-
PDF
- Split View
-
Views
-
Cite
Cite
Daniel Alcalá-López, Jonathan Smallwood, Elizabeth Jefferies, Frank Van Overwalle, Kai Vogeley, Rogier B Mars, Bruce I Turetsky, Angela R Laird, Peter T Fox, Simon B Eickhoff, Danilo Bzdok, Computing the Social Brain Connectome Across Systems and States, Cerebral Cortex, Volume 28, Issue 7, July 2018, Pages 2207–2232, https://doi.org/10.1093/cercor/bhx121
- Share Icon Share
Abstract
Social skills probably emerge from the interaction between different neural processing levels. However, social neuroscience is fragmented into highly specialized, rarely cross-referenced topics. The present study attempts a systematic reconciliation by deriving a social brain definition from neural activity meta-analyses on social-cognitive capacities. The social brain was characterized by meta-analytic connectivity modeling evaluating coactivation in task-focused brain states and physiological fluctuations evaluating correlations in task-free brain states. Network clustering proposed a functional segregation into (1) lower sensory, (2) limbic, (3) intermediate, and (4) high associative neural circuits that together mediate various social phenomena. Functional profiling suggested that no brain region or network is exclusively devoted to social processes. Finally, nodes of the putative mirror-neuron system were coherently cross-connected during tasks and more tightly coupled to embodied simulation systems rather than abstract emulation systems. These first steps may help reintegrate the specialized research agendas in the social and affective sciences.
Introduction
The complexity of the relationships between individuals is a defining feature of the human species. Besides early descriptions of a systems-level neuroscientific framework with implications for social mechanisms (Nauta 1971; Damasio et al. 1991), the “social brain hypothesis” proposed that selection pressures from social interaction, rather than from interaction with the physical environment, led to the continuous refinement of human behavior (Humphrey 1978; Byrne and Whiten 1988). Social capacities have likely enabled and catalyzed human cultural evolution, including achievements such as sciences, arts, philosophy, and technology, that surpassed the speed and breadth of biological evolution (Tomasello 1999; Vogeley and Roepstorff 2009). These capacities potentially account for the disproportionate volume and complexity of the primate brain. Recent research demonstrated that brain volume in monkeys and humans correlates with different measures of social complexity, including group size, cooperative behavior, coalition formation, and tactical deception (Dunbar and Shultz 2007; Lebreton et al. 2009; Powell et al. 2010; Lewis et al. 2011; Sallet et al. 2011). An implication of this social brain hypothesis is that it places at a premium on the capacity to solve social problems. Consistent with this view, social skills are an important contribution to well-being. On the one hand, psychiatric disorders often entail deficits in social interaction. On the other hand, exposure to dysfunctional social environments considerably increases the risk of psychiatric disease onset (Cacioppo and Hawkley 2009; Tost and Meyer-Lindenberg 2012). Ultimately, psychiatric illness has a hidden cost, impacting not only on the life of patients, but also affecting their friends, families, and whole communities.
Although the social brain hypothesis embeds social interaction in a neurocognitive context, its underlying brain mechanisms have only received little attention before the 1990s (Cacioppo 2002; Mitchell 2009; Frith and Frith 2010; Schilbach et al. 2013). In the last 2 decades, the discipline of “social neuroscience” has expanded rapidly, with the development of many different specialized topics which focus on stimulus properties important for social cognition, such as face processing or motor-behavior comprehension, as well as more complex higher-order cognition, such as moral reasoning or mental-state attribution. These sensory-driven and higher-level social-affective processes governing everyday life naturally melt into and transition between each other.
In general terms, we argue that the absence of an overarching framework within which to embed social cognition may lead to different research groups suggesting diverging interpretational streams for similar brain correlates (Spreng et al. 2009; Schilbach et al. 2012; Barrett and Satpute 2013). First, the brain correlates underlying autobiographical memory retrieval, self-projection into the future, theorizing about others’ mental content, and spatial navigation have been statistically shown to feature significant topographical overlap (Spreng et al. 2009). Second, neuroimaging studies on empathy have meta-analytically revealed robust recruitment of the “saliency network” (Fan et al. 2011), while the “default-mode network” can also be engaged depending on the type of stimulus material (Lamm et al. 2011). Third, the neural correlates underlying trustworthiness and attractiveness judgments on faces have long been studied in isolation, but turned out to recruit widely overlapping neural circuits as measured by functional neuroimaging (Bzdok et al. 2011, 2012a; Mende-Siedlecki et al. 2013). On the same token, one group of social neuroscientists advocate the primacy of abstract modeling of thoughts in social cognition (e.g., Saxe 2005), while other social neuroscientists instead embrace primacy of embodied simulation of others’ actions (e.g., Iacoboni 2009). Yet, it is still debated whether humans have an analog to the mirror-neuron system (MNS) discovered in nonhuman primates (cf., Keysers and Gazzola 2010). It is reasonable to assume that effective social interaction unfolds by integrating lower-level stimulus properties within a broader social context. We hence conclude that the absence of a coherent component-process account of social cognition is currently hindering forward progress in social neuroscience.
One attempt to move beyond a fragmented view of social neuroscience would be to propose an overarching framework within which we can understand each discrepant perspective. The abundance of neuroimaging data on social processes and the rapid development of pattern-learning technologies make it now possible to investigate the neural correlates most consistently involved in different social-affective experiments in a bottom-up fashion. To this end, we comprehensively summarized previously published quantitative meta-analyses on social-affective phenomena. This set of available brain-imaging studies naturally covered both lower sensory-related and higher abstract processes as well as the neural correlates underlying embodied simulation and abstract emulation of social interaction. The data-derived localization of social brain regions served as functional seeds in subsequent analyses to identify commonalities and differences in brain connectivity among each other and with the rest of the brain. Meta-analytic connectivity modeling (MACM) provided a task-dependent functional measure of connectivity between network nodes by determining the coactivation and codeactivation across thousands of diverse, database-stored neuroimaging studies. Resting-state fluctuations contributed a task-independent functional measure of connectivity between 2 network nodes by determining correlation strength between metabolic fluctuations. We submitted these complimentary ways of assessing functional coupling to network clustering in order to determine neurobiologically meaningful functional groups in the social brain. This analysis strategy allowed us to produce a quantitative definition of the social brain that describes task-overarching properties of the brain systems subserving social interaction. Henceforth, we use the term “social brain atlas” to denote the set of brain regions with consistent neural activity increases during social and affective tasks, without preassuming their implication to be exclusive for or specific to social cognition. The present data-guided characterization of the human social brain atlas was performed from a methodological perspective that avoids preassuming traditional psychological terminology (Barrett 2009; Wager et al. 2015; Bzdok and Schilbach 2016) and from a conceptual perspective of network integration rather than regional specialization (Sporns 2014; Medaglia et al. 2015; Yuste 2015; Bzdok et al. 2016).
Materials and Methods
Deriving a Quantitative Definition of the Social Brain Atlas
There is widely recognized uncertainty about what parts of the brain are topographically most specific for social processes (Brothers 1990; Behrens et al. 2009; Van Overwalle 2009; Meyer-Lindenberg and Tost 2012). In a first step, we therefore computed a data-driven atlas of the brain regions consistently implicated in social-affective processing based on existing quantitative knowledge from published coordinate-based meta-analyses.
The neuroimaging literature was carefully searched for coordinate-based meta-analyses on a variety of cognitive domains related to processing information on human individuals as opposed to the aspects of the physical world. We searched the PubMed database (https://www.ncbi.nlm.nih.gov/pubmed) for quantitative meta-analyses on fMRI (functional magnetic resonance imaging) and PET (positron emission tomography) studies based on combinations of the search terms: “social,” “affective,” “emotional,” “face,” “judgment,” “action observation,” “imitation,” “mirror neuron,” “empathy,” “theory of mind,” “perspective taking,” “fMRI,” and “PET.” Further studies were identified through review articles and reference tracing from the retrieved papers. We considered statistically significant meta-analytic convergence points obtained from either Activation Likelihood Estimation (ALE; Eickhoff et al. 2012), Kernel Density Estimation (Wager et al. 2007), or Signed Differential Mapping (Radua and Mataix-Cols 2009). The inclusion criteria comprised (1) full brain coverage, (2) the absence of pharmacological manipulations, and (3) the absence of brain lesions or known mental disorders. Additionally, meta-analytic studies were only considered if they reported (4) convergence locations of whole-brain group analyses as coordinates according to the standard reference space Talairach/Tournoux or MNI (Montreal Neurological Institute). Exclusion criteria were experiments assessing neural effects in a priori defined regions of interest. Rather than compiling a hand-selected list of target psychological tasks, all published meta-analytic review papers related to any type of social-affective cognition were eligible for inclusion in the present study. This approach avoids biased choices as to the debate whether uniquely social brain regions exist or whether social thought is instantiated by general-purpose cognitive processes (cf., Mitchell 2009; Van Overwalle 2011; Bzdok et al. 2012b). The ensuing heterogeneous set of published meta-analyses covered many psychological tasks ranging from social-reward-related decision-making, over social judgments on facial stimuli, to constructing autobiographical mental scenes. The considered quantitative meta-analyses hence included affective and nonaffective, more sensory lower-level and more associative higher-level, environment-driven and scene-construction-driven, embodiment- and mentalizing-based, as well as motor-simulation-implemented and motor-unrelated social-affective processes. In total, an exhaustive literature search yielded 26 meta-analysis publications with significant convergence from original 25 339 initial foci from 3972 neuroimaging studies in 22 712 participants (Table 1).
Included quantitative meta-analyses on social-affective neuroimaging studies
Meta-analysis . | Category of cognitive processes . | Studies . | Subjects . | Foci . | Scanner . |
---|---|---|---|---|---|
Bartra et al. (2013) NeuroImage | Decision-making; Reward processing; Valuation system | 206 | 3857 | 3933 | fMRI |
Brooks et al. (2012) NeuroImage | Emotional faces | 12 | 217 | 274 | fMRI |
Bzdok et al. (2011) Brain Structure and Function | Face judgment; Trustworthiness; Attractiveness | 16 | 390 | 268 | fMRI |
Bzdok et al. (2012) Brain Structure and Function | Morality; Theory of Mind; Empathy | 107 | 1790 | 2607 | fMRI and PET |
Caspers et al. (2010) NeuroImage | Action observation; Imitation; Mirror neurons | 87 | 1289 | 1933 | fMRI and PET |
Diekhof et al. (2011) NeuroImage | Emotion regulation; cognitive reappraisal | 49 | 818 | 379 | fMRI and PET |
Fan et al. (2011) Neuroscience and Biobehavioral Reviews | Empathy; Emotion | 40 | – | 664 | fMRI |
Fusar-Poli et al. (2009a) Neuroscience Letters | Emotional processing; Face processing; Lateralization | 105 | 1600 | 1785 | fMRI |
Fusar-Poli et al. (2009b) Journal of Psychiatry & Neuroscience | Emotional processing; Face processing | 105 | 1600 | 1785 | fMRI |
