-
PDF
- Split View
-
Views
-
Cite
Cite
Julie Vandewalle, Aster K Desouter, Bart J Van der Auwera, Sylvie Tenoutasse, Pieter Gillard, Christophe De Block, Bart Keymeulen, Frans K Gorus, Mark Van de Casteele, the Belgian Diabetes Registry, CTLA4, SH2B3, and CLEC16A diversely affect the progression of early islet autoimmunity in relatives of Type 1 diabetes patients, Clinical and Experimental Immunology, Volume 211, Issue 3, March 2023, Pages 224–232, https://doi.org/10.1093/cei/uxad002
- Share Icon Share
Abstract
The HLA region is the major genetic risk determinant of Type 1 diabetes. How non-HLA loci contribute to the genetic risk is incompletely understood, but there are indications that at least some impact progression of asymptomatic autoimmunity. We examined whether SNPs in 7 susceptibility loci (INS, SH2B3, PTPN2, PTPN22, CTLA4, CLEC16A, and IL2RA) could improve prediction of the progression from single to multiple autoantibody positivity, and from there on to diagnosis. SNPs were genotyped in persistently autoantibody positive relatives by allelic discrimination qPCR and disease progression was studied by multivariate Cox regression analysis. In our cohort, only the CTLA4 GA genotype (rs3087243, P = 0.002) and the CLEC16A AA genotype (rs12708716, P = 0.021) were associated with accelerated progression from single to multiple autoantibody positivity, but their effects were restricted to presence of HLA-DQ2/DQ8, and IAA as first autoantibody, respectively. The interaction of CTLA4 and HLA-DQ2/DQ8 overruled the effect of DQ2/DQ8 alone. The HLA-DQ2/DQ8-mediated risk of progression to multiple autoantibodies nearly entirely depended on heterozygosity for CTLA4. The SH2B3 TT genotype (rs3184504) was protective for HLA-DQ8 positive subjects (P = 0.003). At the stage of multiple autoantibodies, only the CTLA4 GA genotype was a minor independent risk factor for progression towards clinical diabetes (P = 0.034). Our study shows that non-HLA polymorphisms impact progression of islet autoimmunity in a subgroup-, stage- and SNP-specific way, suggesting distinct mechanisms. If confirmed, these findings may help refine risk assessment, follow-up, and prevention trials in risk groups.
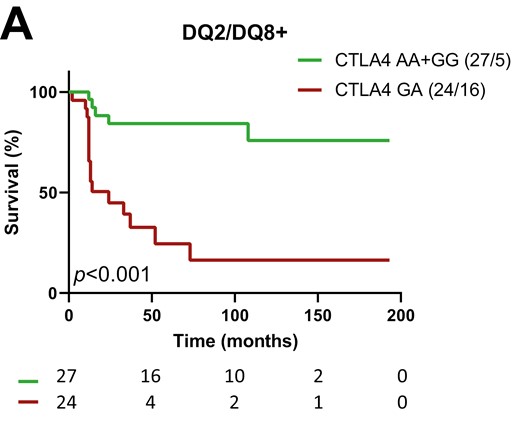
Introduction
Genetic factors, amongst other variables, affect both the risk of developing islet autoimmunity and its subsequent progression to overt Type 1 diabetes [1]. HLA polymorphisms account for a major part of the genetic risk, but genome-wide association studies (GWAS) have also identified about 60 different non-HLA loci each delivering a relatively small, albeit significant, contribution to genetic risk [2–5]. How these non-HLA loci modulate disease progression, is not yet fully understood. However, there are indications that at least some non-HLA polymorphisms may have a relatively large impact in specific subgroups and disease stages in time-to-event analysis [6–11].
In a registry-based cohort of islet autoantibody positive (autoAb+) first-degree relatives (FDRs) our group has shown that the factors that modulate progression of asymptomatic autoimmunity differ according to the autoAb profile present [12, 13], in line with observations by others [14, 15]. At the immune activation stage, heralded by single autoAb positivity, we found that disease progression is driven by HLA Class II-inferred risk, the presence of IAA as first autoAb, and younger age, culminating in the development of multiple autoAbs. In contrast, once the stage of multiple autoAb positivity—considered to represent asymptomatic Type 1 diabetes [16, 17]—is reached, the progression rate towards clinical onset is mainly influenced by HLA Class I-inferred risk and the presence of autoAbs directed against IA-2 (IA-2A) and/or zinc transporter 8 (ZnT8A) [13]. Our group previously reported statistical models built by Cox regression to predict progression through both these stages [10, 12].
Our goal now is to further disentangle the genetic basis of disease progression by studying the possible contribution of selected Type 1 diabetes-associated SNPs—alone or through interactions with known stage-specific risk factors—in single and multiple autoAb+ FDRs separately. This may help to better understand mechanisms underlying disease progression, and to identify those subjects who are at the highest risk of entering the next disease stage and likely to benefit most from participation in novel stage-specific prevention trials. We therefore selected eight SNPs in non-HLA loci (INS, SH2B3, PTPN2, PTPN22, CTLA4, CLEC16A, and IL2RA) to be tested on a cohort of Belgian autoAb+ FDRs under 40 years of age [10]. This selection was based on the relatively high odds ratios for Type 1 diabetes in several GWAS [3]. Moreover, these SNPs were reported to affect the development or the progression of asymptomatic islet autoimmunity in other specific study populations, although sometimes with contradictory findings [6–9, 11, 18–21]. Little is known about the stage-specific impact of non-HLA SNPs. We thus investigated whether any of these Type 1 diabetes non-HLA risk markers affected disease progression in the Belgian cohort of autoAb positive relatives and their potential improvement of stage-specific risk assessment based on multivariate time-to-event analyses.
