-
PDF
- Split View
-
Views
-
Cite
Cite
Ben Eppinger, Hauke R Heekeren, Shu-Chen Li, Age Differences in the Neural Mechanisms of Intertemporal Choice Under Subjective Decision Conflict, Cerebral Cortex, Volume 28, Issue 11, November 2018, Pages 3764–3774, https://doi.org/10.1093/cercor/bhx239
- Share Icon Share
Abstract
Older decision-makers may capitalize on their greater experiences in financial decisions and by this offset decline in cognitive abilities. However, this pattern of results should reverse in situations that place high demands on cognitive control functions. In this study, we investigated how decision conflict affects the neural mechanisms of intertemporal decision-making in younger and older adults. To individually adjust the level of decision conflict we determined the indifference point (IDP) in intertemporal decision-making for each participant. During functional magnetic resonance imaging, participants performed choice options close to their IDP (high conflict) or far away from the IDP (low conflict). In younger adults, decision conflict leads to reduced delay discounting and lower discount rates are associated with higher working memory (WM) capacity. In older adults, high decision conflict is associated with enhanced discounting, hypoactivation in the ventral striatum as well diminished ventral striatal representations of differences in subjective values. Taken together, our results show that under enhanced decision conflict, younger adults engage in a more reflective decision mode that reflects individual differences in WM capacity. In contrast, older adults get more present-oriented under high demands on cognitive control and this decision bias is associated with changes in striatal value signaling.
Introduction
A decision conflict can be understood as a condition of a labile equilibrium, in which choice options have approximately similar subjective values and individuals are indifferent with respect to which to choose (Lewin 1935). These types of decision conflicts occur frequently in daily life. They may arise in unusual, significant situations when having to decide between medical treatments or in moral dilemmas; but decision conflicts also occur during more mundane activities, such as grocery shopping or when having to decide between 2 dishes in a restaurant. In this study, we investigated how age-related changes in brain functions affect the ability to solve intertemporal decision conflicts using fMRI.
Delay (temporal) discounting refers to the fact that humans and other animals tend to discount the value of future reward (Ainslie 1975; Laibson 1997). The degree of delay discounting differs considerably between individuals (e.g., Green et al. (1996); Shamosh et al. (2008)). One factor that has been repeatedly associated with individual differences in delay discounting is fluid intelligence (Shamosh et al. 2008; Wesley and Bickel 2014). Several findings in younger adults suggest that greater fluid abilities are associated with reduced delay discounting (Shamosh and Gray 2008). Moreover, the relation between fluid intelligence and discounting seems to be partially mediated by working memory-related activity in the anterior prefrontal cortex (aPFC) (Shamosh et al. 2008).
Whereas decision options can often be evaluated by objective criteria, the value of different options for the individual is usually subjective (Kable and Glimcher 2007). In the context of delay discounting, the concept of “indifference point (IDP)” reflects the point of equilibrium at which there is no difference in the subjective value between smaller but sooner and larger but later options. Recent studies suggest that the decision conflict that is induced by choice options that are close to the IDP is reflected in longer reaction time RTs (RTs), as well as elevated PFC activity (McClure et al. 2004; Marco-Pallares et al. 2010; Eppinger et al. 2012).
Several behavioral findings point to reduced discount rates in older relative to younger adults (Green et al. 1994; Reimers et al. 2009; Löckenhoff et al. 2011; Li et al. 2013). However, there are also studies that found no significant age differences in delay discounting (Green et al. 1996; Read and Read 2004; Samanez-Larkin et al. 2011). The few age-comparative neuroimaging studies that have been conducted so far, suggest that lower discount rates in older adults are associated with a reduced sensitivity of the ventral striatum to temporal delay information (Samanez-Larkin et al. 2011; Eppinger et al. 2012). Thus, it could be argued that reduced delay discounting in the elderly may results from with aging-related decline in dopaminergic projections to the ventral striatum (Eppinger et al. 2012).
However, it is well-known that cognitive control functions such as the ability to flexibly switch between cognitive tasks or to monitor conflicting response tendendies are impaired in older adults (Kray et al. 2005; Eppinger et al. 2007) and these limitations may affect decision-making abilities (MacPherson et al. 2002; Peters et al. 2007). That is, the pattern of reduced delay discounting in older adults may reverse under high demands on cognitive control functions. In this study, we investigate how control demands affect age differences in the neural correlates of delay discounting. To do so, we asked younger and older participants to perform intertemporal decisions during fMRI assessment and manipulated the level of decision conflict based on individually assessed IDPs. The participants completed 2 experimental sessions. In the first session, they performed a delay-discounting paradigm and we determined their individual IDPs based on the choice behavior during this task. These individual IDPs were used to adjust the choice options in the fMRI session. During the fMRI session, the participants were either confronted with choice options close to their personal IDP (high conflict) or with choice options that were far away from their IDP (low conflict). We expected that the presence of larger decision conflict would result in longer decisions times, steeper discounting of delayed reward and elevated PFC recruitment. Furthermore, we predicted that older adults’ deficits in cognitive control functions might result in greater discounting of delayed reward under high decision conflict and this effect may be reflected in an imbalance in prefrontal control and ventral striatal valuation systems (Hare et al. 2009).
