-
PDF
- Split View
-
Views
-
Cite
Cite
Sára Jellinek, József Fiser, Neural correlates tracking different aspects of the emerging representation of novel visual categories, Cerebral Cortex, Volume 34, Issue 2, February 2024, bhad544, https://doi.org/10.1093/cercor/bhad544
- Share Icon Share
Abstract
Current studies investigating electroencephalogram correlates associated with categorization of sensory stimuli (P300 event-related potential, alpha event-related desynchronization, theta event-related synchronization) typically use an oddball paradigm with few, familiar, highly distinct stimuli providing limited insight about the aspects of categorization (e.g. difficulty, membership, uncertainty) that the correlates are linked to. Using a more complex task, we investigated whether such more specific links could be established between correlates and learning and how these links change during the emergence of new categories. In our study, participants learned to categorize novel stimuli varying continuously on multiple integral feature dimensions, while electroencephalogram was recorded from the beginning of the learning process. While there was no significant P300 event-related potential modulation, both alpha event-related desynchronization and theta event-related synchronization followed a characteristic trajectory in proportion with the gradual acquisition of the two categories. Moreover, the two correlates were modulated by different aspects of categorization, alpha event-related desynchronization by the difficulty of the task, whereas the magnitude of theta -related synchronization by the identity and possibly the strength of category membership. Thus, neural signals commonly related to categorization are appropriate for tracking both the dynamic emergence of internal representation of categories, and different meaningful aspects of the categorization process.
Introduction
Being fundamental building blocks of our cognition, mental categories have been the subject of a wide range of behavioral and neural investigations. In behavioral categorization research, a large number of studies addressed the link between the emergence and quality of categorization and various aspects of the task and stimuli. These investigations focused on stimulus complexity, distribution of stimuli (Ashby and Maddox 2011; Ashby and Valentin 2017; Shepard 1991; Nosofsky and Palmeri 1996; Maddox and Dodd 2003), category membership (Arias-Trejo and Plunkett 2010) and the relationship between stimulus and task (Ell and Ashby 2006). In contrast, much less is known about how these aspects map onto neural responses of the brain, in particular to electroencephalogram (EEG) signals, or whether and how the emergence of those responses follow the ongoing cognitive acquisition of novel categories.
One possible scenario is that the typical neural correlates associated with the process of categorization reflect the same general aspects of the categorization process. Such general aspects could include familiarity with the stimulus, difficulty, or membership of the categorization or uncertainty during the trial. The candidate neural correlates we focus on in the present study are the P300 event-related potential (ERP; Kok 2001; Polich 2007; Harper et al. 2017) and the event-related modulations in the alpha (8–12 Hz; Klimesch et al. 1998; Klimesch et al. 2007) and theta bands (3–8 Hz; Yordanova and Kolev 1998b; Harper et al. 2017). Indeed, there are several response characteristics shared by P300 ERP, upper alpha event-related desynchronization (ERD) and late theta event-related synchronization (ERS) potentially supporting the above scenario. They are all involved in categorization or memory updating, they are independent of the modality of the target stimuli, they reflect task-related high cognitive activation and attention, and they are all sensitive to stimulus frequency reflected by more articulated responses for rare, surprising stimuli (Sochurková et al. 2006; Peng et al. 2015). These common features also allow to study these correlates in a common oddball paradigm for investigating their relation to categorization (Squires et al. 1975).
An alternative hypothesis about the role of various neural correlates of categorization is that they are much more specific and reflect different aspects of the categorization task (e.g. P300 ERP reflecting primarily categorization difficulty while α ERD reflecting category membership during the trial). However, existing oddball studies investigating the role of these neural correlates allow for only limited understanding about the link of these correlates to aspects of the categorization forming process. This is because such studies typically use a measurement at a single time point and discrete, overtrained stimuli—usually only a single stimulus per infrequent category—that are already familiar to the subjects (Sutoh et al. 2000; Yordanova et al. 2001; Sochurková et al. 2006; Peng et al. 2015). As a result of such familiarity and the lack of complexity and gradedness of the stimuli, findings of current studies cannot inform us about the link between the neural correlates and more nuanced aspects of categorization, and neither can they capture the changes occurring in these neural correlates during the emergence of a new internal category.
The goal of the present study was to eliminate these two shortcomings of the classical framework in order to answer two questions. First, whether different neural signals commonly associated with categorization respond selectively and meaningfully to different aspects of the categorization process. Second, whether the appearance of such neural responses require well-known previously consolidated categories, or alternatively, they would gradually emerge tracking the progress of the acquisition of even newly formed novel categories. To answer these two questions, we used the classical oddball paradigm with novel stimuli that continuously varied along multiple integral stimulus dimensions and recorded EEG signals from the very beginning of the category learning process, that is from the naive state of the observer. Using these more complex graded stimuli and recording neural data throughout the process of acquisition, we found that, indeed, different neural measures correlated with different aspects of the categorization task, and the correlating features of the neural signals emerged gradually as the categories were formed.
Materials and methods
Participants
Thirty-one right-handed participants [18 females, mean age = 27 years, age range between 21 and 36 years] completed the experiment. All of the participants had at least ongoing or finished undergraduate studies. Data from six participants were excluded from the analysis due to excessive EEG artifacts based on our criterion that was defined prior to the analysis. According to the criterion, the number of artifacts were considered excessive when in any group of trials where data was subjected to averaging for further analysis more than 50% of trials had to be discarded due to noise present in the analyzed time window. Applying this criterion to each participant’s data resulted in at least 30 data points in each stimulus group (Freq1, Freq2, Freq2 and Infreq) per block and the average of those points provided the basis for our statistical analysis.
Power analysis with the number of participants left for analysis yielded a power of 0.67 with parameters α = 0.05 and effect size of 0.5. The robustness of the obtained data and the adequacy of the derived conclusions was corroborated by computing Bayes factors for each statistical analysis.