Methods
Participants
Between March 1989 and December 2015, BDR followed 461 persistently autoAb+ siblings and offspring (<40 years of age) of Type 1 diabetes patients among a group of 7029 FDRs enrolled after informed consent from the relatives or their legal representative. This study was ethically approved under nr. BUN143201939922 by the institutional review board of Universitair Ziekenhuis Brussel (IRB UZB). The Belgian Diabetes Registry (BDR) provided pseudonymized clinical, anthropometric, and biological data. Blood and DNA samples (−80°C) were available from its associated biobank (IRB UZB nr BUN143201524128). The study was conducted in accordance with the guidelines of the Declaration of Helsinki [22]. The cohort of autoAb+ FDRs developed the first confirmed autoAb positivity at a median (IQR) age of 11.6 (6.4–19.3) years and had a median (IQR) follow-up time of 72 (35–129) months. Blood sampling was performed at study entry, and as a rule, yearly thereafter.
Analytical methods
HLA-DQ and HLA-A haplotypes were previously determined by allele-specific oligonucleotide hybridization and autoAbs against insulin (IAA), GAD65 (GADA), insulinoma-associated protein 2 (IA-2A), and ZnT8A were determined by liquid-phase radiobinding assays [12, 13]. INS rs689, INS rs1004446, PTPN2 rs45450798, PTPN22 rs6679677, CTLA4 rs3087243 (CT60), SH2B3 rs3184504, CLEC16A rs12708716, and IL2RA rs2104286 were genotyped by allelic discrimination using TaqMan SNP genotyping assays C_1223317_10, C_1223303_10, C_86382390_10, C_29537457_10, C_3296043_10, C_2981072_10, C_31075342_10, and C_16095542_10 respectively (cat no 4351379, Applied Biosystems, Foster City, CA) on a QuantStudio™ 12K Flex Real-Time PCR System (Applied Biosystems). Controls without DNA were included in each run. Genotype calling was performed by the software.
Statistical analyses
Stage-specific impact on progression rate was evaluated for each individual SNP. Both univariate and multivariate Cox regression analysis of survival was used to assess progression from single to multiple autoAb positivity, and from there on to disease onset. Besides SNP genotype, other stage-specific independent predictors were included in the analysis that were previously determined in the present cohort [12], together with their respective alleged interactions with SNP genotype (for multivariate analysis). Since BMI was previously shown not to represent a significant factor for progression in this cohort [12], it was not included in the present analyses. For progression from single to multiple autoAb positivity the variables include age at first autoAb positivity, and absence/presence of HLA-DQ8, HLA-DQ2/DQ8, HLA-A*24, and IAA. For progression from multiple autoAb positivity to diabetes these variables include age at first multiple autoAb positivity, absence/presence of HLA-A*24 and IA-2A/ZnT8A, and being offspring of a diabetic mother. Multivariate analysis was performed in dominant, recessive, and additive inheritance models per SNP. Significant interaction effects were visualized by Kaplan-Meier survival curves, which in turn were analyzed with the log rank test. Next, a combined Cox model was constructed for the immune activation stage, starting from a base model including three main variables: age at first autoAb positivity, HLA-DQ2/DQ8, and HLA-A*24. Identified interaction effects and the previously reported protective interaction effect between female sex and ERBB3 GG [10] were added to this model in a conditional forward approach. The goodness of fit of the models was tested using the Akaike information criterion (AIC), which represents an estimate of information not explained by the model and includes a penalty for the number of variables [23]. Models are compared by the relative likelihood calculated from the differences in AIC values [24]. Differences in AIC values of >4 between two models indicate that the model with the higher value is less plausible, while, with differences of >10, the model with the higher AIC value can be omitted [24]. AIC values were calculated for each step, to assess to what extent the predictive model could be improved. Stepwise conditional forward modeling was performed in SPSS version 27.0 software (IBM, Armonk, NY). Two-tailed statistical tests were performed and P-values <0.05 were considered significant. We did not correct for multiplicity (Bonferroni correction) when performing comparisons between groups but used multivariate Cox regression to adjust for possible confounders. Figures were prepared using GraphPad Prism version 9 for Windows (GraphPad Software, San Diego, CA).
Results
SNP genotyping
In our cohort of 461 persistently autoAb+ FDRs, 448 could be genotyped for at least one of the eight studied SNPs; 13 could not, due to unavailable DNA. During a median follow-up time (IQR) of 60 (24–130) months 69 of 261 initially autoAb+ relatives developed one or more additional autoAb, and 18 of them progressed towards diagnosis without first developing additional autoAbs, as visualized in Supplementary Fig. 1. Progression towards Type 1 diabetes could be monitored in 256 FDRs (187 FDRs with multiple autoAb positivity at study entry and 69 initially single autoAb+ FDRs after developing a second autoAb), of whom 145 progressed to clinical onset during a median follow-up time (IQR) of 56 (28–96) months (Supplementary Fig. 1). All eight SNPs tested had call rates above 98%, and all SNP-genotypes followed Hardy-Weinberg equilibrium (data not shown). The amount of missing data per SNP is presented in Supplementary Table 1.