Kohn et al. (2014) NeuroImage | Emotion regulation | 23 | 479 | 505 | fMRI and PET |
Kurth et al. (2010) Brain Structure and Function | Emotion; Empathy | 46 | 657 | 120 | fMRI and PET |
Laird et al. (2009) The Journal of Neuroscience | Default-Mode Network | 62 | 840 | 1056 | – |
Lamm et al. (2011) NeuroImage | Empathy for pain | 32 | 168 | 617 | fMRI |
Liu et al. (2011) Neuroscience and Biobehavioral Reviews | Reward valence | 142 | – | 5214 | fMRI |
Mar (2011) Annual Review of Psychology | Theory of mind; Story comprehension | 86 | 1225 | 766 | fMRI and PET |
Mende-Siedlecki et al. (2013) Social Cognitive & Affective Neuroscience | Face evaluation; Attractiveness; Trustworthiness | 28 | 586 | – | fMRI |
Molenberghs et al. (2009) Neuroscience and Biobehavioral Reviews | Imitation; Mirror neurons | 20 | – | – | fMRI |
Qin et al. (2012) Human Brain Mapping | Familiarity | 80 | 1274 | – | fMRI and PET |
Schilbach et al. (2012) PloS one | Emotional processing; Social cognition; Unconstrained cognition | 2082 | – | – | fMRI and PET |
Sescousse et al. (2013) Neuroscience and Biobehavioral Reviews | Reward processing | 87 | 1452 | 1181 | fMRI and PET |
Sevinc and Spreng (2014) PloS One | Moral decision-making; Moral emotions processing | 40 | 772 | 399 | fMRI and PET |
Shi et al. (2013) Frontiers in Human Neuroscience | Implicit emotional face processing | 41 | 830 | 531 | fMRI |
Shkurko (2013) Social Cognitive & Affective Neuroscience | Social categorization; in-group versus out-group | 33 | – | 314 | fMRI |
Spreng et al. (2009) Journal of Cognitive Neuroscience | Autobiographical memory; Prospection; Navigation; Theory of Mind; Default Mode Network | 84 | 1437 | 988 | fMRI and PET |
Stoodley and Schmahmann (2009) NeuroImage | Emotion; Cerebellum | 9 | 149 | 20 | fMRI and PET |
Van Overwalle et al. (2014) NeuroImage | Mirroring; Event mentalizing; Person mentalizing; abstraction | 350 | 1282 | – | fMRI |
Total | 3972 | 22 712 | 25 339 |
Meta-analysis . | Category of cognitive processes . | Studies . | Subjects . | Foci . | Scanner . |
---|---|---|---|---|---|
Bartra et al. (2013) NeuroImage | Decision-making; Reward processing; Valuation system | 206 | 3857 | 3933 | fMRI |
Brooks et al. (2012) NeuroImage | Emotional faces | 12 | 217 | 274 | fMRI |
Bzdok et al. (2011) Brain Structure and Function | Face judgment; Trustworthiness; Attractiveness | 16 | 390 | 268 | fMRI |
Bzdok et al. (2012) Brain Structure and Function | Morality; Theory of Mind; Empathy | 107 | 1790 | 2607 | fMRI and PET |
Caspers et al. (2010) NeuroImage | Action observation; Imitation; Mirror neurons | 87 | 1289 | 1933 | fMRI and PET |
Diekhof et al. (2011) NeuroImage | Emotion regulation; cognitive reappraisal | 49 | 818 | 379 | fMRI and PET |
Fan et al. (2011) Neuroscience and Biobehavioral Reviews | Empathy; Emotion | 40 | – | 664 | fMRI |
Fusar-Poli et al. (2009a) Neuroscience Letters | Emotional processing; Face processing; Lateralization | 105 | 1600 | 1785 | fMRI |
Fusar-Poli et al. (2009b) Journal of Psychiatry & Neuroscience | Emotional processing; Face processing | 105 | 1600 | 1785 | fMRI |
Kohn et al. (2014) NeuroImage | Emotion regulation | 23 | 479 | 505 | fMRI and PET |
Kurth et al. (2010) Brain Structure and Function | Emotion; Empathy | 46 | 657 | 120 | fMRI and PET |
Laird et al. (2009) The Journal of Neuroscience | Default-Mode Network | 62 | 840 | 1056 | – |
Lamm et al. (2011) NeuroImage | Empathy for pain | 32 | 168 | 617 | fMRI |
Liu et al. (2011) Neuroscience and Biobehavioral Reviews | Reward valence | 142 | – | 5214 | fMRI |
Mar (2011) Annual Review of Psychology | Theory of mind; Story comprehension | 86 | 1225 | 766 | fMRI and PET |
Mende-Siedlecki et al. (2013) Social Cognitive & Affective Neuroscience | Face evaluation; Attractiveness; Trustworthiness | 28 | 586 | – | fMRI |
Molenberghs et al. (2009) Neuroscience and Biobehavioral Reviews | Imitation; Mirror neurons | 20 | – | – | fMRI |
Qin et al. (2012) Human Brain Mapping | Familiarity | 80 | 1274 | – | fMRI and PET |
Schilbach et al. (2012) PloS one | Emotional processing; Social cognition; Unconstrained cognition | 2082 | – | – | fMRI and PET |
Sescousse et al. (2013) Neuroscience and Biobehavioral Reviews | Reward processing | 87 | 1452 | 1181 | fMRI and PET |
Sevinc and Spreng (2014) PloS One | Moral decision-making; Moral emotions processing | 40 | 772 | 399 | fMRI and PET |
Shi et al. (2013) Frontiers in Human Neuroscience | Implicit emotional face processing | 41 | 830 | 531 | fMRI |
Shkurko (2013) Social Cognitive & Affective Neuroscience | Social categorization; in-group versus out-group | 33 | – | 314 | fMRI |
Spreng et al. (2009) Journal of Cognitive Neuroscience | Autobiographical memory; Prospection; Navigation; Theory of Mind; Default Mode Network | 84 | 1437 | 988 | fMRI and PET |
Stoodley and Schmahmann (2009) NeuroImage | Emotion; Cerebellum | 9 | 149 | 20 | fMRI and PET |
Van Overwalle et al. (2014) NeuroImage | Mirroring; Event mentalizing; Person mentalizing; abstraction | 350 | 1282 | – | fMRI |
Total | 3972 | 22 712 | 25 339 |
Included quantitative meta-analyses on social-affective neuroimaging studies
Meta-analysis . | Category of cognitive processes . | Studies . | Subjects . | Foci . | Scanner . |
---|---|---|---|---|---|
Bartra et al. (2013) NeuroImage | Decision-making; Reward processing; Valuation system | 206 | 3857 | 3933 | fMRI |
Brooks et al. (2012) NeuroImage | Emotional faces | 12 | 217 | 274 | fMRI |
Bzdok et al. (2011) Brain Structure and Function | Face judgment; Trustworthiness; Attractiveness | 16 | 390 | 268 | fMRI |
Bzdok et al. (2012) Brain Structure and Function | Morality; Theory of Mind; Empathy | 107 | 1790 | 2607 | fMRI and PET |
Caspers et al. (2010) NeuroImage | Action observation; Imitation; Mirror neurons | 87 | 1289 | 1933 | fMRI and PET |
Diekhof et al. (2011) NeuroImage | Emotion regulation; cognitive reappraisal | 49 | 818 | 379 | fMRI and PET |
Fan et al. (2011) Neuroscience and Biobehavioral Reviews | Empathy; Emotion | 40 | – | 664 | fMRI |
Fusar-Poli et al. (2009a) Neuroscience Letters | Emotional processing; Face processing; Lateralization | 105 | 1600 | 1785 | fMRI |
Fusar-Poli et al. (2009b) Journal of Psychiatry & Neuroscience | Emotional processing; Face processing | 105 | 1600 | 1785 | fMRI |
Kohn et al. (2014) NeuroImage | Emotion regulation | 23 | 479 | 505 | fMRI and PET |
Kurth et al. (2010) Brain Structure and Function | Emotion; Empathy | 46 | 657 | 120 | fMRI and PET |
Laird et al. (2009) The Journal of Neuroscience | Default-Mode Network | 62 | 840 | 1056 | – |
Lamm et al. (2011) NeuroImage | Empathy for pain | 32 | 168 | 617 | fMRI |
Liu et al. (2011) Neuroscience and Biobehavioral Reviews | Reward valence | 142 | – | 5214 | fMRI |
Mar (2011) Annual Review of Psychology | Theory of mind; Story comprehension | 86 | 1225 | 766 | fMRI and PET |
Mende-Siedlecki et al. (2013) Social Cognitive & Affective Neuroscience | Face evaluation; Attractiveness; Trustworthiness | 28 | 586 | – | fMRI |
Molenberghs et al. (2009) Neuroscience and Biobehavioral Reviews | Imitation; Mirror neurons | 20 | – | – | fMRI |
Qin et al. (2012) Human Brain Mapping | Familiarity | 80 | 1274 | – | fMRI and PET |
Schilbach et al. (2012) PloS one | Emotional processing; Social cognition; Unconstrained cognition | 2082 | – | – | fMRI and PET |
Sescousse et al. (2013) Neuroscience and Biobehavioral Reviews | Reward processing | 87 | 1452 | 1181 | fMRI and PET |
Sevinc and Spreng (2014) PloS One | Moral decision-making; Moral emotions processing | 40 | 772 | 399 | fMRI and PET |
Shi et al. (2013) Frontiers in Human Neuroscience | Implicit emotional face processing | 41 | 830 | 531 | fMRI |
Shkurko (2013) Social Cognitive & Affective Neuroscience | Social categorization; in-group versus out-group | 33 | – | 314 | fMRI |
Spreng et al. (2009) Journal of Cognitive Neuroscience | Autobiographical memory; Prospection; Navigation; Theory of Mind; Default Mode Network | 84 | 1437 | 988 | fMRI and PET |
Stoodley and Schmahmann (2009) NeuroImage | Emotion; Cerebellum | 9 | 149 | 20 | fMRI and PET |
Van Overwalle et al. (2014) NeuroImage | Mirroring; Event mentalizing; Person mentalizing; abstraction | 350 | 1282 | – | fMRI |
Total | 3972 | 22 712 | 25 339 |
Meta-analysis . | Category of cognitive processes . | Studies . | Subjects . | Foci . | Scanner . |
---|---|---|---|---|---|
Bartra et al. (2013) NeuroImage | Decision-making; Reward processing; Valuation system | 206 | 3857 | 3933 | fMRI |
Brooks et al. (2012) NeuroImage | Emotional faces | 12 | 217 | 274 | fMRI |
Bzdok et al. (2011) Brain Structure and Function | Face judgment; Trustworthiness; Attractiveness | 16 | 390 | 268 | fMRI |
Bzdok et al. (2012) Brain Structure and Function | Morality; Theory of Mind; Empathy | 107 | 1790 | 2607 | fMRI and PET |
Caspers et al. (2010) NeuroImage | Action observation; Imitation; Mirror neurons | 87 | 1289 | 1933 | fMRI and PET |
Diekhof et al. (2011) NeuroImage | Emotion regulation; cognitive reappraisal | 49 | 818 | 379 | fMRI and PET |
Fan et al. (2011) Neuroscience and Biobehavioral Reviews | Empathy; Emotion | 40 | – | 664 | fMRI |
Fusar-Poli et al. (2009a) Neuroscience Letters | Emotional processing; Face processing; Lateralization | 105 | 1600 | 1785 | fMRI |
Fusar-Poli et al. (2009b) Journal of Psychiatry & Neuroscience | Emotional processing; Face processing | 105 | 1600 | 1785 | fMRI |
Kohn et al. (2014) NeuroImage | Emotion regulation | 23 | 479 | 505 | fMRI and PET |
Kurth et al. (2010) Brain Structure and Function | Emotion; Empathy | 46 | 657 | 120 | fMRI and PET |
Laird et al. (2009) The Journal of Neuroscience | Default-Mode Network | 62 | 840 | 1056 | – |
Lamm et al. (2011) NeuroImage | Empathy for pain | 32 | 168 | 617 | fMRI |
Liu et al. (2011) Neuroscience and Biobehavioral Reviews | Reward valence | 142 | – | 5214 | fMRI |
Mar (2011) Annual Review of Psychology | Theory of mind; Story comprehension | 86 | 1225 | 766 | fMRI and PET |
Mende-Siedlecki et al. (2013) Social Cognitive & Affective Neuroscience | Face evaluation; Attractiveness; Trustworthiness | 28 | 586 | – | fMRI |
Molenberghs et al. (2009) Neuroscience and Biobehavioral Reviews | Imitation; Mirror neurons | 20 | – | – | fMRI |
Qin et al. (2012) Human Brain Mapping | Familiarity | 80 | 1274 | – | fMRI and PET |
Schilbach et al. (2012) PloS one | Emotional processing; Social cognition; Unconstrained cognition | 2082 | – | – | fMRI and PET |
Sescousse et al. (2013) Neuroscience and Biobehavioral Reviews | Reward processing | 87 | 1452 | 1181 | fMRI and PET |
Sevinc and Spreng (2014) PloS One | Moral decision-making; Moral emotions processing | 40 | 772 | 399 | fMRI and PET |
Shi et al. (2013) Frontiers in Human Neuroscience | Implicit emotional face processing | 41 | 830 | 531 | fMRI |
Shkurko (2013) Social Cognitive & Affective Neuroscience | Social categorization; in-group versus out-group | 33 | – | 314 | fMRI |
Spreng et al. (2009) Journal of Cognitive Neuroscience | Autobiographical memory; Prospection; Navigation; Theory of Mind; Default Mode Network | 84 | 1437 | 988 | fMRI and PET |
Stoodley and Schmahmann (2009) NeuroImage | Emotion; Cerebellum | 9 | 149 | 20 | fMRI and PET |
Van Overwalle et al. (2014) NeuroImage | Mirroring; Event mentalizing; Person mentalizing; abstraction | 350 | 1282 | – | fMRI |
Total | 3972 | 22 712 | 25 339 |
The significant convergence locations from the collected quantitative meta-analyses were then condensed into a consensus social brain atlas. To this end, we gathered the locations of the activation foci expressed in standardized coordinates from each eligible meta-analysis. We then assigned each significant activation focus to 1 of our 36 candidate zones based exclusively on the topographical distribution of the coordinate points (Fig. 1B). The candidate zones have been defined based on brain areas generally believed to be relevant in social-affective processing according to comprehensive qualitative reviews on the social neuroscience literature (Haxby et al. 2000; Decety and Jackson 2004; Ochsner 2008; Behrens et al. 2009; Stoodley and Schmahmann 2009; Van Overwalle et al. 2013). An experienced neuroanatomist double-checked the assignments of the coordinate points reported in the previous meta-analyses to the candidate zones of the present study. The resulting coordinates constituted the list of 36 locations of interest (Table 2). Please note that the anatomical labels mentioned in the coordinate-based meta-analyses did therefore not have any influence on the present results. Reported foci whose provided anatomical location did not match any of our 36 candidate zones were discarded. Individually within each of these 36 foci pools, a single consensus coordinate was derived from the Euclidean distance across all foci assigned to a same anatomical label. In this way, a comprehensive social brain atlas with 36 consensus locations was derived from existing meta-analysis papers (Fig. 1C).
