-
PDF
- Split View
-
Views
-
Cite
Cite
Lee Lee Wong, Hiromi Wai Ling Koh, Hyungwon Choi, Ruiyang Zou, Lihan Zhou, Jia Yuen Lim, Dominic Cheng Yang Phua, Oi Wah Liew, Jenny Pek Ching Chong, Jessica Yan Xia Ng, Siew Pang Chan, Yei-Tsung Chen, Poh Shuan Daniel Yeo, Lieng Hsi Ling, David Sim, Kui Toh Gerard Leong, Hean Yee Ong, Fazlur Jaufeerally, Raymond Wong, Ping Chai, Adrian Fatt Hoe Low, Mayanna Lund, Gerry Devlin, Richard Troughton, Robert N Doughty, Carolyn Su Ping Lam, Heng Phon Too, Arthur Mark Richards, Combined circulating microRNA and peptide biomarkers for prognostication in heart failure, Cardiovascular Research, 2025;, cvaf065, https://doi.org/10.1093/cvr/cvaf065
- Share Icon Share
Time of primary review: 47 days
1. Introduction
Heart failure (HF) is a growing concern among the aging populations. Despite clear improvements in management, HF prognosis remains poor.1 After diagnosis, many patients suffer recurrent exacerbations leading to frequent hospitalizations and early mortality. While HF guidelines recommend using validated scoring systems to improve prognostication and tailor treatment,2,3 existing risk tools often lack the necessary accuracy for individualized patient management.
Biomarkers offer the prospect of strengthening existing risk stratification tools. The B-type natriuretic peptides (BNP) and its co-secreted congener, N-terminal pro-BNP (NT-proBNP) are established HF diagnostic and prognostic biomarkers.2 The high-sensitivity assays for cardiac troponin T or I (hs-TnT/I) are also well-proven as prognostic markers.4,5 Reports have examined the use of circulating microRNAs (miRNAs) as biomarkers in chronic HF; however, these studies examined only selected published miRNAs.6–8 We aimed to evaluate the prognostic value of circulating miRNAs and protein/peptide biomarkers, along with clinical variables, in predicting chronic HF outcomes using regularized Cox proportional-hazards (CoxPH) model. We hypothesized that a combination of markers would be a stronger predictor of all-cause mortality than any single biomarker alone. We demonstrated that our panel outperformed NT-proBNP and the MAGGIC score highlighting the value of a multi-variable model for prediction of all-cause mortality in HF patients (Figure 1A).
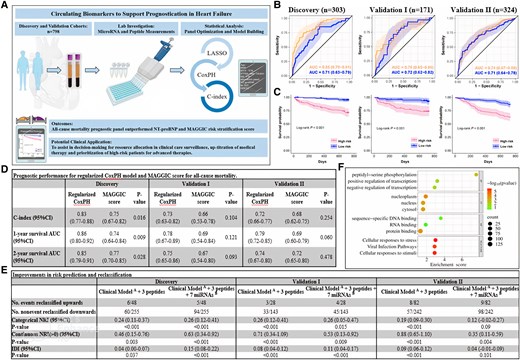
Multi-variable prognostic model for all-cause mortality. (A) Circulating biomarkers to support prognostication in heart failure. The illustrations included in the diagram are adapted from Servier Medical Art (https://smart.servier.com) and BioRender (https://biorender.com). (B) ROC curves compare the predictive ability of all-cause mortality prognostic panel (in orange) vs. NT-proBNP (in blue) across all three cohorts. (C) KM curves show event-free survival in HF patients’ risk-stratified above and below the median score derived from our model in all three cohorts. (D) Comparison of the performance of prognostic models with the MAGGIC score. DeLong’s test is used to compare two ROC curves. (E) Improvements in risk prediction and reclassification based on categorical and continuous NRI and IDI analyses. Patients were categorized into three groups using <5% (low risk), 5–15% (intermediate risk) and >15% (high risk) to reflect the tertile boundaries of the predicted risk scores at 2-year in the discovery, validation I and validation II cohorts. The P-value is calculated using likelihood ratio test. (F) The Reactome and GO analysis on target-pathway prediction. LASSO, least absolute shrinkage and selection operator; CoxPH, Cox proportional-hazards; C-index, Harrell’s concordance index; AUC, area under the receiver operating characteristic (ROC) curves; KM, Kaplan–Meier plots; NT-proBNP, N-terminal pro B-type natriuretic peptide; CI, confidence interval; NRI, net reclassification improvement; IDI, integrated discrimination improvement; Clinical ModelA, age, BMI, hypertension, NYHA class (III/IV) and history of MI; 3 peptides, NT-proBNP, hs-TnT, and GDF15; 7 miRNAsB, hsa-miR-150-5p, hsa-miR-181a-2-3p, hsa-miR-186-5p, hsa-miR-200c-3p, hsa-miR-337-3p, hsa-miR-421, and hsa-miR-503-5p.
