-
PDF
- Split View
-
Views
-
Cite
Cite
Dimitrios Chatziisaak, Pascal Burri, Moritz Sparn, Dieter Hahnloser, Thomas Steffen, Stephan Bischofberger, Concordance of ChatGPT artificial intelligence decision-making in colorectal cancer multidisciplinary meetings: retrospective study, BJS Open, Volume 9, Issue 3, June 2025, zraf040, https://doi.org/10.1093/bjsopen/zraf040
- Share Icon Share
Abstract
The objective of this study was to evaluate the concordance between therapeutic recommendations proposed by a multidisciplinary team meeting and those generated by a large language model (ChatGPT) for colorectal cancer. Although multidisciplinary teams represent the ‘standard’ for decision-making in cancer treatment, they require significant resources and may be susceptible to human bias. Artificial intelligence, particularly large language models such as ChatGPT, has the potential to enhance or optimize the decision-making processes. The present study examines the potential for integrating artificial intelligence into clinical practice by comparing multidisciplinary team decisions with those generated by ChatGPT.
A retrospective, single-centre study was conducted involving consecutive patients with newly diagnosed colorectal cancer discussed at our multidisciplinary team meeting. The pre- and post-therapeutic multidisciplinary team meeting recommendations were assessed for concordance compared with ChatGPT-4.
One hundred consecutive patients with newly diagnosed colorectal cancer of all stages were included. In the pretherapeutic discussions, complete concordance was observed in 72.5%, with partial concordance in 10.2% and discordance in 17.3%. For post-therapeutic discussions, the concordance increased to 82.8%; 11.8% of decisions displayed partial concordance and 5.4% demonstrated discordance. Discordance was more frequent in patients older than 77 years and with an American Society of Anesthesiologists classification ≥ III.
There is substantial concordance between the recommendations generated by ChatGPT and those provided by traditional multidisciplinary team meetings, indicating the potential utility of artificial intelligence in supporting clinical decision-making for colorectal cancer management.
Introduction
Colorectal cancer (CRC) incidence is rising in conjunction with increasing complex management options1. The number of patients discussed at multidisciplinary team meetings (MDTs) is continuously rising, requiring further resources from healthcare professionals2,3. A modern solution that combines quality decision-making with minimizing workload is needed.
MDTs are considered the ‘standard’ in decision-making for patients with colorectal cancer. Decisions are made in an interdisciplinary and evidence-based manner, and patient-specific factors such as age, co-morbidities and personal history should be taken into account4,5. However, following their widespread implementation in everyday clinical practice, some limitations are acknowledged: MDTs are expensive, time-consuming and, because they are human-led committees, their decisions are heavily influenced by their synthesis, which is therefore inherently biased6.
Efficiency improvement measures have been investigated, such as teleconferencing, which at least partially increases performance7. Integrating artificial intelligence (AI) models into the decision-making process in MDTs has excellent potential8. In particular, the widespread use of large language models (LLMs), such as ChatGPT, could provide an approach to using AI technology in medicine9,10. This new technology has the potential to overcome the limitations of previous AI tools11.
LLMs demonstrate an ability to integrate clinical nuances with advanced algorithms, thereby showcasing their proficiency in various medical fields. These models are also capable of extending their utility to recommending medical examinations, providing literature support and enhancing doctor–patient interactions12. AI already accompanies the field of gastrointestinal malignancies, especially in radiology, in the so-called computer-aided diagnosis in screening13.
This study aimed to measure the congruence of MDT decisions compared with a LLM (ChatGPT-4).
Methods
Study design
Retrospective, single-centre, comparative study including a cohort of consecutive patients (≥18 years old) with newly diagnosed colorectal cancer (ICD-10 codes C18 to C20) who were discussed at the weekly MDT between September and December 2023. Both pretherapeutic and posttherapeutic MDT decisions were included.
All MDT recommendations are based on the German S3 guideline14 and internal hospital guidance, which is updated annually in line with the latest literature. MDTs are conducted according to the Swiss council of health directors with the presence of at least one medical expert of each field and conducted in a structured manner.
The institutional review board (swissethics.ch; Project-ID 2023-02170 EKOS 23/224) reviewed and approved this study. All participants provided written informed consent for using their anonymized data for research purposes.
A post-hoc power analysis was conducted to evaluate the adequacy of the sample size for this study. Assuming a small-to-medium effect size (Cohen’s W = 0.3) with a significance level of 0.05 and a desired power of 0.8, the required sample size was calculated to be 107 patients.