All participants gave their informed consent, and the experimental protocols were approved by the Ethics Committee for Hungarian Psychological Research.
Stimuli
We used the parametrically tunable abstract stimuli created by Op de Beeck et al. (2001). The appearance of these stimuli varied gradually along a number of visual feature dimensions, such as aspect ratio, number of articulated parts, or pointiness of those parts as a function of six latent generative parameters. These six parameters jointly determined the changes in all visual feature dimensions without any clear link between a given latent parameter and a particular salient visual feature. Thus, these stimuli changed along various continuous integral dimensions rather than along discrete and/or separable dimensions (Nosofsky and Palmeri 1996). Given the many options for possible encodings of these unfamiliar abstract shapes, participants could develop very different internal representations of the stimulus set with high diversity in their subjective similarity metric across the stimuli.
By continuously varying the underlying latent parameters, we generated a set of morphed shapes varying smoothly along multiple integral feature dimensions and mapped them onto an arbitrary two-dimensional visual picture space. From a subregion of this space depicted by a matrix of 49 visually distinct morphs in Fig. 1(a), we sampled 12 perceptually discriminable but relatively similar complex stimuli, which initially did not offer any obvious categorical information. Each of these stimuli were assigned to one of four stimulus groups (Freq1, Freq2, Freq3, and Infreq) with elements of the Freq groups bearing increasingly higher similarity to those in the Infreq group starting from a very different appearance (Freq1) and progressing to relatively similar shape forms (Freq3).
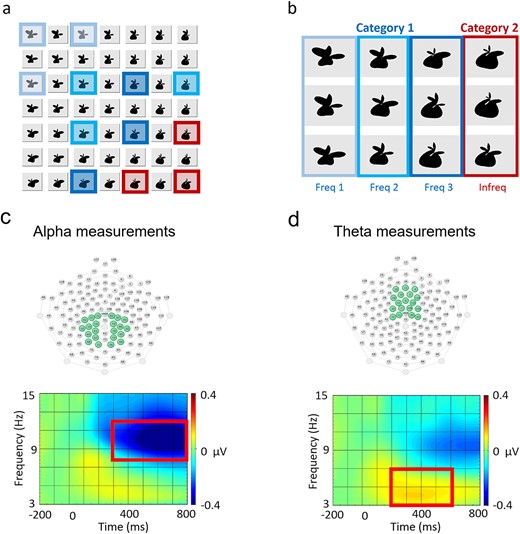
Design and analysis of the experiment. (a) A region of the stimulus space defined by the latent parameters mapped continuously onto an arbitrary two-dimensional plane. The sampled stimuli used in the study are indicated with border and background coloring where the color and brightness indicate one of the used arrangements of subcategories within the design. (b) Stimuli comprising the frequent (upper left region in blue) and the infrequent (lower right corner in red) categories. Stimulus groups in the frequent category were defined in a pilot categorization behavioral test using worse discrimination performance as an indicator of higher similarity to the other, infrequent category. (c–d) ROIs and time window with frequency band defined for alpha (c) and theta response analysis (d). The ROI of P300 ERP measurements were the same as for alpha responses (c).
The ground truth of general similarity between stimuli perceived by observers had been established in a preceding pilot study conducted before the main experiment with a different set of observers. In this pilot study, in each test trial, participants (n = 18) saw one of the same twelve stimuli later used in the main experiment and they had to make a 2-AFC decision whether the shape belonged to Category 1 or 2. Participant’s concept of the categories were established prior to the test following the method used in the main experiment (see Design and Methods). Each stimulus has been used ten times during the test and the presentation order was randomized across subjects. At the end of the session, each participant was asked to explain explicitly what features they used in their judgments, but no prominent pattern from these answers emerged. This confirmed that the dimensions defining the stimuli in the in the participants’ internal representation could indeed be considered as integral dimensions allowing for different interpretations of the stimuli. After the session, each participant’s responses to each stimuli were averaged and these averaged numbers were arranged into a one-dimensional ordering of the stimuli on the Category A vs. Category B axis. The closest three, second three, etc., shapes in this ordering from Category A defined by the ranking were assigned to the four groups (Freq1, 2, 3, and Infreq), respectively, for the given participant. These “group-belongingness” scores of each shape were averaged across all participants and then ordered again along the Category A vs. Category B axis. This ultimate averaged and ordered score was used for establishing the final assignment of each shape to a particular group.
A priori, it would seem logical to define the stimulus groups by drawing straight diagonal lines in the two-dimensional stimulus space according to the magnitude of the parameter changes (Fig. 1a). However, the pilot study revealed that while subjects by-and-large followed this metric, they systematically grouped the rightmost figure in the third row marked with lighter blue color with the other figures marked with lighter blue color. This precise assessment of the ground truth about the participants’ implicit internal representation was important for justifying our claims based on the analyses tracing the correspondence between EEG signals and particular aspects of the input.
In the main experiment, stimuli from three neighboring stimulus groups (Freq1–3) comprised Category 1 under one arbitrary label, while stimuli only from the Infreq groups belonged to Category 2 with a different label (Fig. 1b). The stimulus group comprising the less frequent category, as well as the label assignment to the two categories were independently counterbalanced across participants. As a result, the same stimuli that composed the infrequent category (Fig. 1.b: Infreq) for one participant were the most extreme elements of the frequent category, most obviously different from the infrequent category for another participant (Fig. 1.b: Freq1) so that this counterbalancing decoupled stimuli from the labeling across participants.