2. Methods
2.1 Study cohort
Seven hundred ninety-eight HF patients from previously described Singapore SHOP and New Zealand (NZ) PEOPLE cohorts9 participated in this study. The Singapore discovery (n = 303), validation I (n = 171), and NZ validation II (n = 324) cohorts incurred an 18-month mortality of 48(15.8%), 28(16.4%), and 82(25.3%) cases, respectively. All patients had a confirmed clinical diagnosis meeting ESC criterion. Samples for peptide and miRNA assays were obtained after treatment was established when patients were judged to be in a compensated state. Patients were followed up for 2 years after the baseline visit. All participants provided informed consent. The study protocol adhered to the Declaration of Helsinki, and ethics approval was obtained (NHG/DSRB/2010/0114 and CTY/01.050062/AM11). Sample collection and processing details were described previously (Trial Registry ACTRN12610000374066).9,10
2.2 Measurement of peptides and miRNAs
Peripheral venous blood drawn into EDTA tubes was spun at 3500 g for 10 min, the plasma separated and stored at −80°C prior to assay in batches. Plasma NT-proBNP and hs-TnT were measured by electrochemiluminescence immunoassays on the Cobas e411 auto-analyser (Roche Diagnostics). Plasma GDF15 was measured by enzyme-linked immunosorbent assay (Quantikine®, R&D Systems). Total RNA was extracted using miRNeasy Serum/Plasma kit and QIAcube (Qiagen) semi-automated RNA purification system. Extracted miRNAs, spike-in and synthetic miRNAs used for standard curve were reverse transcribed and followed by multiplex augmentation (MiRXESTM). Singleplex qPCR was used to quantify the cDNA.10 We assayed 100 miRNAs for quantitative measurement using highly sensitive qPCR assays. These miRNAs were chosen based on their measurability, biologically plausible relevance to HF, and prior selection in our in-house studies.
2.3 Target prediction
We used MIENTURNET (http://userver.bio.uniroma1.it/apps/mienturnet/) to predict miRNA targets, focusing on experimentally validated interactions from miRTarbase. DAVID (Database for Annotation, Visualization and Integrated Discovery)11 was then used to analyse the enriched biological functions (Reactome and Gene Ontology pathways) of the genes.
2.4 Statistical analysis
Peptides and miRNA levels were log-transformed. The miRNAs absolute copy numbers were determined by interpolation of standard curves. To select a robust multi-variable panel, we used a machine learning approach based on penalized least absolute shrinkage and selection operator (LASSO). Through 10-fold cross-validation, we optimized a regularization parameter (λ) to derive a panel with the most predictive features that maximizes the Harrell’s C-index and minimizes the partial likelihood deviance of the model. The panel was assessed using C-index, and the predicted survival probabilities at 1-year and the end of the study were assessed using area under the curve (AUC) of the receiver operating characteristic (ROC) curves. Clinical endpoint event rates were compared using log-rank test and Kaplan–Meier (KM) curves. We fitted a regular CoxPH model starting with clinical variables as the base model and assessed the categorical and continuous net reclassification index (NRI) and integrated discrimination improvement (IDI) by adding miRNAs, and the peptide markers. The number of upward and downward reclassifications is defined as the correct movement of risk categories for events and non-event. All analyses were performed using R version 4.0.3, with a statistical significance level of P < 0.05.