Data retrieval
ChatGPT-4 with team-subscription (OpenAI, San Francisco, USA) is an AI-based language model, which was used to formulate MDT recommendations. ChatGPT-4 was chosen because it cannot learn from previously answered questions, making each statement independent from others and, therefore, comparable15. In addition to demographic patient data, the results of the staging examinations that were available to the MDT at the time of the recommendation were recorded in a structured manner. This included the colonoscopy findings, including results from a tumour biopsy, thoraco-abdominal CT scan, pelvic MRI if available, surgical reports, the histology of the primary specimen and any postoperative imaging. The objective was to prompt ChatGPT-4 to propose a single treatment option for each case, in a manner that would emulate the decision-making process of an MDT. This process involves considering numerous potential alternatives and ultimately recommending the most optimal course of action.
Model input
The results of the staging examinations and the patient’s demographic data were transferred to ChatGPT in a structure similar to the one the patient was presented in the MDT. The original findings from the examination reports were used. They had not been filtered or interpreted in any way. The following ChatGPT query was used: ‘what is the treatment of the following patient according to the German S3 guideline? (X) years old (male/female) with an American Society of Anesthesiologists (ASA) classification (X) with (tumour). Clinic: (X). Colonoscopy: (X). Histology (X). CT thoracic/abdomen: (X). Tumour markers: (X). Additional diagnostics (for example gastroscopy, MRI): (X). Tell me your one suggestion that you think is best for the patient and their medical history and medical diagnosis?’ (Fig. 1).
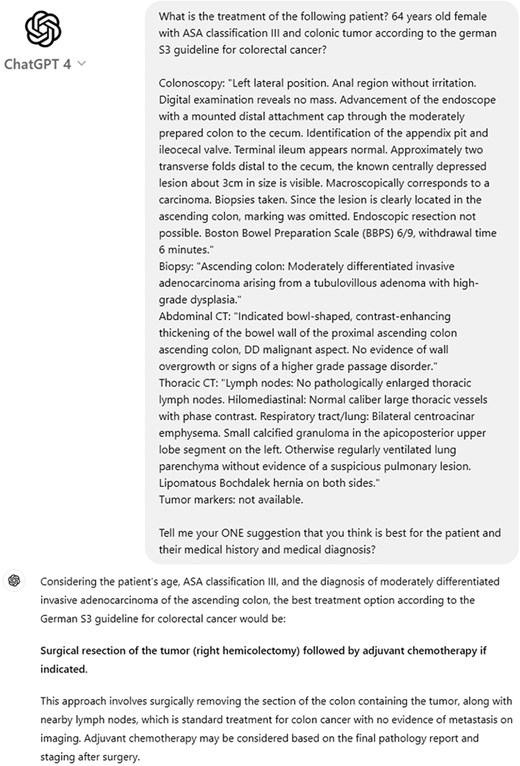
ChatGPT search query
Example of how the search query was designed in ChatGPT including the therapy recommendation received.
Comparative analysis
The AI-generated treatment recommendations were compared with the MDT recommendations on a patient-by-patient basis, focusing on the treatment decision, including surgical intervention and therapeutic strategy. Three reviewers (D.Ch., P.B., S.B.) independently reviewed each recommendation and assessed them independently for concordance between the MDT and the ChatGPT recommendations. Concordance was classified into three categories. Complete concordance was regarded as the maximum agreement. Partial concordance was defined as partial congruency with therapy-relevant differences, for example the omission of adjuvant chemotherapy as a possible option. Discordance was regarded as a significant divergence in recommendations, exemplified by contrasting curative and palliative concepts. Any disagreements were discussed and clarified among the reviewers.
Statistical analysis
P < 0.05 was deemed statistically significant. Continuous variables are presented as the mean (standard deviation; i.e. mean(s.d.)) if they are normally distributed or the median (range) if they are not normally distributed. Categorical variables are presented as frequencies (n, %). The descriptive analysis compares the proportions using the chi-square test16. One-way ANOVA was used to compare means of normally distributed continuous variables over the categories of categorical variables, and the Kruskal–Wallis test was used to compare medians of not normally distributed continuous variables over the categories of categorical variables. A multinomial logistic regression model was employed to analyse nominal outcome variables, whereby the log odds of the outcomes were represented as a linear combination of the predictor variables17,18.
Results
One hundred consecutive patients with newly diagnosed colorectal cancer of all stages were included. The study population’s baseline characteristics are described in Table 1. Except for two emergency operations for colonic ileus, 98 patients were discussed at the pretherapeutic MDT (MDT 1). Ninety-four patients underwent oncologic surgical resection, and 93 were discussed in the posttherapeutic MDT (MDT 2) (Fig. 2).
