an index of shape sensitivity for each voxel. Correlating these sensitivity indices between runs allowed us to determine the degree to which voxel-wise shape selectivity in one attentional condition could predict shape selectivity in another attentional condition and to compare it to within-condition consistency. Higher correlation coefficients would thus denote more stable spatial distribution of shape selectivity across different attentional conditions. Statistical analyses were carried out on Fisher transformed correlation coefficients to permit the use of parametric tests. The described correlations were then back-transformed to raw scores to facilitate interpretation in the presented results.
Representational similarity analysis
While the first two analyses focused on the level of activation (univariate analysis) they do not allow for a detailed examination of activation patterns within each ROI. In order to gain a more comprehensive understanding of the representational space within the different ROIs, we conducted a representation similarly analysis (RSA; Kriegeskorte et al. 2008).
To accomplish this, we extracted beta weights from all the voxels of the various ROIs separately for each run of each task and for each level of scrambling. Similar to Vaziri-Pashkam and Xu (2017), beta values were z-normalized across all the voxels for each condition in each ROI. This approach removes any response amplitude differences across scrambling conditions. We then created a correlation matrix for each task separately, correlating the beta weights from odd runs with even runs of each level of scrambling, resulting in a 4 by 4 matrix (4 levels of shape scrambling -odd runs X 4 levels of shape scrambling—even runs).
Subsequently, we constructed a shape model correlation matrix that posits that images with similar levels of shape information would exhibit greater similarity in their representations (Fig. 4A). We then computed Spearman correlations between this model and the correlation matrices obtained for each ROI, for each task.
In addition to the parametric shape model, we also constructed a diagonal model to serve as a more stringent control in our RSA. This model was designed to isolate the neural response to the unique level of shape coherence by assigning a value of 1 to diagonal elements of the similarity matrix, signifying maximal similarity when a condition is compared with itself. Off-diagonal elements were set to 0, indicating no similarity between different conditions. Such a design provides a conservative test of shape-specific processing, ensuring that any similarity detected is not due to low-level visual features but rather to the distinctiveness of the shape representations themselves. The diagonal model is thus crucial for determining the extent to which shape coherence information, as opposed to general visual similarities, contributes to the patterns of neural activity we observed.
Finally, to assess the maximum correlation considering data noise, we computed reliability for each Region of Interest (ROI) following the method by Bracci and Op de Beeck (2016). Each subject’s correlation matrix within each ROI was compared with the average matrix derived from all other subjects. These scores were averaged across participants to establish the reliability index, indicated by the bright gray bars for reference in Figs. 4 and 8.
Results
Experiment 1 utilized the box-scrambling manipulation alongside different task demands to evaluate the effect of attention on shape processing organization along the dorsal and ventral visual pathways.
Univariate analysis
We projected the calculated slopes (i.e. shape sensitivity index) onto the brain to visualize the topographical organization of shape sensitivity in both tasks. As illustrated in Fig. 2A, both the shape and background tasks demonstrate similar organization. The posterior occipital lobe exhibits a negative slope, indicating the early visual cortex’s responsiveness to edges and high spatial frequencies in the visual input. Moving towards more anterior regions in both the ventral and dorsal pathways, a positive slope emerges. Along the ventral pathway, this was observed in lateral areas such as LO and in more inferior areas such as the Parahippocampal gyrus and Fusiform gyrus. In the dorsal pathway, shape selectivity was evident in the extrastriate occipital cortex and the posterior intraparietal sulcus (IPS). A decline in shape sensitivity was observed in more anterior aspects of the two pathways. In the ventral pathway, this was found in the anterior and medial temporal cortex, and in the dorsal pathway, it was observed in the anterior aspects of the IPS. Notably, as mentioned above, this large-scale organization was preserved regardless of the attentional focus.
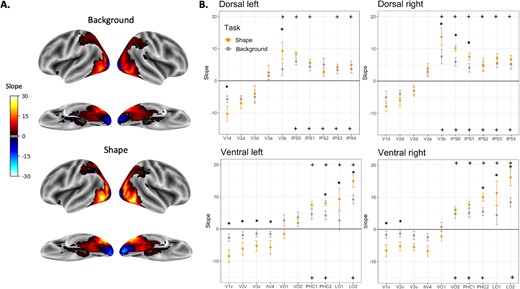
ROI analysis of the box scrambling experiment. A) The visualization displays shape sensitivity values projected onto an inflated brain from an inferior and lateral point of view for the background task (upper panel) and the shape task (lower panel). Warm colors indicate voxels that exhibit shape sensitivity, with activation intensifying as object coherence increases. Conversely, cool colors signify negative slopes i.e. a greater responsiveness to scrambled images compared to intact ones. B) Each point in the graphs shows the slope calculated for each ROI separately for each pathway and hemisphere and averaged across participants. Regions for which the slopes are significantly greater than zero are marked with a plus sign (shape task-top; background task-bottom). Significant differences between tasks are marked with asterisk. Error bars represent the standard error of the mean.