The uneven assignment of the number of stimulus groups to categories created the oddball setup in our experiment. As mentioned in the context of the ground truth obtained by the pilot study, the 2D mapping between the space defined by the latent parameters and participants’ subjective similarity space was not perfectly aligned as indicated by the discrepancy between label of two samples to Freq2 and Freq3 and their position on the lattice in Fig. 1(a). This demonstrates that the participants, indeed, used their own internal coding corresponding only moderately to the true generative integral dimensions during the odd-ball task.
Using stimuli that varied continuously on multiple feature dimensions instead of a few, semantically distinct and visually unrelated figures provided several advantages. First, we could investigate the effect of category structure and task difficulty or the strength of category membership on the nature of neural responses. Second, due to the unfamiliarity of the stimuli and novelty of the categories, there was not any consistent prior on the categories across participants that would impose an a priori similar bias on the category boundary and hence participants’ individual performance in a predictable manner. Third, the combination of the novelty and continuity of feature dimensions also ensured that the category learning task was difficult enough so that different phases of the acquisition process could be separately investigated, and the gradual emergence of the category representation could be traced.
Design and procedure
The experiment was created and presented with Matlab 2014a using the Psychophysics Matlab toolbox (Brainard 1997). Participants were seated in a dimly lit room in front of a computer screen and first, they heard the experimental instructions explaining that they were about to learn classifying two deep sea animal species called by made-up labels (also used in similar studies): Bitye and Tacok, or Dax and Wug for English speaking participants. After the instructions, participants were provided two-two random examples from the infrequent (Infreq) and the most extreme frequent (Freq1) categories with the corresponding labels. After this familiarization phase, participants completed the main part of the experiment, where in three blocks of trials, they were simply asked in each trial to classify the figure they saw according to the two predefined categories. Stimuli appeared at the center of the screen for 800 ms following a central fixation cross, which stayed on for a random duration between 400 and 600 ms. After the stimuli disappeared, a response screen followed with the Category labels appearing on the left and right sides of the screen, in a counterbalanced manner across trials. Participants made their category decision by pressing either the left or the right button on a gamepad. Feedback was provided after each trial for 500 ms by a green circle for a correct answer, and a red cross for an incorrect response. After choosing the category, participants also provided a confidence judgment of their response on a sliding scale from 0 to 100%.
The three experimental blocks were separated by breaks of a few minutes. In each block, the 12 stimuli were presented 20 times in random order. Crucially, the frequency difference between the categories was not created at the level of individual visual stimulus, but rather at the category level. Specifically, Category 1 was composed of the Freq1–3 stimulus groups, therefore from the beginning of the experiment, it was three times more likely to see a member of Category 1 than that of Category 2, which contained only stimuli from the Infreq stimulus group. At the same time, all individual stimuli appeared equally often; thus, the oddball nature of the design emerged only once participants reliably acquired the concept of the two categories, and this occurred despite the balanced presentation of the actual visual stimuli. This method allowed a separate investigation of the category formation process from the naive to the experienced level within individual participants.
EEG recording and analysis
High-density, continuous EEG was recorded using Hydrocel Geodesic Sensor Nets (Electrical Geodesics Inc., Eugene, OR, United States) including 128 channels equally distributed on the scalp, referenced to the vertex (Cz). Recording, pre-processing and export of the data was done with Net Station 4.5.1. The sampling rate was 500 Hz with a low-pass filter of 200 Hz.
EEG was band-pass filtered between 0.3 and 30 Hz. Continuous data were segmented into 12 groups: 4 stimulus groups (Freq1, Freq2, Freq3, and Infreq) × 3 blocks of the experiment (Block1, Block2, Block3). Segments were defined 600 ms before and 1200 ms after stimulus onset. Epochs were classified as artifacts whenever the average amplitude of a 80 ms sliding window exceeded 55 μV at horizontal EOG channels, 140 μV at vertical EOG channels, and 80 μV at any other channel. Bad channels were automatically interpolated in epochs with less than 10% of the channels containing artifacts. Epochs with more than 10% of the channels containing artifacts within a − 200 + 800 ms window around the stimulus onset were automatically rejected.
Wavelets
Retained segments were imported into Matlab using EEGLAB (v9.0.5.6b) and re-referenced to average reference. After referencing, epochs were convoluted by complex Morlet wavelets within the frequency band of 5–15 Hz with a 1-Hz resolution using a custom-made script collection, WTools. Epochs then were baseline corrected to a 200-ms interval immediately preceding stimulus onset. We defined separate ROIs for expected upper alpha ERD and theta ERS, as based on previous literature they are expected to be most prominent at parietal (Yordanova and Kolev 1998a; Yordanova et al. 2001; Klimesch et al. 2006; Peng et al. 2012) and midfrontal regions (Yordanova and Kolev 1998b; Harper et al. 2017), respectively. Absolute values of complex coefficients were computed at the ROIs within the time window of 300–800 (Parise et al. 2018) and 200–600 ms (Harper et al. 2017), and frequency range of 8–12 and 3–8 Hz for alpha ERD and theta ERS, respectively.
Event-related potentials
Epochs were baseline corrected to the 200-ms interval preceding stimulus onset. Bilateral, symmetric ROIs were defined parietally following Parise et al. (2018). The ROI of P300 ERP measurements were the same as the ones used for alpha responses (Fig. 1c). The P300 ERPs were quantified as mean signal amplitude within the time window of 300 and 500 ms after stimulus onset, based on grand average of the channels. The time window was defined by plotting the group average and selecting the time range where the P300 ERP was best articulated.
Results
Statistical analysis was conducted using JASP 0.17.3 for both frequentist and Bayesian analyses. When assumptions of frequentist statistical tests were violated, results of their robust alternatives (Wilcoxon signed-rank T-test instead of Student’s t-tests) or the original statistic with corrections (Greenhouse–Geisser correction for ANOVA) were used. In addition, Bayes factors (BF10) were calculated against the null model and reported together with their frequentist counterparts indicating the reliability of the statistical results.