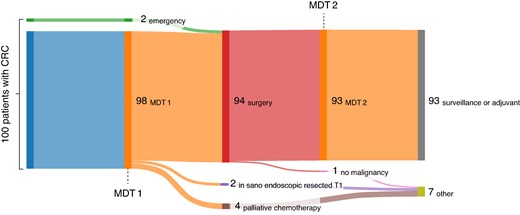
Sankey flow diagram
Sankey flow diagram shows the cohort of all included patients with colorectal cancer (CRC) through the multidisciplinary team meetings (MDTs).
. | Patients . | Percentage . |
---|---|---|
Age (years), mean (s.d.) | 70.1 (12.7) | |
Sex | ||
Male | 58 | 58 |
Female | 42 | 42 |
ASA classification | ||
ASA II | 50 | 50 |
ASA III | 46 | 46 |
ASA IV | 4 | 4 |
UICC stage | ||
Stage 0 | 2 | 2 |
Stage I | 32 | 32 |
Stage II | 28 | 28 |
Stage III | 23 | 23 |
Stage IV | 15 | 15 |
ICD10 classification | ||
Benign/polyp | 2 | 2 |
C18 (colonic) | 55 | 55 |
C19 (rectosigmoid junction) | 5 | 5 |
C20 (rectal) | 38 | 38 |
Concomitant secondary tumour* (bladder, lung, mamma, prostate, small bowel) | 18 | 18 |
. | Patients . | Percentage . |
---|---|---|
Age (years), mean (s.d.) | 70.1 (12.7) | |
Sex | ||
Male | 58 | 58 |
Female | 42 | 42 |
ASA classification | ||
ASA II | 50 | 50 |
ASA III | 46 | 46 |
ASA IV | 4 | 4 |
UICC stage | ||
Stage 0 | 2 | 2 |
Stage I | 32 | 32 |
Stage II | 28 | 28 |
Stage III | 23 | 23 |
Stage IV | 15 | 15 |
ICD10 classification | ||
Benign/polyp | 2 | 2 |
C18 (colonic) | 55 | 55 |
C19 (rectosigmoid junction) | 5 | 5 |
C20 (rectal) | 38 | 38 |
Concomitant secondary tumour* (bladder, lung, mamma, prostate, small bowel) | 18 | 18 |
Baseline demographic characteristics and tumour stage/classification of included patients. All values are n (%) unless otherwise indicated. *Present at the time of diagnosis of colorectal cancer. ASA, American Society of Anesthesiologists; UICC, Union for International Cancer Control; ICD, International Classification of Diseases.
. | Patients . | Percentage . |
---|---|---|
Age (years), mean (s.d.) | 70.1 (12.7) | |
Sex | ||
Male | 58 | 58 |
Female | 42 | 42 |
ASA classification | ||
ASA II | 50 | 50 |
ASA III | 46 | 46 |
ASA IV | 4 | 4 |
UICC stage | ||
Stage 0 | 2 | 2 |
Stage I | 32 | 32 |
Stage II | 28 | 28 |
Stage III | 23 | 23 |
Stage IV | 15 | 15 |
ICD10 classification | ||
Benign/polyp | 2 | 2 |
C18 (colonic) | 55 | 55 |
C19 (rectosigmoid junction) | 5 | 5 |
C20 (rectal) | 38 | 38 |
Concomitant secondary tumour* (bladder, lung, mamma, prostate, small bowel) | 18 | 18 |
. | Patients . | Percentage . |
---|---|---|
Age (years), mean (s.d.) | 70.1 (12.7) | |
Sex | ||
Male | 58 | 58 |
Female | 42 | 42 |
ASA classification | ||
ASA II | 50 | 50 |
ASA III | 46 | 46 |
ASA IV | 4 | 4 |
UICC stage | ||
Stage 0 | 2 | 2 |
Stage I | 32 | 32 |
Stage II | 28 | 28 |
Stage III | 23 | 23 |
Stage IV | 15 | 15 |
ICD10 classification | ||
Benign/polyp | 2 | 2 |
C18 (colonic) | 55 | 55 |
C19 (rectosigmoid junction) | 5 | 5 |
C20 (rectal) | 38 | 38 |
Concomitant secondary tumour* (bladder, lung, mamma, prostate, small bowel) | 18 | 18 |
Baseline demographic characteristics and tumour stage/classification of included patients. All values are n (%) unless otherwise indicated. *Present at the time of diagnosis of colorectal cancer. ASA, American Society of Anesthesiologists; UICC, Union for International Cancer Control; ICD, International Classification of Diseases.