Progression from single to multiple autoAb positivity
Univariate Cox analysis confirmed that age at first autoAb positivity (P < 0.0001), HLA-DQ8 (P = 0.013), HLA-DQ2/DQ8 (P = 0.001), HLA-A*24 (P = 0.023), and IAA as first autoAb (P = 0.022) impacted the progression from single to multiple autoAb positivity in our cohort, in line with our previous findings [12], and indicated that none of the SNPs could influence the progression rate when tested as single independent variable (Supplementary Table 2). To further investigate the possible impact of each of the eight SNPs on disease progression, multivariate Cox regression models were built using a conditional forward approach. While younger age at immune activation, presence of HLA-DQ2/DQ8 haplotype, and absence of HLA-A*24 genotype, remained independent predictors of faster progression towards multiple autoAb positivity in the cohort (Table 1), as previously reported [12, 13], none of the eight SNPs emerged as additional determinants of progression in this model (Table 1, and data not shown). However, some SNPs (CTLA4, SH2B3, CLEC16A, and INS rs689) contributed to the regression model via interaction effects with the determinants identified in the univariate analysis. The INS rs689 TT genotype appeared to affect progression from single to multiple autoAb positivity in HLA-DQ8 positive participants, although, this effect was only borderline significant (P = 0.050), and it was not observed for INS rs1004446. The CTLA4 GA genotype accelerated progression to multiple autoAbs only when the high-risk haplotype HLA-DQ2/DQ8 was present (P = 0.002, HR = 4.929, Table 1), while, in turn, HLA-DQ2/DQ8 was no longer an independent predictor in this model (Table 1). For the SH2B3 TT genotype, progression to multiple autoAb positivity occurred more rapidly in IAA positive FDRs (P = 0.043, HR = 3.550, Table 1). In addition, a more pronounced interaction with HLA-DQ8 was observed, resulting in a delay in progression in individuals positive for both HLA-DQ8 and the SH2B3 TT genotype (P = 0.003, HR = 0.122, Table 1). Lastly, IAA positive FDRs carrying the CLEC16A AA risk genotype progressed faster to the stage of multiple autoAbs (P = 0.021, HR = 1.986, Table 1). No interaction effects were found for IL2RA, PTPN2, and PTPN22. Given our previous findings on the interaction between locus ERBB3 and female sex [10], we also tested all eight SNPs for interaction with the sex variable, but no interactions were found (data not shown).
CTLA4 GA, SH2B3 TT, and CLEC16A AA genotypes in Cox regression analysis of the progression from single to multiple autoAb positivity in FDRs
. | CTLA4 (GA vs. GG+AA) . | SH2B3 (TT vs. CC+TC) . | CLEC16A (AA vs. GG+AG) . | |||
---|---|---|---|---|---|---|
Variable . | P . | HR [95% CI] . | P . | HR [95% CI] . | P . | HR [95% CI] . |
Age first autoAb+ | <0.0001 | 0.915 [0.884−0.948] | <0.0001 | 0.908 [0.876−0.941] | <0.0001 | 0.913 [0.882−0.946] |
IAAb (0/1a) | — | — | — | — | — | — |
Non-(HLA-A*24) (0/1a) | 0.028 | 2.295 [1.093−4.822] | 0.007 | 2.845 [1.329−6.091] | 0.016 | 2.480 [1.183−5.198] |
HLA-DQ8 (0/1a) | — | — | 0.024 | 1.981 [1.092−3.593] | — | — |
HLA-DQ2/DQ8 (0/1a) | 0.824 | 0.021 | 2.122 [1.120−4.023] | <0.0001 | 3.112 [1.811−5.349] | |
SNP (0/1a) | — | — | — | — | — | — |
SNP × age first autoAb+ | — | — | — | — | — | — |
SNP × IAA | — | — | 0.043 | 3.550 [1.041−12.110] | 0.021 | 1.986 [1.109−3.556] |
SNP × non-(HLA-A*24) | — | — | — | — | — | — |
SNP × HLA-DQ8 | — | — | 0.003 | 0.122 [0.030−0.498] | — | — |
SNP × HLA-DQ2/DQ8 | 0.002 | 4.929 [1.783−13.632] | — | — | — | — |
. | CTLA4 (GA vs. GG+AA) . | SH2B3 (TT vs. CC+TC) . | CLEC16A (AA vs. GG+AG) . | |||
---|---|---|---|---|---|---|
Variable . | P . | HR [95% CI] . | P . | HR [95% CI] . | P . | HR [95% CI] . |
Age first autoAb+ | <0.0001 | 0.915 [0.884−0.948] | <0.0001 | 0.908 [0.876−0.941] | <0.0001 | 0.913 [0.882−0.946] |
IAAb (0/1a) | — | — | — | — | — | — |
Non-(HLA-A*24) (0/1a) | 0.028 | 2.295 [1.093−4.822] | 0.007 | 2.845 [1.329−6.091] | 0.016 | 2.480 [1.183−5.198] |
HLA-DQ8 (0/1a) | — | — | 0.024 | 1.981 [1.092−3.593] | — | — |
HLA-DQ2/DQ8 (0/1a) | 0.824 | 0.021 | 2.122 [1.120−4.023] | <0.0001 | 3.112 [1.811−5.349] | |
SNP (0/1a) | — | — | — | — | — | — |
SNP × age first autoAb+ | — | — | — | — | — | — |
SNP × IAA | — | — | 0.043 | 3.550 [1.041−12.110] | 0.021 | 1.986 [1.109−3.556] |
SNP × non-(HLA-A*24) | — | — | — | — | — | — |
SNP × HLA-DQ8 | — | — | 0.003 | 0.122 [0.030−0.498] | — | — |
SNP × HLA-DQ2/DQ8 | 0.002 | 4.929 [1.783−13.632] | — | — | — | — |
a0/1: no/yes.
bIAA as first autoantibody.
—, not retained in conditional forward model (P > 0.050). P values, hazard ratio’s (HR) and 95% confidence intervals are presented. No significant interaction effects were found for INS rs1004446, PTPN2, PTPN22, and IL2RA. A borderline effect was found for INS rs689 × HLA-DQ8 (P = 0.05, HR = 1.727).
