-
PDF
- Split View
-
Views
-
Cite
Cite
Tarek K. Rajji, Reza Zomorrodi, Mera S. Barr, Daniel M. Blumberger, Benoit H. Mulsant, Zafiris J. Daskalakis, Ordering Information in Working Memory and Modulation of Gamma by Theta Oscillations in Humans, Cerebral Cortex, Volume 27, Issue 2, February 2017, Pages 1482–1490, https://doi.org/10.1093/cercor/bhv326
- Share Icon Share
Abstract
Ordering information is a critical process underlying several cognitive functions, especially working memory. Theta phase-gamma amplitude coupling is regarded as a neurophysiological representation of ordering information during working memory performance. However, direct evidence has been lacking in humans. Seventy healthy subjects performed the N-back task, a working memory task that tests ordering information at 3 different levels of difficulties and with 3 different types of trials. Using electroencephalography (EEG) during N-back performance, theta–gamma coupling was assessed during response trials. Multivariate general linear model (GLM) and discriminant analysis were used to assess coupling and theta and gamma power across the N-back conditions and the trial types. During the N-back trials that required ordering of information, N-back condition had independent effects on coupling and on theta and gamma power, with equal contributions among these 3 variables. Theta–gamma coupling contribution declined significantly on the trials that did not require ordering and was intermediate on trials that favored but not necessarily required ordering. Our findings demonstrate for the first time the role of theta–gamma coupling as a mechanism that supports ordering information. They also highlight the potential of using theta–gamma coupling as a neurophysiological marker of brain function in health or disease states.
Introduction
Ordering information is a critical process that subserves several cognitive functions (Lashley 1951; Young 1962), including working memory. During working memory, items of information are maintained over a short time interval and manipulated online during this interval (Baddeley 1986). This manipulation often requires specific ordering of this information (Marshuetz 2005). Ordering information during working memory depends on top-down control processes that can be represented neurophysiologically as brain oscillations (Engel et al. 2001). Computational and animal studies demonstrate that while high-frequency gamma oscillations represent individual items of information and low-frequency theta oscillations provide the neurophysiologic substrate for the time interval during which these items are represented, the modulation of gamma oscillations by the phase of theta (theta–gamma coupling) codes for the ordering of the items during this time interval (Tort et al. 2009; Shirvalkar et al. 2010; De Almeida et al. 2012; Lisman and Jensen 2013). In humans, while some studies found an association between theta–gamma coupling and working memory (Mormann et al. 2005; Canolty et al. 2006; Sauseng et al. 2009; Axmacher et al. 2010; Park et al. 2013), the association between theta–gamma coupling and ordering of information is yet to be established.
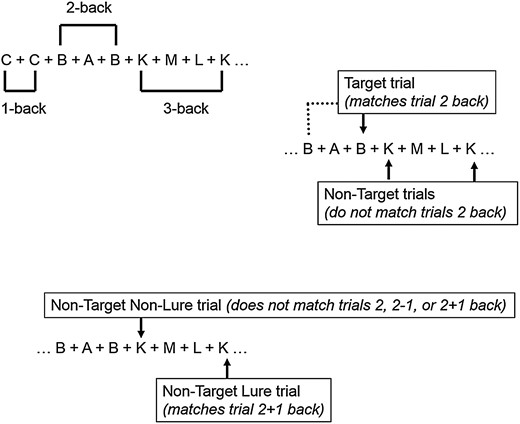
N-back Task: This figure illustrates the N-back task and its different N-back conditions (1, 2, and 3-back) (Upper Panel) and different trial types (Target, Non-Target Lure, and Non-Target trials on a 2-back condition (Middle and Lower Panels).
In brief, for good performance on the N-back task, recollection of the letters order is necessary during Target trials, not necessary but advantageous during Non-Target Lure trials, and not necessary nor advantageous during Non-Target Non-Lure trials. Thus, in this study, we hypothesized that: 1) theta–gamma coupling as captured prior to responding to each trial will be a significant factor in predicting correct performance on Target trials and that its prediction will be as large and independent of gamma and theta oscillations contributions and 2) theta–gamma coupling would be high during Target trials, lower during Non-Target Lure trials and lowest during Non-Target Non-Lure trials.
Materials and Methods
Subjects and Working Memory Task
All subjects completed a written informed consent approved by the local Research Ethics Board before starting the study. Subjects were screened using the Personality Assessment Screener (Morey 1997) and excluded if they had any CNS disorder or medical disorder that affects cognition or were on any substance that affects cognition.
As described earlier, the N-back task (Gevins and Cutillo 1993) is a continuous working memory task that requires subjects to determine whether a stimulus presented on a monitor is the same as, or different from a stimulus presented N trials back. When the stimulus is the same, the trial is labeled as a Target trial. When the stimulus is different, the trial is labeled as a Non-Target trial. N varies among 1, 2, and 3, thus assessing different levels of working memory spans or loads. Some Non-Target trials match the N + 1 or N − 1 trials back. These trials are referred to as Non-Target Lure trials. The Non-Target trials that do not match N + 1 or N − 1 trials are referred to as Non-Target Non-Lure trials (Harbison et al. 2011). The N-back task also includes a 0-back condition during which subjects respond to every letter presented. This condition assesses pure attention to the task. The N-back stimuli consist of black capital letters presented for 250 ms followed by a delay period of 3000 ms during which the subject is required to respond. Finally, a plus sign is presented to indicate the end of the current trial. In the 1- and 2-back conditions, stimuli are presented continuously for 15 min. In the 3-back condition, stimuli are presented for 30 min to ensure that the frequency of Target trials in this condition is comparable with those presented in the 1- and 2-back conditions. The proportion of Target trials in 1-, 2-, and 3-back conditions was 0.23, 0.16, and 0.15, respectively. In this analysis, coupling was assessed during the interval between the presentation of each stimulus and the time when the subjects responded. We think that this is the timeframe during which representing information in an orderly manner is critical and hence coupling is high for subjects who respond correctly.