In MDT 1, complete concordance of 72.5% (71 patients, 95% c.i.: 62.5%-81%) and partial concordance of 10.2% (10 patients, 95% c.i.: 5%-18%) was achieved; 17.3% (17 patients, 95% c.i.: 10.4%-26.3%) of the results were discordant. In MDT 2, complete concordance of 82.8% (77 patients, 95% c.i.: 73.6%-89.9%) and partial concordance of 11.8% (11 patients, 95% c.i.: 6.1%-20.2%) were observed; discordance was observed in 5.4% (5 patients, 95% c.i.: 1.8%-12.2%). A statistically significant difference was noted in the distribution of ‘concordance/partial concordance/discordance’ of the suggestions between the preoperative and postoperative decisions (χ2(2) = 6.71, P = 0.035). This is primarily due to the higher percentage of discordance in MDT 1 in contrast to MDT 2, with a corresponding increase in the concordance rates (72.5% versus 82.8%).
In order to determine the alignment of the treatment recommendations provided by MDT and ChatGPT with those outlined in the German S3 clinical practice guideline, a comparative analysis was undertaken19. A statistically significant difference was observed in the distribution of pretherapeutic decisions (χ2(2) = 16.18, P < 0.001). This was demonstrated in instances of partial concordance and discordance. In instances of partial concordance between MDT 1 and ChatGPT, both decisions concurred with the German S3 guidelines in 70% of cases (7 patients). In cases of discordance, the MDT decision was found to be 94.1% (16 patients) in agreement with the German S3 guidelines. The same analysis failed to show a statistically significant dependency for MDT 2 (χ2(2) = 5.72, P = 0.057) (Fig. 3).
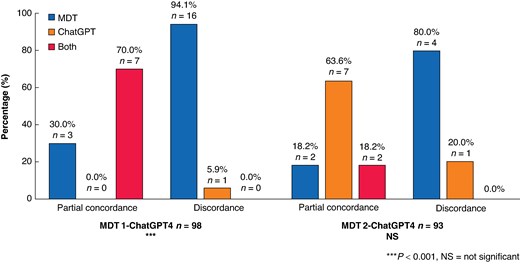
Concordance of the decisions with the German S3 guidelines
Distribution (%) of the side that had the correct recommendation after partial agreement or disagreement before surgery and after surgery.
A subgroup ANOVA was conducted to investigate the potential influence of risk factors on the decision-making process between MDT and ChatGPT. One objective of this analysis was to ascertain whether there were significant differences in the mean age of patients across the categories of concordance, partial concordance and discordance. A significant difference was observed in the distribution of responses (F (2,95) = 4.47, 95% c.i., P = 0.014) in MDT 1. The post-hoc analysis indicated a significant difference between the mean age for concordance and discordance (P = 0.011), indicating a notable correlation between age (mean: 77.6 years, (13)) and probability of discordance compared with those patients who exhibited concordance (67.8 years) (Table 2). Although not significant, the same tendency to disagree with the proposed therapies was also observed in older patients (77.6 years old, s.d. ± 13) in MDT 2 (F(2,90) = 0.62, 95% c.i., P = 0.543). Patients with rectal cancer (ICD10: C20) had significantly more cases of partial agreement (80%) in MDT 1 (χ2(4) = 9.89, 95% c.i., P = 0.042). A significant dependence was demonstrated in patients with an ASA score of IV (χ2(4) = 22.31, 95% c.i., P < 0.001), with 23.5% of cases showing discordance at MDT 1. A significant correlation was found in patients with a pN1 stage and partial concordance. In this subgroup of patients, partial concordance was found in 50% of cases (χ2(4) = 9.63, 95% c.i., P = 0.047) (Table 2). The same subgroup analysis showed no significant dependence for other risk factors. No significant differences or dependencies were found concerning the subgroup analysis on MDT 2. In the multinomial logistic regression analysis, a significant difference in the patients with ASA score III compared with those with ASA score IV regarding patient’s age was found (F(2.97) = 3.50, 95% c.i., P = 0.034 ASA score III to ASA score IV). Because of this statistically strong dependence, only the age of the patients was used in the in-depth analysis.
