-
PDF
- Split View
-
Views
-
Cite
Cite
Bhawana Singh, Manuel Mayr, Enhancing cardiovascular risk prediction through proteomics?, Cardiovascular Research, Volume 120, Issue 3, February 2024, Pages e2–e4, https://doi.org/10.1093/cvr/cvae031
- Share Icon Share
Despite major advances in the risk assessment tools for atherosclerotic cardiovascular disease (ASCVD), there is an unmet need to improve these tools beyond the traditional risk factors to capture the residual lipid and inflammatory risks.1–3 There are likely molecular targets beyond lipids that reduce ASCVD risk.2,3 Recent advances in high-throughput proteomics have enabled the characterization of plasma proteins in large-scale studies, thereby promoting proteomics discovery to the forefront of strategies to identify novel biomarkers.
Two commercial proteomics solutions spearheading the biomarkers discovery are the SomaScan assay from SomaLogic and the multiplexed proximity extension assay (PEA) from Olink™. The SomaScan assay relies on the binding of aptamers, nucleic acid oligomers, that are selected for specificity for certain proteins. Instead, the PEA from Olink™ relies on pairs of antibodies labelled with complementary DNA oligonucleotides, referred to as proximity probes. Both SomaScan and Olink™ are indirect quantification methods that rely on the specificity of binders. While SomaScan is purported to provide the most comprehensive proteome coverage in plasma, recent research comparing the Olink™ Explore 3072 and SomaScan v4 assays indicates that Olink™ provides superior precision and specificity.4 Concerns regarding the specificity of the SomaScan platform include the fact that it relies on single binders with the potential to bind to non-target proteins or different isoforms of the target protein.5 Additionally, some aptamers bind to multiple proteins, while some proteins are targeted by multiple aptamers.6
Helgason et al.6 utilized the SomaScan v4 platform, which profiles approximately 5000 plasma proteins. The study aimed to evaluate the utility of protein risk scores in improving the prediction of primary and secondary ASCVD events. The primary event study involved a retrospective study of a subset of individuals (n = 13 540) from the Icelandic Cancer Project and deCODE genetics, Iceland. The secondary event survey, drawn from the placebo group of the FOURIER clinical trial, was comprised of a subset of participants (n = 10 194) with established ASCVD. The study’s findings highlight the robustness of protein-based predictors across ancestries. In contrast, polygenic risk scores encounter challenges in effectively transferring across diverse racial groups, partly due to the limited data from genome-wide association studies in non-White populations. This disparity underscores the notable distinction between predictions based on protein and genetic scores, emphasizing the resilience of protein-based predictors.
Helgason et al.6 devised a protein risk score based on 70 proteins, derived from the primary event subsample using the lasso-penalized Cox method. Internal cross-validation utilized a random split of 70% for training and 30% for testing to select model hyperparameters. The age- and sex-adjusted Cox model revealed hazard ratios (HRs) of 1.93 [95% confidence interval (CI): 1.75–2.13] and 1.62 [95% CI: 1.48–1.79] for the protein risk score in primary and secondary ASCVD events, respectively. Further adjustment for clinical risk factors resulted in a decreased HR of 1.83 [95% CI: 1.64–2.04] for primary events and 1.35 [95% CI: 1.22–1.50] for secondary events. The authors conclude that, independent of the polygenic risk score, the protein risk score offers a modest improvement in discrimination capability when added to the clinical risk model—0.014 [95% CI, 0.002 to 0.028] in the primary event population and 0.026 [95% CI, 0.011 to 0.042] in secondary event subsample. Despite the study’s extensive scale, there are several constraints associated with this conclusion.
First, lasso-based protein selection has an inherent limitation of randomly choosing one protein among a correlated set, potentially impeding interpretation and affecting prediction power. Additionally, the Cox proportional hazard model, employed by the authors, assumes that risk factors modulate risk in a proportional manner. Secondly, the primary event samples lacked cholesterol and blood pressure (BP) measurements. Instead, the authors relied on available information on statin use (5.6%) and hypertension treatment (33.3%). Cholesterol and BP measurements were available for the secondary events cohort. However, in the secondary events cohort, the authors adapted two published secondary prevention risk scores (SMART2 and TRS2P) to derive clinical risk scores. These scores additionally included lipoprotein(a), apolipoprotein (apo) B, and apoA1.
Notably, the 70 proteins comprising the protein risk score did not include key apolipoproteins, suggesting potential accuracy issues in measuring well-established biomarkers for ASCVD with the SomaScan assay.5 However, other recognized cardiovascular biomarkers, such as NT-proBNP (N-terminal proBNP) and GDF-15 (growth/differentiation factor 15), along with proteins causally implicated in ASCVD, such as PCSK9 (proprotein convertase subtilisin/kexin type 9) and MMP-12 (macrophage metalloelastase),1,7,8 were present. Additionally, a nominal overlap of only six proteins was observed between these 70 proteins and the 27 proteins signature recently proposed by Williams et al.7 who also utilized SomaScan for a 4-year risk prediction. These six proteins were NT-proBNP, MMP-12, ANTR2 (anthrax toxin receptor 2), CILP2 (cartilage intermediate layer protein 2), CA2D3 (voltage-dependent calcium channel subunit alpha-2/delta-3), and TREM1 (triggering receptor expressed on myeloid cells 1). While the mechanistic links of several of these proteins to ASCVD are yet to be explored, it is known that TREM1 promotes the inflammatory signals initially triggered by Toll-like receptors.