Materials and Methods
Participants
Twenty-seven younger adults and 31 older adults participated in the study. Two younger adults were excluded due to head motion (>2 mm in either direction). Three older adults were excluded because their structural scans showed evidence for ischemic lesions. Two older adults were unable to stay in the scanner throughout the experiment. On one occasion, the experiment had to be aborted during the session due to an outside medical emergency (for which the scanner was needed). Taken together, the effective sample consisted of 25 younger adults (mean age = 24.8s SD = 2.5, 12 male) and 25 older adults (mean age = 71.2, SD = 5.6, 13 male), all right-handed. Participants gave written informed consent. The Institutional Review Board of the Charité Universitätmedizin Berlin approved the study. During the first session participants completed a biographical questionnaire, a working memory task as well as several psychometric tests: (1) Identical pictures test (Lindenberger and Baltes 1997); (2) Raven’s Progressive matrices (Raven et al. 1998); (3) Spot-the-Word test (Baddeley et al. 1992). Older adults had lower scores on the identical pictures test and Raven’s matrices than younger adults (P’s < 0.001, η2s > 0.56). However, they reached higher scores on the Spot-the-Word test (P < 0.001, η2 = 0.33). Consistent with previous findings these results suggest age-related declines in fluid abilities and relative stability/increase in crystallized intelligence (Li et al. 2004).
Participants also performed a time estimation task with a working memory load manipulation (for details see Supplementary Information). We had 2 research questions in mind when including this task: First, we were interested in whether there is an association between time perception and intertemporal preferences, as suggested in previous theoretical work (Wittmann and Paulus 2007; Ray and Bossaerts 2011). Second, we wanted to replicate the well-established negative correlation between working memory (WM) function and delay discounting (Shamosh et al. 2008; Wesley and Bickel 2014; Aranovich et al. 2016). Consistent with previous results on age differences in time perception, we found that both age groups tend to overestimate time delays and that this effect is more pronounced in older than younger adults (see Supplementary Fig. S1B) (Balci et al. 2009; Lustig and Meck 2011). However, in contrast to recent theoretical ideas, we found no association between time perception and delay discounting; meaning that one’s ability to accurately estimate time intervals has no bearing on intertemporal preferences. The analysis of the WM data revealed better WM in younger than older adults as well as a reduction of WM performance as a function of WM load across age groups (see Fig. S1C). Moreover, consistent with previous results, we found a significant negative correlation between WM performance and intertemporal choice behavior in younger, but not in older adults (see Supplementary Information).
Experimental Tasks
In this first session, IDPs were assessed. Participants performed a delay-discounting task that involved making 2-choice decisions between a smaller monetary reward that was available today and a larger monetary reward that was available in the future (see Fig. 1A). The immediate reward option was always presented on the left side of the screen and varied randomly between 12 and 20 €. The percent difference in Euro amounts between rewards was drawn randomly from 6 values (1, 5, 10, 20, 30, 40, and 50%). The delay between the early and the late reward was drawn randomly from (today—2 weeks, today—3weeks, today—4 weeks, today—5weeks, today—6 weeks). Each combination of choice pairs was repeated 4 times (120 trials in total). The task was paused every 30 trials to allow participants to rest. Each choice pair was presented for 6 s. Following the choice options, a blank screen was displayed for 1 s.
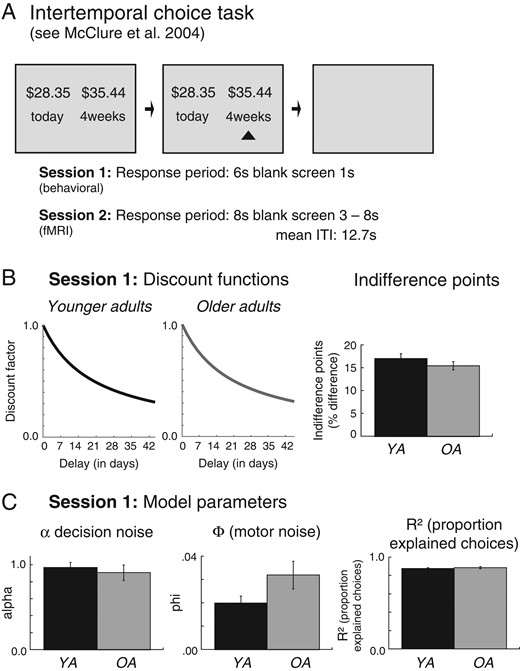
Task, design, and behavioral data from the first (behavioral) session. (A) Schematic figure of the delay discounting task. Participants were asked to make repeated decisions between sooner but smaller and larger but later monetary rewards. (B) Discount functions (left) and IDPs (right) for younger and older adults in Session 1. (C) Model parameters (decision noise, α and motor noise ) as well as model fits (proportion of explained responses) derived by fitting a hierarchical Bayesian model of delay discounting (Vincent 2016) to choice behavior in Session 1.