Macro-anatomical region . | Seed tag . | MNI coordinates . | . | Micro-anatomical region . | ||
---|---|---|---|---|---|---|
x . | y . | z . | . | . | ||
Right inferior frontal gyrus | IFG_R | 48 | 24 | 2 | ![]() | Area 45 (54.5%) and Area 44 (1.5%) |
Left hippocampus | HC_L | −24 | −18 | −17 | CA3 (63%), Subiculum (16.5%), CA2 (12%), and DG (2%) | |
Right hippocampus | HC_R | 25 | −19 | −15 | CA3 (38.5%), Subiculum (27%), CA2 (7%), and DG (4%) | |
Rostral anterior cingulate cortex | rACC | −3 | 41 | 4 | ||
Ventromedial prefrontal cortex | vmPFC | 2 | 45 | −15 | ||
Right amygdala | AM_R | 23 | −3 | −18 | LB (51%), SF (20.5), and CM (8%) | |
Left amygdala | AM_L | −21 | −4 | −18 | LB (57%) and CM (30%) | |
Left nucleus accumbens | NAC_L | −13 | 11 | −8 | ||
Right nucleus accumbens | NAC_R | 11 | 10 | −7 | ||
Left middle temporal gyrus | MTG_L | −56 | −14 | −13 | ||
Precuneus | Prec | −1 | −59 | 41 | ||
Right temporo-parietal junction | TPJ_R | 54 | −55 | 20 | Area PGa (IPL; 70.5%) and Area PGp (IPL; 10.5%) | |
Right middle temporal gyrus | MTG_R | 56 | −10 | −17 | ||
Left temporal pole | TP_L | −48 | 8 | −36 | ||
Right temporal pole | TP_R | 53 | 7 | −26 | ||
Medial frontal pole | FP | 1 | 58 | 10 | Area Fp2 (90.9%) | |
Posterior cingulate cortex | PCC | −1 | −54 | 23 | ||
Dorsomedial prefrontal cortex | dmPFC | −4 | 53 | 31 | ||
Left temporo-parietal junction | TPJ_L | −49 | −61 | 27 | Area PGa (IPL; 98.5%) and Area PGp (IPL; 0.7%) | |
Posterior mid-cingulate cortex | pMCC | |||||
Left middle temporal V5 area | MT/V5_L | −50 | −66 | 5 | ||
Right middle temporal V5 area | MT/V5_R | 50 | −66 | 6 | Area hOc4la (31.5%) and Area hOc5 [MT/V5] (30%) | |
Left fusiform gyrus | FG_L | −42 | −62 | −16 | Area FG4 (54.5%) and Area FG2 (45.5%) | |
Right fusiform gyrus | FG_R | 43 | −57 | −19 | Area FG4 (71%) and Area FG2 (29%) | |
Left posterior superior temporal sulcus | pSTS_L | −56 | −39 | 2 | ||
Right posterior superior temporal gyrus | pSTS_R | 54 | −39 | 0 | ||
Left supplementary motor area | SMA_L | −41 | 6 | 45 | Rostral PMd | |
Left anterior insula | AI_L | −34 | 19 | 0 | ||
Right supramarginal gyrus | SMG_R | 54 | −30 | 38 | Area PFt (IPL; 100%) | |
Right cerebellum | Cereb_R | 28 | −70 | −30 | Lobule VIIa crus I (77.5%) and Lobule VI (22.5%) | |
Left cerebellum | Cereb_L | −21 | −66 | −35 | Lobule VI (55.5%) and Lobule VIIa crus I (43%) | |
Right anterior insula | AI_R | 38 | 18 | −3 | ||
Left supramarginal gyrus | SMG_R | −41 | −41 | 42 | Area PFt (33%), Area hIP2 (23.5%), Area 2 (13%), and Area hIP3 (11%) | |
Right supplementary motor area | SMA_R | 48 | 6 | 35 | Rostral PMd | |
Left inferior frontal gyrus | IFG_L | −45 | 27 | −3 | ||
Anterior mid-cingulate cortex | aMCC | 1 | 25 | 30 |
Macro-anatomical region . | Seed tag . | MNI coordinates . | . | Micro-anatomical region . | ||
---|---|---|---|---|---|---|
x . | y . | z . | . | . | ||
Right inferior frontal gyrus | IFG_R | 48 | 24 | 2 | ![]() | Area 45 (54.5%) and Area 44 (1.5%) |
Left hippocampus | HC_L | −24 | −18 | −17 | CA3 (63%), Subiculum (16.5%), CA2 (12%), and DG (2%) | |
Right hippocampus | HC_R | 25 | −19 | −15 | CA3 (38.5%), Subiculum (27%), CA2 (7%), and DG (4%) | |
Rostral anterior cingulate cortex | rACC | −3 | 41 | 4 | ||
Ventromedial prefrontal cortex | vmPFC | 2 | 45 | −15 | ||
Right amygdala | AM_R | 23 | −3 | −18 | LB (51%), SF (20.5), and CM (8%) | |
Left amygdala | AM_L | −21 | −4 | −18 | LB (57%) and CM (30%) | |
Left nucleus accumbens | NAC_L | −13 | 11 | −8 | ||
Right nucleus accumbens | NAC_R | 11 | 10 | −7 | ||
Left middle temporal gyrus | MTG_L | −56 | −14 | −13 | ||
Precuneus | Prec | −1 | −59 | 41 | ||
Right temporo-parietal junction | TPJ_R | 54 | −55 | 20 | Area PGa (IPL; 70.5%) and Area PGp (IPL; 10.5%) | |
Right middle temporal gyrus | MTG_R | 56 | −10 | −17 | ||
Left temporal pole | TP_L | −48 | 8 | −36 | ||
Right temporal pole | TP_R | 53 | 7 | −26 | ||
Medial frontal pole | FP | 1 | 58 | 10 | Area Fp2 (90.9%) | |
Posterior cingulate cortex | PCC | −1 | −54 | 23 | ||
Dorsomedial prefrontal cortex | dmPFC | −4 | 53 | 31 | ||
Left temporo-parietal junction | TPJ_L | −49 | −61 | 27 | Area PGa (IPL; 98.5%) and Area PGp (IPL; 0.7%) | |
Posterior mid-cingulate cortex | pMCC | |||||
Left middle temporal V5 area | MT/V5_L | −50 | −66 | 5 | ||
Right middle temporal V5 area | MT/V5_R | 50 | −66 | 6 | Area hOc4la (31.5%) and Area hOc5 [MT/V5] (30%) | |
Left fusiform gyrus | FG_L | −42 | −62 | −16 | Area FG4 (54.5%) and Area FG2 (45.5%) | |
Right fusiform gyrus | FG_R | 43 | −57 | −19 | Area FG4 (71%) and Area FG2 (29%) | |
Left posterior superior temporal sulcus | pSTS_L | −56 | −39 | 2 | ||
Right posterior superior temporal gyrus | pSTS_R | 54 | −39 | 0 | ||
Left supplementary motor area | SMA_L | −41 | 6 | 45 | Rostral PMd | |
Left anterior insula | AI_L | −34 | 19 | 0 | ||
Right supramarginal gyrus | SMG_R | 54 | −30 | 38 | Area PFt (IPL; 100%) | |
Right cerebellum | Cereb_R | 28 | −70 | −30 | Lobule VIIa crus I (77.5%) and Lobule VI (22.5%) | |
Left cerebellum | Cereb_L | −21 | −66 | −35 | Lobule VI (55.5%) and Lobule VIIa crus I (43%) | |
Right anterior insula | AI_R | 38 | 18 | −3 | ||
Left supramarginal gyrus | SMG_R | −41 | −41 | 42 | Area PFt (33%), Area hIP2 (23.5%), Area 2 (13%), and Area hIP3 (11%) | |
Right supplementary motor area | SMA_R | 48 | 6 | 35 | Rostral PMd | |
Left inferior frontal gyrus | IFG_L | −45 | 27 | −3 | ||
Anterior mid-cingulate cortex | aMCC | 1 | 25 | 30 |
Cytoarchitectonic assignments were performed based the Jülich atlas using the SPM Anatomy toolbox (Eickhoff et al. 2005). The relation of our seeds to the PMd was derived from a recent connectivity-based parcellation study (Genon et al. 2016).
Macro-anatomical region . | Seed tag . | MNI coordinates . | . | Micro-anatomical region . | ||
---|---|---|---|---|---|---|
x . | y . | z . | . | . | ||
Right inferior frontal gyrus | IFG_R | 48 | 24 | 2 | ![]() | Area 45 (54.5%) and Area 44 (1.5%) |
Left hippocampus | HC_L | −24 | −18 | −17 | CA3 (63%), Subiculum (16.5%), CA2 (12%), and DG (2%) | |
Right hippocampus | HC_R | 25 | −19 | −15 | CA3 (38.5%), Subiculum (27%), CA2 (7%), and DG (4%) | |
Rostral anterior cingulate cortex | rACC | −3 | 41 | 4 | ||
Ventromedial prefrontal cortex | vmPFC | 2 | 45 | −15 | ||
Right amygdala | AM_R | 23 | −3 | −18 | LB (51%), SF (20.5), and CM (8%) | |
Left amygdala | AM_L | −21 | −4 | −18 | LB (57%) and CM (30%) | |
Left nucleus accumbens | NAC_L | −13 | 11 | −8 | ||
Right nucleus accumbens | NAC_R | 11 | 10 | −7 | ||
Left middle temporal gyrus | MTG_L | −56 | −14 | −13 | ||
Precuneus | Prec | −1 | −59 | 41 | ||
Right temporo-parietal junction | TPJ_R | 54 | −55 | 20 | Area PGa (IPL; 70.5%) and Area PGp (IPL; 10.5%) | |
Right middle temporal gyrus | MTG_R | 56 | −10 | −17 | ||
Left temporal pole | TP_L | −48 | 8 | −36 | ||
Right temporal pole | TP_R | 53 | 7 | −26 | ||
Medial frontal pole | FP | 1 | 58 | 10 | Area Fp2 (90.9%) | |
Posterior cingulate cortex | PCC | −1 | −54 | 23 | ||
Dorsomedial prefrontal cortex | dmPFC | −4 | 53 | 31 | ||
Left temporo-parietal junction | TPJ_L | −49 | −61 | 27 | Area PGa (IPL; 98.5%) and Area PGp (IPL; 0.7%) | |
Posterior mid-cingulate cortex | pMCC | |||||
Left middle temporal V5 area | MT/V5_L | −50 | −66 | 5 | ||
Right middle temporal V5 area | MT/V5_R | 50 | −66 | 6 | Area hOc4la (31.5%) and Area hOc5 [MT/V5] (30%) | |
Left fusiform gyrus | FG_L | −42 | −62 | −16 | Area FG4 (54.5%) and Area FG2 (45.5%) | |
Right fusiform gyrus | FG_R | 43 | −57 | −19 | Area FG4 (71%) and Area FG2 (29%) | |
Left posterior superior temporal sulcus | pSTS_L | −56 | −39 | 2 | ||
Right posterior superior temporal gyrus | pSTS_R | 54 | −39 | 0 | ||
Left supplementary motor area | SMA_L | −41 | 6 | 45 | Rostral PMd | |
Left anterior insula | AI_L | −34 | 19 | 0 | ||
Right supramarginal gyrus | SMG_R | 54 | −30 | 38 | Area PFt (IPL; 100%) | |
Right cerebellum | Cereb_R | 28 | −70 | −30 | Lobule VIIa crus I (77.5%) and Lobule VI (22.5%) | |
Left cerebellum | Cereb_L | −21 | −66 | −35 | Lobule VI (55.5%) and Lobule VIIa crus I (43%) | |
Right anterior insula | AI_R | 38 | 18 | −3 | ||
Left supramarginal gyrus | SMG_R | −41 | −41 | 42 | Area PFt (33%), Area hIP2 (23.5%), Area 2 (13%), and Area hIP3 (11%) | |
Right supplementary motor area | SMA_R | 48 | 6 | 35 | Rostral PMd | |
Left inferior frontal gyrus | IFG_L | −45 | 27 | −3 | ||
Anterior mid-cingulate cortex | aMCC | 1 | 25 | 30 |
Macro-anatomical region . | Seed tag . | MNI coordinates . | . | Micro-anatomical region . | ||
---|---|---|---|---|---|---|
x . | y . | z . | . | . | ||
Right inferior frontal gyrus | IFG_R | 48 | 24 | 2 | ![]() | Area 45 (54.5%) and Area 44 (1.5%) |
Left hippocampus | HC_L | −24 | −18 | −17 | CA3 (63%), Subiculum (16.5%), CA2 (12%), and DG (2%) | |
Right hippocampus | HC_R | 25 | −19 | −15 | CA3 (38.5%), Subiculum (27%), CA2 (7%), and DG (4%) | |
Rostral anterior cingulate cortex | rACC | −3 | 41 | 4 | ||
Ventromedial prefrontal cortex | vmPFC | 2 | 45 | −15 | ||
Right amygdala | AM_R | 23 | −3 | −18 | LB (51%), SF (20.5), and CM (8%) | |
Left amygdala | AM_L | −21 | −4 | −18 | LB (57%) and CM (30%) | |
Left nucleus accumbens | NAC_L | −13 | 11 | −8 | ||
Right nucleus accumbens | NAC_R | 11 | 10 | −7 | ||
Left middle temporal gyrus | MTG_L | −56 | −14 | −13 | ||
Precuneus | Prec | −1 | −59 | 41 | ||
Right temporo-parietal junction | TPJ_R | 54 | −55 | 20 | Area PGa (IPL; 70.5%) and Area PGp (IPL; 10.5%) | |
Right middle temporal gyrus | MTG_R | 56 | −10 | −17 | ||
Left temporal pole | TP_L | −48 | 8 | −36 | ||
Right temporal pole | TP_R | 53 | 7 | −26 | ||
Medial frontal pole | FP | 1 | 58 | 10 | Area Fp2 (90.9%) | |
Posterior cingulate cortex | PCC | −1 | −54 | 23 | ||
Dorsomedial prefrontal cortex | dmPFC | −4 | 53 | 31 | ||
Left temporo-parietal junction | TPJ_L | −49 | −61 | 27 | Area PGa (IPL; 98.5%) and Area PGp (IPL; 0.7%) | |
Posterior mid-cingulate cortex | pMCC | |||||
Left middle temporal V5 area | MT/V5_L | −50 | −66 | 5 | ||
Right middle temporal V5 area | MT/V5_R | 50 | −66 | 6 | Area hOc4la (31.5%) and Area hOc5 [MT/V5] (30%) | |
Left fusiform gyrus | FG_L | −42 | −62 | −16 | Area FG4 (54.5%) and Area FG2 (45.5%) | |
Right fusiform gyrus | FG_R | 43 | −57 | −19 | Area FG4 (71%) and Area FG2 (29%) | |
Left posterior superior temporal sulcus | pSTS_L | −56 | −39 | 2 | ||
Right posterior superior temporal gyrus | pSTS_R | 54 | −39 | 0 | ||
Left supplementary motor area | SMA_L | −41 | 6 | 45 | Rostral PMd | |
Left anterior insula | AI_L | −34 | 19 | 0 | ||
Right supramarginal gyrus | SMG_R | 54 | −30 | 38 | Area PFt (IPL; 100%) | |
Right cerebellum | Cereb_R | 28 | −70 | −30 | Lobule VIIa crus I (77.5%) and Lobule VI (22.5%) | |
Left cerebellum | Cereb_L | −21 | −66 | −35 | Lobule VI (55.5%) and Lobule VIIa crus I (43%) | |
Right anterior insula | AI_R | 38 | 18 | −3 | ||
Left supramarginal gyrus | SMG_R | −41 | −41 | 42 | Area PFt (33%), Area hIP2 (23.5%), Area 2 (13%), and Area hIP3 (11%) | |
Right supplementary motor area | SMA_R | 48 | 6 | 35 | Rostral PMd | |
Left inferior frontal gyrus | IFG_L | −45 | 27 | −3 | ||
Anterior mid-cingulate cortex | aMCC | 1 | 25 | 30 |
Cytoarchitectonic assignments were performed based the Jülich atlas using the SPM Anatomy toolbox (Eickhoff et al. 2005). The relation of our seeds to the PMd was derived from a recent connectivity-based parcellation study (Genon et al. 2016).
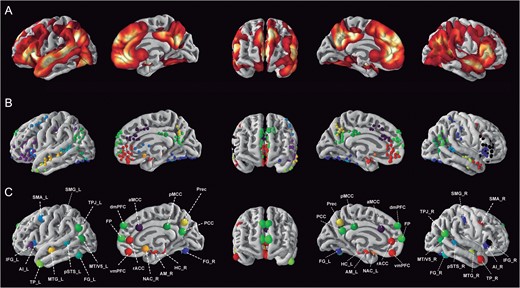
Constructing a quantitative social brain atlas. (A) Probabilistic map of social-affective processing in humans derived from significant convergence foci of previously published neuroimaging meta-analyses (Table 1). (B) Individual locations of significant meta-analytic convergence foci from the previously published meta-analyses. They were color-assigned according to anatomical candidate zones (cf. Methods). (C) Thirty-six consensus seed regions defining the social brain were computed by averaging the locations of all significant foci assigned to a same anatomical term (Table 2). These 36 seeds provided the basis for all presented connectivity analyses. Seeds were surface-rendered for display using PySurfer (http://pysurfer.github.io/). All maps of the social brain atlas are available for display, download, and reuse at the data-sharing platforms ANIMA (http://anima.fz-juelich.de/) or NeuroVault (http://neurovault.org/collections/2462/).