Behavioral results
By the end of the experiment, all participants successfully learned the categories (average classification performance across all subgroups above 90% correct, for stimulus groups in Block3 96.6%; Fig. 2a). Participants’ learning trajectory exhibited two notable characteristics. First, there was a monotonic and significant overall improvement in categorization performance across the three experimental blocks as indicated by the significant main effect for experimental block of a repeated measures two-factor (block and stimulus group) ANOVA [F(2,288) = 114, P < 0.001, ηp2 = 0.82, BF > 100]. However, since the magnitude of improvement was not constant for all four stimulus groups across the three blocks, a significant interaction between stimulus groups and experimental blocks was also found [F(6,288) = 7.4, P < 0.001, ηp2 = 0.37, BF > 100]. Second, the relative improvement of the three subcategories within the frequent category was not uniform as indicated by a significant Stimulus group main effect [F(2,48) = 38.5, P < 0.001, ηp2 = 0.61, BF > 100; Fig. 2a]. Specifically, by the final experimental block (Block 3) the categorization performance for stimuli in stimulus group Freq3 was significantly worse with a high effect size than that in Freq1 [t(24) = 4.11, P < 0.001, Cohen’s d = 7.3, BF = 78] or Freq2 [t(24) = 3.58, P < 0.01, Cohen’s d = 2.2, BF = 24]. These significant differences also held between the Infreq group and Freq1 [t(24) = 5.37, P < 0.001, Cohen’s d = 11.7, BF = 1343], Freq2 [t(24) = 4.74, P < 0.001, Cohen’s d = 3.4, BF =326]. In contrast, there were no decisively significant differences between stimulus groups Freq1 and Freq2 [t(24) = 1.95, P = 0.06, Cohen’s d = 0.37, BF = 1.06] or between Freq3 and Infreq [t(24) = 1.91, P = 0.67, Cohen’s d = 0.47, BF = 1.01].
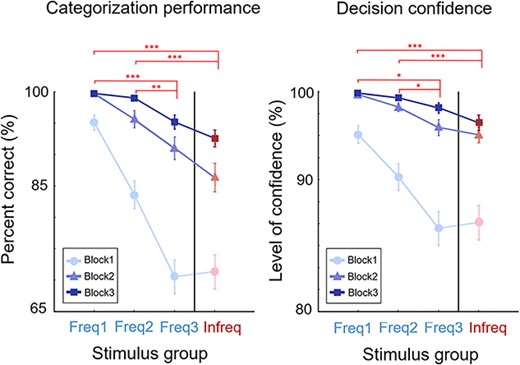
Behavioral results. Left: categorization performance changed monotonically with practice both across experimental blocks and across the Freq1, Freq2, Freq3, Infreq stimulus groups. Right: subjective decision confidence results followed closely the performance measures. Targeted pairwise comparisons indicated at the top signs refer to Block 3 results. Missing signs indicate no significant differences while *0.05, **0.01, ***0.001 level of significance. Note the different ranges for the abscissas in the two panels applied for better visibility. Error bars indicate the SEM.
Improvement in subjective confidence across the three experimental blocks and across the within-block subcategories closely mirrored the changes in behavioral performance (Fig. 2b). There were significant differences between Freq1 and Freq3 [T = 73, z = 2.66, P = 0.009, r = 0.87, BF = 4.3], between Freq1 vs. Infreq [T = 210, z = 3.9, P < 0.001, r = 1.0, BF = 50], and Freq2 vs. Infreq [T = 209, z = 3.88, P < 0.01, r = 0.99, BF = 18] as well as between Freq1 and Freq2 stimulus groups [T = 73, z = 2.66 P = 0.009, r = 0.56, BF = 5] stimulus groups by the final experimental block (Block 3) with high BF values indicating strong evidence. Meanwhile, there was insufficient evidence indicating no significant difference between Freq2 and Freq3 [T = 90, z = 1.7, P = 0.09, r = 0.28, BF = 1.03] and between Freq3 and Infreq [t(24) = 1.96, P = 0.06, Cohen’s d = 0.39, BF = 1.07] with respect to subjective decision confidence.
These results confirmed that our stimulus manipulation was effective in influencing categorization performance, and they also invoked the expected learning effects. Specifically, we found a notable dissociation: while in none of the blocks we found significant difference between performances and confidences with stimuli of the Freq3 stimulus group vs. the Infreq stimulus group, the difference in performance across the three stimulus groups Freq1, Freq2 and Freq3 always remained significant in each block. This result indicates that the behavioral measurements reflect the difficulty of the task of establishing the category membership: The farther the given stimulus is from the category boundary, the easier its categorization is, hence significant differences emerge for elements of Freq3 vs. Freq1 groups despite those elements belonging to the same category. In contrast, stimuli in the Freq3 and Infreq groups are the closest to the category boundary and thus both of them represent the most difficult categorization condition, therefore, they yield equally low performance and confidence. At the same time, the significant improvement in performance and confidence across the three blocks indicates that the participants managed to improve their knowledge about the categories from close to random performance and relatively high uncertainty to almost perfect performance and high confidence approximating a true category formation process.