. | Concordance . | Partial concordance . | Discordance . | . |
---|---|---|---|---|
Age (years), mean (s.d.) | 67.8 (12.1) | 70.4 (11.6) | 77.6 (13) | F(2,95) = 4.47, P = 0.014 Concordance-discordance, P = 0.011 |
Cancer type (C18-C21) | X2(4) = 9.89, P = 0.042 | |||
C18 (0–8) | 43 (60.6) | 1 (10.0) | 9 (52.9) | |
C19 | 4 (5.6) | 1 (10.0) | 2 (11.8) | |
C20 | 24 (33.8) | 8 (80.0) | 6 (35.3) | |
ASA score | X2(4) = 22.31, P < 0.001 | |||
II | 40 (56.3) | 5 (50.0) | 4 (23.5) | |
III | 31 (43.7) | 5 (50.0) | 9 (53.0) | |
IV | 0 (0.0) | 0 (0.0) | 4 (23.5) | |
Tumour T stage | X2(8) = 4.62, P = 0.798 | |||
0 | 6 (9.2) | 0 (0.0) | 0 (0.0) | |
1 | 8 (12.3) | 2 (22.2) | 3 (17.6) | |
2 | 12 (18.5) | 2 (22.2) | 4 (23.5) | |
3 | 30 (46.2) | 4 (44.5) | 6 (35.4) | |
4 | 9 (13.8) | 1 (11.1) | 4 (23.5) | |
Lymph node N stage | X2(4) = 9.63, P = 0.047 | |||
0 | 50 (76.9) | 3 (37.5) | 14 (82.4) | |
1 | 8 (12.3) | 4 (50.0) | 3 (17.6) | |
2 | 7 (10.8) | 1 (12.5) | 0 (0.0) |
. | Concordance . | Partial concordance . | Discordance . | . |
---|---|---|---|---|
Age (years), mean (s.d.) | 67.8 (12.1) | 70.4 (11.6) | 77.6 (13) | F(2,95) = 4.47, P = 0.014 Concordance-discordance, P = 0.011 |
Cancer type (C18-C21) | X2(4) = 9.89, P = 0.042 | |||
C18 (0–8) | 43 (60.6) | 1 (10.0) | 9 (52.9) | |
C19 | 4 (5.6) | 1 (10.0) | 2 (11.8) | |
C20 | 24 (33.8) | 8 (80.0) | 6 (35.3) | |
ASA score | X2(4) = 22.31, P < 0.001 | |||
II | 40 (56.3) | 5 (50.0) | 4 (23.5) | |
III | 31 (43.7) | 5 (50.0) | 9 (53.0) | |
IV | 0 (0.0) | 0 (0.0) | 4 (23.5) | |
Tumour T stage | X2(8) = 4.62, P = 0.798 | |||
0 | 6 (9.2) | 0 (0.0) | 0 (0.0) | |
1 | 8 (12.3) | 2 (22.2) | 3 (17.6) | |
2 | 12 (18.5) | 2 (22.2) | 4 (23.5) | |
3 | 30 (46.2) | 4 (44.5) | 6 (35.4) | |
4 | 9 (13.8) | 1 (11.1) | 4 (23.5) | |
Lymph node N stage | X2(4) = 9.63, P = 0.047 | |||
0 | 50 (76.9) | 3 (37.5) | 14 (82.4) | |
1 | 8 (12.3) | 4 (50.0) | 3 (17.6) | |
2 | 7 (10.8) | 1 (12.5) | 0 (0.0) |
Values are n (%) unless otherwise stated. Subgroup analysis of the treatment recommendations of ChatGPT of multidisciplinary team 1 by category. Statistically significant P-values are highlighted in bold. ASA, American Society of Anesthesiologists.
. | Concordance . | Partial concordance . | Discordance . | . |
---|---|---|---|---|
Age (years), mean (s.d.) | 67.8 (12.1) | 70.4 (11.6) | 77.6 (13) | F(2,95) = 4.47, P = 0.014 Concordance-discordance, P = 0.011 |
Cancer type (C18-C21) | X2(4) = 9.89, P = 0.042 | |||
C18 (0–8) | 43 (60.6) | 1 (10.0) | 9 (52.9) | |
C19 | 4 (5.6) | 1 (10.0) | 2 (11.8) | |
C20 | 24 (33.8) | 8 (80.0) | 6 (35.3) | |
ASA score | X2(4) = 22.31, P < 0.001 | |||
II | 40 (56.3) | 5 (50.0) | 4 (23.5) | |
III | 31 (43.7) | 5 (50.0) | 9 (53.0) | |
