-
PDF
- Split View
-
Views
-
Cite
Cite
Jing Wang, Xiaosha Wang, Jiajie Zou, Jipeng Duan, Zhuowen Shen, Nannan Xu, Yan Chen, Jianfeng Zhang, Hongjian He, Yanchao Bi, Nai Ding, Neural substrate underlying the learning of a passage with unfamiliar vocabulary and syntax, Cerebral Cortex, Volume 33, Issue 18, 15 September 2023, Pages 10036–10046, https://doi.org/10.1093/cercor/bhad263
- Share Icon Share
Abstract
Speech comprehension is a complex process involving multiple stages, such as decoding of phonetic units, recognizing words, and understanding sentences and passages. In this study, we identify cortical networks beyond basic phonetic processing using a novel passage learning paradigm. Participants learn to comprehend a story composed of syllables of their native language, but containing unfamiliar vocabulary and syntax. Three learning methods are employed, each resulting in some degree of learning within a 12-min learning session. Functional magnetic resonance imaging results reveal that, when listening to the same story, the classic temporal-frontal language network is significantly enhanced by learning. Critically, activation of the left anterior and posterior temporal lobe correlates with the learning outcome that is assessed behaviorally through, e.g. word recognition and passage comprehension tests. This study demonstrates that a brief learning session is sufficient to induce neural plasticity in the left temporal lobe, which underlies the transformation from phonetic units to the units of meaning, such as words and sentences.
Introduction
Speech comprehension is a complex process involving various processing stages, such as encoding acoustic features, decoding phonetic categories, recognizing words, and extracting higher-level syntactic and semantic information (Kemmerer 2014; Hickok and Small 2016). These intricate processes are implemented in distributed cortical networks, and numerous influential models have been proposed to elucidate the functional organization of the speech processing network (Hickok and Poeppel 2007; Rauschecker and Scott 2009; Friederici 2011; Hagoort 2019). Although the general architecture of the speech processing network is relatively well characterized, accurately mapping each speech processing function onto a cortical network still faces several challenges. One such challenge is that most neuroimaging studies attempt to localize the neural substrate underlying a speech function by contrasting two conditions. However, constructing two conditions that differ exclusively in one speech function proves to be difficult (Price 2012). For instance, in order to isolate the neural network for sentence processing, a prevalent contrast is between activation to sentences and word lists (Hashimoto and Sakai 2002; Humphries et al. 2007; Friederici 2011; Brennan and Pylkkänen 2012; Fedorenko et al. 2016). These two conditions, however, also diverge in lower-level stimulus properties that do not engage sentence processing, such as the transitional probability between words, speech prosody, and word properties (unless the set of words between the two conditions entirely overlap).
Language learning offers an alternative method for examining the cortical network involved in speech comprehension. This method allows for the comparison of neural activation in response to the same speech utterance before and after learning, effectively controlling the lower-level acoustic and statistical properties of the stimulus. This approach has been applied to localize the cortical regions engaged in mapping distorted speech features onto phonetic categories (Giraud et al. 2004; Eisner et al. 2010) and learning new words that are presented in isolation (Wong et al. 2007; Macedonia et al. 2011; López-Barroso et al. 2013; Ripollés et al. 2014; Glezer et al. 2015; López-Barroso et al. 2015; Yang et al. 2015; Barbeau et al. 2017). Here, we extend this learning paradigm to probe the neural network processing words, sentences, and passages in connected speech.
Specifically, we developed a novel speech learning paradigm, enabling participants to quickly learn to comprehend a brief spoken story in an unfamiliar language that shares the same phonetic units with their native language but has distinct vocabulary and syntax. Specifically, the participants are native Chinese listeners learning to comprehend a story in Classical Chinese, a language employed for formal writing in China until the early 20th century. Classical Chinese and modern Chinese can be produced by the same set of syllables/graphemes, but differ in vocabulary and syntax. Sentence boundaries are not marked by punctuation in Classical Chinese and must be inferred based on the text. The learning outcomes are assessed through four behavioral tests, focusing on the ability to recognize words, identify sentence boundaries, accurately decode word meaning, and comprehend the story, respectively.
Furthermore, to induce substantial individual differences and dissociate the effects of learning outcome and learning method, we employed three distinct learning methods that varied in their effectiveness at inducing learning in different aspects (Fig. 1). The comprehensive learning method, similar to how current Chinese student learned Classical Chinese in school, directly informed the mapping from sound to meaning. The sentence boundary learning method and the translation learning method provided either phonological or semantic information, requiring the participants to infer the mapping from sound to meaning. The former method only cued the boundary between sentences, partly simulating how students learned to read Classical Chinese in the ancient time, and the latter method only provided a modern Chinese translation of the story.
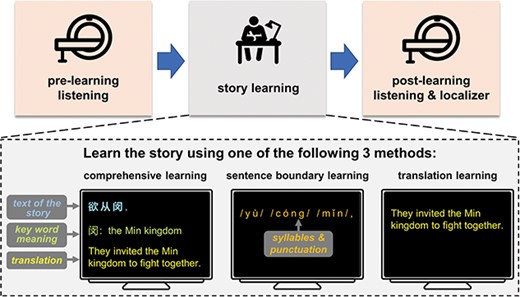
Experimental procedure. The experiment consists of a pre-learning listening session, a story learning session, and a post-learning listening session. MRI scans are acquired during pre-learning and post-learning listening sessions. In the story learning session, which is conducted outside the scanner, three groups of participants learn the story using three different learning methods. Annotations and translations are illustrated in English, but are shown in Chinese characters in the real experiments. After the post-learning listening session, a set of behavioral comprehension tests are applied (not shown here).
We acquired the neural responses to a spoken story using fMRI both before and after learning (Fig. 1). The intense noise generated by the typical fMRI scanning process, however, provided additional challenges to constrain the learning effect to the word level and beyond: in a noisy environment, speech recognition is higher for meaningful sentences than random words (Miller et al. 1951), and is also higher for more familiar sentences (Freyman et al. 1999). Consequently, in the presence of intense noise, phonetic processing is also enhanced after learning. To overcome this challenge, we employed a sparse sampling procedure to ensure that the listeners could hear the story in a quiet environment, so that phones could well recognized both before and after learning. We analyzed (i) which cortical areas are significantly modulated by learning, (ii) which cortical areas were sensitive to the learning method, and (iii) which areas were responsive to the behaviorally measured learning outcome.