The ensuing consensus locations for regions in the social brain were used to define seed regions with a full 3D shape. To avoid partial volume effects, this growing process was guided by previous neuroanatomical knowledge of local gray-matter densities. Starting from a seed region composed of a single voxel at the consensus coordinate point, new voxels were iteratively added at the borders of the current seed region shape. At each step, the directly neighboring voxels with the highest gray-matter probability according to the ICBM (International Consortium on Brain Mapping) tissue probability maps were added to the 3D shape. At any iteration, all seed voxels were hence direct neighbors without spatial gaps. Therefore, instead of building regular spheres, these compact seed regions were thus successively built until reaching a seed volume of 200 topographically connected voxels. By ensuring a fixed number of gray-matter voxels per seed region definition, we improved the comparability of the MACM and RSFC results by accounting for possible partial volume artifacts. In doing so, the previous 36 consensus coordinates in the social brain were expanded to 36 3D volumes in a neuroanatomically-informed fashion.
In summary, the quantitative fusion of existing coordinate-based meta-analyses allowed us to identify a consensus atlas of 36 core regions involved in social and affective information processing across diverse psychological manipulations. This quintessential definition of the social brain topography served as the basis for all subsequent analysis steps. It is important to appreciate that this set of seeds does “not” represent consistent convergence of neural activity in a given brain region “in general.” Rather, for each region corresponding to one of the a priori anatomical terms (Table 2), we derived a seed “within” this region that reflects the location of most consistent activity increase during social and affective processes. All maps of the social brain atlas are available for display, download, and reuse at the data-sharing platforms ANIMA (http://anima.fz-juelich.de/) or NeuroVault (http://neurovault.org/collections/2462/).
Workflow
The 36 seed regions from the quantitative social brain atlas provided the basis for all subsequent analysis steps. First, MACM was used to determine a whole-brain coactivation map individually for each seed of the social brain atlas. Connectivity in brain states in a task setting were quantified as correlative increase and decrease of neural activity in distant brain regions without conditioning on any specific experimental paradigms. Second, resting-state functional connectivity (RSFC) was used to delineate a whole-brain map of correlated fluctuation for each seed of the social brain atlas. It probed connectivity in task-unconstrained brain states as linear correlation between time series of BOLD signal fluctuations in the absence of any experimental context. Hierarchical clustering automatically delineated functional groups of similar connectivity among the social brain seeds. Third, the functional profile of every seed was determined by testing for relevant overrepresentation of both social and nonsocial taxonomic categories in the BrainMap database, which describe psychological and experimental properties of each stored neuroimaging study. The combination of these steps incorporated a data-guided framework for the comprehensive description of the task-constrained connectivity, task-unconstrained connectivity, and functional associations of the human social brain. It is crucial to appreciate that this study did not set out by presupposing yes-no assignments of brain locations to be either “social” or not. Instead, (1) the exact locations and (2) the degree of functional specificity for social-affective processing were both determined as part of the present quantitative investigations.
Task-Constrained Connectivity: MACM
Delineation of whole-brain coactivation maps for each seed of the social brain atlas was first performed based on the BrainMap database (www.brainmap.org; Fox and Lancaster 2002; Laird et al. 2011). The aim of the coactivation analysis was to perform inference on the spatial convergence of neural activity across all foci of all BrainMap experiments in which the seed in question is reported as active. In the first step, we identified all experiments in the BrainMap database that featured at least one focus of activation in a particular seed. We constrained our analysis to fMRI and PET experiments from conventional mapping (no interventions, no group comparisons) in healthy participants, which reported results as coordinates in stereotaxic space. These inclusion criteria yielded ~7500 eligible experiments at the time of analysis (queried in October 2015). Note that we considered all eligible BrainMap experiments because any preselection based on taxonomic categories would have constituted a strong a priori hypothesis about how brain networks are organized. However, it remains elusive how well psychological constructs, such as emotion and cognition, map on regional brain responses (Mesulam 1998; Poldrack 2006; Laird et al. 2009a).
These brain-wide coactivation patterns for each seed were computed by ALE meta-analysis on all BrainMap experiments associated with a given seed. The key idea behind ALE is to treat the foci reported in the associated experiments not as single points, but as centers for 3D Gaussian probability distributions that reflect the spatial uncertainty associated with neuroimaging results. Using the latest ALE implementation (Eickhoff et al. 2009, 2012; Turkeltaub et al. 2012), the spatial extent of those Gaussian probability distributions was based on empirical estimates of between-participant and between-template variance of neuroimaging foci (Eickhoff et al. 2009). For each experiment, the probability distributions of all reported foci were then combined into a modeled activation (MA) map by the recently introduced “nonadditive” approach that prevents local summation effects (Turkeltaub et al. 2012). The voxel-wise union across the MA maps of all experiments associated with the current seed region then yielded an ALE score for each voxel of the brain that describes the coactivation probability of that particular location with the current seed region.
To establish which brain regions were significantly coactivated with a given seed, ALE scores for the MACM analysis of this seed were compared against a null-distribution that reflects a random spatial association between experiments with a fixed within-experiment distribution of foci (Eickhoff et al. 2009). This random-effects inference assesses above-chance convergence across experiments, not clustering of foci within a particular experiment. The observed ALE scores from the actual meta-analysis of experiments activating within a particular seed were then tested against ALE scores obtained under a null-distribution of random spatial association yielding a P-value based on the proportion of equal or higher random values (Eickhoff et al. 2012). The resulting nonparametric P-values were transformed into z-scores and thresholded at a cluster-level corrected threshold of P < 0.05 after applying a cluster-forming threshold of voxel-level P < 0.001 (Eickhoff et al. 2016). While caution has been raised against performing cluster-level inference in single fMRI experiments (Eklund et al. 2016), with false positives more frequently arising in the posteromedial cortex (Eklund et al. 2016), this significance testing procedure was found beneficial for quantitative meta-analysis experiments based on the ALE algorithm in a recent systematic evaluation (Eickhoff et al. 2016).
Task-Unconstrained Connectivity: RSFCs
For cross-validation across disparate brain states, significant clusters-wise whole-brain connectivity was also assessed using resting-state correlations as an independent modality of functional connectivity. RSFC fMRI images were obtained from the Nathan Kline Institute Rockland–sample, which are available online as part of the International Neuroimaging Datasharing Initiative (http://fcon_1000.projects.nitrc.org/indi/pro/nki.html). In total, the processed sample consisted of 132 healthy participants between 18 and 85 years (mean age: 42.3 ± 18.08 years; 78 male, 54 female) with 260 echo-planar imaging (EPI) images per participant. Images were acquired on a Siemens TrioTim 3 T scanner using blood-oxygen-level-dependent (BOLD) contrast [gradient-echo EPI pulse sequence, repetition time (TR) = 2.5 s, echo time (TE) = 30 ms, flip angle = 80°, in-plane resolution = 3.0 × 3.0 mm, 38 axial slices (3.0 mm thickness), covering the entire brain]. The first 4 scans served as dummy images allowing for magnetic field saturation and were discarded prior to further processing using SPM8 (www.fil.ion.ucl.ac.uk/spm). The remaining EPI images were then first corrected for head movement by affine registration using a two-pass procedure by initially realigning all brain scans to the first image and subsequently to the mean of the realigned images (Corradi-Dell’Acqua et al. 2011; Hamilton et al. 2011; Hurlemann et al. 2010). The mean EPI image for each participant was spatially normalized to the MNI single-subject template (Holmes et al. 1998) using the “unified segmentation” approach (Ashburner and Friston 2005). The ensuing deformation was then applied to the individual EPI volumes. Finally, images were smoothed by a 5-mm FWHM Gaussian kernel to improve signal-to-noise ratio and account for residual anatomical variations.
The time-series data of each voxel of a given seed were processed as follows (Fox et al. 2009; Weissenbacher et al. 2009): In order to reduce spurious correlations, variance that could be explained by the following nuisance variables was removed: (1) The 6 motion parameters derived from the image realignment, (2) the first derivative of the realignment parameters, and (3) mean gray matter, white matter, and CSF signal per time point as obtained by averaging across voxels attributed to the respective tissue class in the SPM 8 segmentation (Reetz et al. 2012). All of these nuisance variables entered the model as first- and second-order terms (Jakobs et al. 2012). Data were then band-pass filtered preserving frequencies between 0.01 and 0.08 Hz since meaningful resting-state correlations will predominantly be found in these frequencies given that the BOLD-response acts as a low-pass filter (Biswal et al. 1995; Fox and Raichle 2007).
To measure task-independent connectivity for each seed of the social brain atlas, time courses were extracted for all gray-matter voxels composing a given seed. The overall seed time-course was then expressed as the first eigenvariate of these voxels’ time courses. Pearson correlation coefficients between the time series of the seeds and all other gray-matter voxels in the brain were computed to quantify its resting-state fluctuation pattern. These voxel-wise correlation coefficients were then transformed into Fisher’s Z-scores and tested for consistency across participants using a random-effects, repeated-measures analysis of variance. The main effect of connectivity for individual clusters and contrasts between those were tested using the standard SPM8 implementations with the appropriate nonsphericity correction. The results of these random-effects analyses were cluster-level thresholded at P < 0.05 (cluster-forming threshold at voxel-level: P < 0.001), analogous to significance correction for the MACM analysis above.
Hierarchical Clustering Analysis
To identify the coherent functional groups in the social brain connectivity patterns, we used hierarchical clustering analysis (Thirion et al. 2014; Eickhoff et al. 2015). Instead of issuing only one solution based on a hand-selected number of k clusters, hierarchical clustering algorithms naturally yield a full partition tree from single-element clusters up to the coarsest two-cluster solution. This agglomerative bottom-up approach revealed connectional similarities with increasing coarseness levels. The implementation was taken from the scipy Python package using single linkage algorithm and Bray-Curtis distance metric (http://docs.scipy.org/doc/scipy/reference/cluster.hierarchy.html). Each individual seed initially represented a separate cluster. These were then progressively merged into a hierarchy by always combining the 2 most similar clusters at each step. To achieve a synoptic view of the seed-seed relationships (Fig. 3), we computed a consensus hierarchical clustering averaged across the MACM and RSFC connectivity metrics. On a methodological note, the hierarchical clustering results did not alter how the connectivity or functional profiling analyses of the social brain seeds were performed.
Intranetwork and Extranetwork Connectivity
For the task-constrained and task-unconstrained functional imaging modalities (i.e., MACM and RSFC), 36 whole-brain connectivity maps have been obtained by computing the statistically significant coupling patterns based on every seed region. The seed-specific connectivity maps derived from either MACM or RSFC modalities were then submitted to 2 complementary subanalyses.
“Intranetwork” analyses compared seed regions based on the functional connectivity within the social brain atlas, whereas “extranetwork” analyses compared seed regions based on the functional connectivity between the social brain seeds and the rest of the brain. (1) In the intranetwork analysis, the whole-brain connectivity maps of each seed were used to quantify the connectivity strength between the seed regions themselves. The 36 regions from the social brain atlas were thus considered as seeds and targets. For correlation across seeds, the variables hence corresponded to how strongly each seed was connected to every of the 35 remaining seeds in the atlas. (2) In the extranetwork analysis, the whole-brain connectivity maps of each seed were used to quantify how strongly each seed region was connected to the remaining parts of the brain. Here, the 36 regions from the social brain atlas acted only as seeds (not as targets). The variables to be correlated thus corresponded to how strongly each seed was connected to the gray-matter voxels in the rest of the brain. The ensuing summary statistic therefore provided a notion of “connectivity congruency” that quantified how similar seed pairs were functionally coupled within the social brain (intranetwork analysis) or with the rest of the brain (extranetwork analysis). Note that we use “functional coupling” as a synonym of “statistical dependency”. Nevertheless, it has been shown that alternative explanations can account for changes in functional connectivity such as common input to a seed and a target regions (Friston 2011).
Functional Profiling
Finally, the social brain seeds were individually submitted to an analysis of their functional profiles by forward and reverse inference. It is important to note that this functional characterization constitutes a post hoc procedure that is subsequent to and independent of the connectivity analyses. The functional characterization was based on 2 types of BrainMap meta-data that describe experimental properties of each database-stored neuroimaging study. “Behavioral domains” code the mental processes isolated by the statistical contrasts and comprise the main categories of cognition, action, perception, emotion, and interoception, as well as their related subcategories. “Paradigm classes” categorize the specific task employed (see http://brainmap.org/scribe/ for the complete BrainMap taxonomy). For the sake of statistical robustness, we excluded all cognitive categories with less than 50 experiments in the BrainMap database. “Forward inference” on the functional characterization tested the probability of observing activity in a social brain seed given previous knowledge of a psychological process. Using forward inference, a seed’s functional profile was determined by identifying taxonomic labels for which the likelihood of finding activation in the respective seed was significantly higher than the a priori chance (across the entire database) of finding activation in that particular cluster. In contrast, “reverse inference” tested the likelihood of a specific psychological process being present given previous knowledge of brain activation in a certain social brain seed. Thus, this second functional profiling of the seed regions allowed us to infer a seed’s functional profile by identifying the behavioral domains and paradigm classes given activation in that particular seed region. In summary, forward inference assessed the likelihood of observing neural activity given a psychological term across 2 established description systems of mental operations, while reverse inference assessed the likelihood of engaging a psychological process given a brain activity pattern based on the same 2 descriptions systems of mental operations. Reverse inference has however repeatedly been argued to be challenging to draw in certain neuroimaging analysis settings (Poldrack 2006; Yarkoni et al. 2011; Wager et al. 2016).