To better quantify these results we conducted an ROI analysis (defined using a probabilistic atlas; Wang et al. 2015), which validated the large-scale organization of object processing. In particular, multiple regions demonstrated sensitivity to shape (slope > 0) across pathways and tasks. Shape sensitivity was observed in the dorsal pathway for the shape-focus task in V3b, IPS0, IPS1, IPS3, and IPS4 for the LH and V3b, IPS0–4 for the RH. For the background-focus task, sensitivity was observed in IPS0–4 for both hemispheres, and also in the V3b in the RH. In the ventral pathway, shape sensitivity was observed for the shape-focus task in PHC1, PHC2, LO1, and LO2 for both hemispheres, and also VO2 for the RH. For the background-focus task, sensitivity was observed in PHC1, PHC2, and LO2 for both hemispheres and also VO2 for RH, ts > 2.3, Ps < 0.05.
Next, we examined whether task modulated shape sensitivity in the different ROIs. As depicted in Fig. 2B, several shape-selective ROIs demonstrated sensitivity to the task, ts > 2.5, Ps < 0.05, such that the slope was greater for the shape task. The effect of task (i.e. attention), was observed in shape-selective regions of the dorsal pathway (e.g. V3b, IPS0–1), but also in shape-selective regions of the ventral pathway (e.g. LO, PHC2). Importantly, the observed attentional effect did not modulate the overall pattern of shape selectivity in any of the cortical pathways. Finally, some non-selective regions in the early visual cortex also exhibited attentional modulations (see discussion for more details).
The parametric analysis described above assumes a gradual change in the level of activation as shape information is distorted. However, an alternative explanation is that shape sensitivity in the dorsal pathway reflects a categorical difference between a graspable full shape and nongraspable scrambled shapes. If this is true, we would have expected to see a notably steeper activation slope for a direct contrast between the intact shape and all scrambled shape conditions. In contrast to this prediction, the categorical contrast between shape and nonshape yielded similar results as the parametric slope described above (see Fig. 1S). The similarity in results suggests that the dorsal pathway (as well as the ventral pathway) may be sensitive to the gradations in shape coherence itself, rather than operating on a strict graspable versus non-graspable dichotomy.
Finally, we examined whether differences between tasks are due to different degrees of Temporal Signal-to-Noise Ratio (tSNR). For this aim, we calculated the mean signal intensity for ROIs that showed significant difference between tasks, per participant per task, and divided it by the standard deviation. This analysis showed no significant difference between tSNRs for any of the regions, ts < 1.7, Ps > 0.05.
Next, to further evaluate the spatial consistency of shape selectivity across the different attentional conditions, we conducted a correlation analysis between and within attentional conditions (See Methods and Fig. 3). First, across all experimental conditions, and in both the ventral and dorsal pathways, we found correlations that were robustly greater than zero (rs > 0.53, ts(11) > 6.264, P < 0.001), indicating a consistent spatial arrangement of shape sensitivity across and within attentional conditions.
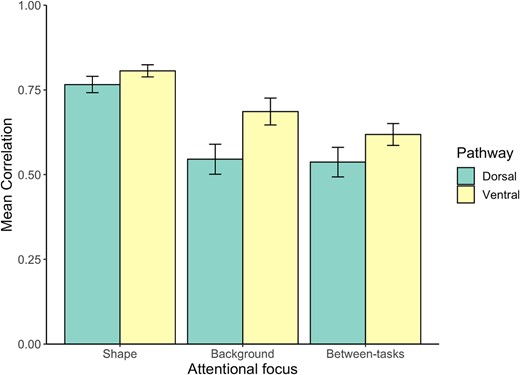
Correlation results within and between attentional conditions for the box-scrambling experiment. Error bars represent the standard error of the mean.
Nevertheless, the impact of attention was also clearly evident. An ANOVA with hemisphere (left, right), pathway (ventral, dorsal) and focus of attention (shape, background, and between-tasks) revealed a main effect of the attentional focus with greater correlation values for the “attention-to-shape” condition compared with the other conditions, F(2,22) = 75.238, P < 0.001, η2p = 0.872.