Materials and methods
Participants
The fMRI experiment recruited 75 right-handed native Chinese speakers (41 females, mean age 23 years, range 20–29 years). The participants were randomly assigned to three learning groups. Four participants were excluded due to severe signal loss in the frontal lobe, coughing or falling asleep during the experiment. We further excluded 11 participants with head motion exceeding 3 mm/3°. Twenty participants were included in the analysis for each learning group, i.e. the comprehensive learning group (12 females, age: 23 ± 2 years old), the sentence boundary learning group (10 females, age: 24 ± 2 years old), and the translation learning group (10 females, age: 23 ± 2 years old). Additional 17 right-handed native Chinese speakers (12 females, mean age 26, range 20–29) participated in the behavioral control test. The participants reported no hearing loss or history of neurological disorders. All participants were undergraduates or graduates of Zhejiang University and were not majored in Chinese Language and Literature, a major that received in-depth training on reading Classical Chinese. Participants all gave written consent and were paid for their participation. The study was approved by the Research Ethics Committee of the College of Medicine, Zhejiang University.
Stimuli
Stories
A segment of a Classical Chinese story and a segment of a modern Chinese story were presented. The Classical Chinese story was adapted from the “Biography of East Yue” in Records of the Grand Historian (i.e. Shiji). Records of the Grand Historian was a monumental history of ancient China written in ~ 94 BC, and East Yue was the name of an ancient state. Some multisyllabic proper nouns in the story were preserved in modern Chinese and therefore might be recognized without learning. To avoid that these words could be easily recognized, we preserved only one syllable for each multisyllabic proper noun. The segment of the story presented in the experiment had 655 characters/syllables, which constituted a relatively complete story (see Supplementary Information). Overall, the story was divided into 131 sentences. In this study, for simplicity, we referred to any sequence of words between two punctuations as a sentence, including, e.g. word sequences separated by a comma. The modern Chinese story was adapted from a modern Chinese translation of “Biography of Dawan” in Records of the Grand Historian. Dawan was the name of another ancient state. The segment of the story presented in the experiment presented also had 655 characters/syllables.
Speech synthesis
The speech for each story was synthesized by concatenating syllables that were independently synthesized using the Neospeech synthesizer (http://www.neospeech.com/; the male voice, Liang). All syllables were adjusted to 250 ms in duration and no acoustic gaps were added between syllables. Consequently, the stimulus duration was 163.75 s for each story. Speech synthesized this way contained no phrasal-level prosodic cues.
Stimulus epoching
Each story was divided into 15 epochs and the duration of each epoch was 12 s. Neighboring epochs had 1 s in overlap, and the last epoch had 1.25 s of silence at the end. The onset and offset of each epoch were smoothed using a 1/2-s ramp (cosine window). In the experiments, epochs of stories and random syllable sequences were interleaved (see Fig. 2). The random syllable sequences were constructed by shuffling the syllables in the same story segment. The duration, overlap, and onset/offset ramp for each random syllable epoch was the same as those for story epochs.
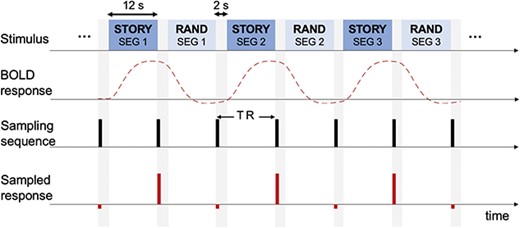
Stimulus and fMRI scanning procedure. Epochs of stories and random syllable sequences are presented interleavingly. The fMRI response to each epoch is acquired using a sparse sampling approach (Hall et al. 1999): no fMRI scanning is engaged during speech listening. After each epoch, fMRI scan is acquired during a 2-s silence period.
Experimental procedures
The experiment consisted of 3 sessions, i.e. a pre-learning listening session, a learning session, and a post-learning listening session. Functional magnetic resonance imaging (MRI) scans were acquired during the pre- and post-learning listening sessions. In the learning session, participants learned about the Classical Chinese story they heard outside the MRI scanner. In the pre-learning listening session, participants listened to the Classical Chinese story interleaved with random syllables. The stimulus was presented twice in two separate runs, and participants were only instructed to listen carefully. No instruction was given about what kinds of speech they would hear, e.g. stories or random syllables. After the pre-learning session, participants subjectively rated their level of comprehension of the Classical Chinese story. A learning session followed, which was detailed in Learning methods.
After the learning session, the participants went through the post-learning listening session. This session presented a Classical Chinese story and a modern Chinese story, both of which were interleaved with random syllables. Each story was presented twice in separate runs, and the listening order was as follows: the Classical Chinese story, the modern Chinese story, the modern Chinese story, and the Classical Chinese story. Before listening, participants were explicitly instructed that they would listen to the learned Classical Chinese story and a modern Chinese story, which were interleaved with random syllables. To ensure attention, we informed the participants that they needed to complete tests for both Classical and modern Chinese stories and write from memory the Classical Chinese story. Nevertheless, after scanning, the participants were only given comprehension tests (see Comprehension tests) but not required to write down the whole Classical Chinese story, since the task was too challenging and time consuming.
Learning methods
In the learning session, participants were explicitly informed that they were to learn the Classical Chinese story they heard in the pre-learning listening session. The participants were randomly assigned to three learning groups, each learning the story using a different method. The three learning methods are comprehensive learning, sentence boundary learning, and translation learning. The translation learning group was only provided with a modern Chinese translation of the story. In contrast, the sentence boundary learning group was provided with punctuation information, on top of the pronunciation information that was also available during pre-learning listening. The comprehensive learning was provided with all information that was available to the translation learning group and the sentence boundary learning group, and was additionally provided with the text of the story and annotations of key word meaning.
All groups of participants followed a two-stage learning procedure that consisted of a silent reading stage and a reading aloud stage. In the silent reading stage, participants read printed materials to learn the story, and the printed materials contained different types of information for different learning groups (Table 1). The stage lasted for 6 min for comprehensive and sentence boundary learning groups, and was shorter, i.e. 3.4 min, for the translation group since the participants could read modern Chinese more quickly.