3. Results
3.1 Biomarker panel for all-cause mortality
We used a regularized CoxPH model to identify a prognostic panel that considers all variables simultaneously for optimal prognostication. In addition to miRNAs and peptides, we included 13 important risk factors of mortality including age, gender, BMI, SBP, heart rate, hypertension, diabetes, NYHA class, eGFR (>60 mL/min/173 m2) and medical history of coronary artery disease and myocardial infarction, in our training model. A combination of five clinical variables (age, BMI, hypertension, NYHA class, and history of myocardial infarction), seven miRNAs (miR-150-5p, miR-181a-2-3p, miR-186-5p, miR-200c-3p, miR-337-3p, miR-421, and miR-503-5p), and three peptides (NT-proBNP, hs-TnT, and GDF15) was predictive of all-cause mortality with AUCs of 0.85 in discovery, 0.75 and 0.74 in validation I and II, respectively (Figure 1B). The panel outperformed NT-proBNP (Figure 1B) and the MAGGIC score12 (Figure 1D). KM curves revealed significant differences in survival between high- and low-risk HF subgroups (log-rank test, P < 0.001; Figure 1C) across all cohorts.
3.2 Improvement in risk prediction
Reclassification and discrimination were assessed using the clinical model as the reference standard. We then evaluated risk estimation improvement based on 1 subtracted by 2-year survival probabilities on adding (i) the peptides and (ii) peptides and miRNAs to the clinical model. Our model yielded largest improvement in classification accuracy and discrimination indices as compared with clinical model with peptide or miRNA. The NRI (>0) values were highly significant in discovery [NRI (>0): 0.63, P < 0.001], validation I [NRI (>0): 0.53, P = 0.009] and II [NRI (>0): 0.35, P = 0.004] cohorts. By categorizing the risk scores into low (<5%), intermediate (5–15%), and high risk (>15%), adding the three peptide and seven miRNAs to the clinical model resulted in an upward reclassification of 5/48 (10.4%) cases with events and a downward reclassification of 94/255 (36.9%) controls without events in the discovery cohort. In validation I and II, the upward reclassification was 4/28 (14.3%) and 9/82 (11.0%) cases with events and downward reclassification were 45/143 (31.5%) and 98/242 (40.5%) controls without events, respectively. The IDI results showed similar preference for our prognostic model (Figure 1E).
3.4 miRNA-target prediction
A total of 164 target genes associated with the seven miRNAs were analysed. Reactome and GO analyses revealed associations with cell responses to stress and stimuli, nucleoplasm, protein binding, and peptidyl-serine phosphorylation (Figure 1F)
4. Discussion
Prognostic models have been pursued in HF for decades. Several published risk models in predicting mortality in ambulatory HF patients had modest predictive power.13 Previous studies explored multi-marker miRNA models combining two miRNAs, NTproBNP, creatine, haemoglobin, age, and sex achieving C-statistics of 0.69–0.70 for all-cause mortality.6 We applied MAGGIC score12 to our cohort, which gave C-statistic of 0.70, 0.66, and 0.68 5 in discovery, validation I and II, respectively. Our multi-marker model significantly outperformed these models with a C-statistic of 0.85 in discovery and 0.74–0.75 in validations. In the validation cohorts, the proportion of patients later suffering events correctly assigned with higher risk scores in the clinical model with peptides and miRNAs compared to the model with only clinical variables were 14.3% in validation I and 11.0% in validation II, respectively. The proportions of patients who remained event-free correctly designated a lower risk score in the new model were 31.5% in validation I and 40.5% in validation II, respectively. Therefore, prognostic accuracy was improved for ∼40% or more of all participants by application of our multi-marker model relative to using a model with only clinical variables. The improved predictive performance gained from incorporation of clinical variables together with protein and nucleic acid markers within risk tools, has the potential to better guide clinical allocation of resources for closer surveillance, tailored up-titration of medical therapy and selection of HF patients for advanced therapies. Prospective evaluation is needed to fully assess its clinical impact and prognostic utility. The robust character of our findings was confirmed by reproduction of results derived within a cohort of Singapore HF patients with that of an independent, geographically, demographically, clinically and ethnically distinct cohort of NZ HF patients. We cannot rule out that other circulating miRNAs, not included in this study, may have better prognostic value. Also, we have yet to fully explore the mechanism underpinning the prognostic associations of our miRNAs. Our target prediction analysis identified miRNAs associated with cellular stress and stimuli response.14 Further studies will yield insights into how these miRNAs are related to the pathophysiology in HF.