In the second (fMRI) session, participants had to choose between smaller sooner rewards that were available today or in 2 weeks and larger later rewards that would become available 2 or 4 weeks from the sooner reward (McClure et al. 2004; Eppinger et al. 2012). For high conflict options the percent difference between rewards was set to the individually determined IDPs from the behavioral session. The high conflict condition involved choices among options that were exactly at the IDP and options that were one standard deviation away from the IDPs. The idea behind this procedure was to account for possible inaccuracies in IDP assessment in Session 1. As shown in Supplementary Figure S2A within the high conflict condition the proportion of delayed choices scales linearly as a function of the distance to the IDP in both age groups. For low conflict options the percent difference between rewards was set to 1.5% (assuming that most subjects would choose the immediate reward) or 95% (assuming that they would choose the delayed reward). The sooner reward option was always presented on the left side of the screen and varied randomly between €12.00 and €20.00. On low conflict trials the delayed reward varied between 12.18€ and €39.00. On high conflict trials delayed reward magnitude depended on the individual IDP and varied between 12.35€ and €38.78. Each possible combination of delay and conflict was repeated 7 times (140 trials in total). During fMRI scanning the task was paused every 35 trials to allow participants to rest. Each choice pair was presented for 8 s. Following the stimulus, a blank screen was displayed for a variable interval of 2–8 s. The inter-trial interval (ITI) varied between 10 and 16 s, according to a long-tailed exponential distribution (λ = 4.0, mean ITI = 12.7 s) (Hagberg et al. 2001).
Procedure
In the first session, participants were screened for MR eligibility and performed the psychometric tests, the time production task, as well as the delay discounting experiment. If they were MR eligible they were invited for the fMRI session at a later date. In the delay discounting tasks, we asked participants to make decisions between smaller but sooner rewards and larger but later rewards. They were informed that there were no correct answers and that at the end of the experiment they would receive one of their choices as compensation. This choice was randomly selected from their choice set and the respective amount of money was paid to them either in cash or via wire transfer at the corresponding time point. In Session 1, stimuli were presented on a 19-inch Computer screen. In Session 2, stimuli were projected using MR compatible goggles (VisuaStim, Resonance Techology Inc.). EPrime 2.0 software (PST Inc.) was used for stimulus presentation. Manual responses were registered on standard keyboards (Session 1) and using a MR-safe button box (Session 2). Pillow and foam cushions were placed inside the head coil to minimize head movements.
fMRI Data Acquisition
MR image acquisition was performed on a 3 Tesla MRI scanner (Tim Trio; Siemens) at the Charité University Medicine Berlin, Campus Benjamin Franklin. At the beginning of the fMRI session high-resolution (1 mm3) T1-weighted structural images were acquired using an MP-RAGE pulse sequence [176 axial slices; field of view (FOV), 244; repetition time (TR), 1550 ms; echo time (TE), 2.34 ms; flip angle, 9°]. AC-PC aligned functional images were acquired using a T2* weighted EPI sequence [36 interleaved slices; voxel size: 3 × 3 × 4 mm; FOV, 216; TR, 2000 ms; TE, 30 ms; flip angle, 80°].
Behavioral Data Analysis
For the discounting data in the first session the computational model was fit separately to the data of the 2 age groups. We then derived the median parameters (discount rate K, decision error α, sensory-motor error , and the proportion predicted responses) for each individual. These parameters where compared using a mixed effects repeated measures ANOVA involving the between-subjects factor age group. For the second session, we fit the model separately for the 2 age groups and the 2 conditions. Median parameters from the model fitting were analyzed using an ANOVA with the between-subjects factor age group and the within-subjects factor conflict condition. For the parametric fMRI analyses, we used the model to determine 2 trial-wise regressors per individual: (1) the discounted value of the delayed option for each trial and (2) the unsigned difference between the discounted value of the delayed option and the value of the sooner option for each trial. We decided to use the discounted value of the delayed option as a subjective value regressor to stay close to procedures that have been published in the literature (e.g., Kable and Glimcher 2007; Peters and Büechel 2009). However, it should be noted that there are alternative strategies, such as taking the absolute subjective value across options as a regressor, which may pick up on neural processes that reflect the value of both options.
An analysis of the proportion of predicted responses in the first session showed no significant age differences in model fits (P > 0.48, YA: Mean: 0.88, SD: 0.04; OA: Mean: 0.89, SD: 0.06), indicating that the model explained the choice behaviors of both age groups to a similar degree. Similarly, in the second session, there was no evidence for age differences in model fits (P = 0.81, YA: Mean: 0.88, SD: 0.13; OA: Mean: 0.86, SD: 0.15).