. | Text . | Key word meaning . | Punctuation . | Pronunciation (pinyin) . | Translation . |
---|---|---|---|---|---|
Comprehensive learning | ✓ | ✓ | ✓ | ✓ | ✓ |
Sentence boundary learning | ✓ | ✓ | |||
Translation learning | ✓ |
. | Text . | Key word meaning . | Punctuation . | Pronunciation (pinyin) . | Translation . |
---|---|---|---|---|---|
Comprehensive learning | ✓ | ✓ | ✓ | ✓ | ✓ |
Sentence boundary learning | ✓ | ✓ | |||
Translation learning | ✓ |
. | Text . | Key word meaning . | Punctuation . | Pronunciation (pinyin) . | Translation . |
---|---|---|---|---|---|
Comprehensive learning | ✓ | ✓ | ✓ | ✓ | ✓ |
Sentence boundary learning | ✓ | ✓ | |||
Translation learning | ✓ |
. | Text . | Key word meaning . | Punctuation . | Pronunciation (pinyin) . | Translation . |
---|---|---|---|---|---|
Comprehensive learning | ✓ | ✓ | ✓ | ✓ | ✓ |
Sentence boundary learning | ✓ | ✓ | |||
Translation learning | ✓ |
In the reading aloud stage, the story was auditorily presented sentence by sentence, and information available in the printed materials was also shown on the screen (Table 1 and Fig. 1). After each sentence, the participants shadowed the sentences. The silent reading stage allowed the participants to quickly go through the printed materials, and the reading aloud stage ensured that the participants were engaged and strengthened verbal memory through active speech production (Adank et al. 2010). The stage lasted for 6 min for comprehensive and sentence boundary learning groups and was longer, i.e. 8.6 min, for the translation group since the modern Chinese translation had many more characters than the Classical Chinese story.
Comprehension tests after post-learning scanning
Immediately after post-learning scanning, participants were given four comprehension tests, i.e. the word recognition test, sentence boundary test, word meaning test, and passage comprehension test, to evaluate their level of understanding (see Table S1 for details). The tests were identical for the three learning groups.
Word recognition test
Participants listened to sentences from the Classical Chinese story and typed in the words they heard. The sentences could be replayed for an unlimited number of times. The test required the participants to convert the syllables into Chinese characters. The task was challenging since the mapping from syllables to morphemes was one to many. For example, the syllable “lv4” corresponded several characters that could mean, e.g. law, green, ratio, and filter. Twenty sentences were presented in total (268 characters). The word recognition accuracy was the percent of characters that were correctly typed in.
Sentence boundary test
Participants were given the unpunctuated text of the Classical Chinese story, and were required to denote the boundaries between sentences using slashes. The accuracy was calculated as the number of slashes that matched the position of a comma/period divided by the total number of commas/periods in the whole story.
Word meaning test
Participants were given 10 short excerpts, each containing one to two sentences, from the Classical Chinese story. For each excerpt, they had to explain the meaning of a couple of key words using modern Chinese. Eighteen words were chosen in total, and 10 words were annotated words in the learning materials provided for the comprehensive learning group. Words with dissimilar meaning between classical and modern Chinese were selected. The corresponding modern Chinese translations of the sentences which contained the marked words were not presented in the learning materials. The experimenter scored whether the explanation was correct or not. This test evaluated more fine-grained interpretation than the word recognition test.
Passage comprehension test
Participants were given 10 general comprehension questions about the Classical Chinese story and had to choose the correct answer from four options. The corresponding modern Chinese translations of the sentences which directly answered the questions were not presented in the learning materials. The test required the participants to deduce a consistent storyline by comprehending the context.
After the four tests, the general ability to read Classical Chinese was also tested. In this test, participants read another Classical Chinese story, which was adapted from “Biography of Hun” in Records of the Grand Historian. After reading the story, the participants accomplished a word meaning test (10 key words in total).
Behavioral control experiment and data analysis
We run an additional behavioral experiment to evaluate how well participants could learn the Classical Chinese story without an explicit learning session. The procedure of the experiment was the same as the procedure of the MRI experiment, except for two changes. First, the whole experiment was conducted in a quiet room and no fMRI scans were acquired. Second, the learning session was skipped. However, after the pre-learning listening session, the participants were informed that what they heard and would hear again was a Classical Chinese story interleaved with random syllables. After post-learning listening, the participants went through the same comprehension tests.
For the behavioral data, a one-way ANOVA was conducted using the car package in R. Follow-up post-hoc analyses were conducted using the emmeans package in R. The P-values were corrected for multiple comparisons using the Bonferroni correction.
fMRI data acquisition and analysis
fMRI data acquisition
Structural and functional data were acquired using a Siemens Prisma 3 T scanner (Siemens Healthcare, Erlangen, Germany) with a 20-channel head–neck coil at the Center for Brain Imaging Science and Technology, Zhejiang University. A high-resolution 3D T1-weighted anatomical scan was collected using the magnetization-prepared rapid-acquisition gradient-echo sequence: repetition time (TR) = 2,300 ms, echo time (TE) = 2.32 ms, inversion time = 900 ms, flip angle (FA) = 8°, field of view (FOV) = 240 mm2, matrix size = 256 × 256, slices = 192, slice thickness = 0.9 mm, and voxel size = 0.9 mm3. To avoid scanning noise during listening, we acquired functional data under a sparse sampling acquisition paradigm using a simultaneous multi-slice echo-planar imaging sequence: TR = 14,000 ms, TE = 34 ms, FA = 50°, FOV = 230 mm2, matrix = 64 × 64, slices = 52, slice thickness = 2.5 mm, voxel size = 2.5 mm3, multi-band acceleration factor = 4, Delay in TR = 13,000 ms.