EEG Recording
While performing the N-back task, EEG was recorded using a 64-channel Synamps 2 EEG system and the 10–20 montage system. An EEG cap was used to record the cortical signals from the scalp, and 4 electrodes were placed on the outer side of each eye, and above and below the left eye to correct for eye movement artifacts. Electrodes were referenced to an electrode posterior to Cz. EEG signals were recorded using DC and a low pass filter of 100 Hz at 20-kHz sampling rate (Daskalakis et al. 2008).
EEG Data Processing
EEG recordings were processed offline using MATLAB (The MathWorks, Inc.) and EEGLAB toolbox. First, data were down sampled to 1 kHz and filtered by using second order, Butterworth, zero-phase shift 1–55 Hz band pass filter. Second, EEG data were segmented −1400 ms to +3100 ms relative to the stimulus onset and baseline corrected with respect to the pre-stimulus interval (1400 ms to 5 ms prior to the stimulus onset). Third, an electrodes-by-trials matrix of ones was created and assigned a value of zero if an epoch had: 1) amplitude larger than ±150 μV, 2) power spectrum that violated 1/f power law, or 3) standard deviation 3 times larger than the average of all trials. Fourth, an electrode was rejected if its corresponding row had >60% of columns (trials) coded as zeros. Fifth, an epoch was removed if its corresponding column had >20% of rows (electrodes) coded as zeros. Sixth, epochs were manually inspected and trials containing other anomalous irregularities were removed. Seventh, an independent component analysis (EEGLAB toolbox; Infomax algorithm) was performed to remove eye-blink traces, muscle artifacts, and other noise from the EEG data. Finally, data were re-referenced to the average mastoid. To demonstrate the quality of EEG recordings and in particular the P300 component in response to the letter presentation, we generated and show Event-Related Potentials (ERPs) for each Trial Type and within each N-back condition.
Theta–Gamma Coupling
Coupling was analyzed for the trials that were responded to correctly across all N-back conditions and trial types. Following published methods (Axmacher et al. 2010; Tort et al. 2010), we first filtered the raw EEG signal for theta (4–7 Hz) and gamma (30–50 Hz) frequency ranges with second-order zero-phase shift. Then, we calculated the time series for theta phase and gamma amplitude using the Hilbert transform. Next, we created a concatenated signal of 5000 ± 150 ms using randomly selected epochs for each trial type, at each electrode. Each epoch started with the stimulus onset and ended at the time of response. The rationale for generating a concatenated signal of 5000 ms was that MI, the index of theta–gamma coupling, is highly dependent on the length of the signal; the shorter the signal the higher the MI (Tort et al. 2010; Voytek et al. 2013; Aru et al. 2015). Thus, given that epoch lengths vary depending on the N-back condition and trial type, creating signals of similar lengths across all trials is critical to compare MI across them. Further, MI is artificially inflated at short signals (Tort et al. 2010), that is, in the range of time to respond. Thus, we used a 5000-ms concatenated signal to ensure stability of MI. Mean numbers of epochs used to generate a concatenated signal were the following (SD): 1-back Target trials = 10.45 (2.93); 2-back Target trials = 8.5 (2.5); 3-back Target trials: 8.23 (2.4); 1-back Non-Target Lure trials = 11.22 (3.2); 2-back Non-Target Lure trials = 12.05 (3.5); 3-back Non-Target Lure trials: 9.44 (1.1); 1-back Non-Target Non-Lure trials = 11.34 (2.1); 2-back Non-Target Non-Lure trials = 10.62 (1.9); 3-back Non-Target Non-Lure trials: 10.26 (3.5).
Using this equation, higher coupling is associated with lower entropy H(P), which in turn results in a higher MI value.
MI for each electrode was calculated, and then an average of MI across the right and left frontal electrode (AF3/4, F7/8, F3/4, F1/2, and Fz) was used in the statistical analysis. All MI values are shown in topoplots.
Surrogate Data Theta–Gamma Coupling
To test whether the real MI values are statistically significant, MI values based on surrogate data for each N-back condition (1, 2, and 3-back) and each Trial Type (Target, Non-Target Lure, and Non-Target Non-Lure) were generated. To generate the surrogate data, a phase-scrambling algorithm was used (Axmacher et al. 2010). The ERP time-series responses were converted into complex frequency domain using fast Fourier transform. While the amplitude kept constant, the phase of the time series was randomized by adding a uniform distributed random values [−π; π]. Then, by applying inverse FFT, data were back transformed into the time domain. A total of 1000 iterations were carried out to generate an empirical distribution under the null hypothesis of no coupling.
Theta and Gamma Powers
Once the signal was filtered as described earlier into the appropriate bands, the power spectrum was calculated from squared amplitude of Hilbert transformed signal. Group average of induced spectral power analysis for all frontal electrodes was calculated and used in further analysis.
Statistical Analysis
To assess performance on the various N-back conditions, repeated-measures GLM was performed using accuracy on Target trials and followed by post-hoc comparisons across conditions and against chance performance of 50% correct, with Bonferroni adjustment for multiple comparisons. To assess the relationship between working memory and theta–gamma coupling and its independence of the relationships between working memory and theta or gamma powers, a multivariate GLM was performed and followed up with discriminant analysis. The dependent variables were theta–gamma coupling, and theta and gamma power. The independent variable was the N-back condition. These analyses were performed on Target, Non-Target Lure, and Non-Target Non-Lure Correct trials.
To assess the relationship between Trial Type and theta–gamma coupling, as well as theta and gamma power, we also performed multivariate GLM, followed up with discriminant analysis with theta–gamma coupling, and theta and gamma power as dependent variables. However, the independent variable was the Trial Type: Target, Non-Target Lure, and Non-Target Non-Lure. These analyses were performed on 1, 2, and 3-back condition.