CTLA4 GA, SH2B3 TT, and CLEC16A AA genotypes in Cox regression analysis of the progression from single to multiple autoAb positivity in FDRs
. | CTLA4 (GA vs. GG+AA) . | SH2B3 (TT vs. CC+TC) . | CLEC16A (AA vs. GG+AG) . | |||
---|---|---|---|---|---|---|
Variable . | P . | HR [95% CI] . | P . | HR [95% CI] . | P . | HR [95% CI] . |
Age first autoAb+ | <0.0001 | 0.915 [0.884−0.948] | <0.0001 | 0.908 [0.876−0.941] | <0.0001 | 0.913 [0.882−0.946] |
IAAb (0/1a) | — | — | — | — | — | — |
Non-(HLA-A*24) (0/1a) | 0.028 | 2.295 [1.093−4.822] | 0.007 | 2.845 [1.329−6.091] | 0.016 | 2.480 [1.183−5.198] |
HLA-DQ8 (0/1a) | — | — | 0.024 | 1.981 [1.092−3.593] | — | — |
HLA-DQ2/DQ8 (0/1a) | 0.824 | 0.021 | 2.122 [1.120−4.023] | <0.0001 | 3.112 [1.811−5.349] | |
SNP (0/1a) | — | — | — | — | — | — |
SNP × age first autoAb+ | — | — | — | — | — | — |
SNP × IAA | — | — | 0.043 | 3.550 [1.041−12.110] | 0.021 | 1.986 [1.109−3.556] |
SNP × non-(HLA-A*24) | — | — | — | — | — | — |
SNP × HLA-DQ8 | — | — | 0.003 | 0.122 [0.030−0.498] | — | — |
SNP × HLA-DQ2/DQ8 | 0.002 | 4.929 [1.783−13.632] | — | — | — | — |
. | CTLA4 (GA vs. GG+AA) . | SH2B3 (TT vs. CC+TC) . | CLEC16A (AA vs. GG+AG) . | |||
---|---|---|---|---|---|---|
Variable . | P . | HR [95% CI] . | P . | HR [95% CI] . | P . | HR [95% CI] . |
Age first autoAb+ | <0.0001 | 0.915 [0.884−0.948] | <0.0001 | 0.908 [0.876−0.941] | <0.0001 | 0.913 [0.882−0.946] |
IAAb (0/1a) | — | — | — | — | — | — |
Non-(HLA-A*24) (0/1a) | 0.028 | 2.295 [1.093−4.822] | 0.007 | 2.845 [1.329−6.091] | 0.016 | 2.480 [1.183−5.198] |
HLA-DQ8 (0/1a) | — | — | 0.024 | 1.981 [1.092−3.593] | — | — |
HLA-DQ2/DQ8 (0/1a) | 0.824 | 0.021 | 2.122 [1.120−4.023] | <0.0001 | 3.112 [1.811−5.349] | |
SNP (0/1a) | — | — | — | — | — | — |
SNP × age first autoAb+ | — | — | — | — | — | — |
SNP × IAA | — | — | 0.043 | 3.550 [1.041−12.110] | 0.021 | 1.986 [1.109−3.556] |
SNP × non-(HLA-A*24) | — | — | — | — | — | — |
SNP × HLA-DQ8 | — | — | 0.003 | 0.122 [0.030−0.498] | — | — |
SNP × HLA-DQ2/DQ8 | 0.002 | 4.929 [1.783−13.632] | — | — | — | — |
a0/1: no/yes.
bIAA as first autoantibody.
—, not retained in conditional forward model (P > 0.050). P values, hazard ratio’s (HR) and 95% confidence intervals are presented. No significant interaction effects were found for INS rs1004446, PTPN2, PTPN22, and IL2RA. A borderline effect was found for INS rs689 × HLA-DQ8 (P = 0.05, HR = 1.727).
Cox regression thus suggested interactions between SNPs and specific population variables to affect the rate of progression from single to multiple autoAb positivity. Kaplan-Meier analysis confirmed that progression towards multiple autoAb positivity was strongly accelerated by the CTLA4 heterozygous GA genotype in HLA-DQ2/DQ8 positive participants (P < 0.001, GA vs. AA+GG, Fig. 1a and b). The progression tended to be slower in presence of the SH2B3 TT genotype (TT vs. CC+TC) in HLA-DQ8 positive participants (P = 0.016, Supplementary Fig. 2 a and b), single autoAb+ participants without IAA (P = 0.062, Supplementary Fig. 2 c and d), and even more so in HLA-DQ8 positive participants without IAA (P = 0.014, Fig. 1c and d), as suggested by the Cox regression (Table 1). For CLEC16A, the association of the AA genotype with more rapid progression towards multiple autoAb positivity in IAA positive participants did not reach significance in Kaplan-Meier analysis (P = 0.169, AA vs. GG+AG, Fig. 1e and f).