In addition to the analysis of the discount rates in Session 2 we also analyzed the (logit-transformed) overall percentage of delayed choices and (log-transformed) RTs. This was done separately for the factor delay (today—2 weeks, today—4 weeks) 2–4 weeks, 2–6 weeks) and the factor conflict (low conflict options, high conflict options). These data were analyzed using a mixed effects repeated measures ANOVA with the between-subjects factors age group and the within-subjects factors delay and conflict. For the following analyses, we define decision conflict effects as the differences in dependent variables (behavioral and fMRI data) between the high and the low conflict condition. To analyze associations between dependent variables we calculated Pearson’s correlation coefficients. Whenever necessary, the statistical significance of differences in correlation coefficients was determined using Fishers r-to-z transformation and relevant statistics are reported.
fMRI Data Analysis
FMRI data analyses were performed using AFNI_17.0.09 (Cox 1996) and SPM (SPM8; Welcome Department of Imaging Neuroscience). In order to avoid a normalization bias toward the anatomy of younger adults (Samanez-Larkin and D’Esposito 2008), the fMRI images were registered to a study-specific gray matter template using the DARTEL toolbox in SPM8 (Ashburner 2007). In an initial step, we registered the functional images to the individual high-resolution T1 image in AFNI using a local Pearson correlation cost function (Saad et al. 2009). Then functional and structural images of each participant were manually aligned with the SPM tissue probability maps and segmented into their tissue components (Ashburner and Friston 2005). The resulting gray and white matter images were used during the DARTEL procedure to create the study-specific gray matter template (Ashburner 2007; Harris et al. 2009). In addition to the template, the procedure results in so-called flow fields that parameterize the nonlinear deformations that are applied to match each individual image to the template. These flow fields were used to normalize the smoothed (Gaussian FWHM 6 mm) EPI data to an MNI-registered version of the template. For presentation purposes we display the functional data on the ICBM 452 T1 brain. The functional data were slice-time corrected to the second volume using Fourier interpolation and realigned using rigid-body 3D motion correction as implemented in AFNI. Transient spikes in the EPI data were removed using the AFNI program 3dDespike. Percent signal change was calculated for each voxel with respect to the mean activation across the time series. For each participant’s fMRI data, we performed a general linear model (GLM) analysis using 20 experimental regressors. Each regressor reflects a unique combination of 4 delays between rewards (today—2 weeks, today—4 weeks, 2 weeks, 4 weeks, 2–6 weeks) and 5% difference values between rewards (1.5%, IDP-1 SD, IDP, IDP + 1sD, 95%). In addition, each regressor modeled the onset of a choice option. The regressors were convolved with a canonical hemodynamic response function. Motion correction parameters were included in the GLM as regressors of no interest. In addition, we included RT and the subjective (discounted) value or the unsigned difference in subjective value between options as additional trial-wise regressors. The beta coefficients of the regressors of interest were subjected to a whole-brain mixed effects model analysis as implemented in the AFNI program 3dMVM. 3DMVM uses a multivariate modeling approach. This approach has the advantage that it does not limit the number of within subject factors of the ANOVA design and it allows the testing of quantitative covariates (Chen et al. 2014). The ANOVA design for the whole-brain analysis involved the between-subjects factor age group (younger vs. older adults), the within-subjects factors reward option (immediate reward vs. “only” delayed reward) and conflict (low conflict (1.5 %; 95% difference) vs. high conflict options (IDP + -1 SD)) as well as the random factor subject. Furthermore, the model involved the subject-wise covariates WM capacity as well the difference in discount functions between conflict conditions. In order to control for multiple comparisons, we used the AFNI program 3dFWHMx to estimate the smoothness of the data. The final estimated smoothness values (8.4556, 8.6613, 8.2446 at a voxel size of 3 × 3 × 4 mm) were then subjected to the updated version of the AFNI program AlphaSim. We determined that a corrected family-wise P-value of 0.05 is achieved with a minimum cluster size of 74 voxels at a P-value of <0.001. For activations that don’t meet this criterion we report the whole-brain significance value as determined using AlphaSim. For visualization purposes (bargraphs in Figs 4–6) we use functional regions of interest (ROI’s).
Results
Choice Data
Session 1
An analysis of the median discount (logK) values and the IDPs in “Session 1” showed no significant main effects of age group P’s > 0.26 (see Fig. 1B). As shown in Figure 1C, there was also no significant effect of age group on the decision noise parameter alpha (P = 0.62), the motor noise parameter epsilon (P = 0.11), or the proportion explained choices (P = 0.48).
Adjustment of Choice Options in Session 2
The adjustments of the choice options in Session 2 (based on the IDPs in Session 1) resulted in overall smaller reward magnitudes for the high than the low conflict condition (P < 0.001, η2 = 0.62). Importantly, however, there were no significant age differences in reward magnitudes (P’s > 0.12) (see Fig. S2B).
Session 2
The analysis of the discount rates in “Session 2” revealed a significant main effect of age group F1,48 = 9.99, P = 0.003, ηg2 = 0.14 and a significant age group × decision conflict interaction F1,48 = 11.42, P = 0.001, ηg2 = 0.05. Separate analyses for the 2 age groups showed significant effects of decision conflict in opposite directions in younger and older adults (P’s < 0.04, ηg2 > 0.05). Separate analyses for the 2 conflict conditions revealed a significant main effect of age group in the high conflict condition (P < 0.001, ηg2 = 0.21), but not the low conflict condition (P = 0.10). As shown in Figure 2A, when under high decision conflict, younger adults tend to discount reward less as a function of time. In contrast, older adults show higher discounting of delayed reward under high decision conflict. The analysis of the decision noise parameter (α) revealed greater decision noise in the high conflict than the low conflict condition (P < 0.001, ηg2 = 0.44) but no significant age differences (P = 0.38). For the motor noise () parameter, we found significant main effects of age group and conflict condition (P’s < 0.01, ηg2’s > 0.09), but no significant interaction between the 2 factors (P = 0.38) (see Supplementary Fig. S2C). These results suggest that older adults were overall more variable in their responses and that both groups showed noisier choice behavior under high than low decision conflict. Importantly, we found no significant effect of age group on the proportion of explained choices (P = 0.38), suggesting that the model explained the behavior of younger and older adults to a similar degree.