Finally, we assessed the relationship between Trial Type and Response Time during 1, 2, and 3-back conditions to determine whether the pattern observed with MI is also observed with Reaction Time. Arguably, recollection of order requires more cognitive effort and therefore more time to respond suggesting that Response Time during Target or Non-Target Lure trials should be longer than during Non-Target Non-Lure trials. To assess this relationship, we performed univariate GLM with Response Time as the dependent variable and Trial Type (Target, Non-Target Lure, and Non-Target Non-Lure) as the independent variable, during each of 1, 2, and 3-back condition, followed by post-hoc analyses with Bonferroni adjustments.
All analyses were conducted using the accepted epochs as described in the EEG data-processing section above. Mean numbers of accepted epochs were the following (SD) [%]: 1-back Target trials = 35 (3) [76.0%]; 2-back Target trials = 26 (9) [83.9%]; 3-back Target trials: 32 (11) [54.2%]; 1-back Non-Target Lure trials = 59 (18) [92.2%]; 2-back Non-Target Lure trials = 62 (10) [88.6%]; 3-back Non-Target Lure trials: 41 (15) [87.2%]; 3-back Non-Target Lure (N − 1) trials = 43 (12) [84.3%]; 1-back Non-Target Non-Lure trials = 52 (8) [89.7%]; 2-back Non-Target Non-Lure trials = 51 (13) [87.9%]; 3-back Non-Target Non-Lure trials: 141 (22) [92.8%].
After generating surrogate MI values for each subject, paired-samples t-tests were used to compare mean MI at each N-back condition and Trial Type with corresponding surrogate data mean MI.
Results
Performance on the N-back Task
Seventy healthy subjects (mean age = 31.1, SD = 13.3, F = 32 [45.7%]) performed the N-back task while EEG was recorded. N-back condition had a significant effect on performance on Target trials across 1-, 2-, and 3-back condition (V = 0.40, F2,68 = 22.69, P < 0.00001). Post-hoc analyses revealed that accuracies differed significantly across conditions. Mean percent correct scores on Target trials were the following (SD): 1-back = 78.3% (12.4); 2-back = 70.8% (19.14); and 3-back: 57.6% (25.5). All performances were above chance level of 50% (P [1- and 2-back] < 0.0001; P [3-back] = 0.015). Performance on 0-back condition was 100% correct.
Testing Whether and to What Extent Theta–Gamma Coupling Predicts Performance Independent of Gamma or Theta Powers on Different Trial Types
Starting with the Target Correct trials, which are dependent on correct ordering of information, we found that there was a significant effect of N-back condition on theta–gamma coupling, and theta and gamma powers (V = 0.26, F6,398 = 10.04, P < 0.00001). Follow-up discriminant analysis revealed 2 discriminant functions that, in combination, significantly differentiated the N-back conditions. We also found that the first function explained 74.7% of the variance. On this function, theta–gamma coupling, theta power, and gamma power loaded comparably (Table 1).
Discriminant analysis to assess the relationships among theta–gamma coupling, and gamma and theta power, in predicting performance on the different N-back trial types
Function . | Variance (%) . | Canonical R2 . | Wilks' Λ . | χ2 (df) . | P . | r theta–gamma coupling . | r theta power . | r gamma power . |
---|---|---|---|---|---|---|---|---|
Target trials | ||||||||
1 | 74.7 | 0.19 | — | — | — | 0.57 | 0.42 | 0.58 |
2 | 25.3 | 0.07 | 0.93 | 15.18 (2) | 0.001 | −0.40 | 0.90 | −0.36 |
1 + 2 | 100 | — | 0.75 | 56.97 (6) | <0.0001 | — | — | — |
Non-target non-lure trials | ||||||||
1 | 95.4 | 0.11 | — | — | — | 0.31 | 0.75 | 0.66 |
2 | 4.6 | 0.006 | 0.99 | 1.22 (2) | 0.54 | — | — | — |
1 + 2 | 100 | — | 0.89 | 25.03 (6) | <0.0001 | — | — | — |
Non-target lure trials | ||||||||
1 | 96.2 | 0.15 | — | — | — | 0.55 | 0.59 | 0.53 |