While correlation values were higher in the ventral pathway compared to the dorsal pathway, F(1,11) = 8.457, P < 0.05, η2p = 0.435, indicating a greater consistency of spatial organization of shape sensitivity irrespective of the attentional focus for ventral pathway voxels, attentional effects remained consistent across both pathways. Specifically, no interaction effect between task and pathway was discerned, F(2,22) < 1, implying that the influence of the task on the spatial distribution of shape selective voxels was similar across pathways.
Representational similarity analysis
Above, we have presented findings derived from a univariate analysis. Despite the robust nature of this approach and the correspondence between voxel-wise and ROI analyses, these assessments solely consider the amplitude of fMRI activation, neglecting activation patterns. To broaden our findings, we employed a multivariate technique known as RSA (Kriegeskorte et al. 2008). It is important to note that most prior studies utilizing RSA have aimed to uncover the representational space of individual instances. However, the RSA used in this study differs from the standard procedure. Due to our use of a block design to enhance statistical power for the voxel-by-voxel univariate mapping mentioned earlier, we could not evaluate the representation of individual instances. Instead, we applied RSA at a block level, where a single block encompasses various degrees of intact or scrambled objects. This approach still enables us to investigate the extent to which the availability of shape information modulates the representational content of the different ROIs.
The RSA examined whether the pattern of activation in the different ROIs could be explained by a shape model which posits that image representations become more alike if they share similar levels of shape information (as shown in Fig. 4A). The results of this analysis are presented in Fig. 4B. For both tasks, both early visual cortices and object-selective cortices exhibited positive correlations with the model. These correlations increased in more anterior regions of both pathways and then gradually diminished in the dorsal pathway. This pattern aligns with the findings from the univariate analysis and demonstrates a consistent pattern of sensitivity to shape information. Significant differences between tasks were found in a few shape-sensitive regions. In the dorsal pathway, this was found in IPS0, t(11) = −4.5, P < 0.001, and IPS1, t(11) = −2.4, P < 0.05, in the LH and IPS1, t(11) = −3.2, P < 0.01, and IP3, t(11) = −2.3, P < 0.05, in the RH. In the ventral pathway, a significant difference was found in PHC2, t(11) = −3.4, P < 0.01; LO1, t(11) = −2.9, P < 0.05; and LO2, t(11) = −2.5, P < 0.05, in the LH.
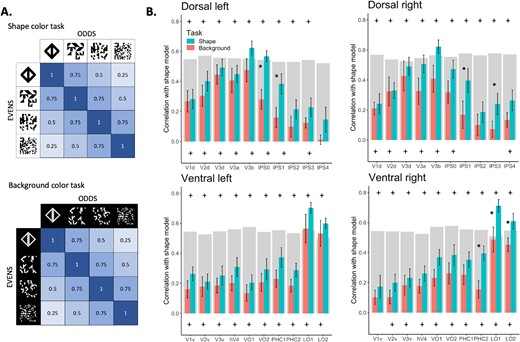
RSA analysis for the Experiment 1. A) Shape correlation model for both the shape color and background color tasks. The model assumes a perfect sensitivity to the availability of shape information. B) Correlation with model is plotted as a function of ROI, separately for each task, pathway, and hemisphere, averaged across participants. Top plus signs indicate significant correlations with the shape model for the shape task. Bottom plus signs indicate significant correlations for the background task. Asterisks indicate significant correlation difference between tasks. Error bars represent the standard error of the mean. The gray bars represent the noise ceiling for each ROI. This indicates the reliability of correlational patterns within each ROI, approximating the upper limit of correlations between fMRI and the shape model, considering the inherent noise in the data.
The results from the RSA align with the univariate findings, indicating that regions within both the dorsal and ventral pathways exhibit highly similar representational structures based on the availability of shape information, regardless of the focus of attention.
In addition to the parametric shape model, we constructed a diagonal model to further delineate the specificity of neural responses to shape information. This model is less sensitive to parametric changes in low-level visual attributes (e.g. spatial frequencies) and therefore reflects a more stringent test of sensitivity to shape information. Importantly, the two analyses yielded similar results (correlation between models for the different ROIs ranged from 0.69–0.97). While the fit of the diagonal model was somewhat reduced in shape-selective regions within both the dorsal and ventral pathways, this was anticipated and aligns with the model’s design to rigorously assess shape-specific processing. Importantly, attentional modulation effects on neural responses were consistently captured by both models. These findings suggest that the observed neural patterns reflect genuine shape processing. For a detailed visualization of these results, please refer to Supplementary Fig. 2S.