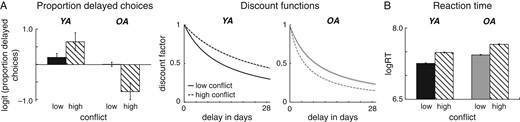
Behavioral data from the second (fMRI) session. (A) Double dissociation between age group and decision conflict in overall choice behavior (proportion delayed options) as well as delay discounting functions in the fMRI session. Older adults (shown in gray) show greater delay discounting under high than low decision conflict (dashed versus solid lines) whereas younger adults (in black) show the reverse pattern (less discounting under conflict). (B) RT (in seconds) as a function of age group and decision conflict. Both, age groups slow down on high compared to low conflict options (dashed versus solid bars). (C) Scatter plots for the correlation between WM and log-transformed discount factors in the high conflict condition in younger adults (shown in black) and older adults (shown in gray). In younger, but not in older adults greater WM performance is associated with lower discount functions under decision conflict.
Reaction Time
As displayed in Figure 2B older adults had slower RTs (logRTs) than younger adults, F1,48 = 6.48, P = 0.01, ηg2 = 0.11 Furthermore, across groups RTs were slower on high than low conflict trials, F1,48 = 103.74, P < 0.001, ηg2 = 0.17. However, younger and older adults did not differ in RT conflict effects (P = 0.78) (see Fig. 2B). To examine RT as a function of subjective decision conflict in the high conflict condition we used the unsigned difference between the subjective value of the delayed reward and the sooner reward and performed a tertile split for each individual. In line with our predictions, we found a significant main effect of value difference F2,94 = 19.81, P < 0.001, ηg2 = 0.02 across groups, as well as within each group (P’s < 0.01, ηg2’s > 0.01). Taken together, these findings show that smaller differences in value between reward options in the high conflict condition are associated with greater RTs (see Fig. 6B).
fMRI Data
As shown in Figure 3, a contrast between high and low decision conflict options revealed greater activity for high conflict options in areas associated with cognitive control, such as the right dorsolateral prefrontal cortex (dlPFC, −42,−48,−16) and the bilateral anterior cingulate cortex (ACC, −6,−21,40), (t-values > 4.19, P-values < 0.05, corrected). However, this contrast also revealed several areas associated with the default network that showed greater deactivation for high rather than low conflict options, such as the dorsal and ventromedial prefrontal cortex (dmPFC, −9,−51,28 vmPFC, 3,−51,4), the bilateral superior temporal gyrus (STG, 54,−51,23) and the posterior cingulate cortex (PCC, −10,48,33) (see deactivations (blue) in Fig. 3). For separate analyses for the 2 age groups please refer to Supplementary Figure S4.
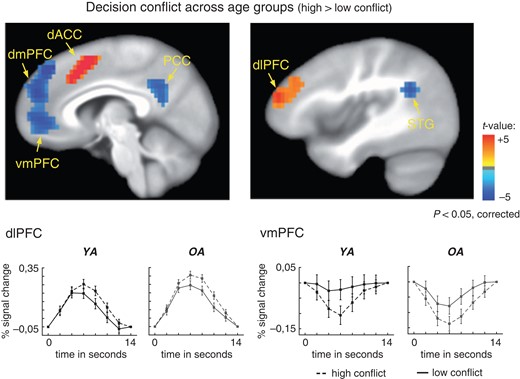
fMRI results across groups. “Upper panel”: Significant effects of decision conflict (t-statistics). Positive activations (shown in red) reflect greater activity in the high than the low conflict condition. We found greater activity for high conflict options in areas associated with cognitive control, such as the right dlPFC and the bilateral ACC. Deactivations (shown in blue) for high than low conflict options were observed in the dorso- and ventromedial PFC, the PCC, and the bilateral superior temporal gyrus. “Lower panel”: Time courses for activity in the dlPFC and vmPFC areas, shown separately for high (dashed lines) and low (solid lines) conflict conditions and the 2 age groups. Younger adults are shown in black, older adults are shown in gray. Error bars reflect the standard error of the mean (SE). The onset of the time course refers to the onset of the decision options.
As shown on Figure 4A the whole-brain analysis also revealed a significant interaction between age group × decision conflict in the bilateral striatum (Talairach coordinates: −11,13,5 (left); 12,12,6 (right), t-values > 3.4, P-values < 0.05, corrected). Separate analyses for the 2 conflict conditions revealed a significant main of effect of age group in the high conflict condition in left ventral striatum (Talairach coordinates: −17,16,−5, t-value > 3.4, P-value < 0.08, corrected). In contrast, no age differences were observed in low conflict condition (P > 0.05) (see Fig. 4B).