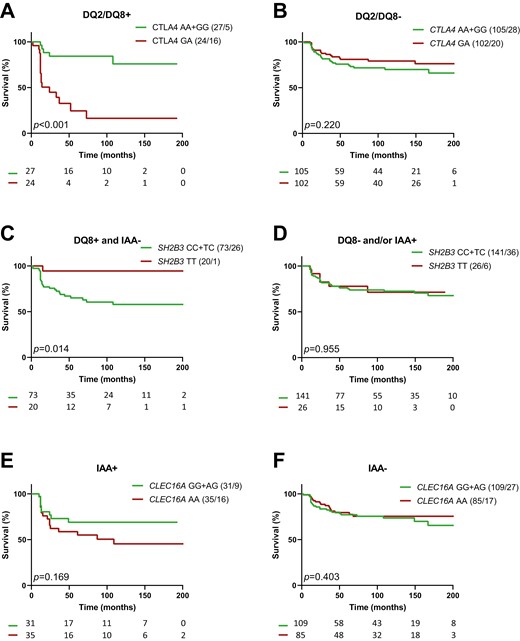
impact of CTLA4, SH2B3, and CLEC16A on the progression from 1 to ≥2 autoAbs in first-degree relatives of T1D patients. Kaplan-Meier survival curves for relatives with or without genotype CTLA4 GA (a and b), SH2B3 TT (c and d) and CLEC16A AA (e and f). Strata are indicated above the graphs; they correspond to subpopulations of FDRs with (left panels) or without (right panels): (a and b) HLA DQ2+/DQ8+ (DQ2/DQ8+); (c and d) HLA-DQ8+ and no IAA as first autoAb (DQ8+ and IAA−); (e and f) IAA as first autoAb (IAA+). P values (P) of log rank tests are presented and numbers of individuals at risk indicated below the time-axis. For each arm the genotype and number (cases/events) are shown above the graph.
To examine whether population risk of progression towards the stage of asymptomatic Type 1 diabetes could be influenced by a combined impact of these SNPs, we assessed the possibility of building a multivariable Cox regression model, including the significant interaction effects for each SNP (Table 1) as well as the previously reported interaction between ERBB3 and female sex [10]. All these interactions remained independent predictors for the progression rate from single to multiple autoAb positivity (CLEC16A AA × IAA, P = 0.035 and HR = 1.871; CTLA4 GA × HLA-DQ2/DQ8, P = 0.002 and HR = 4.869; SH2B3 TT × HLA-DQ8, P = 0.045 and HR = 0.302; ERBB3 GG × female, P = 0.021 and HR = 0.334), except for SH2B3 × IAA (Table 2). Again, the high-risk haplotype HLA-DQ2/DQ8 was no longer an independent predictor in this combined model (P = 0.473, Table 2). Without considering interactions between non-HLA SNPs and previously confirmed predictors of autoimmune progression, the Cox model identified age at first autoAb positivity, absence of HLA-A*24, and presence of HLA-DQ2/DQ8 as significant risk factors, yielding an AIC value of 692.8 (Table 2); stepwise addition of the abovementioned SNP-based interactions into the model progressively decreased the corresponding AIC values to 648.1 (Table 2); this decrease in AIC by >40 points suggested model improvement [24].
Cox regression analysis of the progression from 1 to ≥2 autoAb including all interaction effects identified so far between non-HLA SNPs and main predictive variables in the FDRs cohort: ERBB3 GG × female sex (10), CLEC16A AA × IAA, CTLA4 GA × HLA-DQ2/DQ8, SH2B3 TT × HLA-DQ8, SH2B3 TT × IAA
Variable . | P . | HR (95% CI) . | Step . | AIC . |
---|---|---|---|---|
Age first autoAb+ | <0.001 | 0.910 [0.878−0.944] | 1 | 692.8 |
Non-(HLA-A*24) (0/1a) | 0.031 | 2.275 [1.080−4.792] | 1 | 692.8 |
HLA-DQ2/DQ8 (0/1a) | 0.473 | 1 | 692.8 | |
HLA-DQ8 (0/1a) | NM | |||
CTLA4 GA × HLA-DQ2/DQ8 | 0.006 | 4.226 [1.526−11.706] | 2 | 660.0 |
CLEC16A AA × IAA | 0.030 | 1.904 [1.064−3.410] | 3 | 657.0 |
ERBB3 GG × sex (0/1b) | 0.021 | 0.334 [0.132−0.848] | 4 | 652.9 |
SH2B3 TT × HLA-DQ8 | 0.045 | 0.275 [0.085−0.889] | 5 | 648.1 |
SH2B3 TT × IAA | NM |
Variable . | P . | HR (95% CI) . | Step . | AIC . |
---|---|---|---|---|
Age first autoAb+ | <0.001 | 0.910 [0.878−0.944] | 1 | 692.8 |
Non-(HLA-A*24) (0/1a) | 0.031 | 2.275 [1.080−4.792] | 1 | 692.8 |
HLA-DQ2/DQ8 (0/1a) | 0.473 | 1 | 692.8 | |
HLA-DQ8 (0/1a) | NM | |||
CTLA4 GA × HLA-DQ2/DQ8 | 0.006 | 4.226 [1.526−11.706] | 2 | 660.0 |
CLEC16A AA × IAA | 0.030 | 1.904 [1.064−3.410] | 3 | 657.0 |
ERBB3 GG × sex (0/1b) | 0.021 | 0.334 [0.132−0.848] | 4 | 652.9 |
SH2B3 TT × HLA-DQ8 | 0.045 | 0.275 [0.085−0.889] | 5 | 648.1 |
SH2B3 TT × IAA | NM |
a0/1: no/yes.
b0/1: male/female.
NM, not retained in model. P values, hazard ratio’s (HR) and 95% confidence intervals are presented for each variable and interaction effect, as well as model AIC values at the indicated step in the model construction by conditional forward approach.