In our sparse sampling procedure, 1-s image acquisition was followed by a 13-s MRI silent period; and 0.5 s after the first image acquisition, 12-s epochs were presented with a 2-s interval between each epoch. This resulted in a 0.5-s gap before and after each scan to avoid sound overlap between the stimuli and the scanning noise. Six runs of data were acquired, with two runs for each condition, i.e. pre-learning listening, post-learning listening, and modern Chinese listening. Each run started with 6 volumes during which no stimulus was presented, followed by 15 volumes for each sequence (stories and random syllables). The first 5 volumes were acquired but not included in the final dataset, resulting in a total of 31 volumes for each run. The duration for the MRI scan was ~ 14 min before learning and ~ 28 min after learning.
fMRI data preprocessing
Four participants were excluded due to severe signal loss in the frontal lobe, coughing or falling asleep during the experiment. Functional images of the remaining participants were preprocessed using Statistical Parametric Mapping (SPM12; Wellcome Trust Center for Neuroimaging, London, UK, http://www.fil.ion.ucl.ac.uk/spm/). For each subject, the first volume of each functional run was discarded. Other images were firstly corrected for head motion. Eleven participants were excluded from further data analysis due to excessive head movement (> 3 mm or 3|${}^{\circ}$|). The realigned images were then coregistered to the T1 image scanned at the beginning of each session, spatially normalized to the Montreal Neurological Institute (MNI) space via unified segmentation (resampling into 3 mm × 3 mm × 3 mm voxel size), and high-pass filtered at 128 s. These images were further smoothed using a 6-mm full-width half-maximum Gaussian kernel.
fMRI analysis
For the first-level analysis, a generalized linear model was built for each participant, in which each scan was coded as belonging to one of the three conditions (pre-learning Classical Chinese story listening, post-learning Classical Chinese story listening, and modern Chinese story listening). The random syllables served as the baseline, and we always reported the contrast between stories and random syllables. Within the design matrix, each run consisted of one regressor corresponding to the story-listening condition, convolved with the canonical hemodynamic response function (HRF), and six movement regressors of no interest. Contrast images for each of the three experimental conditions were generated by averaging the two parameter estimates within conditions.
For the second-level analysis, to identify brain regions sensitive to speech comprehension, we carried out one-sample t-test on the beta images of modern Chinese story learning across all the subjects. The same one-sample t-tests were carried out for the beta images of the pre- and post-learning Classical Chinese story listening, respectively. We further conducted a ROI analysis to examine the responses in the early auditory cortex during Classical Chinese story listening. The left Heschl’s gyrus was anatomically defined using the WFU Pickatlas toolbox (REF) and the parameter estimates before and after learning were extracted, averaged across voxels, and then compared with zero across all the subjects using one-sample t-test. To examine the main effect of method, we averaged the contrast images of pre- and post-learning per subject at the first level and then performed a one-way ANOVA at the second level. The main effect of learning and the interaction between learning and method were performed using a flexible factorial design; the design matrix, contrast weights, and Matlab script are available at https://osf.io/pe3fw/?view_only=07c36d3efbcf45a0a6b022c008ceae32f. Specifically, subjects were included as a factor to account for random variance between subjects; method (comprehensive learning [CL], sentence boundary learning [SBL], and translation learning [TL]) was included as a between-subject factor and learning (pre- and post-learning) as a within-subject factor. The contrasts of interest were the main effect of learning [(CL_post & SBL_post & TL_post) versus (CL_pre & SBL_pre & TL_pre)], and the learning × method interaction [(CL_post & TL_pre) versus (CL_pre & TL_post); (SBL_post & TL_pre) versus (SBL_pre & TL_post)]. All statistical maps above were thresholded at voxelwise P < 0.001, FWE-corrected cluster-level P < 0.05, one-tailed.
To examine the correlations between post-learning cortical activation and each of the four behavioral tests, we carried out the second-level regression analysis in SPM on the post-learning activation images, using the performance on a test of interest as the independent variable and further including the performance on the proficiency test in Classical Chinese as a nuisance variable. Statistical maps were first thresholded at voxelwise P < 0.001 and Bonferroni correction was further used to adjust for multiple comparisons of the four behavioral tests, i.e. cluster-level p-value was set at FWE-corrected P < 0.0125, one-tailed.
Results
Behavioral results: comprehension tests
After the pre-learning listening session, the participants subjectively rated that they understood 16.25% (standard error 1.81%, range 0–37.5%, Fleiss’ Kappa = −0.017) of what they heard. After the post-learning listening session, the comprehension of the story was assessed using four comprehension tests. Performance on the four tests was significantly influenced by the learning method (Fig. 3, F3,73 = 30.87, 17.37, 7.12, and 8.75, for the word recognition, sentence boundary, word meaning, and passage comprehension tests, P < 0.01 for all tests, Bonferroni corrected). For all tests, the performance was highest for participants in the comprehensive learning group. For the word recognition test, the sentence boundary learning group also outperformed the translation learning and control groups. Furthermore, for the four groups of participants, the ability to read Classical Chinese was not significantly different (one-way ANOVA, F3,73 = 1.19, P = 0.321).
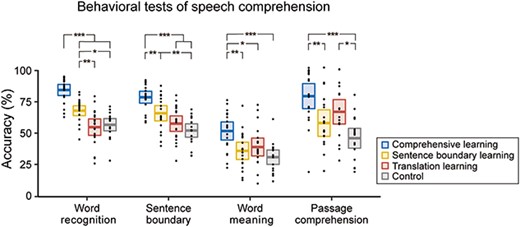
Behavioral comprehension tests. Black dots denote individual results. Colored horizontal lines denote the mean across participants. Rectangles cover the 95% confidence interval of the mean. *P < 0.05, **P < 0.01, ***P < 0.001, Bonferroni corrected.
fMRI results: whole-brain analysis
We first reported the cortical activation to the modern Chinese story localizer. One-sample t-test revealed broad activation in the fronto-temporal networks, including, e.g. bilateral superior temporal gyrus (STG) and bilateral inferior frontal gyrus (IFG; Fig. 4; Table S2). This cortical activation pattern was highly consistent with previous studies (Fedorenko et al. 2010), confirming the validity of the sparse sampling procedure. We also illustrated the cortical activation to the Classical Chinese story both before and after learning (Fig. 4; Table S2). During pre-learning listening, significant activation was mainly limited to the middle part of the left STG, and a ROI analysis suggested no significant activation in the Heschl’s gyrus (t(59) = −1.63, P = 0.109). During post-learning listening, however, significant activation was observed in more extended cortical areas, including bilateral S/MTG, bilateral M/IFG, and left angular gyrus (AnG). A ROI analysis suggested no significant post-learning activation in the Heschl’s gyrus (t(59) = −0.66, P = 0.509).