Behavioral results
Due to a technical failure, the behavioral data for three participants was unavailable. Consequently, we present the analysis results of the remaining nine participants. Accuracy rates were close to perfect (98.8%). A repeated measures ANOVA with task and scrambling level as independent variables and accuracy revealed a main effect for task, F(1, 8) = 6.403, P < 0.05, η2p = 0.132, indicating higher accuracy for the shape task (M = 99%) than the shape color task (M = 98.3%). Additionally, despite a trend, no differences between scrambling levels were observed, F(3,24) = 2.485, P = 0.085. Notably, however, given the overall high accuracy level and the lack of interaction between task and scrambling, F(3,24) < 1, these differences are not likely to account for the observed fMRI responses. A similar analysis was employed on the RT results and revealed no main effects, Task: F (1,8) < 1; Scrambling: F (3,24) =2.096 P = 0.12, nor interaction, F(3,24) < 1.
Experiment 2—diffeomorphic scrambling
Materials and methods
Participants
Twelve participants completed the experiment; however, one participant was excluded due to excessive head movements, resulting in 11 participants in total (eight females; mean age = 25.09, SD = 3.3). All participants had normal or corrected-to-normal vision and were financially compensated for their participation. Informed consent was obtained prior to the experiment. All experimental procedures were approved by the York University Human Participants Review Committee. The number of participants was predefined based on previous studies that utilized a similar approach (Freud et al. 2017a; Vaziri-Pashkam and Xu 2019).
Stimuli
Experiment 2 utilized the same set of 40 images as the first one. Each of these images was colored in six different colors: red, green, blue, cyan, magenta, and yellow. Subsequently, four variations were generated for each color, corresponding to four different levels of distortion (no distortion, one step of distortion, two steps of distortion, and four steps of distortion, with a distortion level of 80). The process involved employing a MATLAB function provided by Stojanoski and Cusack (2014), that applies a flow field generated from a set of 2D cosine components with random phase and amplitude (Fig. 5A). Every iteration of each stimulus was presented twice throughout the experiment.
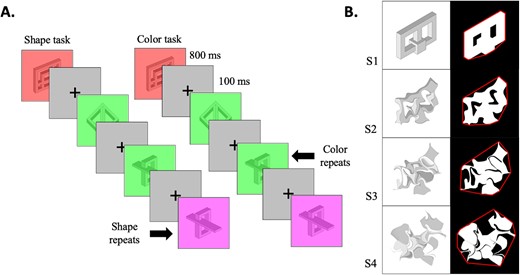
Experiment 2 stimuli and experimental paradigm. A) Participants performed a one-back repetition detection task on either color or shape. Importantly, the visual stream of information was identical across tasks. B) Analysis of the images was carried out with respect to the level of scrambling. For each image, we calculated the minimum distance between the shape edges and the image’s convex hull (depicted by the frame), which was subsequently normalized relative to the original, unaltered image. The analysis demonstrates that the manipulation resulted in a decrease in shape information.
As in Experiment 1, we used two algorithms to assess the “goodness of shape” of the stimuli—entropy and normalized distance (Fig. 5B). Both methods showed a significant increase in irregularity and complexity as shape distortion increases, Entropy: F(3,498) = 84.608, P < 0.001,η2p = 0.338; Normalized distance: F(3,498) = 516.976, P < 0.001,η2p = 0.757.
Procedure
Functional magnetic resonance imaging setup
Similar to Experiment 1.
Experimental procedure
Similar to Experiment 1. The only modification was that in half of the twelve runs, participants were instructed to focus on the shape and press a button when two consecutive shapes appeared. Conversely, in the remaining six runs, participants were instructed to focus on the color of the image (color was uniformly distributed across the shape and background; Fig. 5B) and press when two consecutive images were in the same color.
Data analysis
Similar to Experiment 1.
Results
Experiment 2 further examined how attention influences the organization of shape processing in the dorsal and ventral visual pathways. Similar to Experiment 1, we combined univariate and multivariate analyses to gain further insights into this question.
Univariate analysis
First, we conducted an ROI analysis as depicted in Fig. 6. This analysis revealed sensitivity to shape (slope > 0) in regions along the dorsal and ventral pathways, with minimal differences between tasks. Similar to Experiment 1, sensitivity to shape was observed in the posterior aspect of the dorsal pathway (V3b, IPS0–1) and in the inferior (PHC, VO) and lateral (LO) aspects of the ventral pathway, t > 2.3, P < 0.05. A significant difference between tasks was found only for two ventral regions in the RH—VO1, t(10) = −2.41, P < 0.05, and PHC2, t(10) =2.58, P < 0.05. Task did not modulate univariate activation in any of the dorsal ROIs. A subsequent analysis which was based on the contrast between shape and all nonshape conditions (similar to Experiment 1) yielded similar results as the slope analysis and is presented in the Supplementary Fig. 3S.