Acknowledgements
We would like to thank the SHOP investigators: Poh Shuan Daniel Yeo, David Sim, Kui Toh Gerard Leong, Hean Yee Ong, Fazlur Jaufeerally, Raymond Wong, Ping Chai, Adrian Fatt Hoe Low, and the study participants and coordinators from SHOP and PEOPLE studies. We would like to acknowledge the nurses as well as colleagues from CVRI especially Cui Xia Ang, and Yu Pei Peh and our collaborator team members, Alrik Tan Kai Bin, Weng Ruifen, and Melissa Tang Xue Mei from DxD Hub, A*STAR, who contributed to this paper.
Funding
This work was supported by grants from National University of Singapore-Cardiovascular Research Institute Start-up Grant (R-172-000-206-733), National University of Singapore-Cardiovascular Research Institute Core Fund (N-172-000-047-001), National Medical Research Council-Translational and Clinical Research Flagship Programme Grant (NMRC/TCR/006-NUHS/213-Theme 2: NR13NMR108) and Centre Grant (SCEPTRE-Theme 2: CG-N172-000-089-001), Health Research Council of New Zealand Programme Grant (HRC 02/152), and Accelerate Technologies Pte Ltd, A*STAR Gap Fund ETPL/14-R15GAP-0017-C and ETPL/14-R15GAP-0017-J.
Data availability
All data supporting the findings reported herein will be shared upon reasonable request to the corresponding author.
References
Author notes
Lee Lee Wong and Hiromi Wai Ling Koh contributed equally.
Conflict of interest: R.Z., L.Z., and H.P.T. are the co-founders and founder respectively of MiRXES Pte Ltd. C.S.P. Lam is supported by a Clinician Scientist Award from the National Medical Research Council of Singapore; has received research support from NovoNordisk and Roche Diagnostics; has served as consultant or on the Advisory Board/Steering Committee/Executive Committee for Alleviant Medical, Allysta Pharma, AnaCardio AB, Applied Therapeutics, AstraZeneca, Bayer, Biopeutics, Boehringer Ingelheim, Boston Scientific, Bristol Myers Squibb, CardioRenal, Cytokinetics, Darma Inc., EchoNous Inc, Eli Lilly, Impulse Dynamics, Intellia Therapeutics, Ionis Pharmaceutical, Janssen Research & Development LLC, Medscape/WebMD Global LLC, Merck, Novartis, Novo Nordisk, Prosciento Inc, Quidel Corporation, Radcliffe Group Ltd., Recardio Inc, ReCor Medical, Roche Diagnostics, Sanofi, Siemens Healthcare Diagnostics and Us2.ai; and serves as co-founder and non-executive director of Us2.ai. Richard Troughton receives support from Merck, Roche Diagnostics, Bayer and American Reagent. A.M.R. has received support in kind from Roche Diagnostics, Abbott Laboratories and MiRXES and research grants from Roche Diagnostics, the National Medical Research Council of Singapore, the Health Research Council of New Zealand and the New Zealand Heart Foundation. Prof Richards currently holds the NZ Heart Foundation Chair of Cardiovascular Studies. The remaining authors have nothing to disclose.