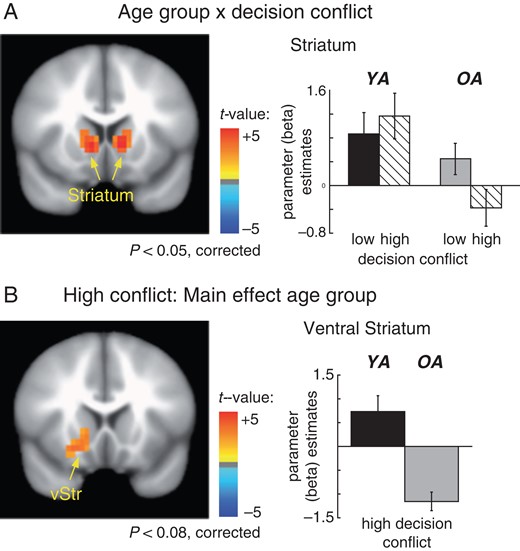
(A) Age group × conflict interaction in the striatum. Older adults (gray bar graphs) show a significant deactivation for high compared to low conflict options in the bilateral dorsal striatum. No such effect is observed in younger adults (black bar graphs). Error bars reflect the standard error of the mean (SE). (B) Separate analyses for the 2 decision conflict conditions showed hypoactivation in the ventral striatum for older compared to younger adults in the high conflict condition. No significant age differences were observed in the low conflict condition. Note that the bargraphs were determined using functional ROIs.
To investigate age differences in the degree to which subjects value the choice options we analyzed intra-individual correlations of BOLD activity with the subjective (discounted) value of the delayed reward option. The results showed a significant correlation between BOLD signal and subjective value in the right ventral striatum (Talairach coordinates: −9,−6,0), the left putamen (Talairach coordinates: 27,−9,0) and the left thalamus (Talairach coordinates: −15,−18,0) (t-value > 3.4, P-value < 0.05, corrected). As shown in Figure 5 ventral striatal activity reflects the (discounted) subjective value of the choice options across age groups. However, no significant age differences in value encoding are observed.
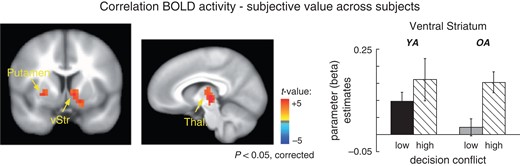
Correlations between subjective value and BOLD activity. Top: Across groups significant correlations were observed in the right ventral striatum, the left putamen and the left thalamus. Bottom: Mean activity in the right ventral striatum shown separately for younger adults (black) and older adults (gray) and the high and the low conflict condition (solid and dashed bars respectively). Note that the bargraphs were determined using functional ROIs.
In order to follow-up on the condition-based conflict analysis we analyzed trial-to-trial correlations of BOLD activity and decision conflict in the high conflict condition. For this purpose, we calculated the unsigned difference in subjective value between the (discounted) later reward and the sooner reward for each decision, assuming that decision conflict increases the more similar the values of the 2 options are. Consistent with the condition-based analysis we found significant age differences in the correlation of BOLD activity and subjective decision conflict in the left dorsal and ventral striatum (Talairach coordinates: left dStr: 15,−6,+8, left vStr: 6,−6,−12) (t-values > 3.4, P-values < 0.05, corrected, see Fig. 6A). Separate analyses for the 2 age groups showed a significant correlation between BOLD activity and value differences in the left ventral striatum in younger (t-value > 3.4, P-value < 0.05, corrected), but not in older adults (P-values < 0.05) (see Fig. S7). Taken together, these findings suggest that in younger adults activity in the ventral striatum reflects fluctuations in subjective decision conflict. No such association is observed in older adults.

(A) Correlations between unsigned differences in subjective value between reward options and BOLD activity in the high conflict condition. Left: Greater intra-individual correlation between decision conflict (the unsigned differences in subjective value between reward options) and BOLD activity in the left dorsal and ventral striatum in younger than older adults. Note that the bargraphs were determined using functional ROIs. (B) RT as a function of the (unsigned) difference in subjective value between reward options in the high conflict condition. Greater decision conflict (smaller differences in the value) in the high conflict condition are associated with higher RT in both age groups.
Discussion
Decision conflicts are common in our daily life and the ability to resolve them is important for adaptive decision behavior across the lifespan. In this study, we investigated how age-related changes in brain functions during adulthood may be associated with the ability to solve intertemporal decision conflicts using functional MRI.
Age-Related Changes in Delay Discounting Under Decision Conflict
Based on previous findings (Green et al. 1994; Reimers et al. 2009; Löckenhoff et al. 2011; Li et al. 2013), we expected reduced discount rates for older rather than younger adults in the first session. As shown in Figure 1B this was not the case. This (no-) result adds to a somewhat mixed pattern of findings on age differences in delay discounting. Several recent studies showed reduced discounting in older than younger adults (Green et al. 1994; Reimers et al. 2009; Löckenhoff et al. 2011; Eppinger et al. 2012; Li et al. 2013). However, other studies showed no age differences in discount rates (Green et al. 1996; Read and Read 2004; Samanez-Larkin et al. 2011; Seaman et al. 2016). It could be that some of the inconsistencies in the current data are due to the specific choice sets used in the different studies. For example, the Eppinger et al. (2012) study also involved decisions between only delayed options, which have been shown affect choice preferences (Laibson 1997). Moreover, the findings described below suggest that decision preferences in different age groups depend on how close the choice sets are to the IDPs of the individuals. Finally, many of the aforementioned studies involved small and presumably selective samples. Future studies should aim for large-scale assessments to circumvent these problems (see e.g., Rutledge et al. (2016)).