Neuropsychological results
No change in P300 ERP
A repeated measures two-way ANOVA of the mean amplitude of power in the time window of 300–500 ms after stimulus onset including the four Stimulus groups and the three Blocks as factors did not reveal significant main effect of either the Stimulus groups [F(3,72) = 0.82, P = 0.48, ηp2 = 0.03, BF = 0.05] (Fig. 3), or any interaction between the factors [F(6,114) = 0.35, P = 0.9, ηp2 = 0.01, BF = 0.14]. However, we found a significant main effect of Block with almost substantial evidence [F(2,48) = 3.88, P = 0.038, ηp2 = 0.13, BF = 2.1]. Based on marginally sufficient evidence, post-hoc t-tests indicated a significant increase in P3 ERP amplitude from Block2 to Block3 [t(24) = 2.46, P = 0.021, Cohen’s d = 0.49, BF = 2.5] (Fig. 3). In addition, just prior to the time window used for assessing P300 ERP, a prominent separation in amplitude emerged between the Frequent and Infrequent categories around the 250-ms time point. However, a similar, repeated measures two-way ANOVA with Blocks and Stimulus groups as independent variables conducted on the neural responses within the time window of 230–300 ms did not result either significant main effects of Stimulus group [F(3,72) = 0.04, P = 0.98, ηp2 < 0.01, BF = 0.12] or Block [F(2,48) = 1.96, P = 0.15, ηp2 < 0.01, BF = 0.31] or any interaction between the factors [F(6,114) = 1.2, P = 0.3, ηp2 < 0.01, BF = 0.2]. These results suggest that in our experimental design, the traditional causes of P300 are underrepresented, in general, and other causes might dominate the signal development.
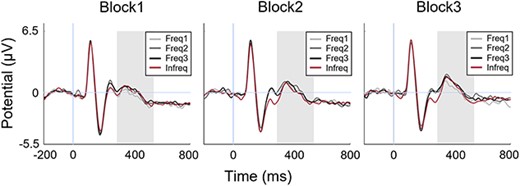
P300 results. Changes in P300 ERP amplitude as a function of time across the three experimental blocks for each stimulus group. Gray area signals the time window of analysis (300–500 ms).
Task-difficulty-based change in alpha ERD
Similarly to results of earlier odd-ball paradigms obtained with conventional stimuli, the α power in our experiment was not modulated significantly in the right hemisphere (Parise et al. 2018) (Fig. 4, right). The recorded α ERD responses in the left hemisphere developed gradually following the consolidation of the categories as indicated by the behavioral results (Fig. 4, left). While there was no difference in the α ERD signal across the four stimulus groups in Block1 [F(3,96) = 0.014, P = 0.96, ηp2 = 0.004, BF = 0.06], in the subsequent two blocks a highly prominent differentiation emerged: the level of α power for the two easy groups Freq1 and Freq2 remained at the same level as in Block1, whereas α power depression for the other two groups (Freq3 and Infreq) defining the category boundary increased substantially. This resulted in a significant difference between the depression caused by stimulus groups Freq1 and Freq2 vs. Freq3 and Infreq. This emergence of separation across blocks was confirmed by a repeated-measures two-way ANOVA showing a significant interaction between the four stimulus groups and the three blocks in the experiment with medium to large effect size and marginally strong evidence [F(6,144) = 2.96, P < 0.01, ηp2 = 0.12, BF = 2.6]. Apart from this interaction, there was also a significant main effect of stimulus groups [F(3,72) = 3.92, P = 0.031, ηp2 = 0.13, BF = 3.2].
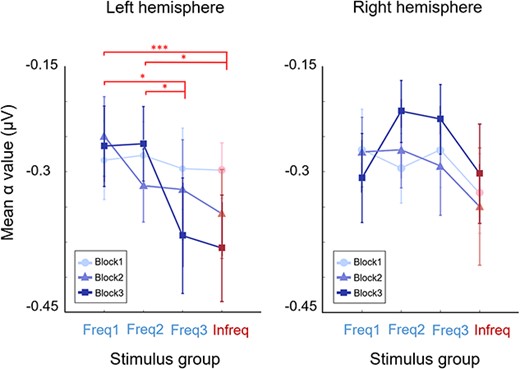
Alpha ERD results. Changes in the desynchronization patterns in the alpha band as a function of stimulus groups and practice (blocks) in the left and right hemispheres. Targeted pairwise comparisons indicated at the top refer to Block3 results. Missing signs indicate no significant differences, while *0.05, **0.01, ***0.001 level of significance. Plot depicts mean measured power in the band with SEM as error bars.
To identify the origin of the interaction effect, we investigated the pattern of the pairwise comparisons of the ERD responses. The pattern of these responses followed closely the pattern found with the participants’ behavioral performance but in the reverse direction. Specifically, significant differences were found between Freq3 vs. Freq1 by Wilcoxon signed-rank test [T = 249, z = 2.32, P = 0.019, r = 0.53, BF = 3.05] and between Freq3 vs. Freq2 [t(24) = 2.7, P = 0.012, Cohen’s d = 0.54, BF = 4.01] groups. Similarly significant differences were detected between Infreq vs. Freq1 [T = 276, z = 3.05, P = 0.001, r = 0.69, BF = 3.7] and Infreq vs. Freq2 [t(24) = 2.77, P = 0.011, Cohen’s d = 0.55, BF = 4.5] stimulus groups. Meanwhile, there was no significant difference between α responses for Freq1 vs. Freq2 [t(24) = 0.45, P = 0.65, Cohen’s d = 0.08, BF = 0.23] or between Freq3 vs. Infreq groups [t(24) = 0.3, P = 0.7, Cohen’s d = 0.03, BF = 0.22]. In summary, the most prominent changes in the α ERD responses due to learning in the left hemisphere occurred by a monotonic shift across blocks in the Freq3 and the Infreq groups, and these differences in the patterns emerged by starting with no differences across the four groups of stimuli in Block1 and converging to highly different α ERD responses in Freq1 and 2 vs. Freq3 and Infreq stimuli in Block3.