Cox regression analysis of the progression from 1 to ≥2 autoAb including all interaction effects identified so far between non-HLA SNPs and main predictive variables in the FDRs cohort: ERBB3 GG × female sex (10), CLEC16A AA × IAA, CTLA4 GA × HLA-DQ2/DQ8, SH2B3 TT × HLA-DQ8, SH2B3 TT × IAA
Variable . | P . | HR (95% CI) . | Step . | AIC . |
---|---|---|---|---|
Age first autoAb+ | <0.001 | 0.910 [0.878−0.944] | 1 | 692.8 |
Non-(HLA-A*24) (0/1a) | 0.031 | 2.275 [1.080−4.792] | 1 | 692.8 |
HLA-DQ2/DQ8 (0/1a) | 0.473 | 1 | 692.8 | |
HLA-DQ8 (0/1a) | NM | |||
CTLA4 GA × HLA-DQ2/DQ8 | 0.006 | 4.226 [1.526−11.706] | 2 | 660.0 |
CLEC16A AA × IAA | 0.030 | 1.904 [1.064−3.410] | 3 | 657.0 |
ERBB3 GG × sex (0/1b) | 0.021 | 0.334 [0.132−0.848] | 4 | 652.9 |
SH2B3 TT × HLA-DQ8 | 0.045 | 0.275 [0.085−0.889] | 5 | 648.1 |
SH2B3 TT × IAA | NM |
Variable . | P . | HR (95% CI) . | Step . | AIC . |
---|---|---|---|---|
Age first autoAb+ | <0.001 | 0.910 [0.878−0.944] | 1 | 692.8 |
Non-(HLA-A*24) (0/1a) | 0.031 | 2.275 [1.080−4.792] | 1 | 692.8 |
HLA-DQ2/DQ8 (0/1a) | 0.473 | 1 | 692.8 | |
HLA-DQ8 (0/1a) | NM | |||
CTLA4 GA × HLA-DQ2/DQ8 | 0.006 | 4.226 [1.526−11.706] | 2 | 660.0 |
CLEC16A AA × IAA | 0.030 | 1.904 [1.064−3.410] | 3 | 657.0 |
ERBB3 GG × sex (0/1b) | 0.021 | 0.334 [0.132−0.848] | 4 | 652.9 |
SH2B3 TT × HLA-DQ8 | 0.045 | 0.275 [0.085−0.889] | 5 | 648.1 |
SH2B3 TT × IAA | NM |
a0/1: no/yes.
b0/1: male/female.
NM, not retained in model. P values, hazard ratio’s (HR) and 95% confidence intervals are presented for each variable and interaction effect, as well as model AIC values at the indicated step in the model construction by conditional forward approach.
Progression from multiple autoAb positivity towards clinical onset
At the stage of multiple (≥2) autoAb positivity (Supplementary Table 3), positivity for IA-2A and/or ZnT8A (P < 0.001), presence of HLA-A*24 (P = 0.019), and younger age (P = 0.045), were all associated with accelerated progression towards clinical onset in univariate Cox regression analysis, while being offspring of a diabetic mother was found to confer protection (P = 0.014), in line with previous reports [12, 13, 25, 26]. None of the tested SNPs individually affected progression towards diagnosis (Supplementary Table 3). In multivariate Cox regression, CTLA4 GA emerged as an independent predictor of accelerated progression in this disease stage (P = 0.034, HR = 1.444, Table 3), while INS, SH2B3, PTPN2, PTPN22, CLEC16A, and IL2RA showed no effect, either alone or in interaction with aforementioned main variables. The interaction between the CTLA4 GA genotype and HLA-DQ2/DQ8 was no longer significant at this stage (data not shown) and the suggested association of the CTLA4 GA genotype with a faster progression rate to clinical onset in Cox regression analysis could not be confirmed in Kaplan-Meier survival (P = 0.144, Fig. 2).
CTLA4 in Cox regression analysis of the progression from multiple autoAb positivity to clinical onset in FDRs
Variable . | P . | HR (95% CI) . |
---|---|---|
Age multiple autoAb+ | 0.015 | 0.971 [0.949−0.994] |
IA-2A and/or ZnT8Aa (0/1b) | <0.0001 | 2.499 [1.734−3.602] |
HLA-A*24 (0/1b) | 0.001 | 1.969 [1.308−2.962] |
No diabetic mother (0/1b) | 0.032 | 1.852 [1.054−3.252] |
CTLA4 GA (0/1b) | 0.034 | 1.444 [1.029−2.026] |
Variable . | P . | HR (95% CI) . |
---|---|---|
Age multiple autoAb+ | 0.015 | 0.971 [0.949−0.994] |
IA-2A and/or ZnT8Aa (0/1b) | <0.0001 | 2.499 [1.734−3.602] |
HLA-A*24 (0/1b) | 0.001 | 1.969 [1.308−2.962] |
No diabetic mother (0/1b) | 0.032 | 1.852 [1.054−3.252] |
CTLA4 GA (0/1b) | 0.034 | 1.444 [1.029−2.026] |
aHigh risk autoAb profile.
b0/1: no/yes. P values, hazard ratio’s (HR) and 95% confidence intervals are presented. For INS (rs689 and rs1004446), SH2B3, PTPN2, PTPN22, CLEC16A, and IL2RA no significant effects or interaction effects were retained.
CTLA4 in Cox regression analysis of the progression from multiple autoAb positivity to clinical onset in FDRs
Variable . | P . | HR (95% CI) . |
---|---|---|
Age multiple autoAb+ | 0.015 | 0.971 [0.949−0.994] |
IA-2A and/or ZnT8Aa (0/1b) | <0.0001 | 2.499 [1.734−3.602] |
HLA-A*24 (0/1b) | 0.001 | 1.969 [1.308−2.962] |
No diabetic mother (0/1b) | 0.032 | 1.852 [1.054−3.252] |
CTLA4 GA (0/1b) | 0.034 | 1.444 [1.029−2.026] |
Variable . | P . | HR (95% CI) . |
---|---|---|
Age multiple autoAb+ | 0.015 | 0.971 [0.949−0.994] |
IA-2A and/or ZnT8Aa (0/1b) | <0.0001 | 2.499 [1.734−3.602] |
HLA-A*24 (0/1b) | 0.001 | 1.969 [1.308−2.962] |
No diabetic mother (0/1b) | 0.032 | 1.852 [1.054−3.252] |
CTLA4 GA (0/1b) | 0.034 | 1.444 [1.029−2.026] |
aHigh risk autoAb profile.
b0/1: no/yes. P values, hazard ratio’s (HR) and 95% confidence intervals are presented. For INS (rs689 and rs1004446), SH2B3, PTPN2, PTPN22, CLEC16A, and IL2RA no significant effects or interaction effects were retained.