Results
This study attempts a comprehensive characterization of the “social brain” as it can be experimentally probed and quantitatively measured using common brain-imaging techniques. For 36 regions in the social brain, we computed the exact location of highest topographical consistency for social-affective processes from existing meta-analyses (Fig. 1; Tables 1 and 2). This step was motivated by recent connectivity-based parcellation studies showing many target regions in the present study to be decomposable into functionally distinct subregions, such as the amygdala (Saygin et al. 2011), prefrontal cortex (Sallet et al. 2013), cingulate cortex (Beckmann et al. 2009), or insula (Cauda et al. 2012). Please note that, among all 36 seed locations, the posterior medial orbitofrontal cortex is probably most susceptible to signal dropout (Glover and Law 2001; Deichmann et al. 2003), which may have disadvantageously influenced our meta-analytic results on this part of the brain. This is because the BOLD signal acquisition in the orbitofrontal region is affected by magnetic field gradients generated by the proximity of air-tissue interfaces (Deichmann et al. 2002; Wilson et al. 2002). Different methods have been introduced to reduce the susceptibility to this effect and increase signal recovery (e.g., Turner et al. 1990; Merboldt et al. 2001). However, the present meta-analytic study could not control that the original databased studies included in our functional connectivity analyses accounted for this effect. To elucidate the functional network stratification within the social brain, the 36 derived seed regions were used to delineate the whole-brain connectivity based on task-dependent coactivations (MACM) and task-unconstrained time-series correlations (RSFC) (Fig. 2). In a subanalysis, the connectivity architecture of the social seeds was then evaluated with emphasis on the social brain (intranetwork connectivity) or taking into account the entire brain (extranetwork connectivity). Finally, all social brain seeds were automatically linked to their quantitative functional engagements across psychological tasks. The present study is therefore objectively reproducible and did not itself impose subjective limitations to any subset of social-affective processes. The present results, however, bear unavoidable dependence on the research trends in the neuroimaging community, the technical limitations of fMRI technology (e.g., signal dropout), and the restriction to psychological experiments that are feasible within brain scanners.
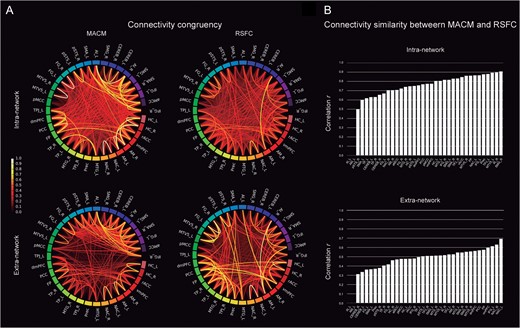
Task and rest connectivity of the social brain. (A) The circle plots depict the “congruency” among the connectivity patterns of any given pair of seed regions in the task-dependent (MACM; “left column”) and task-independent (RSFC; “right column”) brain states when taking into account only the social seed regions (intranetwork analysis; “upper row”) or the entire brain (extranetwork analysis; “lower row”). The color scale of the lines represents the shared connectional architecture from the lesser (“red”) to the greater degree of topographical overlap (“yellow”). (B) “Similarity” between the whole-brain connectivity maps of each individual seed between both MACM and RSFC analyses. The seed regions are ranked in increasing order of task-rest correspondence. The order varies accordingly in the intra- and extranetwork subanalyses. The seeds exhibit more similar connectivity between seeds of the social brain rather between seeds and the rest of the brain. For abbreviations see Table 1.
Hierarchical Clustering Analysis
The hierarchical clustering of the social brain seeds based on their functional connectivity profiles from MACM and RSFC provided evidence for a division of the social brain into 4 principal systems (Fig. 3; for seed abbreviations see Table 2): (1) A set of “visual-sensory seeds” was composed of the FG, pSTS, and MT/V5 from the left and right hemispheres. (2) A set of “limbic” seeds analogous to (3) and (4) consisted of the AM, HC, and NAC from both hemispheres, as well as the rACC and vmPFC (but not medial FP or dmPFC), also yielded a connectionally coherent assembly. (3) A set of “intermediate-level seeds” was composed of the aMCC and bilateral AI, IFG, SMG, SMA, and Cereb. (4) A set of “higher-level seeds” was composed of brain regions that all belonged to the association cortices, including dmPFC (but not vmPFC), medial FP, PCC and Prec, as well as bilateral TPJ, MTG, and TP. While segregation into these 4 main functional systems was most prominent, the consensus hierarchical clustering (Fig. 3) naturally exposed alternative finer-grained clustering solutions that successively decompose the 4 main systems into their constituent subsystems. Note that the chosen nomenclature of visual-sensory/limbic/intermediate/higher-level clusters only reflects a topographical approximation to the facilitate reporting of the results, rather than a subjective judgment on the functional implications of the cluster seeds (cf., below for functional profiling analysis).
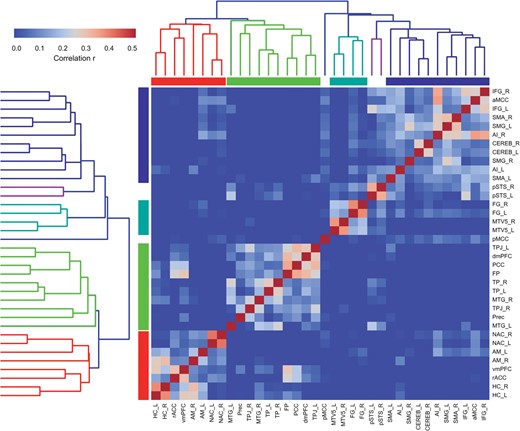
Functional networks in the social brain. We computed a consensus hierarchical clustering across the 2 functional connectivity analyses measuring task-constrained coactivations (MACM) and task-free activity fluctuations (RSFC). Seed regions automatically grouping into a same cluster agree in connectivity across the 2 different brain states. Four major clusters of connectionally coherent social brain regions emerged. These were situated in (from lower-left to upper-right): (1) limbic, (2) higher-level, (3) visual-sensory, and (4) intermediate subnetworks. For abbreviations see Table 1.
We performed clustering subanalyses that individually considered each of 4 different scenarios: (1) task-dependent versus (2) resting-state connectivity, and the connections to (3) the social brain exclusively (intranetwork analysis) versus (4) the whole-brain (extranetwork analysis). Comparing the cluster configurations emerging from MACM and RSFC within the social brain, the bilateral pSTS and left FG seeds joined the intermediate-level cluster composed of the IFG, SMG, SMA, and the Cereb in MACM. Based on RSFC, however, the pSTS, FG, and MT/V5 seeds remained clearly differentiated from the rest of the social brain. Additionally, the bilateral NAC and left AM seeds were more functionally related to this same intermediate-level cluster in RSFC than with the limbic cluster that we found in the consensus analysis.
In a series of subanalyses to test the robustness of the results, we performed 100 split-half procedures of the clustering approach based on connectivity data. In MACM and in RSFC, we observed essentially identical clustering solutions to emerge from the separate data splits. This corroborates the suitability of the obtained clustering solution across perturbations of the input data.
Relation Between Higher-Level and Lower-Level Regions
We adopted a biologically grounded notion of neural processing hierarchy. It emphasizes axon connections of neuron-neuron chains that relay information between the lowest-level photoreceptor cells in the retina or auditory hair-cell receptors in the inner ear, and the highest-level association cortex without any direct connections to sensory areas (Pandya and Kuypers 1969; Jones and Powell 1970; Van Essen et al. 1992; Mesulam 1998). “Lower-level” regions are few synaptic switches away from sensory receptors, whereas what we call “higher-level” regions are most relaying neurons away from areas that process incoming information from the external environment.
Regions from the lower-level, “visual-sensory cluster” (Fig. 4) included the FG, pSTS, and MT/V5. The intranetwork RSFC analysis showed more coherent connectivity among these seeds than the MACM-based counterpart. The FG and pSTS seeds showed significant functional connectivity to the SMA and AI across MACM and RSFC, as well as to the SMG in MACM. Both FG and pSTS showed functional connectivity to the AM. The MT/V5 seed featured significant connectivity to SMG across MACM and RSFC, as well as to SMA in MACM and MTG in RSFC.
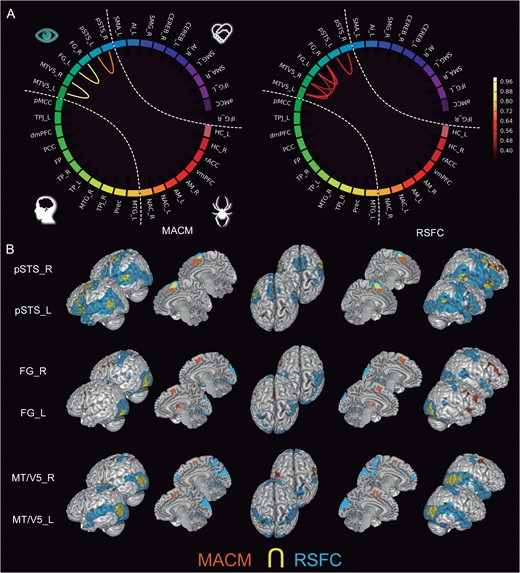
Connectivity of the visual-sensory subnetwork. (A) The circle plots visualize the “congruency” in the connectivity patterns of each pair of seeds across diverse experimental tasks (MACM; “left circle”) and fluctuations across time (RSFC; “right circle”). It shows the intranetwork characterization comparing to what extend seeds are identically connected within the social brain. (B) The task-dependent (“orange”) and task-free (“blue”) connectivity maps of each seed as well as their spatial overlap (“yellow”) are displayed separately on the left, left-midline, superior, right-midline, and right surface views of a T1-weighted MNI single-subject template rendered using Mango (multi-image analysis GUI; http://ric.uthscsa.edu/mango/). All results are cluster-level corrected for multiple comparisons. For abbreviations see Table 1.
In the “limbic cluster” (Fig. 5), the AM seeds exhibited task-dependent coactivation with the hierarchically higher regions dmPFC, IFG, and AI. Further, the HC in the left and right hemispheres were connected to a large set of higher-level regions including the FP, PCC, and TPJ in both MACM and RSFC analyses, as well as to the AI in MACM and to the aMCC in RSFC. The vmPFC seed showed strong connectivity to most regions of the higher-level cluster according to MACM and RSFC, including the FP, dmPFC, PCC, TPJ, and MTG. The NAC seeds yielded connectivity to the vmPFC, AI, and SMA across MACM and RSFC, as well as to the rACC and aMCC at rest.
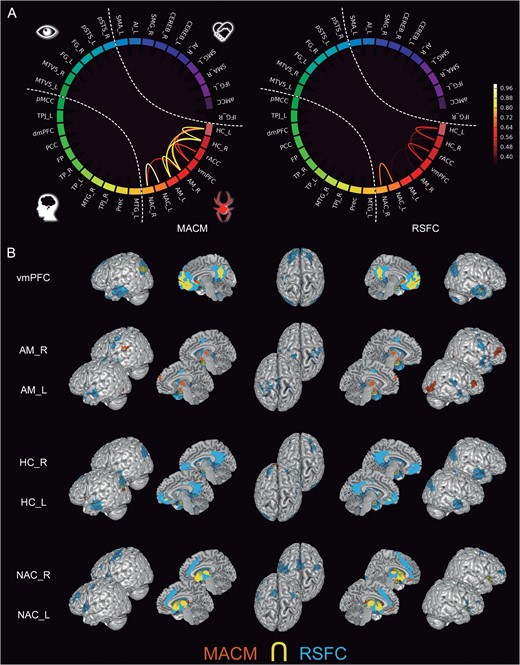
Connectivity of the limbic subnetwork. (A) The circle plots visualize the “congruency” in the connectivity patterns of each pair of seeds across diverse experimental tasks (MACM; “left circle”) and fluctuations across time (RSFC; “right circle”). It shows the intranetwork characterization comparing to what extend seeds are identically connected within the social brain. (B) The task-dependent (“orange”) and task-free (“blue”) connectivity maps of each seed as well as their spatial overlap (“yellow”) are displayed separately on the left, left-midline, superior, right-midline, and right surface views of a T1-weighted MNI single-subject template rendered using Mango (multi-image analysis GUI; http://ric.uthscsa.edu/mango/). All results are cluster-level corrected for multiple comparisons. For the abbreviations see Table 1.
The regions from the “intermediate-level cluster” (Fig. 6) included, among others, the aMCC and bilateral AI. These seeds yielded significant functional connectivity to bilateral SMG across MACM and RSFC, while the aMCC showed resting-state correlations with bilateral dlPFC. The intermediate-level cluster also included the bilateral IFG, SMA, SMG, and pSTS. While the bilateral IFG and SMA seeds showed strong connectivity between each other according to both MACM and RSFC, the left and right SMA seeds were strongly connected to the FG in both connectivity modalities. Seeding from the SMG, we found connectivity targets in the limbic cluster across MACM and RSFC, as well as resting-state correlations with aMCC. Interestingly, the pSTS in this cluster showed a distributed connectivity pattern with the higher-level IFG and SMA bilaterally in MACM and RSFC, as well as with the lower-level regions FG and MT/V5 in MACM and the higher-level dmPFC in RSFC and MTG in MACM.
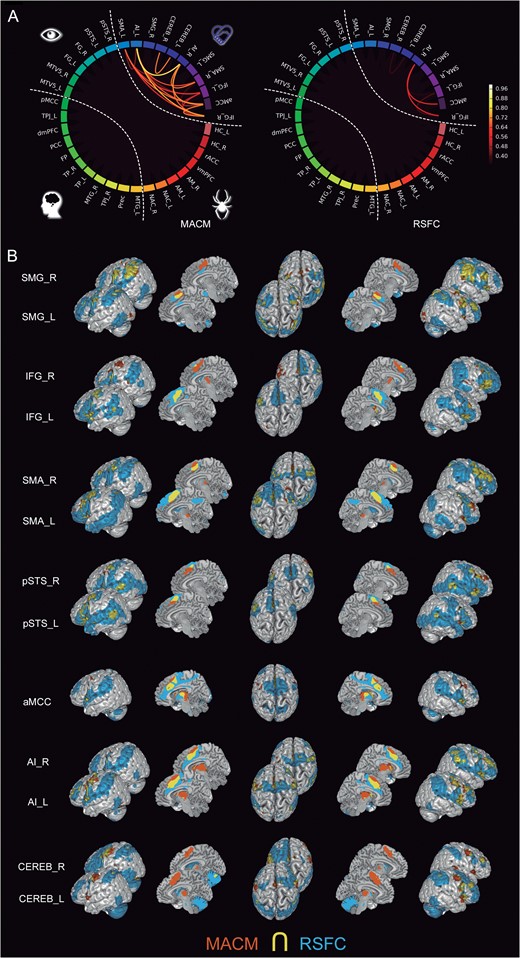
Functional connectivity of the intermediate-level subnetwork. (A) The circle plots visualize the “congruency” in the connectivity patterns of each pair of seeds across diverse experimental tasks (MACM; “left circle”) and fluctuations across time (RSFC; “right circle”). It shows the intranetwork characterization comparing to what extend seeds are identically connected within the social brain. (B) The task-dependent (“orange”) and task-free (“blue”) connectivity maps of seed as well as their spatial overlap (“yellow”) are displayed separately on the left, left-midline, superior, right-midline, and right surface views of a T1-weighted MNI single-subject template rendered using Mango (multi-image analysis GUI; http://ric.uthscsa.edu/mango/). All results are cluster-level corrected for multiple comparisons. For abbreviations see Table 1.
