-
PDF
- Split View
-
Views
-
Cite
Cite
Lachlan Dick, Connor P Boyle, Richard J E Skipworth, Douglas S Smink, Victoria Ruth Tallentire, Steven Yule, Automated analysis of operative video in surgical training: scoping review, BJS Open, Volume 8, Issue 5, October 2024, zrae124, https://doi.org/10.1093/bjsopen/zrae124
- Share Icon Share
Abstract
There is increasing availability of operative video for use in surgical training. Emerging technologies can now assess video footage and automatically generate metrics that could be harnessed to improve the assessment of operative performance. However, a comprehensive understanding of which technology features are most impactful in surgical training is lacking. The aim of this scoping review was to explore the current use of automated video analytics in surgical training.
PubMed, Scopus, the Web of Science, and the Cochrane database were searched, to 29 September 2023, following PRISMA extension for scoping reviews (PRISMA-ScR) guidelines. Search terms included ‘trainee’, ‘video analytics’, and ‘education’. Articles were screened independently by two reviewers to identify studies that applied automated video analytics to trainee-performed operations. Data on the methods of analysis, metrics generated, and application to training were extracted.
Of the 6736 articles screened, 13 studies were identified. Computer vision tracking was the common method of video analysis. Metrics were described for processes (for example movement of instruments), outcomes (for example intraoperative phase duration), and critical safety elements (for example critical view of safety in laparoscopic cholecystectomy). Automated metrics were able to differentiate between skill levels (for example consultant versus trainee) and correlated with traditional methods of assessment. There was a lack of longitudinal application to training and only one qualitative study reported the experience of trainees using automated video analytics.
The performance metrics generated from automated video analysis are varied and encompass several domains. Validation of analysis techniques and the metrics generated are a priority for future research, after which evidence demonstrating the impact on training can be established.
Introduction
Surgery, a complex and high-stakes medical intervention, requires technical finesse, as well as shrewd decision-making. Improving patient outcomes is of particular importance, given approximately half a million operations are performed worldwide each day1. Whilst many factors associated with a poorer postoperative outcome are challenging to modify (for example functional status)2, two-thirds of adverse events in surgical patients occur during the intraoperative phase3, with over half being preventable (for example bleeding)4. Good technical performance is associated with reduced mortality in patients undergoing gastric bypass surgery5 and is a predictor of postoperative complications after surgery for gastric cancer6. Non-technical skills of surgery, including decision-making, situational awareness, communication, teamwork, and leadership7, are equally influential with regard to performance, with breakdown in team communication being the most common failure identified from adverse intraoperative events3.
Assessment of intraoperative skills is an important aspect of surgical training. The Objective Structured Assessment of Technical Skills (OSATS) and its multiple derivatives can reliably assess technical proficiency8 and demonstrate development over time9. However, such assessment is time-consuming to perform, resource intensive, and prone to observer bias. The methods of non-technical skill assessment (for example the Non-Technical Skills for Surgeons (NOTSS) framework), although validated10, face similar challenges when implemented in the real-world environment.
Since its inception, minimally invasive surgery (MIS) has become the established surgical approach across multiple specialties11. Given the nature of MIS, the potential availability of video for education is vast and its use is supported by the current literature. Multiple-choice test performance and intraoperative knowledge of medical students are superior when operative video-based teaching is used, compared with conventional methods12,13. For surgical trainees, improved performance, scored using a global assessment scale, is also observed in laparoscopic colorectal surgery14 and the performance of surgical trainees can be further improved with the addition of expert coaching15.
Perhaps the most utilized application of operative video is post-hoc review. For trainees performing inguinal hernia repair, postoperative video-based assessment is feasible16 and, after reviewing the video footage with a supervising surgeon, subsequent performance has been shown to improve17. The limitations of facilitated debriefing using video are that it is time-consuming to perform and inter-observer variation can make it difficult to determine a trainee’s true level of competence. Alternatively, self-assessment of operative video is associated with a reduced learning curve and circumvents the need for expert involvement18. However, negative correlation has been shown between performance and self-assessment scores, with a concerning trend for individuals who perform poorly to rate themselves highly19.