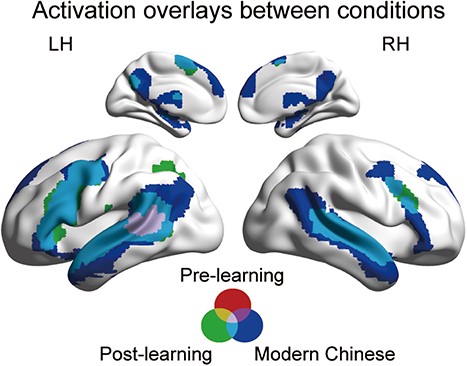
Whole brain activation during modern Chinese story listening, pre-learning Classical Chinese story listening, and post-learning Classical Chinese story listening. Each activation plot was thresholded at voxelwise P < 0.001, FWE-corrected cluster-level P < 0.05.
Next, we tested which cortical areas were significantly affected by learning or method. The main effect of learning was significant in broad cortical areas, including the bilateral STG, left IFG, and left MFG (Fig. 5; Table 2). A post-hoc t-test revealed that these areas were more strongly activated during post-learning listening compared with pre-learning listening (Fig. S1). Significant interaction was observed in the right cerebellum (Table 2). No significant main effects of method were observed.
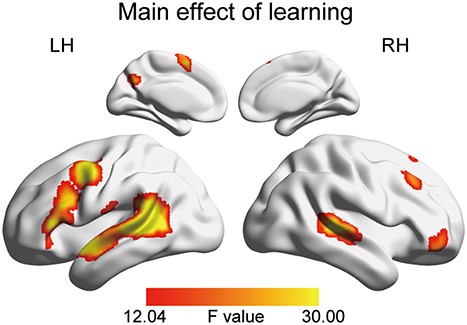
Whole-brain results of the main effect of learning. The activation map was thresholded at voxelwise P < 0.001, FWE-corrected cluster-level P < 0.05.
The whole-brain activation results on the main effect of learning and the interaction between learning and method.
Anatomical label . | Maxima MNI coordinates . | Peak F value . | Cluster PFWE-corr . | Cluster size . | Peak PFWE-corr . | ||
---|---|---|---|---|---|---|---|
x . | y . | z . | |||||
Main effect of learning | |||||||
L MTG | −57 | −48 | 12 | 68.573 | <10−14 | 1127 | 1.23 × 10−6 |
L MTG | −60 | −42 | 6 | 61.172 | 7.15 × 10−6 | ||
L MTG | −48 | −45 | 9 | 59.334 | 1.12 × 10−5 | ||
L PreCG | −39 | 3 | 48 | 46.392 | 2.78 × 10−10 | 276 | 3.44 × 10−4 |
L PreCG | −51 | −3 | 48 | 37.668 | 0.004 | ||
R STG | 54 | −30 | 3 | 41.902 | 2.12 × 10−9 | 243 | 0.001 |
R STG | 69 | −36 | 12 | 16.277 | 0.992 | ||
R ORBmid | 45 | 54 | −3 | 30.586 | 0.002 | 63 | 0.044 |
R ORBmid | 30 | 42 | −9 | 18.071 | 0.934 | ||
R ORBinf | 42 | 45 | −12 | 17.525 | 0.961 | ||
L SMA | −6 | 6 | 72 | 29.656 | 5.01 × 10−5 | 102 | 0.060 |
L SMA | −6 | 9 | 57 | 27.172 | 0.141 | ||
R MFG | 33 | 30 | 51 | 27.049 | 9.89 × 10−5 | 94 | 0.147 |
R MFG | 39 | 30 | 45 | 23.827 | 0.362 | ||
R SFG | 24 | 27 | 60 | 17.324 | 0.968 | ||
R Cerebellum | 30 | −66 | −24 | 26.158 | 5.68 × 10−6 | 129 | 0.199 |
R Cerebellum | 12 | −75 | −21 | 25.246 | 0.253 | ||
R Cerebellum | 18 | −66 | −27 | 24.832 | 0.282 | ||
L Cuneus | −15 | −60 | 30 | 25.062 | 0.001 | 65 | 0.266 |
Interaction | |||||||
R Cerebellum | 9 | −75 | −24 | 15.850 | 5.30 × 10−6 | 112 | 0.176 |
R Cerebellum | 30 | −66 | −24 | 14.819 | 0.331 | ||
Vermis | 3 | −69 | −15 | 10.596 | 0.988 |
Anatomical label . | Maxima MNI coordinates . | Peak F value . | Cluster PFWE-corr . | Cluster size . | Peak PFWE-corr . | ||
---|---|---|---|---|---|---|---|
x . | y . | z . | |||||
Main effect of learning | |||||||
L MTG | −57 | −48 | 12 | 68.573 | <10−14 | 1127 | 1.23 × 10−6 |
L MTG | −60 | −42 | 6 | 61.172 | 7.15 × 10−6 | ||
L MTG | −48 | −45 | 9 | 59.334 | 1.12 × 10−5 | ||
L PreCG | −39 | 3 | 48 | 46.392 | 2.78 × 10−10 | 276 | 3.44 × 10−4 |
L PreCG | −51 | −3 | 48 | 37.668 | 0.004 | ||
R STG | 54 | −30 | 3 | 41.902 | 2.12 × 10−9 | 243 | 0.001 |
R STG | 69 | −36 | 12 | 16.277 | 0.992 | ||
R ORBmid | 45 | 54 | −3 | 30.586 | 0.002 | 63 | 0.044 |
R ORBmid | 30 | 42 | −9 | 18.071 | 0.934 | ||
R ORBinf | 42 | 45 | −12 | 17.525 | 0.961 | ||
L SMA | −6 | 6 | 72 | 29.656 | 5.01 × 10−5 | 102 | 0.060 |
L SMA | −6 | 9 | 57 | 27.172 | 0.141 | ||
R MFG | 33 | 30 | 51 | 27.049 | 9.89 × 10−5 | 94 | 0.147 |
R MFG | 39 | 30 | 45 | 23.827 | 0.362 | ||
R SFG | 24 | 27 | 60 | 17.324 | 0.968 | ||
R Cerebellum | 30 | −66 | −24 | 26.158 | 5.68 × 10−6 | 129 | 0.199 |
R Cerebellum | 12 | −75 | −21 | 25.246 | 0.253 | ||
R Cerebellum | 18 | −66 | −27 | 24.832 | 0.282 | ||
L Cuneus | −15 | −60 | 30 | 25.062 | 0.001 | 65 | 0.266 |
Interaction | |||||||
R Cerebellum | 9 | −75 | −24 | 15.850 | 5.30 × 10−6 | 112 | 0.176 |
R Cerebellum | 30 | −66 | −24 | 14.819 | 0.331 | ||
Vermis | 3 | −69 | −15 | 10.596 | 0.988 |
Note: Areas surviving voxelwise P < 0.001, FWE-corrected cluster-level P < 0.05 in the whole-brain analysis. L, left; R, right; MFG, middle frontal gyrus; MTG, middle temporal gyrus; PreCG, precentral gyrus; SFG, superior frontal gyrus; SMA, supplementary motor area; STG, superior temporal gyrus; ORBinf, inferior frontal gyrus, orbital part; ORBmid, middle frontal gyrus, orbital part. Brain regions are labeled based on the AAL atlas (Tzourio-Mazoyer et al. 2002).