The regions from the “higher-level cluster” (Fig. 7) included the dmPFC, FP, PCC, TPJ, MTG, Prec, and TP, which clustered robustly based on their connectivity patterns in task-structured (MACM) and unstructured (RSFC) brain states. These higher-level seeds were more strongly connected among each other than to any lower- or intermediate-level seeds. Still, we found functional connectivity between these higher-level seeds and other intermediate- or lower-level regions. Specifically, the dmPFC and medial FP seeds were connected to the bilateral IFG across MACM and RSFC. The PCC seed was also connected to the IFG in MACM. The left TPJ seed showed connectivity to the IFG and SMA across MACM and RSFC. Instead, the right TPJ seed yielded task-dependent connectivity to the AI as well as resting-state correlations with SMA and IFG. All these seed regions showed resting-state correlations with the Cereb. The MTG and TP seeds yielded functional connectivity patterns with the vmPFC and IFG across MACM and RSFC. The dmPFC and left TPJ seeds coactivated with the pSTS in MACM. The FP and PCC seeds were functionally connected to the HC (in MACM for the FP seed and RSFC for the PCC seed). The TP seed in the left hemisphere showed task-dependent coactivations with the pSTS and MT/V5, while the right TP seed yielded functional connectivity to the HC in MACM. Both MTG seeds were functionally connected to the pSTS across MACM and RSFC, but only the MTG seed in the right hemisphere showed functional connectivity to the HC across MACM and RSFC.
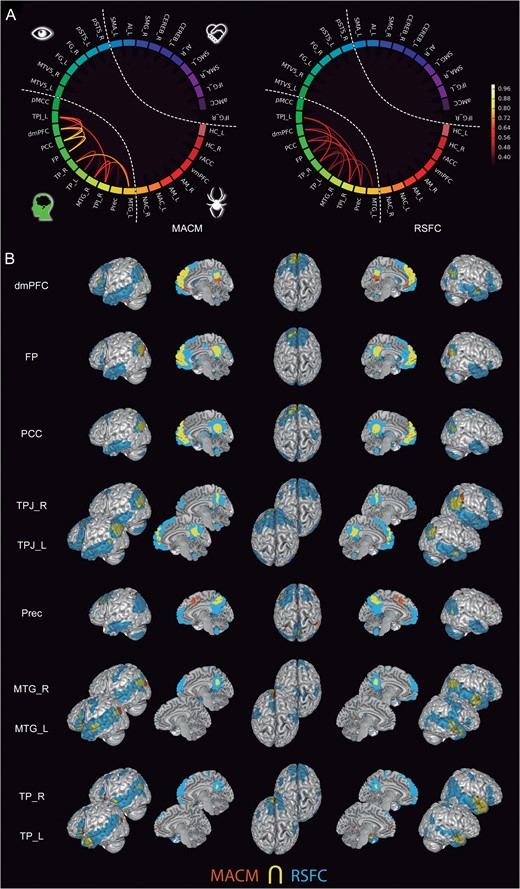
Connectivity of the high-level subnetwork. (A) The circle plots visualize the “congruency” in the connectivity patterns of each pair from the most associative seeds across diverse experimental tasks (MACM; “left circle”) and fluctuations across time (RSFC; “right circle”). It shows the intranetwork characterization comparing to what extend seeds are identically connected within the social brain. (B) The task-dependent (“orange”) and task-free (“blue”) connectivity maps of seed as well as their spatial overlap (“yellow”) are displayed separately on the left, left-midline, superior, right-midline, and right surface views of a T1-weighted MNI single-subject template rendered using Mango (multi-image analysis GUI; http://ric.uthscsa.edu/mango/). All results are cluster-level corrected for multiple comparisons. For abbreviations see Table 1.
In summary, we found networked configurations along different levels of the natural processing hierarchy. These connectivity analyses detailed how higher- and intermediate-level neural processing intertwines with lower-level regions, such as the AM, FG, and pSTS that preprocess social-affective environmental inputs. These functional relationships between the coherent brain networks provide quantitative links between major topics in the social and affective neurosciences.
Lateralization
Hemispheric asymmetries were more often observed in task-constrained brain states than at rest (Fig. 8). Most lateralization effects were found in the high-level, limbic, and sensory-visual clusters and were directed towards the left hemisphere (Fig. 8A). The higher-level cluster displayed mostly bilateral connections among each other and to regions outside of the social brain atlas. However, we found a task-dependent, left-favored lateralization in some of these seeds. Coactivations were found between the medial FP seed and the left HC, the PCC seed and the left MTG, the left TPJ seed and the left pSTS, as well as the right TPJ and the left AI (Fig. 8B). Seeding from the FP, dmPFC, PCC, and left TPJ congruently resulted in prominent lateralized connections only to the IFG in the left hemisphere. Further, the TP and MTG in the temporal lobe featured prominent left-lateralized connectivity pattern not only to the left IFG, but the left MTG and right TP seeds were also connected to the left TPJ in MACM, and the left TP seed yielded connectivity to left pSTS, HC, and MT/V5 in MACM.
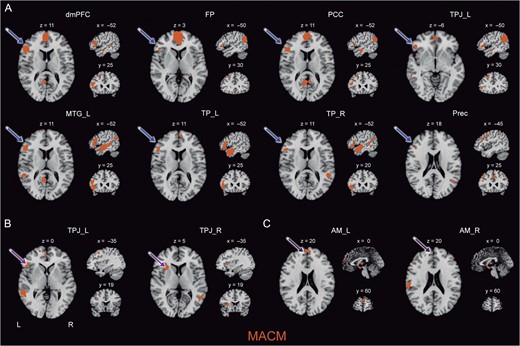
Lateralization effects in the social brain. Depicts most important hemispheric asymmetries in task-constrained brain states (MACM) in axial, sagittal, and coronal slices. (A) Most regions from the higher-level subnetwork showed a lateralized connectivity pattern with the IFG constrained to the left hemisphere. (B) The left TPJ seed yielded lateralized coactivation with semantic processing regions such as the IFG in the left hemisphere, whereas the right TPJ seed coactivated with attention-related structures such as the AI. (C) The AM seed in the left hemisphere showed specific connectivity with the dmPFC, in contrast to the AM seed in the right hemisphere. All results are cluster-level corrected for multiple comparisons. For abbreviations see Table 1.
In the visual-sensory and limbic clusters, MACM analysis also revealed a strong tendency for connections to the left hemisphere (Fig. 8C). The left AM, HC, and MT/V5 seeds were significantly connected to the left but not right AI. Moreover, the right AM and bilateral HC seeds showed coactivation with the TPJ only in the left hemisphere. The left HC seed also yielded task-dependent coactivation with the left MTG. Both left and right FG seeds yielded functional connectivity to left but not right HC. However, we also found hemispheric asymmetries lateralized to the right hemisphere between the left AM seed and the right IFG, as well as between the right AM seed and the right SMA, both in MACM.
The remaining seeds showed a more bilaterally distributed connectional architecture. We found that the aMCC and both AI seeds yielded a particularly strong overlap in functional connectivity between each other during tasks. Lateralization effects in these hierarchically intermediate set of seeds reduced to a task-dependent coactivation between the left AI and the left FG, as well as between the right AI and the right SMA. While the IFG, SMG, and SMA yielded mostly bilateral connectivity patterns across MACM and RSFC, we found lateralization differences in some regions, including task-constrained coactivations in the left IFG seed with the left pSTS, the right IFG seed with the right NAC, and the right SMA seed with the left FG. While both pSTS seeds were functionally connected to the left HC and FG in both analyses, only the left pSTS seed showed coactivation with MTG and MT/V5 in MACM.
In summary, a trend for lateralization to the left hemisphere was apparent for social brain seeds during tasks. These asymmetries converged to connectivity targets along the surface of the frontal and temporal lobes in the left hemisphere.
Neural Correlates of a Putative “MNS”
We found significant task-constrained coupling between the IFG, SMG, SMA, and pSTS seeds. The monkey homologs of these regions have been repeatedly related to action observation and imitation in animal studies (Gallese et al. 1996; Fogassi et al. 1998). As a global observation, networked configurations of a potential MNS in humans were only present in the task-constrained brain. The RSFC analysis failed to show a networked functional connectivity between these seeds. Only the SMG and SMA seeds showed coherent RSFC correlations with the rest of the social brain. This is similar to our findings for social brain seeds related to the intermediate cluster and contrary to those related to the higher-level cluster.
As an important specific observation, hierarchical clustering led to a shared cluster of seeds in the social brain that included the AI and aMCC, together with the potential MNS (mostly IFG, SMA, SMG, but also pSTS and MT/V5) in humans. We found many instances of task-dependent coupling of these seed regions, especially when only taking into account the social brain seed regions (intranetwork analysis). Additionally, MACM and RSFC connectivity analyses agreed in clearly segregating this set of seeds from the regions belonging to the higher-level cluster. That is, the connectional configurations of the dmPFC, FP, vmPFC, PCC, and bilateral TPJ did not show strong connections to seeds in the intermediate-level cluster in MACM or RSFC.
The MNS-related seeds showed a particularly strong connectivity pattern between the IFG and SMA seeds (see Fig. 6). The RSFC analysis only revealed weak functional correlations between the bilateral IFG seeds, as well as between the bilateral SMG and right SMA. The left IFG seed also showed task-dependent coactivation with the NAC, while the left SMG and right SMA seeds showed resting-state correlations with the NAC. In RSFC analyses, the right IFG and bilateral SMG seeds were connected to the aMCC. The left IFG and bilateral SMA seeds were also connected at rest with the bilateral TPJ. While the left SMG, left SMA, and right pSTS seeds yielded task-dependent coactivation with the bilateral AI, only the left SMG seed was functionally connected to this structure in RSFC. Furthermore, the left pSTS seed showed resting-state correlations with dmPFC, while the right pSTS seed was connected to this same structure in MACM. The left SMA seed yielded connectivity to PCC only in RSFC. Finally, bilateral SMG and SMA seeds were all connected to the Cereb in RSFC.
In summary, pronounced overlaps of MACM results were observed between seed regions in the putative mirror neuron system as well as in the bilateral AI and aMCC. Additionally, these connectional configurations were quite different from social brain connectivity in the higher-level seeds.
Task-Constrained versus Resting-State Connectivity
A general trend for agreement was observed between task-dependent coactivations and resting-state correlations for our social brain atlas (Fig. 2B). However, the strength of intranetwork connectivity patterns varied to a greater extent across the 2 connectivity modalities, being more prominent in task-constrained coupling as measured by MACM than in task-free coupling as measured by RSFC.
In the “higher-level cluster,” convergence across the 2 analyses was observed for the FP, dmPFC, PCC, TPJ, TP, and MTG seeds. However, as mentioned above, the functional connections between these seeds and the IFG were lateralized to the left hemisphere in MACM but bilaterally distributed in RSFC. Furthermore, the FP, dmPFC, PCC, and TPJ seeds showed resting-state correlations with the Cereb that were not present in MACM. Moreover, the TP in the left hemisphere yielded significant task-dependent coactivations with the lower-level regions pSTS, MT/V5, and HC.
In the “intermediate-level cluster,” the aMCC seed showed task-dependent functional connectivity to the MNS-related target regions SMA and SMG. This seed was additionally connected to the bilateral dlPFC as well as to the NAC and Cereb only at rest. The bilateral AI seeds were both significantly connected to the PCC in RSFC but not in MACM. Functional connectivity between the left AI seed and the bilateral pSTS and NAC was found only in MACM. Further, the SMA and SMG seeds also showed a congruent functional connectivity pattern across MACM and RSFC results. Task-constrained specific connectivity patterns were found between the left pSTS seed and the left MTG and MT/V5, as well as between the right pSTS seed and the dmPFC, bilateral AI, and left FG and HC. However, resting-state correlations were found between the bilateral SMA seed and the bilateral TPJ, as well as between the right IFG and SMA seeds with the NAC. Moreover, the left SMG seed showed connectivity to the FG only at rest. Again, all the IFG, SMG, SMA, and pSTS seeds from both hemispheres showed functional connections to the Cereb only in RSFC.
In the “limbic cluster,” especially the AM seeds showed connectivity differences between MACM and RSFC. We found that both AM seeds were coactivated with the bilateral AI, IFG, and FG in MACM, while only the left AM seed yielded functional connectivity to the dmPFC in MACM. In contrast, both left and right AM seeds were connected to the vmPFC in RSFC. While the left HC seed was also connected to the vmPFC across MACM and RSFC, the right HC seed only yielded connectivity to the vmPFC at rest. Furthermore, we found task-dependent connectivity between the right HC seed and the bilateral NAC, FG, AI, and left TPJ, as well as resting-state correlations between the left HC seed and the bilateral TPJ, MTG, and IFG. We also found functional connectivity between the bilateral FG seeds and the bilateral AI, SMA, and left HC only in MACM.
In summary, our functional connectivity analyses comprehensively characterized the task-rest differentiation of the social brain. Social brain seeds tended to exhibit a higher number of specific connections during tasks, rather than at rest, and these predominantly targeted regions in the left hemisphere.
Functional Profiling
Each social brain seed was separately characterized by quantitative association with 2 comprehensive description systems of mental operations (Fig. 9 and Supplementary Figs 5–7): the Behavioral Domains (BD) and Paradigm Classes (PD) from the BrainMap taxonomy. We measured the likelihood of observing neural activity in a seed given previous knowledge of a given cognitive category (i.e., forward inference) as well as the likelihood of a particular cognitive category given observed neural activity in a certain region (i.e., reverse inference).

Functional specialization for behaviors in the social brain. Forward inference was drawn to comprehensively profile each seed according to the “Behavioral Domain” categories that are part of the BrainMap taxonomy (http://brainmap.org/scribe/). Each cube represents the likelihood of observing activity in a seed given previous knowledge of a specific cognitive process. The taxonomy is ordered and colored into social (“red”) versus nonsocial (“blue”) Behavioral Domains to facilitate visual comparison. First, all seeds turned out not to be “functionally specific” for subserving social-affective, as opposed to nonsocial, processes. Second, each region in our social brain atlas exhibits an “idiosyncratic portfolio” of associations with various psychological tasks. For abbreviations see Table 1.