IV | 0 (0.0) | 0 (0.0) | 4 (23.5) | |
Tumour T stage | X2(8) = 4.62, P = 0.798 | |||
0 | 6 (9.2) | 0 (0.0) | 0 (0.0) | |
1 | 8 (12.3) | 2 (22.2) | 3 (17.6) | |
2 | 12 (18.5) | 2 (22.2) | 4 (23.5) | |
3 | 30 (46.2) | 4 (44.5) | 6 (35.4) | |
4 | 9 (13.8) | 1 (11.1) | 4 (23.5) | |
Lymph node N stage | X2(4) = 9.63, P = 0.047 | |||
0 | 50 (76.9) | 3 (37.5) | 14 (82.4) | |
1 | 8 (12.3) | 4 (50.0) | 3 (17.6) | |
2 | 7 (10.8) | 1 (12.5) | 0 (0.0) |
. | Concordance . | Partial concordance . | Discordance . | . |
---|---|---|---|---|
Age (years), mean (s.d.) | 67.8 (12.1) | 70.4 (11.6) | 77.6 (13) | F(2,95) = 4.47, P = 0.014 Concordance-discordance, P = 0.011 |
Cancer type (C18-C21) | X2(4) = 9.89, P = 0.042 | |||
C18 (0–8) | 43 (60.6) | 1 (10.0) | 9 (52.9) | |
C19 | 4 (5.6) | 1 (10.0) | 2 (11.8) | |
C20 | 24 (33.8) | 8 (80.0) | 6 (35.3) | |
ASA score | X2(4) = 22.31, P < 0.001 | |||
II | 40 (56.3) | 5 (50.0) | 4 (23.5) | |
III | 31 (43.7) | 5 (50.0) | 9 (53.0) | |
IV | 0 (0.0) | 0 (0.0) | 4 (23.5) | |
Tumour T stage | X2(8) = 4.62, P = 0.798 | |||
0 | 6 (9.2) | 0 (0.0) | 0 (0.0) | |
1 | 8 (12.3) | 2 (22.2) | 3 (17.6) | |
2 | 12 (18.5) | 2 (22.2) | 4 (23.5) | |
3 | 30 (46.2) | 4 (44.5) | 6 (35.4) | |
4 | 9 (13.8) | 1 (11.1) | 4 (23.5) | |
Lymph node N stage | X2(4) = 9.63, P = 0.047 | |||
0 | 50 (76.9) | 3 (37.5) | 14 (82.4) | |
1 | 8 (12.3) | 4 (50.0) | 3 (17.6) | |
2 | 7 (10.8) | 1 (12.5) | 0 (0.0) |
Values are n (%) unless otherwise stated. Subgroup analysis of the treatment recommendations of ChatGPT of multidisciplinary team 1 by category. Statistically significant P-values are highlighted in bold. ASA, American Society of Anesthesiologists.
The results of the multinomial logistic regression analysis for MDT 1 indicate that the age of the patients is a significant risk factor for discordance relative to concordance (P = 0.008, OR 1.084, 95% c.i. 1.021–1.15), with the cut-off approaching 77 years of age in MDT 1 (Fig. 4). The effect size was found to be relatively modest. Accordingly, the significant risk factors for partial concordance over concordance are the presence of rectal cancer (C20) (P = 0.028, OR 16.046, 95% c.i. 1.357–189.787) and the N status (P = 0.006, OR 19.099, 95% c.i. 2.37–153.934). This indicates that patients with rectal cancer have a 16.04% greater chance for partial discordance, and the patients with N1 nodal status have 19.09% partial concordance between ChatGPT and MDT suggestions in MDT 1.
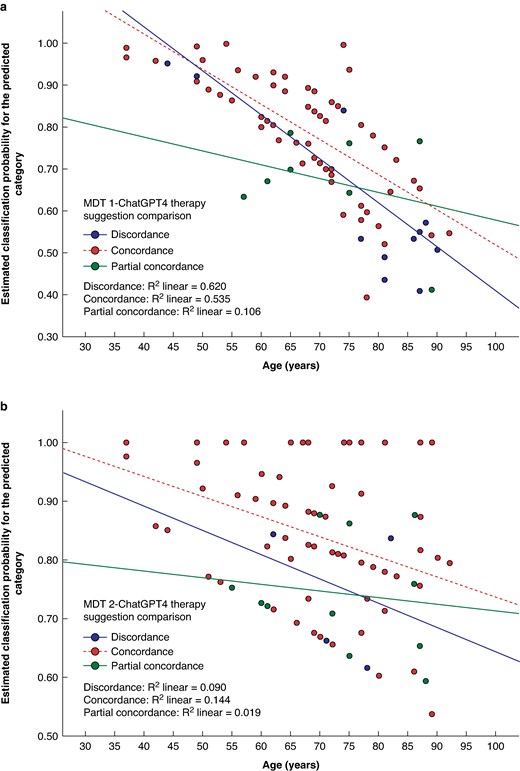
Age cut-off in suggestion comparison
Scatter plot of age and estimated classification probability for the predicted category from multinomial logistic regression model a preoperative multidisciplinary team (MDT 1) and b postoperative MDT 2.