2 | 3.8 | 0.01 | 0.99 | 1.38 (2) | 0.50 | — | — | — |
1 + 2 | 100 | — | 0.85 | 33.95 (6) | <0.0001 | — | — | — |
Function . | Variance (%) . | Canonical R2 . | Wilks' Λ . | χ2 (df) . | P . | r theta–gamma coupling . | r theta power . | r gamma power . |
---|---|---|---|---|---|---|---|---|
Target trials | ||||||||
1 | 74.7 | 0.19 | — | — | — | 0.57 | 0.42 | 0.58 |
2 | 25.3 | 0.07 | 0.93 | 15.18 (2) | 0.001 | −0.40 | 0.90 | −0.36 |
1 + 2 | 100 | — | 0.75 | 56.97 (6) | <0.0001 | — | — | — |
Non-target non-lure trials | ||||||||
1 | 95.4 | 0.11 | — | — | — | 0.31 | 0.75 | 0.66 |
2 | 4.6 | 0.006 | 0.99 | 1.22 (2) | 0.54 | — | — | — |
1 + 2 | 100 | — | 0.89 | 25.03 (6) | <0.0001 | — | — | — |
Non-target lure trials | ||||||||
1 | 96.2 | 0.15 | — | — | — | 0.55 | 0.59 | 0.53 |
2 | 3.8 | 0.01 | 0.99 | 1.38 (2) | 0.50 | — | — | — |
1 + 2 | 100 | — | 0.85 | 33.95 (6) | <0.0001 | — | — | — |
Discriminant analysis to assess the relationships among theta–gamma coupling, and gamma and theta power, in predicting performance on the different N-back trial types
Function . | Variance (%) . | Canonical R2 . | Wilks' Λ . | χ2 (df) . | P . | r theta–gamma coupling . | r theta power . | r gamma power . |
---|---|---|---|---|---|---|---|---|
Target trials | ||||||||
1 | 74.7 | 0.19 | — | — | — | 0.57 | 0.42 | 0.58 |
2 | 25.3 | 0.07 | 0.93 | 15.18 (2) | 0.001 | −0.40 | 0.90 | −0.36 |
1 + 2 | 100 | — | 0.75 | 56.97 (6) | <0.0001 | — | — | — |
Non-target non-lure trials | ||||||||
1 | 95.4 | 0.11 | — | — | — | 0.31 | 0.75 | 0.66 |
2 | 4.6 | 0.006 | 0.99 | 1.22 (2) | 0.54 | — | — | — |
1 + 2 | 100 | — | 0.89 | 25.03 (6) | <0.0001 | — | — | — |
Non-target lure trials | ||||||||
1 | 96.2 | 0.15 | — | — | — | 0.55 | 0.59 | 0.53 |
2 | 3.8 | 0.01 | 0.99 | 1.38 (2) | 0.50 | — | — | — |
1 + 2 | 100 | — | 0.85 | 33.95 (6) | <0.0001 | — | — | — |
Function . | Variance (%) . | Canonical R2 . | Wilks' Λ . | χ2 (df) . | P . | r theta–gamma coupling . | r theta power . | r gamma power . |
---|---|---|---|---|---|---|---|---|
Target trials | ||||||||
1 | 74.7 | 0.19 | — | — | — | 0.57 | 0.42 | 0.58 |
2 | 25.3 | 0.07 | 0.93 | 15.18 (2) | 0.001 | −0.40 | 0.90 | −0.36 |
1 + 2 | 100 | — | 0.75 | 56.97 (6) | <0.0001 | — | — | — |
Non-target non-lure trials | ||||||||
1 | 95.4 | 0.11 | — | — | — | 0.31 | 0.75 | 0.66 |
2 | 4.6 | 0.006 | 0.99 | 1.22 (2) | 0.54 | — | — | — |
1 + 2 | 100 | — | 0.89 | 25.03 (6) | <0.0001 | — | — | — |
Non-target lure trials | ||||||||
1 | 96.2 | 0.15 | — | — | — | 0.55 | 0.59 | 0.53 |
2 | 3.8 | 0.01 | 0.99 | 1.38 (2) | 0.50 | — | — | — |
1 + 2 | 100 | — | 0.85 | 33.95 (6) | <0.0001 | — | — | — |
In contrast, on the Non-Target Non-Lure trials where theta–gamma coupling is neither required nor advantageous, we found different relationships. We found a significant effect of N-back condition on theta–gamma coupling, and theta and gamma powers (V = 0.12, F6,412 = 4.19, P < 0.00001. Follow-up discriminant analysis also revealed 2 discriminant functions that, in combination, significantly differentiated the N-back conditions. We also found that the first explained 95.4% of the variance. However, theta–gamma coupling load was <50% of theta power or gamma power load on this function (Table 1).
Different contributions of theta–gamma coupling based on different needs for information ordering are further supported by our analysis of Non-Target Lure trials on which theta–gamma coupling is advantageous for good performance. Given that for 1-back and 2-back conditions, Lure trials match N + 1 and not N − 1, we chose the N + 1 Lure trials for the 3-back condition too. Again, we found that there was a significant effect of N-back condition on theta–gamma coupling, and theta and gamma powers (V = 0.27, F6,400 = 10.34, P < 0.00001). We also found that follow-up discriminant analysis revealed 2 discriminant functions that, in combination, significantly differentiated the N-back conditions, with the first function explaining 96.2% of the variance. However, and in line with what we found with the Target trials rather than the Non-Target Non-Lure trials, we found that theta–gamma coupling, theta power, and gamma power loaded comparably on this first function (Table 1).