In order to link participants’ α ERD more closely to their behavioral performance, we ran an additional analysis calculating the correlation between categorization performance and average α power for each individual stimulus in each block. To correct for large individual differences, we scaled both α ERD and categorization performance into the range of [0–1] for each participant separately. Despite the increased ceiling effect in the behavioral results in Block3 compared to Blocks 1–2, we found a strong and significant correlation between categorization performance and alpha ERD in Block3 [r(10) = 0.64, P = 0.023, BF = 3.55], while the same analysis showed no significant correlations in Block1 [r(10) = 0.43, P = 0.16, BF = 0.8] or Block2 [r(10) = 0.42, P = 0.18 BF = 0.8] (Fig. 5). The emergence of this correlation across blocks can be spotted by noticing the simultaneous shrinkage of the range in the behavior performance (y-axis) and the expanding range of the average α ERD values (x-axis) across blocks in Fig. 5 resulting in a transition from a strongly vertical distribution of data points in Block1 to an oblique structure in Block3. The significant correlation between behavioral and neural results emerging in Block3 supports the idea that α ERD reflects the difficulty by which the observer can classify a stimulus to one of the newly formed categories. The individual patterns of the same correlation between performance and α ERD broken down by subjects corroborate these observations (Fig. S1).
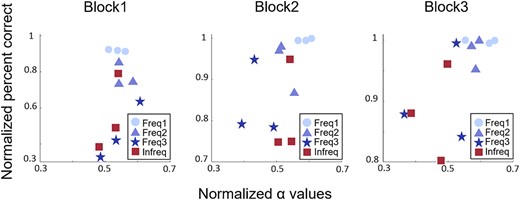
Relationship between α power and behavioral performance. Correlation between normalized mean absolute α ERD (x-axis) and normalized categorization performance (y-axis) for the 12 stimuli used in the experiment averaged across subjects. Correlations were calculated for each block separately. Note the expanding range of the mean α ERD values across blocks and their gradual separation between the easy- (Freq 1–2) and the hard-to-classify (Freq3, Infreq) groups. Also note the different ranges of the y axes in the three panels reflecting the improved categorization performance across the three blocks and leading to an emerging ceiling effect in Block3.
Category-related change in theta ERS
The θ responses displayed a markedly different pattern from that of the α ERD responses (Fig. 6). Results of a repeated measures two-way ANOVA on the effects of stimulus groups and blocks showed no significant interaction [F(6,144) = 1.78, P = 0.10, ηp2 = 0.07, BF = 0.48] nor significant main effect of experimental block [F(2,48) = 0.47, P = 0.62, ηp2 = 0.02, BF = 0.078], but indicated a main effect of stimulus groups [F(3,72) = 5.25, P = 0.002, ηp2 = 0.18, BF = 6.1]. However, this main effect came predominantly from the steady and monotonic increase of θ ERS in the Infreq group alone. By Block3, significant θ differences emerged between the Infreq group vs. all the Freq stimulus groups: Freq1 [t(24) = 3.57, P = 0.002, Cohen’s d = 0.77, BF = 24]; Freq2 [t(24) = 3.79, P < 0.001, Cohen’s d = 0.75, BF = 38]; Freq3 [t(24) = 2.39, P = 0.025, Cohen’s d = 0.42, BF = 3.2]. In contrast with this and also with the pattern observed with α responses, θ ERS for the three stimulus groups within the frequent category did not show any significant change as quantified by three different measurements. First, while there was an apparent effect of proximity of the stimulus group to the category boundary in Block3, the statistical evidence significantly excluded this effect [F(2,48) = 0.95, P = 0.39, ηp2 = 0.026, BF = 0.28]. Second, the effect of category formation did not alter the θ ERS of the three frequent blocks in any significant way as indicated by the lack of the main effect of the blocks factor in the repeated measure two-way ANOVA above. This was also confirmed by the substantial evidence supporting the lack of a main effect due to blocks in a separate ANOVA performed only on the three Freq groups [F(2,48) = 0.45, P = 0.63, ηp2 = 0.005, BF = 0.09]. Third, based on the results of a one-way ANOVA with blocks as grouping variable, the θ ERS of the Freq3 group did not show any monotonic drift across blocks [F(2,48) = 0.5, P = 0.6, ηp2 = 0.014, BF = 0.16] in contrast to the drift found in the Infreq group. This behavior of the θ responses was in stark contrast with the monotonic simultaneous change in the α ERD signals of the Freq3 and Infreq groups across blocks.
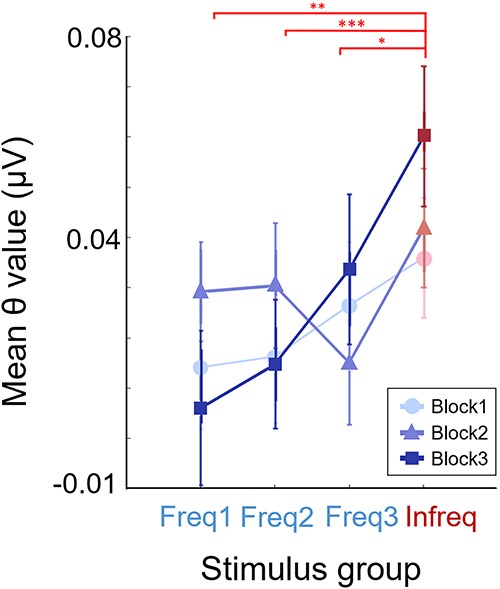
Theta ERS results. Changes in synchronization patterns in the theta band as a function of stimulus groups and practice (blocks). Plot depicts mean the measured power in the band with SEM as error bars.
Discussion
We created a modified version of the widely used oddball paradigm to investigate two main questions in our study. First, whether the temporal evolution of the commonly investigated neural correlates of categorization behavior (P300 ERP, α ERD, and θ ERS) would reflect the gradual formation of new visual categories. Second, whether the changes in those signals due to learning new categories under more complex scenarios could be meaningfully linked to various aspects of the learning process such as the difficulty or category membership. To this end, participants had to gradually build a stable representation about the unknown integrated-dimensional categories block-by-block throughout the experiment without reaching a ceiling in their categorization performance for the majority of stimulus groups even in Block3. Stimuli in the Infreq and the Freq3 groups that were the closest to the category boundary proved to be significantly more difficult for participants to categorize than the rest of the stimuli with significantly lower confidence for these stimulus groups. This unique and quantified setup allowed a more nuanced evaluation of neural signatures in the light of the behavioral performance providing notable results regarding all three neural correlates investigated.