The multinomial logistic regression analysis of the therapeutic recommendations for MDT 2 did not demonstrate any significant risk factor concerning the decisions or specific age cut-off (Fig. 4).
A further analysis to ascertain whether there is a difference in the distribution of proposals between MDT and ChatGPT concerning the initial and subsequent decisions after excluding the high-risk groups (patients older than 77 years old and ASA score more than III) was conducted. The analysis yielded a concordance rate of 85.1% (40 of 47 patients), with a mere 4.3% (2 patients) exhibiting discordance in the initial decision. Regarding the MDT 2, a concordance rate of 80.4% (37 of 46 patients) and a discordance rate of 8.7% (4 patients) was observed. The distribution of answers exhibited no significant deviation (χ2(2) = 0.77, 95% c.i., P = 0.679), signalling a more normal distribution of decisions (Table 3).
. | MDT 1–ChatGPT-4 (n = 47) . | MDT 2–ChatGPT-4 (n = 46) . | . | ||
---|---|---|---|---|---|
. | . | 95% c.i. . | . | 95% c.i. . | Statistic . |
Concordance | 40 (85.1) | 71.7–93.8 | 37(80.4) | 66.1–90.6 | X2(2) = 0.77, P = 0.679 |
Partial concordance | 5 (10.6) | 3.5–23.1 | 5(10.9) | 3.6–23.6 | |
Discordance | 2 (4.3) | 0.5–14.5 | 4(8.7) | 2.4–20.8 |
. | MDT 1–ChatGPT-4 (n = 47) . | MDT 2–ChatGPT-4 (n = 46) . | . | ||
---|---|---|---|---|---|
. | . | 95% c.i. . | . | 95% c.i. . | Statistic . |
Concordance | 40 (85.1) | 71.7–93.8 | 37(80.4) | 66.1–90.6 | X2(2) = 0.77, P = 0.679 |
Partial concordance | 5 (10.6) | 3.5–23.1 | 5(10.9) | 3.6–23.6 | |
Discordance | 2 (4.3) | 0.5–14.5 | 4(8.7) | 2.4–20.8 |
Values are n (%) unless otherwise stated. Therapy suggestion comparison for preoperative or postoperative decision between MDT and ChatGPT after excluding risk factor patients (ASA score III–IV, and age > 77 years old). MDT, multidisciplinary team.
. | MDT 1–ChatGPT-4 (n = 47) . | MDT 2–ChatGPT-4 (n = 46) . | . | ||
---|---|---|---|---|---|
. | . | 95% c.i. . | . | 95% c.i. . | Statistic . |
Concordance | 40 (85.1) | 71.7–93.8 | 37(80.4) | 66.1–90.6 | X2(2) = 0.77, P = 0.679 |
Partial concordance | 5 (10.6) | 3.5–23.1 | 5(10.9) | 3.6–23.6 | |
Discordance | 2 (4.3) | 0.5–14.5 | 4(8.7) | 2.4–20.8 |
. | MDT 1–ChatGPT-4 (n = 47) . | MDT 2–ChatGPT-4 (n = 46) . | . | ||
---|---|---|---|---|---|
. | . | 95% c.i. . | . | 95% c.i. . | Statistic . |
Concordance | 40 (85.1) | 71.7–93.8 | 37(80.4) | 66.1–90.6 | X2(2) = 0.77, P = 0.679 |
Partial concordance | 5 (10.6) | 3.5–23.1 | 5(10.9) | 3.6–23.6 | |
Discordance | 2 (4.3) | 0.5–14.5 | 4(8.7) | 2.4–20.8 |
Values are n (%) unless otherwise stated. Therapy suggestion comparison for preoperative or postoperative decision between MDT and ChatGPT after excluding risk factor patients (ASA score III–IV, and age > 77 years old). MDT, multidisciplinary team.
Discussion
ChatGPT interprets an age over 77 years and an ASA ≥ III as a risk factor for surgical or adjuvant therapy, which correlates with the German S3 guidelines14. However, the AI model lacks the ability to account for factors such as frailty, functional reserve or psychosocial determinants, which play a critical role in MDT decision-making. Additionally, ChatGPT relies solely on textual data of imaging findings, which limits its capacity to replicate MDT decisions based on nuanced visual data from CT or MRI scans. This results in the recommendation for palliative care and, therefore, partial concordance or discordance compared with the recommendations of the MDT, which were proven to align with the current guidelines.