In line with our predictions, older adults showed steeper discount rates under high compared to low decision conflict, indicating that enhanced control demands lead to a preference for sooner reward in the elderly (see Fig. 2A). Interestingly, however, younger adults showed the reverse effect. As displayed in Figure 2A they discounted reward less under high decision conflict. One interpretation for the decision-making bias in older adults could be that it results from age-related limitations in cognitive control abilities (West 1996; Braver et al. 2001; Kray et al. 2005; Eppinger et al. 2007). This interpretation would be consistent with recent findings that point to an association between cognitive control deficits and discount rates in older adults (Sasse et al. 2017). However, it could also be argued that the observed decision bias in the elderly reflects a decision heuristic. That is, when faced with high demands on cognitive control older adults may default to choosing the sooner reward (Ericson et al. 2015).
The bias towards delayed reward options in younger adults could be interpreted in terms of an over-deliberation. That is, under decision conflict younger adults may engage in a more reflective, controlled decision mode. Support for this interpretation comes from research showing that under greater demands on cognitive control younger adults engage more in future episodic thinking, which has been associated with reduced discount rates (Peters and Büchel 2010a; Sasse et al. 2017). Consistent with this idea we found a strong positive relationship between discount rates under high decision conflict and working memory in younger, but not in older adults (see Fig. S3). That is, it is the younger adults with high fluid abilities who show this shift in discount rates under increased control demands (Shamosh and Gray 2008).
Neural Systems Involved in Delay Discounting
The fMRI analysis revealed greater BOLD activity for high rather than low decision conflict in cognitive control areas, such as the dlPFC, ACC, and parietal cortex. The activity maps shown in Figure 3 reveal a strong overlap with conflict-related activity in cognitive tasks (Carter et al. 1998; Kerns et al. 2004; Kool and Botvinick 2014). Moreover, they overlap strongly with areas involved in mediating deliberate decisions during delay discounting (McClure et al. 2004; Figner et al. 2010; Wesley and Bickel 2014). However, the contrast also revealed significant “deactivations” for high rather than low conflict options in areas such as the vmPFC, the PCC and the medial temporal gyrus (see Fig. 3). Previous studies suggest that activity in these para-limbic areas contributes to an immediacy bias (McClure et al. 2004, 2007) or reflects the subjective value of decision options (Kable and Glimcher 2007). Yet, there are also alternative interpretations: For example, this activation pattern shows a striking overlap to the so-called default network (Andrews-Hanna 2012; Scheres et al. 2015). Results of previous studies indicate that default network activity is associated with goal-directed attention towards internal states (Buckner and Carroll 2007; Spreng and Grady 2009; Andrews-Hanna 2012; Grady et al. 2012). Based on these findings it could be argued that the ability to resolve decision conflicts depends on the degree to which subjects can guide internal attention to disambiguate conflicting value representations. Interestingly, activity in the cognitive control and the default network was not related to decision preferences (such as discount functions). We did, however, observe strong correlations of activity in both networks and RT conflict effects (see Fig. S5). This pattern of findings may result from the specific choice situations created in the current study. However, the findings may also suggest that the engagement of the 2 networks has less to do with “how” participants decide but with “how long” it takes them to do so.
The fMRI analysis also showed a significant interaction between age group and decision conflict in the bilateral ventral striatum (see Fig. 4A). Separate analyses for the 2 conflict conditions revealed a significant hypoactivation of the left ventral striatum for older compared to younger adults in the high but not the low conflict condition (Fig. 4B). Given absence of differences in prefrontal activity, these findings seem inconsistent with the idea that steeper discount rates under decision conflict in older adults result from cognitive control deficits. Rather, they support the view that when facing enhanced demands on a limited resource (cognitive control) older adults may default to choosing the sooner option. According to this interpretation the striatal hypoactivation may reflect a shift from a deliberate processing of value differences between options to a decision strategy of choosing the sooner option under decision conflict. Consistent with this view, greater discounting of delayed reward under decision conflict in older adults is associated with reduced RTs suggesting that older adults who engage in this strategy spend less time on deliberating the 2 options. The question is what motivates these shifts in decision strategies. In the interpretation above we argue that older adults are aware of their limitations in cognitive control and therefore converge on strategies that simplify decision-making under high conflict. Alternatively, it could be argued that independently of their cognitive control limitations, older adults may be less willing to engage in cognitively demanding decision processes and this is why they show a reduced recruitment of the ventral striatum under decision conflict. This view would be consistent with an increasing literature that suggests that value representations in the ventral striatum are modulated by cognitive effort (Botvinick et al. 2009; Croxson et al. 2009; Prevost et al. 2012; Treadway et al. 2012).