The whole-brain activation results on the main effect of learning and the interaction between learning and method.
Anatomical label . | Maxima MNI coordinates . | Peak F value . | Cluster PFWE-corr . | Cluster size . | Peak PFWE-corr . | ||
---|---|---|---|---|---|---|---|
x . | y . | z . | |||||
Main effect of learning | |||||||
L MTG | −57 | −48 | 12 | 68.573 | <10−14 | 1127 | 1.23 × 10−6 |
L MTG | −60 | −42 | 6 | 61.172 | 7.15 × 10−6 | ||
L MTG | −48 | −45 | 9 | 59.334 | 1.12 × 10−5 | ||
L PreCG | −39 | 3 | 48 | 46.392 | 2.78 × 10−10 | 276 | 3.44 × 10−4 |
L PreCG | −51 | −3 | 48 | 37.668 | 0.004 | ||
R STG | 54 | −30 | 3 | 41.902 | 2.12 × 10−9 | 243 | 0.001 |
R STG | 69 | −36 | 12 | 16.277 | 0.992 | ||
R ORBmid | 45 | 54 | −3 | 30.586 | 0.002 | 63 | 0.044 |
R ORBmid | 30 | 42 | −9 | 18.071 | 0.934 | ||
R ORBinf | 42 | 45 | −12 | 17.525 | 0.961 | ||
L SMA | −6 | 6 | 72 | 29.656 | 5.01 × 10−5 | 102 | 0.060 |
L SMA | −6 | 9 | 57 | 27.172 | 0.141 | ||
R MFG | 33 | 30 | 51 | 27.049 | 9.89 × 10−5 | 94 | 0.147 |
R MFG | 39 | 30 | 45 | 23.827 | 0.362 | ||
R SFG | 24 | 27 | 60 | 17.324 | 0.968 | ||
R Cerebellum | 30 | −66 | −24 | 26.158 | 5.68 × 10−6 | 129 | 0.199 |
R Cerebellum | 12 | −75 | −21 | 25.246 | 0.253 | ||
R Cerebellum | 18 | −66 | −27 | 24.832 | 0.282 | ||
L Cuneus | −15 | −60 | 30 | 25.062 | 0.001 | 65 | 0.266 |
Interaction | |||||||
R Cerebellum | 9 | −75 | −24 | 15.850 | 5.30 × 10−6 | 112 | 0.176 |
R Cerebellum | 30 | −66 | −24 | 14.819 | 0.331 | ||
Vermis | 3 | −69 | −15 | 10.596 | 0.988 |
Anatomical label . | Maxima MNI coordinates . | Peak F value . | Cluster PFWE-corr . | Cluster size . | Peak PFWE-corr . | ||
---|---|---|---|---|---|---|---|
x . | y . | z . | |||||
Main effect of learning | |||||||
L MTG | −57 | −48 | 12 | 68.573 | <10−14 | 1127 | 1.23 × 10−6 |
L MTG | −60 | −42 | 6 | 61.172 | 7.15 × 10−6 | ||
L MTG | −48 | −45 | 9 | 59.334 | 1.12 × 10−5 | ||
L PreCG | −39 | 3 | 48 | 46.392 | 2.78 × 10−10 | 276 | 3.44 × 10−4 |
L PreCG | −51 | −3 | 48 | 37.668 | 0.004 | ||
R STG | 54 | −30 | 3 | 41.902 | 2.12 × 10−9 | 243 | 0.001 |
R STG | 69 | −36 | 12 | 16.277 | 0.992 | ||
R ORBmid | 45 | 54 | −3 | 30.586 | 0.002 | 63 | 0.044 |
R ORBmid | 30 | 42 | −9 | 18.071 | 0.934 | ||
R ORBinf | 42 | 45 | −12 | 17.525 | 0.961 | ||
L SMA | −6 | 6 | 72 | 29.656 | 5.01 × 10−5 | 102 | 0.060 |
L SMA | −6 | 9 | 57 | 27.172 | 0.141 | ||
R MFG | 33 | 30 | 51 | 27.049 | 9.89 × 10−5 | 94 | 0.147 |
R MFG | 39 | 30 | 45 | 23.827 | 0.362 | ||
R SFG | 24 | 27 | 60 | 17.324 | 0.968 | ||
R Cerebellum | 30 | −66 | −24 | 26.158 | 5.68 × 10−6 | 129 | 0.199 |
R Cerebellum | 12 | −75 | −21 | 25.246 | 0.253 | ||
R Cerebellum | 18 | −66 | −27 | 24.832 | 0.282 | ||
L Cuneus | −15 | −60 | 30 | 25.062 | 0.001 | 65 | 0.266 |
Interaction | |||||||
R Cerebellum | 9 | −75 | −24 | 15.850 | 5.30 × 10−6 | 112 | 0.176 |
R Cerebellum | 30 | −66 | −24 | 14.819 | 0.331 | ||
Vermis | 3 | −69 | −15 | 10.596 | 0.988 |
Note: Areas surviving voxelwise P < 0.001, FWE-corrected cluster-level P < 0.05 in the whole-brain analysis. L, left; R, right; MFG, middle frontal gyrus; MTG, middle temporal gyrus; PreCG, precentral gyrus; SFG, superior frontal gyrus; SMA, supplementary motor area; STG, superior temporal gyrus; ORBinf, inferior frontal gyrus, orbital part; ORBmid, middle frontal gyrus, orbital part. Brain regions are labeled based on the AAL atlas (Tzourio-Mazoyer et al. 2002).
fMRI results: brain–behavior correlation
Finally, we analyzed the correlation between cortical activation during post-learning listening to the Classical Chinese story and the performance on speech comprehension tests after regressing out participants’ individual proficiency in Classical Chinese. Significant correlation was observed for the word recognition test, the sentence boundary test, and the passage comprehension test, and the correlation was mainly observed in the left STG, with varying results observed for different tasks (P < 0.05, Bonferroni corrected; Fig. 6). Specifically, post-learning cortical activation exhibited significant correlation with the performance on the word recognition test in the left pSTG and adjacent temporo-parietal junction areas, as well as the posterior medial structures (e.g. precuneus and posterior cingulate gyrus) and the AnG. For the performance on the sentence boundary test, cortical activation showed significant correlation in the left pSTG and the left supplementary motor area (SMA). A significant correlation was found between cortical activation and performance on the passage comprehension test, specifically in the left aSTG. However, no significant correlation was found between cortical activation and the performance on the word meaning test.