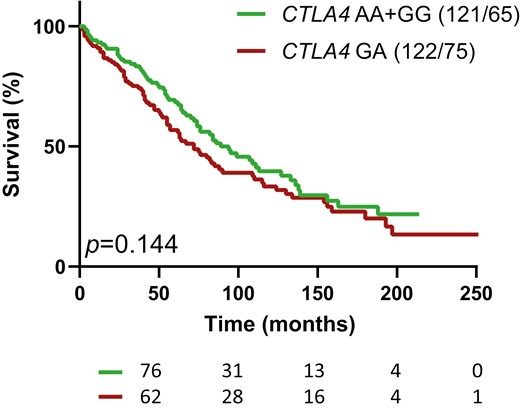
Kaplan-Meier survival analysis of progression from multiple autoAb positivity to clinical onset, for relatives with or without the CTLA4 GA genotype. P value (P) is from log rank test on differences in survival. The numbers of individuals at risk are indicated below the time-axis. For each arm the genotype and number (cases/events) are shown above the graph.
Discussion
In our Belgian cohort of FDRs at risk, polymorphisms at the non-HLA loci INS, CTLA4, SH2B3, CLEC16A, PTPN2, PTPN22, and IL2RA did not impact the progression of asymptomatic islet autoimmunity when considered in isolation, but our multivariate analysis unveiled that CTLA4, SH2B2, and CLEC16A could exert distinct effects in a subgroup-, stage- and SNP-specific way through interaction with established risk factors of disease progression. Thus, for 3 out of 7 non-HLA loci investigated we found evidence that polymorphisms are associated with progression of islet autoimmunity.
Our most important observation was the striking acceleration of progression from single to multiple autoAb positivity due to the interaction effect between HLA-DQ2/DQ8 and the heterozygous CTLA4 (rs3087243 or CT60) GA genotype, which even overruled the effect of HLA-DQ2/DQ8 alone. Furthermore, CTLA4 GA was a minor independent risk factor for progression towards clinical diabetes at the stage of multiple autoantibodies. To our knowledge, these effects have not been reported before in other longitudinal studies [6, 8, 9, 21]. This may relate to our approach consisting of looking for interactions with established risk factors at distinct disease stages, or to differences between various cohorts in terms of age range of participants, family history of Type 1 diabetes, preselection based on HLA-DQ/DR-inferred risk, and prevalence of risk alleles in the background population [6, 7, 9]. If confirmed in other populations, the strong interaction between the HLA-DQ2/DQ8 and CTLA4 GA genotypes, occurring in about 50% of the HLA-DQ2/DQ8 cases, may impact risk assessment in autoAb+ individuals and inclusion criteria for participation in future prevention trials, especially at the stage of antigen spreading. Our finding also warrants further investigation into the mechanisms underlying disease progression at the molecular and cellular level. CTLA4 has been reported to be associated with Type 1 diabetes in general [27, 28], and in a subset of patients with autoimmune thyroid disease [29, 30]. Interestingly, the TEDDY Study Group reported that gestational respiratory infections interacted with offspring HLA and CTLA4 to modify the nature of the first appearing beta cell autoAb specificity, with a consistent protective influence on the development of IAA among children with specific CTLA4 Ala17 genotypes [31]. The gene product of CTLA4 acts as a negative regulator for T-cell proliferation [32, 33]. Its activity may be modulated by soluble CTLA4, an isoform lacking the exon encoding the transmembrane region, whose expression level appears to correlate with CTLA4 CT60 [34]. Additionally, heterozygous germline CTLA4 mutations have been linked to immune response overactivation [35]. Furthermore, CTLA4 is a target gene in cancer therapy, with immune checkpoint blockade-induced diabetes as a common side effect, often in patients with HLA-DQ risk haplotypes [36, 37]. Apart from Type 1 diabetes, CTLA4 gene polymorphisms have been shown to be associated with other autoimmune diseases [33, 38–42], with notable parallelisms regarding their impact on other endocrinopathies. For instance, an interaction between CTLA4 polymorphism (Ala17) and HLA-DQ has been reported in Addison’s disease [43]. Moreover, in an Italian population, CTLA4 CT60 (rs3087243) polymorphism was found to be associated with Addison’s disease, only in heterozygous (A/G) individuals [44].
For SH2B3, epitope spreading was accelerated in IAA positive FDRs. However, an even more pronounced interaction with HLA-DQ8 was observed, resulting in a delayed disease progression in individuals positive for both HLA-DQ8 and the SH2B3 TT genotype. The protein SH2B3 acts as a negative regulator of cytokine signaling and is necessary for lymphoid hematopoiesis [45]. SH2B3 genotypes have been linked to activation levels of the NOD2 pathway [46], a pathway known to induce proinflammatory response and impaired insulin signaling, and to be upregulated in kidneys of diabetes patients [47]. The TEDDY study reported the risk allele SH2B3 T to be linked with initial autoantibody development [8, 9]. However, other large studies did not observe significant associations with preclinical diabetes progression [6]. As far as we know, no primary autoantigen- or HLA genotype-dependent association has been reported before for this SNP.