In the case of P300 ERPs, acquisition of the categories across blocks was accompanied by a gradual increase in amplitude of the signal. However, significant differences in P300 ERPs did not emerge between any of the stimulus groups even by Block3. Although this finding is at odd with reports of existing causal relationship between P300 ERP and α ERD during an oddball paradigm (Peng et al. 2012), there exist at least two possible explanations for the lack of finding any difference in our study. First, it is possible that a more pronounced frequency difference between category exemplars is necessary to elicit P300 differences in such complex tasks. Typical frequency differences between expected and infrequent stimuli applied in oddball paradigms range from 1:9 to 1:4 (Yordanova and Kolev 1998a, 1998b; Yordanova et al. 2001; Sochurková et al. 2006; Peng et al. 2015; Harper et al. 2017), and the amplitude of the P300 ERP was modulated with all these frequency differences. In our study, the frequency difference was 1:3 that, in principle, might have been insufficient to ignite the effect responsible for the emergence of the P300 ERP differences. However, this explanation is less likely as oddball effects have been found even with 1:3 rations and there exist other differences between the setups of those studies and ours that could be more directly responsible for the lack of the P300 effect.
In particular, the second possible explanation for the lack of an effect is that neural processes related to P300 need a longer consolidation period for the acquired knowledge to be affected by the frequency differences than the 1-h period allowed in our experiments. Indeed, similarly to our study, Parise et al. (2018) taught participants to build two new categories but based on 3-3 novel objects during their training phase. During the training phase, one category was defined by all three elements, while only one member of the other training triplet was used to define the other category creating a 1:3 frequency ratio similarly to our design. In the subsequent test phase, participants had to categorize the elements based on the recently acquired categories and showed no P300 differences for the newly acquired categories. However this result was in sharp contrast to the outcome of a preceding study from the same authors using the same frequency ratio and design but already familiar rather than novel objects for creating the categories, where the observers exhibited significant P300 ERP differences. A second example is the above-mentioned study (Peng et al. 2012), in which only a triangle and a dot were used as rare and frequent stimuli yielding again significant P300 ERP differences. Thus, the difference between presence and absence of a pronounced P300 effect in those two studies vs. ours could be related to familiar vs. non-familiar shapes forming the categories. In our study, this lack of a detectable effect might have been further enhanced by the fact that the stimuli used in the experiment were visually very similar requiring scrutiny to encode any stimulus during categorization. We posit that building up familiarity with such new stimuli –a prerequisite for category formation- might require additional learning at the level of the individual stimuli. If this proposal is correct, the emergence vs. lack of a P300 oddball effect in an oddball study coupled with oddball effects present in other frequency ranges might potentially be a useful tool for studies investigating whether or not the overall oddball effect is driven by features and/or categories previously already well established in the observer’s mind. Furthermore, sleep-related consolidation studies could directly test this proposed link between the emergence of P300 effects and the amount of consolidation provided for the new categories.
In contrast to P300 ERP, the significant change in the α ERDs emerging by Block3 indicate that α ERD was sensitive even to newly acquired categories. Since the oddball effect in our study can be interpreted only at the level of the abstract categories but not at the level of the individual stimuli, we propose that eliciting such a difference in α ERD across blocks is a valid indication of forming internal representations of these abstract categories. The gradual nature of this emergence makes it a useful tool for tracking the ongoing acquisition of novel categories. Despite its gradual emergence and link to category formation, the α ERD does not seem to reflect membership to the different categories in an all-or-none manner. Based on the close similarity between the pattern of desynchronization and behavioral categorization performance (Fig. 2 vs. Fig. 4), we propose that α ERD is modulated mainly by task difficulty through the amount of involvement a given stimulus requires to be classified in a given task, and this modulation emerges in parallel with the formation of the new categories in our task. Specifically, early on, in Block1, none of the stimuli require significant involvement to process, hence all stimuli elicit a moderate amount of α ERD. Later as the categories emerge, shapes in Freq1 and Freq2 still need no additional processing as they clearly do not belong to the infrequent category. In contrast, shapes in the Freq3 and Infreq groups have to be scrutinized to obtain the correct categorization and this process elicits a significantly larger α ERD. This interpretation is supported by the observed significant correlations between α ERD and behavioral performance in Block3 depicted in Fig. 5.
The above interpretation also fits well into the more general proposals about what cognitive functions α-power variations might indicate. While earlier theories posited that change in α-power merely signaled brain’s transition from idle to engaged state (Pfurtscheller et al. 1996), more recent findings suggest a clear link between those changes and cognitive functions (Klimesch 1999; Basar and Güntekin 2012). Moreover, contemporary views acknowledge the multifaceted nature of α modulation that includes α ERS indicating vigilance, α ERD related to selective attention and long-range α-phase locking that facilitates phasic adaptive control (Sadaghiani and Kleinschmidt 2016). Our method probably taps mainly into the selective attention function focusing on details of the stimulus as this function is relevant for identifying which category a stimulus belongs to and it should reflect proportionally the difficulty of this categorization. Accordingly, we found ERD of our α signal manifested in the electrodes over the occipitoparietal cortex the proposed location of selective attention processes (Sadaghiani and Kleinschmidt 2016).