In recent years, the development of artificial intelligence (AI) in healthcare has increased rapidly20. Whilst there are now numerous clinical applications21, an emerging field is the use of AI to enhance surgical education. The accuracy of ChatGPT (OpenAI, San Francisco, CA, USA), an application based on large language models, with regard to answering board examination questions in orthopaedics has been explored22 and advanced simulators now allow trainees to increase caseload attainment, without exposing patients to harm, in urological settings23. An even more promising area of development is the analysis of video to assess operative performance. Motion tracking has been applied with favourable results in simulated settings for both open and laparoscopic skills24,25. However, these studies required the use of physical markers on the target object, limiting the transferability into the live operating room environment. Recently, more advanced techniques that can analyse operative video and produce performance metrics without the need for adjuncts have been developed26. Automated analysis of non-technical skills using operative video has also been trialled27.
Given the challenges of traditional methods of skill assessment and the need to train surgeons who are competent, there is a growing need for an objective and reliable method of providing feedback that is complementary to expert-based assessment. With the wealth of video available, alongside rapidly developing technologies that can perform analytics, there is the potential to provide meaningful feedback on performance. The aim of this scoping review was to explore the current uses of automated analyses from real-world operative video in the context of surgical training.
Methods
The framework developed by Arksey and O’Malley28 and the PRISMA extension for scoping reviews (PRISMA-ScR)29 were used in the design, conduct, and reporting of this scoping review. A scoping review was chosen to gain a broad understanding of this emerging field in surgical education.
Definitions
Automated operative video analytics was defined as the use of footage from any part of a real-world operation that had non-human assessment applied. The assessment could be performed during or after the operation. Application to surgical training was defined as inclusion of surgical trainees or fellows in the study and production of metrics from the automated assessment that were used to guide feedback and training.
Search strategy
Electronic searches of PubMed, Scopus, the Web of Science, and the Cochrane database were performed from database inception to 29 September 2023. The search criteria were developed with the assistance of a medical librarian with key search terms including ‘surgeon’, ‘trainee’, ‘resident’, ‘video analytics’, ‘education’, and ‘training’ combined with the Boolean operators AND and OR. The full search criteria for each database are available in the Supplementary Methods. The reference lists of the included studies were also searched for relevant articles. Covidence (Veritas Health Innovation, Melbourne, Victoria, Australia) was used to manage references throughout the review and extraction process.
Study eligibility
Given the broad search criteria adopted, title screening was conducted by a single reviewer (L.D.), with sensitivity prioritized over specificity, to prevent exclusion of potentially relevant studies. Abstract and full-text screening was performed independently by two reviewers (L.D. and C.P.B.). Disagreements were settled by either consensus or discussion with a third reviewer (S.Y.). Full texts were considered to have met the inclusion criteria when: at least one method of automated video analysis was used; the setting was a real-world operation; operative video performed by a trainee was used; and metrics were generated from the assessment with the potential to facilitate feedback and training. Participants had to have at least one video analysed for inclusion. In addition, qualitative studies exploring trainee and trainer experience of using automated operative video analytics were also eligible for inclusion. Articles were excluded when they involved a simulated or virtual reality setting or when they were reviews, case reports, editorials, commentaries, or conference abstracts. Studies were also excluded when they included video analysis based solely on expert assessment (for example OSATS), relied on non-video analysis of performance (for example kinematic data from robotic arms), or described a method of automated analysis that did not produce specific metrics that could be applied to training.
Data charting
All data from the included full texts were extracted independently by two reviewers (L.D. and C.P.B.) using a predefined data extraction template. Extraction data included, but were not limited to, demographics of participants, operation type, method of automated video analytics used, metrics generated, and application to training. Full extraction data are available in the Supplementary Methods.