Our results also suggest that the CLEC16A AA risk genotype selectively increases epitope spreading in IAA positive FDRs. This gene is involved in mitophagy, and mutations can cause impaired glucose-stimulated insulin release [48]. Additionally, the studied SNP within CLEC16A appears to affect thymus-specific splice regulation [49]. With regards to diabetes disease progression, Krischer et al. [9] reported an association between CLEC16A with the appearance of any islet autoimmunity, but not Type 1 diabetes, within 6 years of age, while Lempainen et al. [6] reported no significant associations for this SNP in their cohort followed for a median time of 11 years.
At variance with other time-to-event studies, we did not find any significant association between disease progression and polymorphisms at IL2RA, PTPN22, PTPN2, and INS (rs1004446), neither when studied alone nor via interactions [6–9]. We only noted a borderline significant interaction between INS rs689 and DQ8. Possible explanations for these conflicting results include our different study design (not pre-selecting for HLA-DQ risk haplotypes, and studying relatives instead of the general population), or the fact that SNP allelic distributions often vary based on geographic and demographic factors. Additionally, our study population contains subjects of relatively older age compared with other large studies in this field. Although this may complicate comparisons, it also provides a unique insight in the less studied disease progression in adults and may thus be considered as a strength of our study. Other strengths include the long-term recruitment and follow-up of FDRs of diagnosed Type 1 diabetes patients and the low amount of missing data (Supplementary Table 1). The underrepresentation of young children may constitute a limitation. Also, for many subjects the exact time of seroconversion is unknown, as they were already single or multiple autoAb positive at study entry. However, previous studies by our group have shown that omitting these subjects from the analysis did not significantly impact its conclusions [50].
Interestingly, almost all the interactions mentioned above remain independent predictors of initial autoAb development rate when combined into one all-encompassing multiple regression model, except for the increased risk of SH2B3 genotype in IAA positive FDRs. Once again, the interaction effect of CTLA4 GA and HLA-DQ2/DQ8 overruled the effect of HLA-DQ2/DQ8 alone. None of the SNPs showed an interaction effect with the non-A*24 variable in the progression from one to multiple autoAbs, therefore, there is no indication that being negative for A*24 does affect the outcome of the SNP on the progression of autoimmunity. On the other hand, being positive for A*24 independently slows the progression from one to multiple autoAbs in our cohort regardless of the unveiled SNP interactions (Table 1, and [12]). This is in line with a previous report showing attenuated humoral responses in HLA-A*24 positive individuals at risk [51]. After addition of the SNP interaction effects (steps 2–5 in Table 2) to the previous predictive model (step 1 in Table 2) the AIC values decreased from 692.8 to 648.1, which makes our previous model rather less likely to contain the same amount of information [24] than the new model including interactions. This suggests that the non-HLA polymorphisms can indeed enhance our predictive models in a subgroup- and stage-specific way.
To conclude, our observations on non-HLA genes could provide additional tools to differentiate between rapid and slow progressors in the different stages of preclinical diabetes. They also emphasize the importance of stratification when analyzing time-to-event data or when preparing prevention trials, as non-HLA SNPs could induce significant effects in very specific subgroups. When assessing the risk of non-HLA polymorphisms in future studies, e.g. by composing risk scores for predicting preclinical progression rate, we thus suggest a stratified approach according to these specific subgroups, in contrast to a generalized approach where susceptibility genotypes are equally weighted in an entire study population. Of course, our findings now require confirmation in an independent cohort, but we hope that they can eventually contribute to the improvement of our understanding of the elusive hidden disease phase.
Abbreviations
- autoAb
autoantibody
- autoAb+
autoantibody positive
- BDR
Belgian Diabetes Registry
- FDRs
first-degree relatives
- GWAS
genome-wide association studies
- IA-2A
insulinoma associated-2 autoantibodies
- IAA
insulin autoantibodies
- ZnT8A
zinc transporter 8 autoantibodies
Acknowledgements
We thank Erik Quartier, Mays Al-Hachami, and Soukaina Ben Tato (VUB, Brussel, Belgium) for experimental assistance. The authors sincerely thank all BDR members who have contributed to the recruitment of relatives for this study. They are listed in Supplementary Appendix.
Ethical approval
This study was ethically approved under nr. BUN143201939922 by the institutional review board of Universitair Ziekenhuis Brussel (IRB UZB).
Conflict of interest
The authors declare that the research was conducted in the absence of any commercial or financial relationships that could be construed as a potential conflict of interest.
Funding
The study was supported by Grant WFWG18-18 of the “Wetenschappelijk Fonds Willy Gepts” of the University Hospital Brussels (UZ Brussel), and the Vrije Universiteit Brussel (Strategic Research Programmes [SRP] Growth 43 and SRP Spearhead 55).
Data availability
The datasets generated and/or analyzed during the present study are not openly available as they were derived from pseudonymized data and samples collected by the Belgian Diabetes Registry, a controlled access repository of sensitive human data. They can be made available upon reasonable request to coauthor Prof. Dr Bart Keymeulen, president of the Belgian Diabetes Registry.
Author contributions
J.V., M.V.D.C., and F.K.G. contributed to the study design, data acquisition, analysis and interpretation of data, and drafting of the article. B.J.V.D.A., A.K.D. contributed to data acquisition. S.T., P.G., C.D.B., and B.K. contributed to the study design. All the authors critically revised the manuscript and approved the version to be published. M.V.D.C. is the guarantor of this work and, as such, had full access to all the data in the study and takes responsibility for the integrity of the data and the accuracy of the data analysis.
References
Author notes
Members of the Belgian Diabetes Registry who enrolled patients for this study are listed in Supplementary Material.