Testing Whether Theta–Gamma Coupling, and Theta and Gamma Powers, Differentiate among Target, Non-Target Lure, and Non-Target Non-Lure Correct Trials within Each N-back Condition
While the above analyses demonstrate that theta–gamma coupling is an independent and strong predictor of correct performance on N-back trials that require or favor ordering of information (Target and Non-Target Lure trials), and independent but less meaningful on trials that do not require such ordering (Non-Target Non-Lure trials), we wanted to test whether theta–gamma coupling differentiated among these trials types given their different demands for ordering of information.
Discriminant analysis to assess the relationships among theta–gamma coupling, and gamma and theta power, in differentiating N-back trial types across all N-back conditions
Function . | Variance (%) . | Canonical R2 . | Wilks’ Λ . | χ2 (df) . | P . | r theta–gamma coupling . | r theta power . | r gamma power . |
---|---|---|---|---|---|---|---|---|
1-back | ||||||||
1 | 92.4 | 0.21 | — | — | — | 0.72 | 0.11 | 0.67 |
2 | 7.6 | 0.02 | 0.98 | 4.51 (2) | 0.11 | — | — | — |
1 + 2 | 100 | — | 0.77 | 53.46 (6) | <0.0001 | — | — | — |
2-back | ||||||||
1 | 99.5 | 0.51 | — | — | — | 0.77 | 0.24 | 0.61 |
2 | 0.5 | 0.005 | 0.99 | 0.99 (2) | 0.61 | — | — | — |
1 + 2 | 100 | — | 0.49 | 148.0 (6) | <0.0001 | — | — | — |
3-back | ||||||||
1 | 100 | 0.38 | — | — | — | 0.65 | 0.27 | 0.60 |
2 | 0 | 0.00 | 1.00 | 0.05 (2) | 0.98 | — | — | — |
1 + 2 | 100 | — | 0.62 | 95.58 (6) | <0.0001 | — | — | — |
3-back with Lure as N − 1 | ||||||||
1 | 99.4 | 0.27 | — | — | — | 0.66 | 0.30 | 0.63 |
2 | 0.6 | 0.002 | 1.00 | 0.47 (2) | 0.79 | — | — | — |
1 + 2 | 100 | — | 0.73 | 62.42 (6) | <0.0001 | — | — | — |
Function . | Variance (%) . | Canonical R2 . | Wilks’ Λ . | χ2 (df) . | P . | r theta–gamma coupling . | r theta power . | r gamma power . |
---|---|---|---|---|---|---|---|---|
1-back | ||||||||
1 | 92.4 | 0.21 | — | — | — | 0.72 | 0.11 | 0.67 |
2 | 7.6 | 0.02 | 0.98 | 4.51 (2) | 0.11 | — | — | — |
1 + 2 | 100 | — | 0.77 | 53.46 (6) | <0.0001 | — | — | — |
2-back | ||||||||
1 | 99.5 | 0.51 | — | — | — | 0.77 | 0.24 | 0.61 |
2 | 0.5 | 0.005 | 0.99 | 0.99 (2) | 0.61 | — | — | — |
1 + 2 | 100 | — | 0.49 | 148.0 (6) | <0.0001 | — | — | — |
3-back | ||||||||
1 | 100 | 0.38 | — | — | — | 0.65 | 0.27 | 0.60 |
2 | 0 | 0.00 | 1.00 | 0.05 (2) | 0.98 | — | — | — |
1 + 2 | 100 | — | 0.62 | 95.58 (6) | <0.0001 | — | — | — |
3-back with Lure as N − 1 | ||||||||
1 | 99.4 | 0.27 | — | — | — | 0.66 | 0.30 | 0.63 |
2 | 0.6 | 0.002 | 1.00 | 0.47 (2) | 0.79 | — | — | — |
1 + 2 | 100 | — | 0.73 | 62.42 (6) | <0.0001 | — | — | — |
Discriminant analysis to assess the relationships among theta–gamma coupling, and gamma and theta power, in differentiating N-back trial types across all N-back conditions
Function . | Variance (%) . | Canonical R2 . | Wilks’ Λ . | χ2 (df) . | P . | r theta–gamma coupling . | r theta power . | r gamma power . |
---|---|---|---|---|---|---|---|---|
1-back | ||||||||
1 | 92.4 | 0.21 | — | — | — | 0.72 | 0.11 | 0.67 |
2 | 7.6 | 0.02 | 0.98 | 4.51 (2) | 0.11 | — | — | — |
1 + 2 | 100 | — | 0.77 | 53.46 (6) | <0.0001 | — | — | — |
2-back | ||||||||
1 | 99.5 | 0.51 | — | — | — | 0.77 | 0.24 | 0.61 |
2 | 0.5 | 0.005 | 0.99 | 0.99 (2) | 0.61 | — | — | — |
1 + 2 | 100 | — | 0.49 | 148.0 (6) | <0.0001 | — | — | — |
3-back | ||||||||