Summarizing data
Data extracted from the included studies are described narratively, with quantitative analysis applied, where appropriate (for example total number of participants included in studies). Study characteristics extracted included first author, year of publication, primary country of research, and study type. Quality assessment of quantitative studies was performed independently by two reviewers (L.D. and C.P.B.) using the Medical Education Research Study Quality Instrument (MERSQI)30. This tool assesses study design, sampling, type of data, validity of evaluation instrument, data analysis, and outcomes, to provide an overall score, with a maximum of 18 (Table S1). Disagreements were settled by either consensus or discussion with a third reviewer (S.Y.). For studies with ‘not applicable’ MERSQI items, scores were based on maximum points available, adjusted to a standard denominator of 18.
Results
The search generated a total of 10 520 articles, of which 3788 were duplicates and were removed. Of the 6736 articles screened, 39 underwent full-text review. A total of 9 studies met the inclusion criteria, with a further 4 studies identified from searches of reference lists, giving a total of 13 studies (Fig. 1)31–43. Reasons for exclusion during full-text review included simulated setting (10 articles), no automated analysis (8 articles), no application to training (6 articles), and being conference abstracts (2 articles). All of the included studies were published within the last 10 years, with the majority (8 studies) published within the last 5 years.
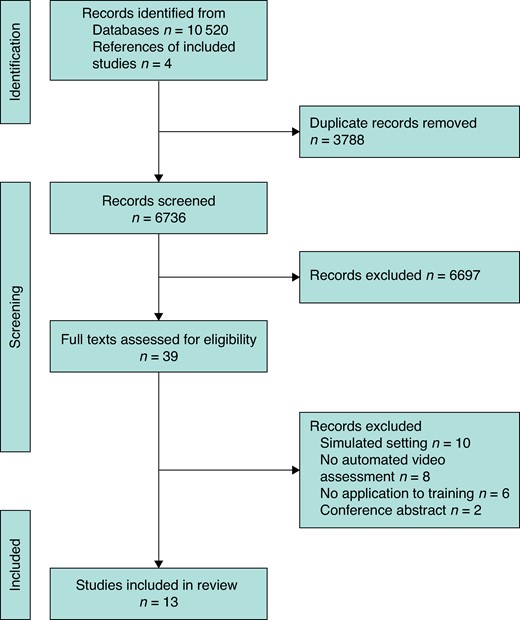
Quality assessment of the included studies
The mean adjusted MERSQI score for the included studies was 12.73 out of 18. All of the studies reported objective measures and went beyond descriptive analysis only, apart from one study, which was a feasibility study and thus not powered for statistical analysis35. Full MERSQI scoring is available in Table S2.
General study characteristics
The majority of studies were observational and one qualitative study was included. There was one multicentre study (17 centres included), with the number of institutions not reported in five studies. Cataract surgery and cholecystectomy were the two most common operations assessed and the majority of studies involved open surgery (6 studies). None of the included studies reported any patient or clinical outcomes (for example mortality). The median number of videos included for analysis was 69 (range 6–1107). Full study characteristics are available in Table 1.