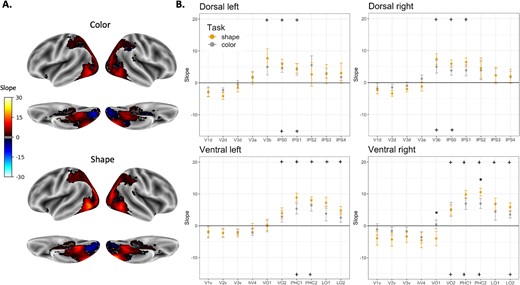
ROI analysis of the diffeomorphic scrambling experiment. A) The visualization depicts shape sensitivity projected onto an inflated brain, shown from both inferior and lateral perspectives for both the shape task (upper panel) and the color task (lower panel). Warm colors indicate voxels exhibiting shape sensitivity, with activation increasing as object coherence improves. Conversely, cold colors indicate low shape sensitivity (negative slopes) i.e. a greater responsiveness to scrambled images compared to intact ones. B) Each point in the graphs shows the slope calculated for each ROI separately for each pathway and hemisphere and averaged across participants. Regions where the slopes are significantly higher than zero are indicated with a plus sign, with the top for the shape task and the bottom for the background task. Significant difference between tasks is marked with an asterisk. Error bars represent the standard error of the mean.
Next, a correlation analysis was conducted within and between attentional conditions (Fig. 7). Similar to Experiment 1, robust positive correlations were found within and between attentional conditions, rs > 0.35, ts(10) > 3.388, P < 0.007. Yet, the values of the correlations were lower than those observed in Experiment 1. Notably, no effects of pathway, F(1,10) < 1, attentional condition, F(2,20) = 1.599, P > 0.22, or interaction between these variables were found, F(2,20) < 1. These findings further suggest that the attentional manipulation did bot modulate the spatial organization of shape selectivity in both pathways.
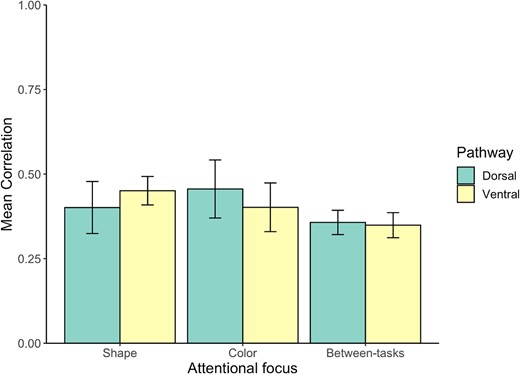
Correlation results within and between attentional conditions for the diffeomorphic scrambling experiment. Error bars represent the standard error of the mean.
Representational similarity analysis
The RSA evaluated the similarity of spatial activation patterns across different conditions in various ROIs. It then investigated whether these results could be explained by a shape model, suggesting that image representations become more similar if they share comparable levels of shape information (as illustrated in Fig. 8A). The outcomes of this analysis are displayed in Fig. 8B. In both tasks, both early visual cortices and object-selective cortices showed a positive correlation with the model. However, moving towards more anterior regions in both pathways, the correlation strengthens and then gradually diminishes in the dorsal pathway, particularly around the anterior aspects of the IPS. This pattern corresponds with the findings from the univariate analysis, demonstrating a consistent sensitivity to shape information.
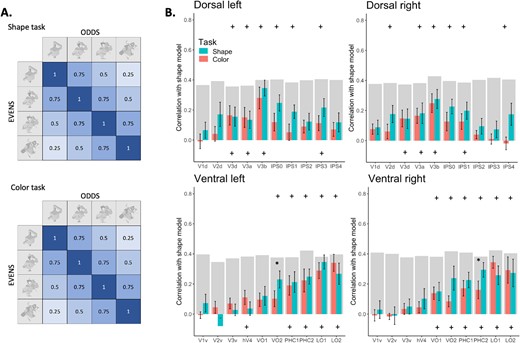
RSA analysis for the diffeomorphic experiment. A) The shape correlation model applied to both the shape and color tasks. The model posits a perfect correlation between similar levels of scrambling, gradually decreasing as the scrambling levels differ more. B) Correlation with the model is graphed as a function of ROI, defined using the Wang atlas (2015), separately for each task, pathway, and hemisphere, and averaged across participants. Plus signs at the top indicate a significant correlation for the shape task, at the bottom for the color task. Asterisks signify a significant difference in correlation between the two tasks. Error bars represent the standard error of the mean. The gray bars represent the noise ceiling for each ROI. This indicates the reliability of correlational patterns within each ROI, approximating the upper limit of correlations between fMRI and the shape model, considering the inherent noise in the data.