1 | 100 | 0.38 | — | — | — | 0.65 | 0.27 | 0.60 |
2 | 0 | 0.00 | 1.00 | 0.05 (2) | 0.98 | — | — | — |
1 + 2 | 100 | — | 0.62 | 95.58 (6) | <0.0001 | — | — | — |
3-back with Lure as N − 1 | ||||||||
1 | 99.4 | 0.27 | — | — | — | 0.66 | 0.30 | 0.63 |
2 | 0.6 | 0.002 | 1.00 | 0.47 (2) | 0.79 | — | — | — |
1 + 2 | 100 | — | 0.73 | 62.42 (6) | <0.0001 | — | — | — |
Function . | Variance (%) . | Canonical R2 . | Wilks’ Λ . | χ2 (df) . | P . | r theta–gamma coupling . | r theta power . | r gamma power . |
---|---|---|---|---|---|---|---|---|
1-back | ||||||||
1 | 92.4 | 0.21 | — | — | — | 0.72 | 0.11 | 0.67 |
2 | 7.6 | 0.02 | 0.98 | 4.51 (2) | 0.11 | — | — | — |
1 + 2 | 100 | — | 0.77 | 53.46 (6) | <0.0001 | — | — | — |
2-back | ||||||||
1 | 99.5 | 0.51 | — | — | — | 0.77 | 0.24 | 0.61 |
2 | 0.5 | 0.005 | 0.99 | 0.99 (2) | 0.61 | — | — | — |
1 + 2 | 100 | — | 0.49 | 148.0 (6) | <0.0001 | — | — | — |
3-back | ||||||||
1 | 100 | 0.38 | — | — | — | 0.65 | 0.27 | 0.60 |
2 | 0 | 0.00 | 1.00 | 0.05 (2) | 0.98 | — | — | — |
1 + 2 | 100 | — | 0.62 | 95.58 (6) | <0.0001 | — | — | — |
3-back with Lure as N − 1 | ||||||||
1 | 99.4 | 0.27 | — | — | — | 0.66 | 0.30 | 0.63 |
2 | 0.6 | 0.002 | 1.00 | 0.47 (2) | 0.79 | — | — | — |
1 + 2 | 100 | — | 0.73 | 62.42 (6) | <0.0001 | — | — | — |
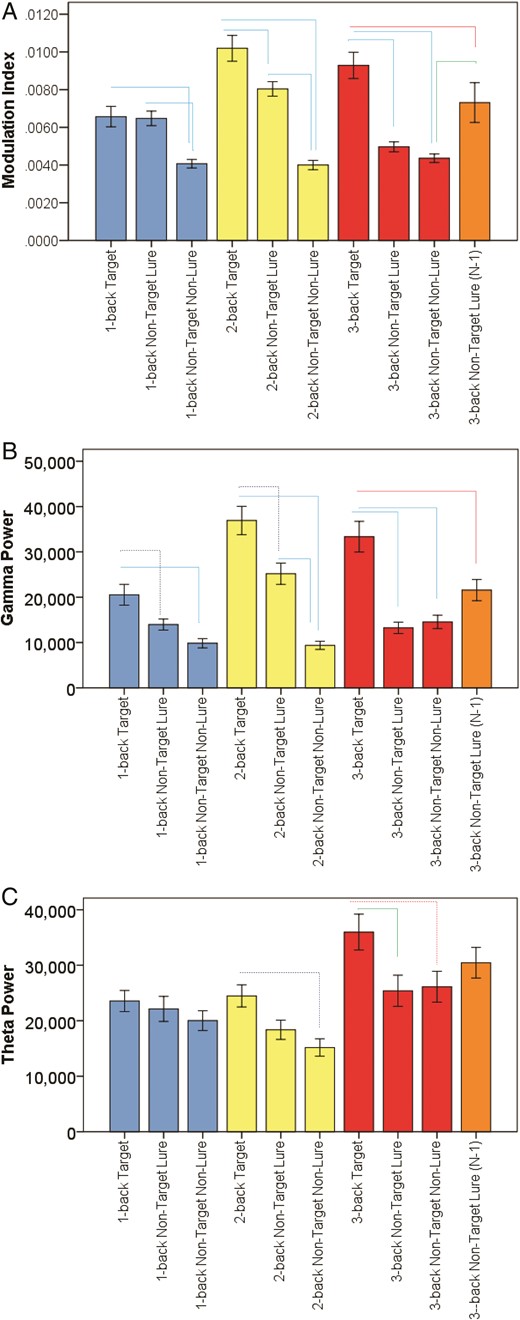
Theta–Gamma Coupling, Gamma and Theta Power, and N-back Conditions and Trial Types: (A) This figure illustrates differences in theta–gamma coupling across different trials types within each N-back condition. The lines indicate statistically significant differences after Bonferroni adjustments at P < 0.0001 (blue), P < 0.005 (green), and P = 0.041 (red). (B) This figure illustrates differences in gamma power (µV2) across different trials types within each N-back condition. The lines indicate statistically significant differences after Bonferroni adjustments at P = 0.001 (dashed blue), P < 0.0001 (blue), and P = 0.0005 (red). (C) This figure illustrates differences in theta power (µV2) across different trials types within each N-back condition. The lines indicate statistically significant differences after Bonferroni adjustments at P = 0.001 (dashed blue), P = 0.023 (green), and P = 0.033 (dashed red).
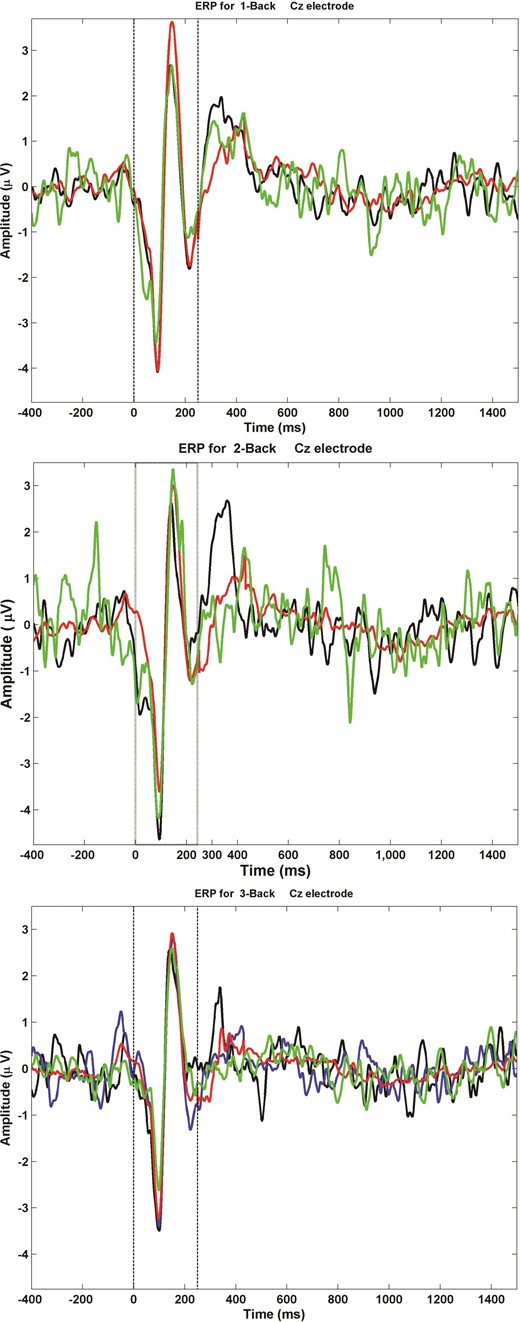
Event-Related Potentials (ERPs) Across Trial Types during N-back Conditions: This figure illustrates the ERPs as captured from Cz electrode during of 1-back (Top Panel), 2-back (Middle Panel), and 3-back (Lower Panel) conditions. The ERPs demonstrate the P300 component in response to the letter presentation. Target Correct trials are labeled in black. Non-Target Non-Lure Correct trials are labeled in green. Non-Target Lure Correct trials are labeled in red for 1- and 2-back conditions, and blue for 3-back condition. Non-Target Lure (N − 1) Correct trials are labeled in red for 3-back condition. The dashed lines indicate the onset and offset of the letter presentation.
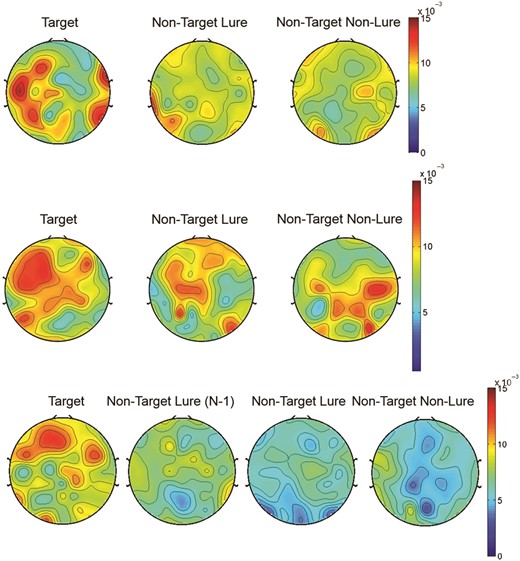
Theta–Gamma Coupling Across Trial Types during N-back Conditions: Top Panel: These topoplots illustrate theta–gamma coupling as measured using the Modulation Index during Target Correct (left), Non-Target Lure Correct (middle), and Non-Target Non-Lure Correct (right) trials of 1-back condition. Middle Panel: These topoplots illustrate theta–gamma coupling as measured using the Modulation Index during Target Correct (left), Non-Target Lure Correct (middle), and Non-Target Non-Lure Correct (right) trials of 2-back condition. Lower Panel: These topoplots illustrate theta–gamma coupling as measured using the Modulation Index during Target Correct (left), Non-Target Lure (N − 1) Correct (second to left, Non-Target Lure Correct (second to right), and Non-Target Non-Lure Correct (right) trials of 3-back condition.