Generally, each seed of the social brain was associated with several cognitive categories to a relevant extent. Based on BDs or on PCs, none of the seeds was linked to few or no cognitive terms. This piece of evidence indicated that “each seed individually contributes to a diverse and distinct set of cognitive facets,” even if they act in concert to entertain social cognition. This observation prompted us to be more cautious about the results from the reverse inference analysis. We therefore focus on the results derived from the forward analysis (see Supplementary Figs 6 and 7 for the reverse inference results). Specifically, both BDs and PCs agreed in 3 main observations.
First and foremost, we found a similar number of relevant functional associations with taxonomic terms with and without relation to social-affective processing. In BDs, the dmPFC for instance showed relevant associations with the social categories emotion, especially disgust, fear, and sadness, as well as sexuality but also with the nonsocial categories reasoning, working memory, orthography, and spatial processing. In PCs, the left amygdala for instance showed relevant associations with the social categories facial judgments, action observation, affective words, and whistling but also the nonsocial categories finger tapping, olfactory discrimination, pain processing, memory retrieval, semantic reasoning, and go/no-go attentional processing. This trend of “lacking exclusivity for social-cognitive categories” held for every seed in the social brain atlas. This provided data-driven evidence against the existence of a brain system that would be uniquely devoted to social-affective processing in humans. Note however that the nature of the present study entails a limitation of functional association results to the level of “entire” seed regions. Recent studies using other analysis approaches have shown that multivariate patterns “within” specific regions in the brain can possibly account for social-specific processes, such as in the temporo-parietal junction for perceived behavioral relevance of other agents (Carter et al. 2012), in the anterior insula for affective empathy (Tusche et al. 2016), or in the dorsal anterior cingulate cortex for social rejection (Woo et al. 2014).
Second, “seeds that belong to the same cluster (i.e., visual-sensory, limbic, intermediate, and high-level) exhibited more similar functional associations than seeds from any 2 of these clusters.” In BDs, the seeds from the high-level cluster for instance showed the highest likelihood for the social cognition category (except for the left TPJ and pMCC) comparing to seeds from the other 3 clusters. Similarly in BDs, the high-level cluster showed among the highest likelihood for processing of musical information (except for the pMCC). It was only seconded by the left and right pSTS in the limbic cluster. As an interesting side note, the closest associations with fear were not only found in the limbic cluster (especially AM, vmPFC, and rACC) but also in the high-level cluster (especially pMCC, FP, dmPFC, and right TPJ). These findings provided a cross-confirmation for the presented network parcellation solution into 4 clusters based on functional profiles derived from different data and statistics.
Third, the functional profiling results are consistent with a left lateralization of language-related processes and a right lateralization of attention-related processes. Stronger language association on the left versus right was observed for: SMG (all language categories), MTG (orthography, phonology, semantics, and speech), IFG (semantics, speech, and phonology), TP (semantics and orthography), AM (semantics), and NAC (semantics and syntax), TPJ (semantics and syntax), IFG (semantics and speech), SMA (speech), and MT/V5 (syntax). Stronger association to attention processes, in turn, on the right versus left was observed for: FG (visuospatial attention, tone discrimination and attention, action observation, as well as visual motion and tracking), IFG (classical conditioning, saccades, as well as pain, vibrotactile and thermal monitoring), MT/V5 (action observation, saccades, tone discrimination, as well as stoop, go/no-go, oddball and n-back tasks), AI (acupuncture, saccades, oddball tasks, thermal and vibrotactile stimulation), AM (cue recall and finger tapping, pain and Wisconsin card sortin tasks), HC (saccades, pain, n-back and covert naming tasks), pSTS (go/no-go tasks, oddball tasks, action observation), NAC (delay matching, flanker and Wisonsin card sorting tasks), SMA (tone discrimination and visuospatial attention), SMG (acupuncture and pitch discrimination), TPJ (action observation and visual motion tasks), TP (paired associate recall and stroop), MTG (oddball and pain tasks), and Cereb (saccades, cued explicit recognition).
Discussion
Previous research on the neural instantiation of social-affective information processing has typically tapped on only a small set of cognitive processes and concentrated interpretation on preselected brain regions. This local function to social cognition research motivated the present study to undertake a comprehensive exploration of all social brain systems. Thirty-six seeds were determined by deriving the quintessence from published quantitative meta-analyses on 3 972 social-affective experiments in 22 712 participants. The derived social brain atlas, as a quantitative summary of how social-affective behavior is commonly measured in brain-imaging research, was the basis for measuring concomitant neural activity changes in the task-focused mind set (MACM) as well as time-series correlation of activity fluctuations in the task-free mind set (RSFC). The complementary modalities allowed investigating connectivity patterns within and outside the social brain, without constraining the present study to a specific theoretical concept, a particular brain region, or a preselected target network.
Hierarchical clustering across seed connectivity profiles established on its most coarse-grained level a segregation into 4 different functional systems: (1) a “limbic cluster” of seed regions (vmPFC, rACC, AM, hippocampus, NACC), (2) a “visual-sensory cluster” of processing regions (FG, pSTS, MV/V5), (3) a “cluster of intermediate-level processing” (AI, aMCC, IFG, SMG, SMA, Cereb, possibly also pSTS), and (4) a “cluster of higher-level processing” (FP, dmPFC, PCC, TPJ, TP, MTG, Prec). We observed a tendency of the seed regions to yield predominant connectivity within either higher-level or lower-level hierarchical processing levels. In contrast, several seeds in the social brain, such as the AI, AM, vmPFC, pSTS, and TPJ, yielded a transitional connectional profile bridging advanced associative and lower-level sensory processing areas. While most seed regions featured connectivity patterns largely symmetrical across cerebral hemispheres, a number of exceptions with frequent left lateralization were found, including the dmPFC, AM, IFG, HC, and pSTS. Thus, the present investigation quantitatively characterizes the connectional architecture of the brain networks underlying social-cognitive processes with regard to (1) task-unconstrained versus task-conditioned brain states, (2) sensory-related versus abstract associative processing, (3) hemispheric asymmetries, as well as (4) the frequently discussed functional networks underlying ToM, empathy, and the MNS, which we will consider in the following.
The Environment-Engaged versus Detached Social Brain
The constructed social brain atlas was analyzed using 2 different approaches to functional connectivity that emphasize distinct aspects of functional brain architecture (Buckner et al. 2013; Eickhoff et al. 2015). MACM analysis captures the coactivation probability of brain regions across a large quantity of diverse neuroimaging experiments, whereas RSFC analysis is based on fMRI time series obtained while participants are scanned in the absence of a structured task set. The large majority of seed regions showed an almost identical whole-brain connectivity pattern in MACM and RSFC, including the dmPFC, FG, SMG, MT/V5, and TPJ. This concurs with previous neuroimaging studies where MACM and RSFC analyses show widespread topographical agreement (Cauda et al. 2011; Hardwick et al. 2015). Thus, the currently increasing evidence for a close task-rest correspondence extends to the human social brain whose brain network stratifications were shown to be largely robust in the context of volatility in the external environmental and throughout cognitive sets (Smith et al. 2009; Mennes et al. 2013; Bzdok et al. 2016).
However, our analyses also showed notable differences across both connectivity techniques. Considering connectivity only within the social brain (i.e., intranetwork analyses), both AM were congruently coupled with the HC, vmPFC, and NAC during tasks (MACM), while these coupling patterns among nodes of the limbic system were absent outside of the task setting (RSFC). More specifically, the AM featured congruent connectivity comparing to the IFG and aMCC of the salience network at rest but not during tasks in both intranetwork and extranetwork analyses. Although the present study qualifies as exploratory in nature, these results provide evidence that the AM assumes a double-integrator role by functionally binding limbic system nodes during environmental stimulation and a general maintenance network in the unconstrained brain state. This is congruent to previous coordinate-based meta-analyses using another modality of connectivity: psychophysiological interactions. These studies support a differential role of the AM as both an input-processing region and as an integrator of other regions important for large-scale network dynamics including the prefrontal cortex (Smith et al. 2016; Di et al. 2017). The different levels of task-dependent interaction between the AM and other regions highlight the importance of this seed in distinct brain mechanisms and, thus, potentially cognitive and affective processes. Additionally, the vmPFC, typically involved in stimulus-value association and decision-making (Kringelbach and Rolls 2004; Gläscher et al. 2012), showed a task-dependent coactivation with the NAC of the reward circuitry that was not observed in the idling social brain. It concurs with the vmPFC’s proposed role in approach-avoidance choices towards individuals and objects in the here and now that predict social competences and social network properties (Lebreton et al. 2009; Powell et al. 2010), whereas the medial FP and the dmPFC are more intimately related to environment-detached, internally-generated mentation (Laird et al. 2009b; Nicolle et al. 2012; Bzdok et al. 2013; Bado et al. 2014). Finally, as mentioned above, characteristic lateralization patterns, observed especially to the left IFG, were mostly a property of the task-engaged rather than mind-wandering social brain.
In summary, we detail the previous idea of ongoing social cognition as a possible neurophysiological baseline (Schilbach et al. 2008, 2012; Krienen et al. 2010) by identifying a characteristic task-rest sub-differentiation in social brain systems. DMN-related regions exhibited the highest and saliency-network-related regions the lowest coherence across the 2 disparate brain states. The known antagonistic physiology between the default mode and saliency networks therefore appears to extend to social brain function (Fox et al. 2005; Fransson 2005; Bressler and Menon 2010).
Social Cognition Requires Integration between Sensory and Associative Processing
It is an ongoing debate to what extent social cognition is predominantly instantiated by high association cortices (Stone and Gerrans 2006; Decety and Lamm 2007; Mitchell 2009). We quantitatively revisited this question by conjoint analysis of lower- and higher-level social regions. For instance, dedicated modules were suggested to provide the basis of representing others’ mental states (Baron-Cohen et al. 1985). However, after more than 20 years of neurological lesion reports (Apperly et al. 2004; Samson et al. 2004), electrophysiological and neuroimaging studies in autism (for a review, see: Pelphrey et al. 2011), more and more investigators adopt mosaic explanations for theory of mind (Behrens et al. 2009; Bzdok et al. 2012a). Instead of exclusive reliance on a specialized monolithic system, theory of mind and other social capacities might develop ontogenetically and be maintained by collaboration of lower-level social-affective systems. These include face perception and joint attention, as well as general-purpose systems, such as working memory, executive function, and scene construction (Decety and Grezes 2006; Stone and Gerrans 2006; Schurz et al. 2014). In other words, the lower-level regions perform preprocessing of sensory input needed to inform, elaborate, and update internal models of social phenomena and interaction scenarios, while the back projection from higher-level regions exert influence over these lower-level processes controlled by pertinence of predictions of actual inputs (Corbetta et al. 2008; Abu-Akel and Shamay-Tsoory 2011; Song et al. 2011; Friston et al. 2013). This emerging contention is invigorated by the present functional profiling findings that unveiled a characteristic fingerprint of psychological task engagements for every single seed. Put differently, there is not one characteristic task for each seed. A mosaic view of the social brain was also confirmed by a number of further findings.
We obtained a “high-level subnetwork” of connectionally coherent seed regions known to be closely associated with theory of mind (i.e., bilateral TPJ, PCC, Prec, FP, MTG, TP, and dmPFC). They featured stronger coupling among each other than with any other seed region in the social brain during tasks (MACM) and at rest (RSFC). This set of brain regions is typically referred to as the “default mode” network (DMN) in the neuroimaging literature (Buckner et al. 2008). Please note that there is controversy whether the Prec should be considered a core part of the DMN (Utevsky et al. 2014) or does not belong to the “DMN proper” (Margulies et al. 2009; Bzdok et al. 2015). Iacoboni et al. (2004) specifically explored the relationship between this network and social-cognitive processes in an fMRI study. These authors found that participants watching social interactions in movie clips showed increased BOLD signal in the DMN compared with when they watched movie clips with single individuals performing everyday-life actions or during rest. This is congruent with another fMRI experiment showing that the neural activity in the posterior parietal region decreases when participants are required to retrieve self-knowledge relative to rest, but increases during social-knowledge retrieval compared with rest (Pfeifer et al. 2007). In a similar fashion, Spunt and colleagues (2015) have very recently suggested that the link between the DMN and social-cognitive mentalizing ability is not only coincidental in terms of the neural infrastructure. The authors tested in an fMRI study whether resting-state neural activity within the DMN regions prepares us to infer other individuals’ mental states. They found that increased spontaneous activity within the dmPFC preceding a social judgment task was related to shorter response times (Spunt et al. 2015). Moreover, individuals showing greater dmPFC activation at rest scored higher in a self-report measure of social skills. Together, these findings have been interpreted as a social priming effect in the resting-state activity of the DMN.
Another social brain cluster automatically grouped an “intermediate-level subnetwork” (AI, aMCC, IFG, SMG, SMA, Cereb, possibly also pSTS). The AI was long believed to be specific for disgust processing (Adolphs 2002), later consistently identified in vicarious emotion processing (i.e., empathy) and pain in social neuroscience (Lamm and Singer 2010; Fan et al. 2011; Bzdok et al. 2012b), and is today understood as an integrating link between large-scale brain systems (Kurth et al. 2010; Kelly et al. 2012). Confirming the latter, our connectivity results linked the AI to the bilateral IFG, precentral gyrus and SMA/aMCC in the frontal lobe, bilateral TPJ and SMG in the parietal lobe, FG in the posterior temporal lobe, and the Cereb in both MACM and RSFC analyses. The present results thus supplement the conceptualization of the AI (Seeley et al. 2007; Craig 2009; Menon and Uddin 2010) as salience and relevance detectors, which can underlie not only social but also nonsocial behaviors (cf., Ousdal et al. 2008; Kurth et al. 2010).