Study . | Country . | Study type . | Specialty . | Operation . | Approach . | Number of videos in study . |
---|---|---|---|---|---|---|
Azari et al.31 | USA | PCS | Pan-specialty | Multiple | Open | 103 |
Balal et al.32 | UK | PCS | Ophthalmology | Cataract surgery | Open | 120 |
Din et al.33 | UK | PCS | Ophthalmology | Cataract surgery | Open | 22 |
Frasier et al.34 | USA | PCS | Pan-specialty | Multiple | Open | 138 |
Glarner et al.35 | USA | PCS | Plastic surgery | Reduction mammoplasty | Open | 6 |
Hameed et al.36 | Canada | Qualitative | Hepatobiliary | Cholecystectomy | Laparoscopic | Not applicable |
Humm et al.37 | UK | RCS | Hepatobiliary | Cholecystectomy | Laparoscopic | 159 |
Kitaguchi et al.38 | Japan | RCS | Colorectal | Transanal total mesorectal excision | Endoscopic | 45 |
Lee et al.39 | South Korea | RCS | Endocrinology | Thyroidectomy | Robotic | 40 |
Smith et al.40 | UK | PCS | Ophthalmology | Cataract surgery | Open | 20 |
Wawrzynski et al.41 | UK | PCS | Otolaryngology | Dacryocystorhinostomy | Endoscopic | 20 |
Wu et al.42 | China | PCS | Hepatobiliary | Cholecystectomy | Laparoscopic | 1107 |
Yang et al.43 | USA | PCS | Colorectal | Rectopexy | Robotic | 92 |
Study . | Country . | Study type . | Specialty . | Operation . | Approach . | Number of videos in study . |
---|---|---|---|---|---|---|
Azari et al.31 | USA | PCS | Pan-specialty | Multiple | Open | 103 |
Balal et al.32 | UK | PCS | Ophthalmology | Cataract surgery | Open | 120 |
Din et al.33 | UK | PCS | Ophthalmology | Cataract surgery | Open | 22 |
Frasier et al.34 | USA | PCS | Pan-specialty | Multiple | Open | 138 |
Glarner et al.35 | USA | PCS | Plastic surgery | Reduction mammoplasty | Open | 6 |
Hameed et al.36 | Canada | Qualitative | Hepatobiliary | Cholecystectomy | Laparoscopic | Not applicable |
Humm et al.37 | UK | RCS | Hepatobiliary | Cholecystectomy | Laparoscopic | 159 |
Kitaguchi et al.38 | Japan | RCS | Colorectal | Transanal total mesorectal excision | Endoscopic | 45 |
Lee et al.39 | South Korea | RCS | Endocrinology | Thyroidectomy | Robotic | 40 |
Smith et al.40 | UK | PCS | Ophthalmology | Cataract surgery | Open | 20 |
Wawrzynski et al.41 | UK | PCS | Otolaryngology | Dacryocystorhinostomy | Endoscopic | 20 |
Wu et al.42 | China | PCS | Hepatobiliary | Cholecystectomy | Laparoscopic | 1107 |
Yang et al.43 | USA | PCS | Colorectal | Rectopexy | Robotic | 92 |
PCS, prospective cohort study; RCS, retrospective cohort study.
Study . | Country . | Study type . | Specialty . | Operation . | Approach . | Number of videos in study . |
---|---|---|---|---|---|---|
Azari et al.31 | USA | PCS | Pan-specialty | Multiple | Open | 103 |
Balal et al.32 | UK | PCS | Ophthalmology | Cataract surgery | Open | 120 |
Din et al.33 | UK | PCS | Ophthalmology | Cataract surgery | Open | 22 |
Frasier et al.34 | USA | PCS | Pan-specialty | Multiple | Open | 138 |
Glarner et al.35 | USA | PCS | Plastic surgery | Reduction mammoplasty | Open | 6 |
Hameed et al.36 | Canada | Qualitative | Hepatobiliary | Cholecystectomy | Laparoscopic | Not applicable |
Humm et al.37 | UK | RCS | Hepatobiliary | Cholecystectomy | Laparoscopic | 159 |
Kitaguchi et al.38 | Japan | RCS | Colorectal | Transanal total mesorectal excision | Endoscopic | 45 |
Lee et al.39 | South Korea | RCS | Endocrinology | Thyroidectomy | Robotic | 40 |
Smith et al.40 | UK | PCS | Ophthalmology | Cataract surgery | Open | 20 |
Wawrzynski et al.41 | UK | PCS | Otolaryngology | Dacryocystorhinostomy | Endoscopic | 20 |
Wu et al.42 | China | PCS | Hepatobiliary | Cholecystectomy | Laparoscopic | 1107 |