Consistently, on the 2-back condition, we found that there was a significant effect of Trial Type on theta–gamma coupling, and theta and gamma powers (V = 0.52, F6,410 = 23.80, P < 0.00001. Follow-up discriminant analysis revealed 2 discriminant functions that, in combination, significantly differentiated the Trial Types. The first explained 99.5% of the variance. Theta–gamma coupling and gamma power loaded comparably and highly on this first function compared with theta power (Table 2). Finally, post-hoc analyses revealed that theta–gamma coupling on Target Correct trials was higher than theta–gamma coupling on Non-Target Lure Correct trials, which, in turn was also higher than on Non-Target Non-Lure Correct trials (Figs 2–4).
Finally, on 3-back condition, we found consistent results too. There was a significant effect of Trial Type on theta–gamma coupling, and theta and gamma powers (V = 0.38, F6,400 = 15.64, P < 0.00001. Follow-up discriminant analysis revealed 2 discriminant functions that, in combination, significantly differentiated the Trial Types. The first function explained 100% of the variance. Theta–gamma coupling and gamma power loaded comparably and highly on this first function compared with theta power (Table 2). Finally, post-hoc analyses revealed that theta–gamma coupling on Target Correct trials was higher than theta–gamma coupling on Non-Target Lure and Non-Lure Correct trials (Figs 2–4).
In the 3-back condition, Non-Target Lure trials include also trials that match N − 1, that is, two trials back. Focusing on these Lure trials, we also found that there was a significant effect of Trial Type on theta–gamma coupling, and theta and gamma powers (V = 0.27, F6,400 = 10.35, P < 0.00001). Follow-up discriminant analysis revealed 2 discriminant functions that, in combination, significantly differentiated the Trial Types. The first function explained 99.4% of the variance. Theta–gamma coupling and gamma power loaded comparably and highly on this first function compared with theta power (Table 2). Finally, post-hoc analyses revealed that theta–gamma coupling on Target Correct trials was higher than theta–gamma coupling on Non-Target Lure Correct trials, which in turn was also higher than on Non-Target Non-Lure Correct trials (Fig. 2). Interestingly, on these types of Lure trials, theta–gamma coupling was significantly higher than Non-Lure trials but not on the N + 1 Lure trials, again supporting the role of theta–gamma coupling in ordering of information. During Lure trials that match 2 trials back (N − 1), the full 3-back sequence needs to be recollected in order so that the Non-Target Lure trial is ascertained that it does not match 3 letter back but 2 instead. In contrast, during Lure trials that match 4 trials back (N + 1), it is conceivable that familiarity can be enough given that the matching letter to the Non-Target Lure trial is quite distant (4 letters back).
Testing Whether Response Time Differed among Target, Non-Target Lure, and Non-Target Non-Lure Correct Trials within Each N-back Condition
It is arguable that the cognitive effort associated with recollecting order of information requires more time and therefore more time to respond than recollecting this information without its order. Starting with 1-back condition, we found that there was a significant effect of Trial Type on Response Time (F2,207 = 27.37, P < 0.0001). And as expected, post-hoc analyses revealed that Response Time during Target and Non-Target Lure trials was significantly longer than during Non-Target Non-Lure trials (Ps < 0.0001). There was no statistical difference between Response Time during Target and Non-Target Lure trials. Mean Response Time (ms) [SD]: Target = 706.1 (120.2); Non-Target Lure = 711.3 (120.5); Non-Target Non-Lure = 583.2 (106.7).
Consistently, during 2-back condition, we found a significant effect of Trial Type on Response Time (F2,204 = 11.21, P < 0.0001). And also as expected, post-hoc analyses revealed that Response Time during Target and Non-Target Lure trials was significantly longer than during Non-Target Non-Lure trials (P < 0.0001, and P = 0.04, respectively). There was no statistical difference between Response Time during Target and Non-Target Lure trials. Mean Response Time (ms) [SD]: Target = 726.7 (141.0); Non-Target Lure = 650.1 (230.9); Non-Target Non-Lure = 564.8 (218.8).
On 3-back condition, we also found a significant effect of Trial Type on Response Time (F2,199 = 83.61, P < 0.0001). Also, post-hoc analyses revealed that Response Time during Target trials was significantly longer than during Non-Target Lure and Non-Lure trials (Ps < 0.0001), which is consistent with what was found with MI. In contrast, Response Time was longer during Non-Target Non-Lure trials than during Non-Target Lure trials (P < 0.0001). Mean Response Time (ms) [SD]: Target = 1886.5 (372.8); Non-Target Lure = 1002.6 (230.9); Non-Target Non-Lure = 1452.4 (474.0).
On 3-back condition with Non-Target Lure trials that match N − 1, we also found a significant effect of Trial Type on Response Time (F2,199 = 52.87, P < 0.0001). Also, post-hoc analyses revealed that Response Time during Target trials was significantly longer than during Non-Target Lure and Non-Lure trials (Ps < 0.0001), which is consistent with what was found with MI. Finally, Response Time was also longer during Non-Target Non-Lure trials than during Non-Target Lure trials (P = 0.001). Mean Response Time (ms) [SD]: Target = 1886.5 (372.8); Non-Target Lure = 1201.6 (292.3); Non-Target Non-Lure = 1452.4 (474.0).