Importantly, most regions, in both pathways, showed comparable adherence to the shape model under both tasks. A notable difference between tasks was observed only in two regions in the ventral pathway, VO2 in the LH, t(10) = −2.6,P < 0.05, and PHC2 in the RH, t(10) = −2.5,P < 0.05.
Overall, the RSA results align with the univariate findings, indicating that regions within both the dorsal and ventral pathways exhibit highly similar representational structures based on the presence of shape information, regardless of the attentional focus. We also performed an additional RSA analysis using a diagonal model. The outcome of this analysis resembles those obtained with the shape model and are included in the Fig. 4S.
Behavioral analysis
Accuracy level was very high (98.7%). A repeated measures ANOVA was conducted, with task and scrambling level as independent variables and accuracy as the dependent variable. Importantly, no interaction between task and scrambling level was observed, F(3,30) = 1.621, P = 0.205, and no effect of task was found, F(1,10) = 1.869, P = 0.201. The effect of scrambling level was close to significance, F(3,30) = 2.786, P = 0.058, η2p = 0.042. Nevertheless, the lack of interaction with task and the high accuracy scores suggest that this behavioral effect is unlikely to account for any observed differences in terms of the fMRI results described below. An additional repeated measures ANOVA was conducted with the same independent variables and RT as the dependent variable. Once again, no main effects or interaction were found [all Fs < 1].
Discussion
The current study was designed to examine whether shape selectivity in the dorsal and ventral visual pathways is modulated by attentional mechanisms. In two fMRI experiments, we presented novel objects and gradually distorted the availability of shape information, using two complementary scrambling methods, while participants completed tasks that differed in their focus of attention (to the shape or away from the shape).
In Experiment 1, we used box scrambling to map shape sensitivity along the two visual pathways and found that sensitivity to coherent shape information increased from the posterior to anterior regions and then decreased in the most anterior aspects of the two pathways. This pattern was consistent regardless of the task, suggesting a fundamental organizational principle in the visual cortex.
An additional voxel-wise correlation analysis further examined the spatial consistency of shape selectivity responses across and within conditions. Importantly, we found high correlation values within and between tasks further supporting the consistency of the large-scale sensitivity to shape information. Notably, this analysis also demonstrates the adaptability of the visual system. When attention was focused on shape, there was a stronger correlation between runs compared to runs in which the attention was focused away from the objects. This finding shows the system’s ability to prioritize relevant features through top-down modulation. Second, we also observed higher correlations in the ventral pathway which emphasize its role in object recognition, while notable correlations in the dorsal pathway also highlight consistent shape sensitivity. Finally, the lack of interaction between task and pathway suggests a unified capacity across the visual system to adjust processing based on attentional demands.
Multivariate RSA supported these findings, indicating that the overall pattern of shape sensitivity did not significantly differ with the focus of attention, although some task-dependent differences were found.
In Experiment 2, we used the diffeomorphic scrambling manipulation to distort shape information. The univariate and multivariate results were consistent with the findings observed in Experiment 1. Critically, our analyses revealed only limited effects of task (attention to object or away from the object) on specific regions on both pathways, while the large-scale organization of shape sensitivity remained consistent across task manipulations.
Shape sensitivity in the ventral and dorsal pathways
Recent advancements in visual neuroscience have begun to view the division between the two visual pathways as more of a spectrum rather than a strict dichotomy (Freud et al. 2016, 2020; Erlikhman et al. 2018). An increasing body of research, encompassing neuropsychological (Freud et al. 2017b; Medina et al. 2020), neuroimaging (Bracci and Op De Beeck 2016; Freud et al. 2017a; Garcea et al. 2018), psychophysical (Almeida et al. 2010; Freud et al. 2018), and animal studies (Theys et al. 2015; Dromme et al. 2016), has highlighted that the dorsal pathway, traditionally associated with visuomotor control, also facilitates perceptual behaviors, particularly those related to spatial processing of shapes.
Early indications of the dorsal pathway’s role in shape processing were observed in nonhuman primates. Neurons in the lateral intraparietal area (LIP) of the PPC showed sensitivity to simple 2D shapes, a phenomenon not solely attributable to eye movements or other visuomotor factors (Sereno and Maunsell 1998). This finding was further supported by human fMRI studies, which detected a shape-selective response in the PPC (Konen and Kastner 2008; Freud et al. 2015; Bracci and Op De Beeck 2016). These responses, akin to those in the ventral pathway, were invariant to transformations such as size, retinal position, and viewpoint, and correlated with perceptual behaviors (Konen and Kastner 2008; Freud et al. 2017a). Finally, both dorsal and ventral pathways, including regions like LO1, LO2, IPS0, IPS1, and IPS2, exhibit a robust retinotopic organization, which might serve as a basis for visual cognition (Groen et al. 2022).