It is proposed that the above statistically significant discrepancy in the discordance rates of MDT 1 in comparison to MDT 2 is strongly correlated with the fact that at the time of the initial MDT 1, a decision was being made as to whether the patient should receive a curative therapeutic approach or be placed in a palliative setting. Our statistical analysis revealed that the AI model categorized patients above 77 years of age and ASA ≥ III as palliative care cases. One of the objectives of this retrospective study was to ascertain whether the AI model could respond following the prevailing guidelines if the human logical analysis of the cases were to be excluded from the equation. This is a current limitation of the model in its broad use without the involvement of human interaction and MDT. Following the exclusion of the patients mentioned above from the statistical comparison between the two decisions, it becomes evident that the distribution of answers between MDT and ChatGPT exhibits no statistically significant difference, thereby indirectly supporting our initial assumption.
In this cohort, ChatGPT demonstrated an ability to interpret more complex data. This involved considering details of the pathological reporting system and attempting to restage the patients. Current literature describes the current potential of the new AI protocols, and could improve decision-making within the next few years20.
The primary concern surrounding using AI models in medical decision-making pertains to the reliability of the AI-generated responses and the potential for AI hallucination (generated response that presents false or misleading information in a manner that is perceived as factual)21,22. One key limitation observed in ChatGPT’s performance was its tendency to oversimplify complex clinical scenarios. Whilst in this study no patients had false or misleading (‘hallucinated’) recommendations detected, the possibility remains in scenarios involving incomplete or ambiguous inputs. It is evident that rigorous clinical supervision and monitoring of the AI-derived decisions is imperative to prevent any potential misinterpretations.
In their publication, Choo et al. employed an earlier iteration of ChatGPT in the context of well defined complex colorectal cases, reporting an 86.7% concordance between MDT and ChatGPT decisions23. However, the patient’s data had been prefiltered and processed before being entered into ChatGPT. The findings of this study align with those of that previous investigation, demonstrating a notably high pretherapeutic concordance rate (72.5%). When partially concordant cases, defined as those where the treatment strategies do not diverge completely, are included, the concordance figures for MDT 1 increase to 82.7%. Furthermore, the concordance figures for MDT 2 are particularly encouraging, with a rate of 94.6% complete or partial concordance.
In another publication there was a limited utilization of LLMs in the decision-making process for acute surgical problems, such as appendicitis or cholecystitis24. Conversely, the data in this study indicated a high concordance with MDT suggestions. It is hypothesized that these discrepancies may be attributed to the differing natures of the medical problems under consideration in the two studies.
In the present study, a consecutive cohort of patients from a single centre were recruited for a retrospective analysis that mimicked the enrolment of patients in the MDT setting. The only restriction was the type of malignancy (colorectal cancer only). This allowed the challenges associated with decision-making in a real-life MDT to be considered. Previous studies had significantly limited the characteristics of the patient cohort presented19,23.
This study offers preliminary insights into the potential utility of ChatGPT in colorectal cancer management. However, as a retrospective study, it is inherently limited in establishing causal relationships. Further prospective studies are needed to validate the benefit of ChatGPT in MDT. In a prospective setting, the ChatGPT recommendation may be directly compared with the tumour board decision in the first step. In the second step, the ChatGPT recommendation may be integrated into the tumour board discussion. While the single-centre approach ensures uniformity in methodology and decision-making standards, it limits the generalizability of the findings. Multicentre studies, encompassing diverse patient populations and varying MDT practices, are needed to establish external validity.
In any research into the potential applications of AI models in medical decision-making, it is essential to recognize that these tools can provide supplementary support, rather than replace the complex, nuanced processes involved in the decision-making of an MDT, at least for the time being. Although anonymized patient data has been used to mitigate immediate concerns related to privacy and consent, broader integration of AI tools into clinical practice raises critical ethical and legal questions. Standardized frameworks for liability management are essential to address these challenges. Explicit patient consent for the use of AI in clinical decision-making may become a necessary ethical standard to ensure transparency, trust and patient autonomy.
Funding
The authors have no funding to declare.
Acknowledgements
The authors thank Angelos Vouris for the statistical analysis and support.
Disclosure
The authors declare no conflict of interest.
Data availability
The data underlying this article will be shared on reasonable request to the corresponding author.
Author contributions
Dimitrios Chatziisaak (Conceptualization, Data curation, Investigation, Methodology, Visualization, Writing—original draft, Writing—review & editing), Pascal Burri (Data curation, Investigation, Writing—review & editing), Moritz Sparn (Writing—review & editing), Dieter Hahnloser (Methodology, Supervision, Writing—review & editing), Thomas Steffen (Methodology, Supervision, Writing—review & editing) and Stephan Bischofberger (Conceptualization, Methodology, Project administration, Supervision, Visualization, Writing—original draft, Writing—review & editing).