Our finding that α ERD tracks the ongoing emergence of novel categories defined on complex stimuli with smoothly varying features and it reliably reflects the subjective difficulty of performing this task with a given stimulus has two significant implications. First, beyond being in line with previous literature showing that α ERD is modulated by the amount of mental effort required for solving a task (Klimesch 1997; Sutoh et al. 2000; Zhu et al. 2021), our observation also expands those findings and provides a new tool. Specifically, our smoothly changing stimuli modulate the task difficulty across groups Freq 1–3 in a particular way, through a clearly observable metric conveying the strength of the evidence that the stimulus belongs to a given category. However, our results showing that this “level of belongingness” is tracked by α ERD also implies that α ERD can be used to gauge how much an observer subjectively considers a particular stimulus to be a member of a given category even in tasks where the stimuli lack any clearly observable metric of category belongingness from the experimenter’s standpoint. Thus, our method can give a behavior-based glimpse into the observer’s internal interpretation of the stimulus set contrary to the conclusion of earlier studies based on perceptual learning paradigms that found no stimulus-specific component of the changes of α ERD during learning (Bays et al. 2015) The second implication of our findings is that linking the neurophysiological measure of α ERD process to the emergence of arbitrary novel categories makes α ERD useful not only for gauging the level of belongingness of a particular stimulus but also for indicating the progress of the formulation of new categories.
Similar to α ERD, θ ERS responses emerged gradually throughout our experiment, following the course of learning. Significant differences in θ ERS between members of the frequent and infrequent categories clearly signaled the acquisition of mental categories. In addition, θ ERS seemed to be somewhat sensitive to the strength of category membership, as within the frequent category, θ synchronization showed a tendency to increase with the proximity of stimulus groups to the category boundary. However, in contrast to the case with α ERD, the validity of this modulation by the strength of membership has been decisively rejected by evidence. This separation between α ERD and θ ERS is incongruent with earlier reports finding a strong modulation of theta activity in the same cortical area by the difficulty of the task and with practice in verbal and spatial matching tasks involving working memory (Gevins et al. 1997). However, the discrepancy can be reconciled by realizing that despite the existing strong interplay between the processes related to α- and θ-band activity creating a covariation of these signals (Klimesch et al. 1994), these processes are likely to concern different aspects of cognitive functioning. While desynchronization in the α band is linked to processes involving semantic memory, θ synchronization is related to episodic memory often through working memory (Klimesch 1999). This dichotomy raises the possibility that while α ERD, strictly localized in the left hemisphere (Klimesch et al. 1997), is driven by the difficulty to establish the conceptual mapping of the stimulus to one of the categories, θ ERS is more related to the successful or unsuccessful retrieval of one of the infrequent episodic memory traces stored. This would explain why changes in both types of signals emerge gradually following the course of learning, but once the category representations are formed, α ERD indicates more the task difficulty, whereas θ ERS instead reflects more prominently the strength of within-category membership driven mostly by responses to the infrequent stimuli. As the interplay and relative weight between semantic and episodic aspects of any task strongly vary, further studies will be required to carefully establish the conditions under which the tendency of θ ERS to indicate class-membership becomes reliable.
In a broader context, our work is related in a particular way to the research area of how humans learn new internal representation. On the one hand, our paradigm can be viewed as classical perceptual learning since we use feedback, the shape stimuli are relatively hard to discriminate and the task is category formation based on fine differences of features (Fiser and Lengyel 2022). On the other hand, our session does not stretch across multiple days, the categories are novel and arbitrary and our feature dimensions are complex reminiscent to conditions in classical category learning or reinforcement learning paradigms (Ashby and Maddox 2005; Botvinick et al. 2019). The neural correlates of such learning are typically investigated in the context of neural encoding theoretically based on either rate-base (Kriegeskorte and Kievit 2013) or probabilistic approaches (Ma et al. 2006; Fiser et al. 2010). However, the goal of our work was different from exploring neural encoding per se, we aimed at tying widely used measures related to ERP more specifically to various aspects of the categorization process. Our results can be viewed as an existence proof of such more specific links, which is a first step toward a deeper understanding how and why the links between alpha ERD or theta ERS and difficulty or category membership emerge and how the actual neural encoding of categories is linked to these phenomena.
In conclusion, we have demonstrated that commonly investigated neural signals associated with the process of categorization can meaningfully be interpreted with more complex and more natural category structures than the ones previously used in similar studies. We found that P300 ERP was not a useful signal for tracking ongoing emergence of new category representations of members with continuously and finely varying feature dimensions. In contrast, both α ERD and θ ERS not only followed the process of category acquisition, but they predominantly signaled different, but equally important aspects of this categorization. Specifically, α ERD was modulated more by subjective task difficulty, while θ ERS reflected, at least the category membership and possibly the strength of category membership for a given stimulus. Our results provide an implicit tool for mapping the emergence and the structure of category representations in humans and they help refining previous hypotheses on the functions of the investigated neural responses.
List of abbreviations
EEG—electroencephalogram; ERP—event related potential; ERD—event related desynchronization; ERS—event related synchronization; P300—a positive change in neural potential about 300 ms after stimulus presentation; Freq1, Freq2, Freq3—name of the stimulus subgroups in the “frequent” category of the oddball setup ordered from most to least dissimilar from the Infreq group, respectively; Infreq—refers to the group of stimuli comprising the “infrequent” category in the oddball setup of the experiment; d—Cohen’s d: measure of effect size; ηp2—partial eta: measure of effect size.
CRediT author statement
Sara Jellinek (Conceptualization, Data curation, Formal analysis, Investigation, Methodology, Writing—original draft), Jozsef Fiser (Conceptualization, Funding acquisition, Methodology, Supervision, Writing—review and editing).
Funding
This work was supported in part by grants ONRG-NICOP-N62909-19-1-2029 (to J.F.), CEU-ITI-2020 (to J.F.), and NSF PHY-1748958 to the Kavli Institute for Theoretical Physics (KITP).
Conflict of Interest statement: None declared.