A similarly heterogeneous functional connectivity pattern bridging hierarchical processing levels was found for the AM in the limbic cluster. It showed connectivity to the intermediate seed clusters (aMCC and IFG) but also lower-level regions (thalamus, subthalamus, HC, and parahippocampal cortex in both MACM and RSFC) and higher-level regions (dlPFC, vmPFC, FP, and MTG). Furthermore, our FG seed in the visual-sensory cluster corresponds to the “fusiform face area” involved in socially-relevant input processing (Puce et al. 1995; Kanwisher et al. 1997; Haxby et al. 1999). Its connectivity results range from AM, visual cortex, FG (MACM and RSFC analyses) and right pSTS (MACM) to the higher-level regions AI, SMG, and MTG (MACM). This concurs with the described model of FG connectivity to an extrastriate core system for face perception and a distributed, extended system for gaze perception and spatial attention (Mishkin et al. 1983; Harries and Perrett 1991; Colby and Goldberg 1999; Hoffman and Haxby 2000). Appraisal and binding of environmental input carrying social information is modulated by the NAC, a major node for motivation and reward (Schultz 2004; Knutson and Cooper 2005). In line with a previous study on NAC connectivity (Cauda et al. 2011), our results showed connectivity patterns from the NAC seeds to AI, AM, HC, and dorsal thalamus in both MACM and RSFC analyses. The functional connectivity between the NAC and the most ventral mPFC seed concurs with their well-described direct anatomical connections (Haber and McFarland 1999). In contrast to AI, AM, and NAC, the cerebellum is typically neglected in studies on social cognition (but see: Stoodley and Schmahmann 2009; Van Overwalle et al. 2013). The present seeds in the cerebellar lobules VII and VI exhibited motor-related connections to the SMA, bilateral dorsal striatum, and precentral gyrus in MACM and RSFC emphasize a possible role in motor-mediated representation for embodied simulation facets in social-affective processing. Yet, recent connectivity studies support functional connectivity of the cerebellum with the ToM network (Van Overwalle and Mariën 2016).
In summary, the social brain spans across different neural processing levels when divided into 4 broad functional components. The high-level subnetwork, previously known for cohesive response such as in theory-of-mind tasks, showed a number of links to brain parts outside of the association cortex that are generally implicated in attention, executive, memory, and spatial processes. The intermediate-level subnetwork, known for cohesive response such as in empathy and pain tasks, featured more links with brain parts dedicated to preprocessed sensory input and motor response preparation. Yet, it was itself superseded by the limbic and visual-sensory subnetworks, known as collectively responsive such as to facial and other biologically relevant cues, with the most immediate relation to perception-action cycles in social cognition.
Functional Lateralization in the Social Brain
Neuroscientific investigations on hemispheric specialization have broadly converged to the left cerebral hemisphere as dominant for language (Broca 1865; Wernicke 1874; Lichtheim 1885) and the right hemisphere as dominant for attention functions (Gazzaniga et al. 1965; Sperry 1982; Stephan et al. 2003). This contention is largely confirmed by the present functional profilings results. The present lateralization findings in connectivity will therefore be interpreted with emphasis on functional asymmetry between semantics versus attention (Stephan et al. 2007; Seghier 2013). In general, we found more inter-hemispheric differences in social-affective network architectures based on task-constrained functional connectivity than in the absence of experimental constraints.
Regarding the high-level cluster in the social brain, most regions showed a left-favored lateralization pattern of functional connectivity. For instance, MACM revealed FP, dmPFC, PCC, and left TPJ to be connected to left (but not right) IFG, which is topographically related to Broca’s region (Amunts et al. 2010). The FP seed showed functional connectivity to the left HC, while PCC yielded connectivity to the left MTG, related to elaboration of preprocessed auditory and semantic information (Binder et al. 2009). While some previous studies on ToM-related regions have reported bilateral activity patterns (Mar 2011; Schilbach et al. 2012), several social cognition studies argued for a contribution of the left TPJ to processing semantic aspects (Saxe and Kanwisher 2003; Hensel et al. 2015; Price et al. 2015) and the right TPJ to processing lower-level attentional aspects (Decety and Lamm 2007; Mitchell 2008; Scholz et al. 2009; Santiesteban et al. 2012) of task performance. It is conceivable, however, that higher-level, self-related cognitive processes, such as prospection, delay discounting or self-others distinction, partially rely on world knowledge stored as semantic concepts, characterized by consistent left lateralization (Binder et al. 1999; Suddendorf and Corballis 2007; Binder et al. 2009; Carruthers 2009; Gotts et al. 2013). The left TPJ seed even showed widely distributed connectivity to targets across the lateral temporal lobe from pSTS to TP in the task-unconstrained brain state. In contrast, the right TPJ seed was connected to AI and lateral SMA indicative of putative links to attention and motor control. Consequently, the ToM-related default-mode regions generally showed a close relation to left-sided semantic processing networks during tasks and at rest.
Analogous to the high-level subnetwork in the social brain, the intermediate-level subnetwork showed left-skewed connectivity profiles, again more in MACM than RSFC. Lateralization effects of the bilateral pSTS, left SMG, and right SMA converged to the left FG during tasks. However, the overall left lateralization of FG-seeded coactivations stands in contrasts with the widespread idea that the right fusiform gyrus is more specifically tuned to face perception in humans (De Renzi 1986; Kanwisher et al. 1997; Wada and Yamamoto 2001; Barton 2008). Interestingly, further left-lateralized coactivations were found from left pSTS with left MTG and left MT/V5 and from right pSTS with left HC. The established role of the pSTS in multi-modal sensory integration during both stimulation with and without speech (Buchsbaum et al. 2001; Leech et al. 2009) appears to be implemented in a set of heterogeneous nodes with pronounced left participation. The neural response of the so-called saliency network, closely related to empathic performance, has mainly been reported to be bilaterally distributed (Fan et al. 2011; Lamm et al. 2011; Bzdok et al. 2012b). This is confirmed by our analyses that showed bilateral connectivity patterns for the AI and aMCC across task and rest. The exception of task-dependent coactivation between the right (not left) TPJ seed and the AI concurs with the general impression that the salience network subserves empathic processing by preferential relation to attention, consciousness awareness, and detection of self-relevant social cues (Craig 2002; Luo et al. 2014), in contrast to the left-lateralized DMN subserving ToM processing.
Switching from more associative regions to the visual and limbic subnetworks of the social brain, the AM seeds yielded a particularly heterogeneous and distributed lateralization pattern. Besides many bilateral connections, the AM coactivated during tasks with higher-level regions such as FP, dmPFC, IFG, and AI. More important, the FP connected specifically to the left AM, while the vmPFC equally connected to both AM across MACM and RSFC. Therefore, the left AM seems to be more specialized in information modulation in concert with high association cortices. While meta-analysis evidenced the left AM to activate more often than its right counterpart during emotion-perception tasks (Sergerie et al. 2008), this physiological effect might be explained by faster habituation in the right AM (Wright et al. 2001). Generally, different authors voiced the suspicion that the right AM is relatively specialized in rapid, dynamic emotional stimuli detection, whereas the left AM is more dedicated to sustained evaluation of environmental stimuli (Morris et al. 1998; Markowitsch 1999; Phillips et al. 2001; Wright et al. 2001). For instance, left AM has been specifically associated with particularly elaborate social cognition processes such as moral cognition (Bzdok et al. 2012b), emotion regulation (Delgado et al. 2008; Diekhof et al. 2011; Kohn et al. 2014), story-based theory of mind (Mar 2011), in-group versus out-group social categorization (Shkurko 2013), and unconstrained cognition (Schilbach et al. 2012). Conversely, exposure to emotional faces for less than 100 ms showed right lateralized AM activity (Morris et al. 1998; Costafreda et al. 2008).
The left lateralization of the AM seed is partly mirrored by the neighboring HC. Both HC seeds were connected to left TPJ, and the left HC was also connected to left MTG in MACM. Similar to the amygdalar connectivity pattern, the left HC yielded connectivity to the vmPFC extending to FP in both MACM and RSFC, while right HC only showed connectivity to vmPFC in RSFC. This concurs with previous meta-analytic reports that implicated the left HC relatively more in various higher-level functions, including autobiographical memory, prospection, navigation, and theory of mind (Spreng et al. 2009). Similarly, both FG seeds yielded functional connectivity to left HC. Face perception has been described as highly lateralized to the right hemisphere based on neurological lesion patients (De Renzi 1986; Wada and Yamamoto 2001; Barton 2008). However, since its specific role in face processing was proposed (Kanwisher et al. 1997), there has been an active discussion on its functional specialization. Some authors have pointed out that complex, multi-part visual stimuli such as chess-game distributions (Bilalić et al. 2011) can also elicit greater activation in FG in experts compared with novices. In a recent study, Ma and Han (2012) found a left-favored activation in FG for physical recognition of one’s own face, while right FG was more sensitive to self-identity recognition. Together, these left-favored connectivity patterns shown by lower-level regions, including AM, HC, and FG, might reflect a global tendency for social-affective input processing regions to be lateralized to the left hemisphere as a possible consequence of unavoidable semantic process recruitment in experimental neuroimaging paradigms.
In summary, lateralization concepts from the animal and human amgdala proposed that “rapid automatic detection” is more related to the right hemisphere and “detailed evaluative elaboration” is more related to the left hemisphere. Our findings suggest that this functional lateralization account of amygdalar responses extends to other parts of the social brain.
A “MNS” in the Social Brain?
Mirror neurons are defined by identical spiking activity during passive observation and active execution of specific motor movements (Di Pellegrino et al. 1992; Gallese et al. 1996; Fogassi et al. 2005). They have initially been described in monkeys in the frontal area F5 and the parietal area PF/PFG (Gallese et al. 1996; Fogassi et al. 1998). In humans, the precise nature of the MNS has remained a topic of debate (Keysers and Gazzola 2010). Recently, existence of a human MNS was directly indicated by invasive single-cell recordings during neurosurgery (Mukamel et al. 2010) and located to the inferior frontal gyrus, posterior superior temporal sulcus, ventral premotor, and somatosensory parietal cortices by neuroimaging meta-analysis (Van Overwalle and Baetens 2009; Caspers et al. 2010). Action simulation in an observer’s MNS was often proposed to enable inference of others’ mental states from their nonverbal behavior (e.g., Grezes et al. 2004; Vogeley and Bente 2010). This is extended by the present results to coherent network connectivity during various psychological tasks that is much scarcer during rest. The bilateral SMG, IFG, SMA, and pSTS seeds were connected to the medial and lateral SMA, while left SMA and left pSTS seeds were functionally connected to the left IFG. The strong functional coupling between alleged MNS nodes according to MACM but much less RSFC is contrasted with the robust connectivity among DMN seeds in task and rest. Consequently, environmental cues of other individuals’ actions might indeed flow from audiovisual integration in the pSTS to the SMG. From the SMG the information would be forwarded to the IFG for planned action execution (i.e., imitation) informed by simulated motor representation of the observed action to reduce error in predicting environmental events (Iacoboni et al. 1999; Keysers and Perrett 2004; Kilner et al. 2007). Note however that this account of mental-state inference based on action understanding has been subject to a number of critics (Saxe 2005; Hickok 2009; Hickok and Hauser 2010).
Further, the functional connections between these MNS-related (i.e., bilateral IFG, SMA and SMG) and empathy-related (i.e., aMCC and bilateral AI) seed regions were prominent up to the point of forming the shared intermediate-level cluster in our hierarchical clustering analysis. This result entices to speculate about an intimate functional relation between brain regions related to action observation and execution and those related to vicarious appraisal of someone else’s emotional states (Carr et al. 2003). It is in line with the previous argument (Gallese 2001) that action observation and execution may be crucially important for brain systems that instantiate empathy processes. As an important conclusion, our results discourage authors who have suggested that the cognitive mechanisms of abstract emulation in theory of mind might be a core processing facet underlying simulation and embodiment processes (Keysers and Gazzola 2007; Uddin et al. 2007), especially during video watching of motor actions (Iacoboni et al. 2005) and emotional judgments on faces (Schulte-Rüther et al. 2007). This is because no relevant functional connectivity was observed between the MNS-related IFG or SMG seed regions and the ToM-related medial FP, dmPFC, PCC, TP, MTG, and TPJ. More generally, our results do not exclude the possibility that the MNS seeds exhibit general-purpose physiological properties by conjoint connections to sensory and motor systems. Even if our seed regions topographically coincide with core nodes of a human MNS, the amount of neurons showing mirror-like firing properties have been reported to account for only 10% in total (Rizzolatti et al. 1988; Gur et al. 2002).
In summary, the idea of a MNS in humans receives support from the coherent network coupling observed in the present connectivity investigations that is typical in connectivity strength for other well-defined brain networks. More important, we propose a reconciliation of the debated primacy of mentalizing versus motor simulation in social cognition by showing that the putative human MNS was stronger connected to the canonical network underlying “embodied simulation” (e.g., empathy) in stark contrast to that of “abstract emulation” of social events (e.g., theory of mind). This insight underlines the advantage of a systems-neuroscience approach to the neurobiological implementation of social cognition.
Conclusion
Human social behavior results from neural processes in the brain. Yet, there are few neuroscientific studies that attempt to explore the neurobiological implementation of social behavior from a systems neuroscience perspective. The present study extracted 36 brain regions that have been topographically defined based on relatively highest involvement for social processes and systematized their physiological relationships in connectivity analyses. Using a toolbox of data-driven methods, we achieved far-reaching conclusions about the functional relationships between social brain systems as they are routinely quantified by means of brain-imaging experiments. Most importantly, we provided quantitative evidence that “social cognition is realized by neither a single nor a uniquely social (1) region, (2) network, or (3) hierarchical processing level.” As another important conclusion, seed regions consistently associated with empathy and MNSs gathered in a same functional group and clearly segregated from the theory-of-mind-associated default-mode system. This makes the case for combining abundant neuroimaging resources and machine-learning statistics (cf. Bzdok and Yeo, 2017) to design a nomenclature of social cognition directly derived from brain recordings. Trans-disciplinary understanding of social behavior would benefit tremendously from a vocabulary that originates from neurobiological reality rather than human invention.
Supplementary Material
Supplementary material are available at Cerebral Cortex online.
Funding
The research leading to these results has received funding from the European Union Seventh Framework Programme (FP7/2007-2013) under grant agreement no. 604102 (Human Brain Project). The study was further supported by the Deutsche Forschungsgemeinschaft (DFG, BZ2/2-1, BZ2/3-1, and BZ2/4-1 to D.B.; International Research Training Group IRTG2150), Amazon AWS Research Grant (D.B.), and the START-Program of the Faculty of Medicine, RWTH Aachen (D.B.), and an NWO VIDI grant (452-13-015, R.B.M.).
Notes
We are grateful for helpful comments by Jorge Moll and Sarah Krall on a previous version of this manuscript. Conflict of Interest: None declared.