Neural Representations of Subjective Value in Younger and Older Adults
To investigate age-related changes in the neural representations of subjective value, we analyzed intra-individual correlations between BOLD activity and the discounted value of the delayed reward option in the 2 age groups. This analysis revealed a positive association between the subjective value and BOLD activity in the right ventral striatum, the left putamen and left thalamus (see Fig. 5). The value-related ventral striatal activity is consistent with several reports in the literature (Kable and Glimcher 2007; Peters and Büchel 2010a). However, most of these studies showed more consistent value signals in the medial prefrontal cortex. The reason for this inconsistency is unclear. It has been argued that some of the ventral striatal value signals actually reflect prediction errors because options with high subjective value may cause positive expectancy violations, whereas options with low subjective value may cause the opposite effect (Hare et al. 2009; Peters and Büchel 2010b). The design of the current study might have enhanced such an effect because in addition to the options around the IDP it involves options in which the magnitude of the delayed reward varies strongly (1.5%/95% difference between rewards in the low conflict condition). This is in contrast to most previous study designs which tried to continuously cover a range of reward differences. However, it could also be argued that the age comparison added variability that obscured the medial prefrontal value signals. Indeed, as shown in Figure S6 separate analyses for the 2 age groups showed significant value signals in the medial prefrontal cortex in younger adults, similar to what has beed observed in previous studies (Kable and Glimcher 2007).
Surprisingly, we did not find significant effects of decision conflict on subjective value representations. To address this question more specifically, we performed an additional fMRI analysis using the unsigned difference between the discounted subjective value of the delayed reward and the value of the sooner reward in the high conflict condition as a parametric regressor. Based on the literature we predicted that greater subjective decision conflict should be reflected in changes in ventral striatal activity as well as RTs (Botvinick et al. 2009; Croxson et al. 2009; Prevost et al. 2012; Treadway et al. 2012). In line with these predictions we found a positive correlation between ventral striatal BOLD activity and subjective value differences in younger, but not in older adults (see Fig. 6A). Furthermore, in both age groups, smaller value differences were associated with higher RTs (see Fig. 6B), indicating that these decisions are perceived as cognitively more demanding. Taken together, our results show that in addition to the discounted value of reward, ventral striatal activity also tracks differences in subjective value (Botvinick et al. 2009; Croxson et al. 2009; Prevost et al. 2012; Treadway et al. 2012). However, this is only the case for younger but not for older adults, indicating that aging is associated with changes in value representations under high demands on cognitive control.
Results of several previous studies using reward learning paradigms suggest that decision-making deficits in older adults may result from a reduced updating of reward value representations in the ventral striatum. Furthermore, these deficits have been linked to diminished dopaminergic projections from the midbrain to these areas (Dreher et al. 2008; Eppinger et al. 2011; Hämmerer and Eppinger 2012). In line with these findings it could be argued that in the high conflict condition the demands on the precise representation of value differences are increased. Deficits in this ability in older adults might be reflected in diminished ventral striatal value signals as well as the observed decision bias in the elderly. That is, older adults who have difficulties representing value differences might default to choosing the sooner option under high demands on cognitive control. Alternatively, it could be argued that the observed effects in the ventral striatum are the consequence rather than the source of the observed decision biases. That is, as suggested above, under high demands on cognitive control older adults may shift to simpler, more present-oriented decision strategies (Peters et al. 2007; Samanez-Larkin and Knutson 2015). These changes of decision strategies could lead to the observed decoupling of ventral striatal activity and subjective value. Future studies should try to address this hypothesis more directly by explicitly manipulating the degree to which participants can (or should) engage in decision heuristics.
To summarize, our findings show that decision conflict has differential effects on intertemporal choice preferences in younger and older adults. In younger adults, decision conflict leads to reduced discounting of delayed reward and lower discount rates in the young are associated with higher WM abilities. These findings indicate that under high decision conflict younger adults may engage in a more reflective, controlled decision mode that reflects individual differences in fluid abilities. In older adults, decision conflict leads to enhanced delay discounting as well as hypoactivation in the ventral striatum. These results suggest that under high demands on cognitive control older adults get more present-oriented and this decision bias is reflected in diminished ventral striatal activity. Model-based fMRI analyses showed that activity in the ventral striatum tracks the subjective (discounted) value of reward in both age groups. Moreover, in younger, but not in older adults, ventral striatal activity correlated with differences in subjective value between decision options. These findings show that in younger adults activity in the ventral striatum reflects the enhanced cognitive demands of making intertemporal choices under high decision conflict. In contrast, in older adults ventral striatal activity seems to be decoupled from differences in subjective value, indicating that they may engage in simplifying decision strategies when under increased demands on cognitive control.
Funding
This research was funded by the Bundesministerium für Bildung und Forschung (Bernstein Focus Neuronal Basis of Learning Grants Nos. FKZ 01GQ0913 and FKZ 01GQ1313, awarded to S.C.L. and H.R.H.) as well as the Deutsche Forschungsgemeinschaft (SFB 2 940, subproject B7, awarded to B.E.).
Notes
Conflict of Interest: The authors declare no competing financial interests.