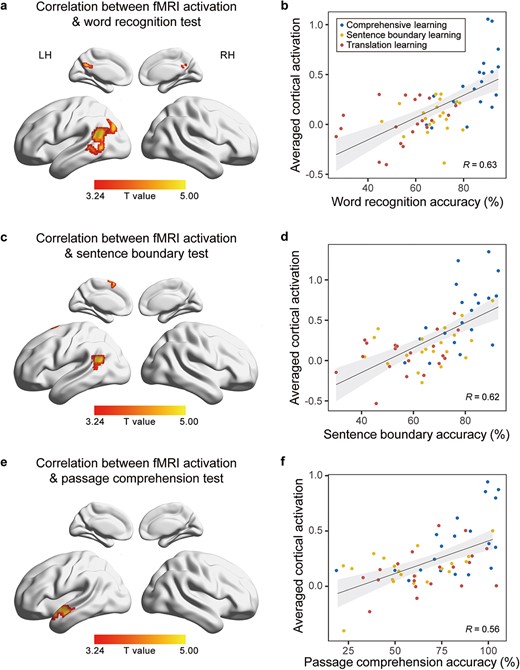
Correlation between fMRI activation and behavioral performance. The left panel shows the whole-brain correlation between fMRI activation and behavioral performance on different tests (voxelwise P < 0.001, FWE-corrected cluster-level P < 0.05, Bonferroni corrected). The right panel shows the scatter plots between individual behavioral performance and the mean fMRI activation in areas significantly correlated with the behavior performance.
Discussion
This study explores the neural substrate underlying semantic and syntactic processing during passage comprehension using a learning paradigm. Our results demonstrate that a 12-min learning session can effectively activate the classic fronto-temporal language network when participants listen to the same passage. We apply three learning methods that provided distinct cues to induce learning, and assess the learning outcomes using behavioral tests. No cortical areas are significantly modulated by the learning method, but neural activation in the temporal lobe can predict learning outcomes, suggesting that learning induced by different cues converges to similar cortical processing mechanisms.
The study compares three learning methods. The comprehensive learning method directly informs participants of the mapping from sound to meaning and is expected to be the most effective learning method. The sentence boundary learning method provides only the boundary between sentences, requiring participants to use this information to infer meaning. As Classical Chinese is highly concise and each sentence in the current passage only has 5.0 syllables on average, knowing sentential boundaries could reduce the difficulty of lexical segmentation. Learning sentence boundaries, referred to as “ju dou,” used to be a key element to learn Classical Chinese, since Classical Chinese is traditionally written without any punctuation marking sentence boundaries. In the ancient time, teachers taught the students to segment sentences by reading the text aloud with exaggerated prosody and some teachers barely explained the text. A proverb goes that “the meaning of a passage will emerge after you read it 100 times”. The translation learning method, in contrast, informs passage meaning by presenting a modern Chinese translation of the story, and the learners have to use passage meaning to guide the interpretation of words and sentences. Behavioral data confirm that comprehensive learning is the most effective learning method, and sentence boundary learning leads to better word recognition performance than the translation learning. Translation learning only leads to better performance on the passage comprehension test compared with the control group, although the answers to the questions are not directly available in the translation. Interestingly, although the three methods induce learning using different cues (Table S3), the current study did not find any cortical area that shows method-dependent neural activation. Furthermore, although the neural activation of some cortical areas is correlated with the learning outcome, the learning method does not further modulate the correlation. These results suggest that similar neural responses are engaged to process a language, regardless of how the language is learned.
The current study aims to elucidate the cortical areas specific to higher-level speech processing beyond the syllable level. By employing a learning paradigm, we avoid the construction of a control stimulus and compare the cortical responses to the same speech sequence before and after learning to localize the higher-level network. This study diverges from previous studies on perceptual learning of connected speech, in which the participants learn to decode phonetic information from degraded speech (Giraud et al. 2004; Eisner et al. 2010). Instead, we investigate how participants learn to decode meaning from a clearly presented syllable sequence. In other words, participants could recognize individual syllables before learning, but after learning, they could recognize words and process higher-level linguistic structures.
The primary effect of learning is observed in the left frontal lobe and bilateral temporal lobe, which are all commonly observed language processing areas. There is no significant activation in the Heschl’s gyrus either before or after learning. In other words, early auditory areas do not show any significant difference between the story condition and the random syllable condition, confirming that these two conditions are perfectly matched in terms of acoustic and phonetic properties. Nevertheless, even before learning, the middle part of the left STG responds more strongly to stories than to the random syllables. The effect may reflect the sensitivity of the left STG to the transitional probability between syllables (Leonard et al. 2015) or that the listeners can decode a small fraction of words or phrases even before learning. Neural activation in the anterior and posterior temporal lobes is correlated with various measures of comprehension tests, whereas neural activation in the frontal lobe does not exhibit correlation with behavior. It is commonly believed the frontal lobe is involved in the parsing of the phrasal structure (Nelson et al. 2017). In the current experiment, the parsing process often relies on syntactic rules that are uncommon or non-existent in the modern Chinese, and thus is too challenging and is rarely successful. Therefore, it is not correlated with the performance of finding sentence boundaries or any other behavioral measures.