In contrast to our previous studies (Freud et al. 2017a), an additional finding from the current study is the observation of shape sensitivity in the dorsal pathway for novel objects, which notably lack semantic information. This suggests that similar to the ventral pathway, the dorsal pathway’s involvement in shape processing extends beyond familiar and semantically loaded objects, indicating a more generalized role in computations related to the processing of shape information.
Notwithstanding these similarities, distinct differences exist between shape representations derived by the two pathways. Techniques with high temporal resolution, like event-related potentials, magnetoencephalography, and single-unit recordings, have shown that shape-selective signals in the dorsal pathway precede those in the ventral regions, suggesting that object representations in the dorsal pathway are not simply cascaded from computations carried out in ventral regions (Ayzenberg et al. 2023; Collins et al. 2019; Lehky and Sereno 2007; L. Liu et al. 2017; Regev et al. 2018). This suggests that dorsal shape sensitivity is not merely a result of signal cascading from the ventral pathway, and may involve different inputs, such as the faster magnocellular input for the dorsal and the parvocellular input for the ventral (Freud et al. 2018; Collins et al. 2019).
The different properties of the representations derived by the two pathways led to a series of studies (Bracci et al. 2017; Vaziri-Pashkam and Xu 2017, 2019) that show that these representations also differ in their sensitivity to attentional modulations. In particular, representations derived by the dorsal pathway showed greater susceptibility to task demands in comparison to the representations derived by the ventral pathway. The results of the current experiments suggest that despite the apparent susceptibility of category representations in the dorsal pathway to task demands, more basic shape processing mechanisms are only minimally affected by the same attentional modulations. This differential impact underscores the resilience of basic shape processing mechanisms in both pathways in the face of varying attentional states.
Effects of task demands on shape processing in the dorsal pathway
Attention and vision are intricately connected. While some visual processes such as grouping and figure-ground segmentation can occur without directed attention (Russell and Driver 2005; Kimchi and Peterson 2008), others demand attentional resources. These attention-dependent processes are integral to both spatial and nonspatial aspects of visual processing in the dorsal pathway, influencing how visual information is processed and represented in the parietal cortex. Ibos and Freedman (2016) highlight how spatial and feature attention independently influence activity in the LIP, suggesting a high degree of attentional gating in this region. Such gating allows the PPC to modulate the representation of task-relevant spatial and nonspatial features, aligning with behavioral contexts of visual input. These findings align with Xu's (2018, 2023) proposal according to which visual information is uploaded from the ventral pathway to support adaptive, goal-directed, visual processing to support different functions such as working memory and visuomotor.
Nevertheless, our empirical evidence supports other findings that suggest that, although the dorsal pathway is sensitive to attentional modulation, it may also support representations that operate somewhat independently of such mechanisms. For instance, Ayzenberg and Behrmann's (2022b) finding that pIPS and aIPS prioritize the processing of the global shape of an object (i.e. part relations) could suggest that certain aspects of shape processing are less reliant on attention, reflecting intrinsic properties of object representation in the dorsal pathway. This nuanced perspective contributes to a more comprehensive understanding of the interaction between attention and shape processing within the dorsal pathway.
Thus, while attention plays a significant role in guiding visual processing within the dorsal pathway, it does not solely account for the observed shape perception capabilities, suggesting a more complex and integrated role for the dorsal pathway in visual perception.
The effect of scrambling methods on shape sensitivity
In the current study we employed two scrambling methods—box-scrambling (Experiment 1) and diffeomorphic scrambling (Experiment 2). Box-scrambling breaks the object into pieces efficiently eliminating shape information. Notwithstanding, this approach also changes low-level visual properties (e.g. increasing the availability of high spatial frequencies in the image). Diffeomorphic scrambling, on the other hand, distorts shape information gradually while retaining some low-level properties. However, even in the most distorted version of this method, a 2D, nonrecognizable, shape is still available. Hence, those two approaches are complementary and allow us to explore shape processing mechanisms in a more comprehensive fashion.
Importantly, the utilization of the two scrambling methods compelled us to use slightly different tasks. Experiment 2 directly replicated Vaziri-Pashkam and Xu (2017) task—participants were required to attend to either the shape or the color. Notably, even in the “color” condition, participants could still focus on the object as it was colored as well. Nevertheless, this task is still effective in manipulating the attention of subjects as demonstrated by Vaziri-Pashkam and Xu (2017).