Yang et al.43 | USA | PCS | Colorectal | Rectopexy | Robotic | 92 |
Study . | Country . | Study type . | Specialty . | Operation . | Approach . | Number of videos in study . |
---|---|---|---|---|---|---|
Azari et al.31 | USA | PCS | Pan-specialty | Multiple | Open | 103 |
Balal et al.32 | UK | PCS | Ophthalmology | Cataract surgery | Open | 120 |
Din et al.33 | UK | PCS | Ophthalmology | Cataract surgery | Open | 22 |
Frasier et al.34 | USA | PCS | Pan-specialty | Multiple | Open | 138 |
Glarner et al.35 | USA | PCS | Plastic surgery | Reduction mammoplasty | Open | 6 |
Hameed et al.36 | Canada | Qualitative | Hepatobiliary | Cholecystectomy | Laparoscopic | Not applicable |
Humm et al.37 | UK | RCS | Hepatobiliary | Cholecystectomy | Laparoscopic | 159 |
Kitaguchi et al.38 | Japan | RCS | Colorectal | Transanal total mesorectal excision | Endoscopic | 45 |
Lee et al.39 | South Korea | RCS | Endocrinology | Thyroidectomy | Robotic | 40 |
Smith et al.40 | UK | PCS | Ophthalmology | Cataract surgery | Open | 20 |
Wawrzynski et al.41 | UK | PCS | Otolaryngology | Dacryocystorhinostomy | Endoscopic | 20 |
Wu et al.42 | China | PCS | Hepatobiliary | Cholecystectomy | Laparoscopic | 1107 |
Yang et al.43 | USA | PCS | Colorectal | Rectopexy | Robotic | 92 |
PCS, prospective cohort study; RCS, retrospective cohort study.
Participant details
There were a total of 107 trainees in the nine studies that reported on numbers of participants (mean(s.d.) 12(9.2)). The majority of studies (8 studies) did not report the grade of training. A total of five studies reported the baseline skills of trainees, using the total prior number of operations performed as the sole marker of skill. Of the studies, one used baseline skills to differentiate between novices, intermediates, and experts.
All of the included studies had a comparator group, with a total of 152 attendings or experts in the ten studies reporting these numbers (mean(s.d.) 15(17.5)). In contrast to trainees, the baseline skills of attendings and experts were more frequently reported (7 studies), using the prior number of operations performed as the marker of skill. Full participant characteristics are available in Table 2.
Study . | Number of trainees . | Level of training . | Baseline reported* . | Number of experts for comparison . | Baseline reported . |
---|---|---|---|---|---|
Azari et al.31 | 3 | Resident (unspecified) | Unknown | 6 | Unknown |
Balal et al.32 | 20 | Resident (unspecified) | <200 operations performed | 20 | >1000 operations performed |
Din et al.33 | 31 | Resident (unspecified) | <200 operations performed | 31 | >1000 operations performed |
Frasier et al.34 | 3 | PGY3–PGY5 | Not reported | 6 | Unknown |
Glarner et al.35 | 3 | PGY3–PGY5 | Unknown | 3 | 8–30 years of experience |
Hameed et al.36 | 13 | Fellow, Senior resident, Junior resident | Unknown | 7 | Mean of 57 laparoscopic cholecystectomies per year |
Humm et al.37 | Unknown | Resident (unspecified) | Unknown | 1 | Unknown |
Kitaguchi et al.38 | Unknown | Unknown | Novice <10 operations, intermediate 10–30 operations | Unknown | >30 operations performed |
Lee et al.39 | Unknown | Fellow | Unknown | Unknown | Unknown |
Smith et al.40 | 10 | Resident (unspecified) | <200 operations performed | 10 | >1000 operations performed |
Wawrzynski et al.41 | 10 | Resident (unspecified) | <20 operations performed | 10 | >100 operations performed |
Wu et al.42 | 14 | Resident (unspecified) | Unknown | 58 | Unknown |
Yang et al.43 | Unknown | PGY3–PGY7 | Unknown | Unknown | Unknown |