Another area that showed robust learning effect was the left SMA (Fig. 5 and Fig. 6c). This area is considered as a fundamental element of the domain-general working-memory system, commonly referred to as the multiple-demand network (MDN). Some researchers argue that language processing is highly domain-specific, and the involvement of the domain-general cognitive systems, such as the MDN, in language processing is very limited (Diachek et al. 2020; Wehbe et al. 2021). Conversely, other researchers have found evidence supporting the involvement of domain-general working memory areas in high-level language processes (e.g. discourse comprehension; Yang et al. 2018; Yang et al. 2023). Our current findings of the involvement of SMA during post-learning listening supports the latter view, which emphasize the role of domain-general working memory in higher-level language processing. Therefore, the finding of the current study has potential implications for an ongoing debate regarding the necessity of domain-general working memory or the MDN in language processing.
Activation of an area in the left posterior temporal lobe is correlated with the performance on the word recognition test. Word recognition test requires the precise sound-to-character mapping, which implicates several stages of comprehension over and above early acoustic processing. A considerable number of prior studies have investigated the cortical network underlying word recognition (Hickok and Poeppel 2007; Price 2012), leading to the hypothesis that the pMTG plays a fundamental role in lexical access (Howard et al. 1992; Lau et al. 2008; Friederici 2012). The current study offers additional support for this hypothesis by demonstrating that activation in this area is correlated with word recognition behavior. The present study differs from previous studies on word recognition in two aspects. First, it employs a naturalistic listening task: participants listen to a story and are not explicitly instructed to recognize words. This approach contrasts with studies that investigate cortical activation in response to isolated words using less natural tasks, such as lexical judgment (Poldrack et al. 1999; Kotz et al. 2002). Second, unlike previous studies comparing normal sentences and jabberwocky (Humphries et al. 2006; Matchin et al. 2017), the current study contrasts two conditions that are perfectly matched in their stimulus properties, including acoustic properties and the transitional probability between syllables.
Correlation between cortical activation and the performance on the word recognition test was also found in areas outside the classic language network. As previously mentioned, a significant correlation was found between post-learning cortical activation and word recognition test scores in the posterior medial structures and AnG (Fig. 6a). Two possible explanations exist. First, these areas are associated with familiarity (Eisner et al. 2010; Davey et al. 2015), suggesting that the effect may be attributed to the transformation of unfamiliar stimuli into familiar stimuli through learning. Second, these areas are also associated with situation model (Martín-Loeches et al. 2012; Acunzo et al. 2022). As the participants gained a better understanding of the Classical Chinese story after learning, it is possible that a situation model was built, resulting in the activation of these areas during post-learning listening.
Activation in the left temporal lobe also correlates with the performance on the sentence boundary test and the passage comprehension test. Both the sentence boundary test and the passage comprehension test require syntactic analysis. Several brain regions are supposed to support syntactic analysis. For example, a considerable body of literature has reported activation in the left IFG and left pSTG during syntactic processing (Friederici et al. 2000; Musso et al. 2003; Pallier et al. 2011; Zaccarella et al. 2015; Murphy et al. 2022), and the left ATL is suggested to play a critical role in building basic phrases (Friederici et al. 2000; Humphries et al. 2006; Rogalsky and Hickok 2009; Bemis and Pylkkanen 2013), and semantic analysis (Pylkkänen 2019). Some studies, however, argue for the unnecessary role of the left IFG and pSTG in syntactic processing (Matchin et al. 2017). Instead, they claim that these areas are involved only in top-down syntactic prediction. Here, we observed a significant correlation between activation in the left pSTG and the performance on the sentence boundary test, while a significant correlation between activation in the left ATL and the performance on the passage comprehension test. The sentence boundary test mainly reflects relatively coarse sentence-level parsing and does not require the participants to reveal the phrasal structure within a sentence. The passage comprehension test, however, requires the integration over multiple sentences. Additionally, it requires semantic processing that is often based on correct parsing of the underlying phrasal structure. The current results are thus consistent with the view that the left posterior temporal lobe is responsible for coarse syntactic analysis (Lopopolo et al. 2021; Murphy et al. 2022), whereas the anterior temporal lobe is concerned with fine-grained combinatorial operations.
We found no significant correlation between the cortical activation and the performance on the word meaning test. The word meaning test asks the participants to determine the precise meaning of a word in the context and requires more fine-grained interpretation than other tests. It is possible that the cortical activation related to such fine-grained interpretation is subtle and distributed, and is not resolved in the current experiment. It is also possible that fine-grained comprehension is not engaged during story listening, and is only engaged when performing the task.
Conclusion
In conclusion, we examined the neural basis of speech comprehension beyond phonetic processing by comparing pre- and post-learning activation in response to identical acoustic stimuli. We found learning effects in the fronto-temporal language network. The brain–behavior correlation suggests a strong relationship between brain activities in the anterior and posterior temporal lobe, along with posterior medial structures and the left SMA, and post-learning comprehension performances across various measures.
Acknowledgments
The authors declare no competing financial interests. Correspondence and requests for materials should be addressed to N.D.
Author contributions
N.D. conceptualized the study and designed the experiment. H.H. implemented the fMRI scanning sequence. J.-J.Z., J.D., Z.S., N.X., Y.C., and J.-F.Z. performed the study. J.W. performed the data analysis and interpretation under the supervision of N.D., X.W., and Y.B. J.W. drafted the manuscript. N.D., X.W., and B.Y. edited the manuscript.
CRediT author statement
Jing Wang (Formal analysis, Investigation, Visualization, Writing—original draft), Xiaosha Wang (Supervision, Writing—review and editing), Jiajie Zou (Data curation), Jipeng Duan (Data curation), Zhuowen Shen (Data curation), Nannan Xu (Data curation), Yan Chen (Data curation), Jianfeng Zhang (Data curation), Hongjian He (Software), Yanchao Bi (Supervision, Writing—review and editing), Nai Ding (Conceptualization, Funding acquisition, Supervision, Writing—review and editing).
Funding
This work was partly supported by the STI2030-Major Project (no. 2021ZD0204105) and Key R&D Program of Zhejiang (no. 2022C03011).
Conflict of interest: The authors declare no competing financial interests.
Data availability
The data that support the findings of this study are available from the corresponding author upon reasonable request.
Matlab scripts for preprocessing and the second-level flexible factorial design are available at https://osf.io/pe3fw/?view_only=07c36d3efbcf45a0a6b022c008ceae32f. Other codes used to collect functional task data and to analyze behavioral and imaging data are available from the corresponding author upon reasonable request.