In Experiment 1 (box scrambling), this task was not used as the difficulty of the shape task was confounded with the level of scrambling (i.e. harder 1-back task for more scrambled objects). Thus, we used a variation of the task, such that participants actively shifted their attention from the color of the objects to the color of the background. This task might require greater attentional resources relative to Experiment 2.
Consequently, in Experiment 1, when the shift of focus was more pronounced, we found a greater discrepancy between tasks, in comparison to Experiment 2 where the shift of focus was subtle. This was evident across the univariate ROI analysis and the multivariate RSA. Most importantly, despite the observed effects of task demands on shape sensitivity, the large-scale topographical organization of shape processing in the dorsal and ventral pathways remained consistent across tasks and scrambling methods.
Finally, at least in Experiment 1 there were evidence for an effect of task on early visual cortex. In particular, the activation profile of these posterior regions is usually characterized by a negative slope (i.e. greater activation for scrambled objects). However, when attention was directed to the color of the background the negative slope was not evident. This finding is consistent with previous studies which demonstrated that spatial attention leads to early modulations in the extrastriate visual cortex, altering the amplitude of evoked neural activity (Mangun 1995). Furthermore, contextual cues and shifts in visual attention can modulate activity in early visual areas through late top-down and alpha-band activity modulations, respectively, indicating a complex interplay of attentional processes at various stages of visual processing (Olson et al. 2001; Rihs et al. 2009). Notably, in the current study, the coarse temporal resolution of fMRI does not allow us to determine the time course of the attentional modulation observed in early visual cortex.
Limitations
While this study contributes to our understanding of the effects of attentional modulation on shape processing within the ventral and dorsal pathways, there are several considerations that qualify our findings. The relatively simple nature of the tasks in our experiments, reflected in the high accuracy levels achieved by participants, may not have fully deplete the participants’ attentional resources. This raises the possibility, as suggested by Lavie’s Load Theory, that additional cognitive capacity remained available, allowing for the processing of shapes even when attention was ostensibly directed towards another aspect of the task (Lavie 2005). According to this view, the subtle attentional shift might allow the unused processing resources to be deployed for shape processing unintentionally, which might explain the similar large-scale organization across tasks.
Nevertheless, in similar paradigms, subtle manipulations have led to notable differences in MVPA classification accuracies (Vaziri-Pashkam and Xu 2017), suggesting that even minimal shifts in attention can be detected with sensitive analytical methods. The engagement of vigilant attention-related brain regions during task maintenance, as well as their responsiveness during reorientation to new targets (Langner and Eickhoff 2013), underscores the intricacies of sustained and transient attention processes. As such, the duration of our experiment could have incrementally contributed to the attentional load through the sustained focus required.
Future research should consider employing more demanding tasks that adequately engage attentional capacity to explore the role of attention in shape processing. Such studies could provide deeper insights into the interplay between attentional load and visual processing within the visual pathways, potentially revealing differential effects across varying levels of task complexity.
Finally, the current study employed a block design to increase the statistical power of the RSA and ensure comparability with prior work. While this approach has proven robust for the questions at hand, it precludes the investigation of sensitivity to individual shape representations that could be afforded by an event-related design. Recognizing this as a limitation of the present study, we suggest that future research could build upon our findings by incorporating event-related designs to advance our understanding of the nuanced aspects of shape processing. Such studies would complement the present work by disentangling the neural responses to individual shapes from the broader category-level processing that our block design primarily captures.
Conclusions
In conclusion, our findings reveal a consistent large-scale organization for shape processing, irrespective of the focus of attention. Notably, the dorsal pathway demonstrated sensitivity to object shape in both tasks, underscoring its role in shape processing. While attention had the capacity to modulate shape sensitivity, it did not fundamentally alter the large-scale topographical organization of the visual system. These results provide valuable insights into the interplay between attentional mechanisms and shape processing.
Acknowledgements
The authors thank Dr Diana Gorbet, Dr Jeremy Wolfe and Dr Marlene Behrmann for fruitful discussions that contributed to the development of this work.
Author contributions
Yael Goldstein-Marcusohn (Writing—original draft, Formal Analysis, Data curation, Methodology, Investigation), Rahaf Asaad (Investigation), Leen Asaad (Investigation), and Erez Freud (Conceptualization, Resources, Supervision, Writing—original draft, Formal Analysis, Writing—review & editing).
Funding
This work was supported by the Natural Sciences and Engineering Research Council of Canada (NSERC) (EF), by the Vision Science to Applications (VISTA) program funded by the Canada First Research Excellence Fund (CFREF, 2016–2023) (YGM, EF).
Conflict of interest statement: None declared.