Figure 1 illustrates the significance of understanding assay agreements and heterogeneity for a meaningful interpretation of protein measures using different proteomics platforms. Mass spectrometry (MS) remains the gold standard for protein quantitation5 as it does not rely on binders but measures peptides directly. Generally, there is a moderate to poor correlation for apolipoproteins measured by the SomaScan v4 assay and MS (Figure 1A) in the same individuals.9 Similarly, in the Icelandic dataset,4 utilized by Helgason et al.,6 a weak to moderate correlation of apolipoproteins was observed between Olink™ Explore 3072 and SomaScan assay v4 (Figure 1B). Regardless of the underlying reasons for these discordant results (binding to different proteoforms, misidentification, interference, and etc.), they underscore the imperative for orthogonal assay validation.
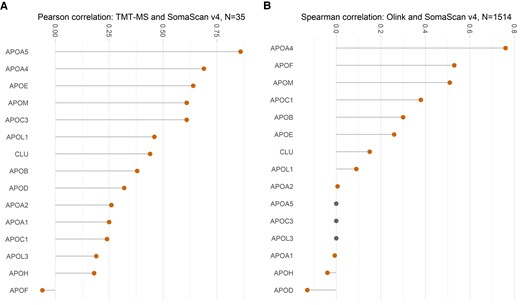
Correlation of apolipoprotein quantification by different proteomics platforms. (A) Pearson correlation between SomaScan v4 assay and multiplexed mass spectrometry by tandem mass tags (TMT-MS) in n = 35 individuals, as performed by Dammer et al.9 (B) Spearman correlation between SomaScan and Olink™ in n = 1514 participants undertaken by Eldjarn et al.4 The participants were a subset of individuals from the Icelandic Cancer Project and deCODE genetics, Iceland. Apolipoproteins (apo) A5, C3, and L3 were not measured by Olink™ and indicated by grey dots. Notably, only few apolipoproteins showed a correlation of >0.5. CLU denotes clusterin (apoJ). Plots were generated using ggplot package from R ver 4.2.2.
While commercial proteomic platforms offer valuable complementarity to MS in large-scale studies, they may prioritize quantity over the quality of protein measurements. The lack of reliable measurements for many apolipoproteins, arguably one of the most crucial categories of proteins related to ASCVD, weakens the study’s claim that a proteomics-based risk score can only moderately enhance the discrimination of clinical risk scores. Through targeted MS to accurately quantify apolipoproteins, we established an independent association between apolipoproteins and ASCVD, even after accounting conventional risk factors.10 While commercial solutions enhance the accessibility of proteomics, a thoughtful interpretation of such data is needed, as these methods rely on the specificity of binders and often provide discordant results. Consequently, the assessment of biomarker performance relies on assay performance rather than solely on the target protein itself.
‘But science is one of the very few human activities — perhaps the only one — in which errors are systematically criticized and fairly often, in time, corrected’ Karl R. Popper, Conjectures and Refutations: The Growth of Scientific Knowledge.
Funding
B.S. was funded by a British Heart Foundation (BHF) PhD studentship (FS/19/58/34895). M.M. is a BHF Chair Holder with BHF programme and project grant support (CH/16/3/32406, RG/F/21/110053, PG/20/10387). M.M. is also supported by the Fondation Leducq (18CVD02) and the excellence initiative VASCage (Centre for Promoting Vascular Health in the Ageing Community) of the Austrian Research Promotion Agency FFG (COMET programme—Competence Centers for Excellent Technologies, FFG project number 898252) funded by the Austrian Ministry for Transport, Innovation, and Technology; the Austrian Ministry for Digital and Economic Affairs; and the federal states Tyrol (via Standortagentur), Salzburg, and Vienna (via Vienna Business Agency).
References
Authors
Biography: Bhawana Singh is a British Heart Foundation final-year PhD student at King’s College London. She is a data scientist with the confluence of academic research and extensive work experience in information technology where she held senior management and leadership roles. After working for nearly two decades in the information technology sector, she went back to higher studies that took her to the path of undertaking a PhD. She holds a Master in data science and a Master of research in cardiovascular diseases from King’s College London. She is also a management graduate from S.P. Jain Institute of Management & Research, India, and holds a Bachelor of Science in Engineering, Electrical from Vinoba Bhave University, India. Her research focuses on leveraging cutting-edge data science techniques to study the molecules and proteins that alter with age and in disease. Ultimately, this could help identify early interventions and facilitate better risk stratification aimed towards increasing not just lifespan but also health span. Her academic accomplishments include an award for best MSc project across all the MSc programmes in the Department of Informatics, King’s College London. She was also awarded for best overall performance on the MSc in Data Science, King’s College London.
Biography: Manuel Mayr is the British Heart Foundation (BHF) Professor for Cardiovascular Proteomics. He qualified in Medicine from the University of Innsbruck (Austria) in 1999. He then moved to London to undertake a PhD on combining proteomics and metabolomics. Upon completion of his PhD in 2005, he achieved promotion to Professor in 2011. In 2017, he has been awarded a BHF Personal Chair, which he moved to Imperial College London in 2023. His group uses proteomics in combination with other -omics technologies to integrate biological information in disease-specific networks that drive pathophysiological changes. While studying molecular interactions has been a research focus for many years and has provided important insight into biology, the attention has now shifted towards a more integrative network biology approach (Nat Rev Cardiol. 2021;18(5):313–330). His academic achievements have been recognized by the inaugural Michael Davies Early Career Award of the British Cardiovascular Society (2007), the inaugural Bernard and Joan Marshall Research Excellence Prize of the British Society for Cardiovascular Research (2010), the Outstanding Achievement Award by the European Society of Cardiology Council for Basic Cardiovascular Science (2013), and the President’s Distinguished Lecture of the International Society for Heart Research (2022).
Author notes
Conflict of interest: M.M. filed and licensed patent applications on microRNAs as cardiovascular biomarkers.