Testing Whether Theta–Gamma Coupling is Significantly Higher Than Surrogate Data-Based Theta–Gamma Coupling across Target, Non-Target Lure, and Non-Target Non-Lure Correct Trials within Each N-back Condition
Using surrogate data generated as described earlier, paired-samples t-tests revealed that all MI, at each N-back condition and Trial Type, was significantly higher than corresponding surrogate data MI (Ps < 0.005).
Discussion
In this study, we examined the relationship between theta–gamma coupling and ordering of information during working memory performance. Working memory refers to the ability to encode, manipulate and retrieve information online and over a limited period of time (Baddeley 1996). Our results demonstrate, to our knowledge for the first time, that coupling is a significant and independent factor that specifically predicts ordering of information during working memory performance. These findings confirm indirect evidence from pre-clinical studies and establish for the first time in humans the hypothesized role of theta–gamma coupling in coding order of information.
We think we were to demonstrate this specific role because of the following: 1) assessing theta–gamma coupling at different levels of working memory loads using different N-back conditions; 2) assessing theta–gamma coupling at different types of trials that require different levels of ordering; and 3) focusing on the interval between stimulus presentation and time to respond, the interval during which recollecting a specific order of information is arguably most salient. The challenge of this last approach is overcoming the impact of variable times to respond across different N-back conditions and trial types on the measurement of theta–gamma coupling. Developing the concatenation approach allowed us to overcome this challenge.
Few studies in humans assessed the relationship between coupling and working memory. Three studies assessed this relationship in patients with intractable epilepsy. These patients had intracranial electrodes inserted as part of their epilepsy management and were used to record brain electrical activity. One study among 12 patients found that coupling increased when patients responded correctly on a continuous word recognition task during which patients had to identify whether a word had been presented before or not, with 3 or 14 intervening stimuli. This study did not differentiate between the 2 loads (Mormann et al. 2005). A second study among 5 patients demonstrated that various cognitive tasks were associated with various patterns of coupling across the intracranial electrodes. This study did not assess for a relationship between performance and coupling (Canolty et al. 2006). A third study among 14 patients assessed coupling during a working memory task with 3 levels of working memory loads. They were presented with 1, 2, or 4 photographs of faces. After 3000-ms retention phase, they were presented with a probe trial and asked whether the face(s) on this trial match the one(s) they have been before. This study demonstrated that during the retention phase of the correct trials, that is, before probing, the strength of coupling was increased compared with baseline. However, no relationship was found between working memory load and strength of coupling (Axmacher et al. 2010). Two other human studies assessed healthy subjects using scalp EEG. One study among 16 healthy subjects assessed theta phase-gamma phase coupling during the retention phase of a visual working memory task with 4 levels of working memory loads. Analyzing correct and incorrect responses together, this study demonstrated an increase in theta phase-gamma phase coupling during the retention phase (Sauseng et al. 2009). A second study among 13 healthy subjects assessed coupling during the performance of a visual 2-back working memory task or a vigilance task. In this study, theta–gamma coupling was found to be higher during the working memory task than during the vigilance task as captured from 1 of 19 electrodes. This study did not include various levels of working memory loads (Park et al. 2013). Together, these studies demonstrated a relationship between theta–gamma coupling and working memory; however, they did not demonstrate a relationship with ordering of information which is what is thought to be specifically supported by theta–gamma coupling. They were also limited by small samples sizes and the inclusion of patients with an underlying brain disorder.
Theta phase-gamma amplitude coupling is thought to code for ordering of information because it reflects different neuronal assemblies firing at sequential time points (Lisman and Idiart 1995; Buzsaki 2010). Anatomically, these neuronal assemblies are defined by the connections and firing of perisomatic basket cells. These cells fire at a theta frequency bursts of action potentials at gamma frequencies (Traub et al. 1996; Gloveli et al. 2005; Wulff et al. 2009). As more specific information needs to be ordered within a defined period of time, more distinct neuronal assemblies need to be activated in sequence to correctly code for this additional information. The distinctiveness of these various neuronal assemblies is captured by the difference in amplitude between consecutive gamma oscillations along a theta cycle. Hence, the activation of more distinct neuronal assemblies would result in larger differences in amplitude between consecutive gamma oscillations along a theta cycle, that is, stronger modulation (“coupling”) of gamma amplitude by theta phase (Tort et al. 2010). The brain ability to support such distinct neuronal assemblies requires robust networks. Hence, coupling may represent a neurophysiologic marker of the robustness of such networks during working memory (Buzsaki et al. 2013). Of note, coupling has been associated with other forms of information, for example, auditory steady state stimulation (Kirihara et al. 2012), supporting its role in organizing information in general.
Some limitations apply to our study. One limitation is related to the poor source localization that is inherent to scalp EEG. While there are approaches that have attempted to address this limitation, we believe that this is beyond the scope of this analysis. Also, since neuroimaging studies demonstrate a robust activation of the prefrontal cortex during N-back performance (Owen et al. 2005), we believe that EEG signals captured by the frontal electrodes are—at least to a significant extent—driven by prefrontal processing. Another limitation is the fact that the N-back task that was used in the study had a maximum load of 3. One could argue that we could have explored different levels of Lure trials had we used an N-back task that included higher loads (4 or 5). However, at such higher loads, correct performance is likely to significantly drop limiting the ability for meaningful analyses.
In conclusion, our study is the first to demonstrate direct evidence for a relationship between theta–gamma coupling and ordering of information, the latter being a fundamental process during optimal working memory performance and the key hypothesized function of theta–gamma coupling.
Funding
This work was supported by funds from the Brain and Behavior Research Foundation (YIA 17826 to T.K.R.), Canadian Foundation for Innovation (Grant ID: 25861 to T.K.R.), and Canadian Institutes of Health Research (OOG 244041 to T.K.R.).
Notes
Conflict of Interest: None declared.