Study . | Number of trainees . | Level of training . | Baseline reported* . | Number of experts for comparison . | Baseline reported . |
---|---|---|---|---|---|
Azari et al.31 | 3 | Resident (unspecified) | Unknown | 6 | Unknown |
Balal et al.32 | 20 | Resident (unspecified) | <200 operations performed | 20 | >1000 operations performed |
Din et al.33 | 31 | Resident (unspecified) | <200 operations performed | 31 | >1000 operations performed |
Frasier et al.34 | 3 | PGY3–PGY5 | Not reported | 6 | Unknown |
Glarner et al.35 | 3 | PGY3–PGY5 | Unknown | 3 | 8–30 years of experience |
Hameed et al.36 | 13 | Fellow, Senior resident, Junior resident | Unknown | 7 | Mean of 57 laparoscopic cholecystectomies per year |
Humm et al.37 | Unknown | Resident (unspecified) | Unknown | 1 | Unknown |
Kitaguchi et al.38 | Unknown | Unknown | Novice <10 operations, intermediate 10–30 operations | Unknown | >30 operations performed |
Lee et al.39 | Unknown | Fellow | Unknown | Unknown | Unknown |
Smith et al.40 | 10 | Resident (unspecified) | <200 operations performed | 10 | >1000 operations performed |
Wawrzynski et al.41 | 10 | Resident (unspecified) | <20 operations performed | 10 | >100 operations performed |
Wu et al.42 | 14 | Resident (unspecified) | Unknown | 58 | Unknown |
Yang et al.43 | Unknown | PGY3–PGY7 | Unknown | Unknown | Unknown |
PGY, postgraduate year. *Definition used in each study to quantify experience of participants. In all studies, except one, reporting baseline, the number of operations previously performed was used.
Study . | Number of trainees . | Level of training . | Baseline reported* . | Number of experts for comparison . | Baseline reported . |
---|---|---|---|---|---|
Azari et al.31 | 3 | Resident (unspecified) | Unknown | 6 | Unknown |
Balal et al.32 | 20 | Resident (unspecified) | <200 operations performed | 20 | >1000 operations performed |
Din et al.33 | 31 | Resident (unspecified) | <200 operations performed | 31 | >1000 operations performed |
Frasier et al.34 | 3 | PGY3–PGY5 | Not reported | 6 | Unknown |
Glarner et al.35 | 3 | PGY3–PGY5 | Unknown | 3 | 8–30 years of experience |
Hameed et al.36 | 13 | Fellow, Senior resident, Junior resident | Unknown | 7 | Mean of 57 laparoscopic cholecystectomies per year |
Humm et al.37 | Unknown | Resident (unspecified) | Unknown | 1 | Unknown |
Kitaguchi et al.38 | Unknown | Unknown | Novice <10 operations, intermediate 10–30 operations | Unknown | >30 operations performed |
Lee et al.39 | Unknown | Fellow | Unknown | Unknown | Unknown |
Smith et al.40 | 10 | Resident (unspecified) | <200 operations performed | 10 | >1000 operations performed |
Wawrzynski et al.41 | 10 | Resident (unspecified) | <20 operations performed | 10 | >100 operations performed |
Wu et al.42 | 14 | Resident (unspecified) | Unknown | 58 | Unknown |
Yang et al.43 | Unknown | PGY3–PGY7 | Unknown | Unknown | Unknown |
Study . | Number of trainees . | Level of training . | Baseline reported* . | Number of experts for comparison . | Baseline reported . |
---|---|---|---|---|---|
Azari et al.31 | 3 | Resident (unspecified) | Unknown | 6 | Unknown |
Balal et al.32 | 20 | Resident (unspecified) | <200 operations performed | 20 | >1000 operations performed |
Din et al.33 | 31 | Resident (unspecified) | <200 operations performed | 31 | >1000 operations performed |
Frasier et al.34 | 3 | PGY3–PGY5 | Not reported | 6 | Unknown |
Glarner et al.35 | 3 | PGY3–PGY5 | Unknown | 3 | 8–30 years of experience |
Hameed et al.36 | 13 | Fellow, Senior resident, Junior resident | Unknown | 7 | Mean of 57 laparoscopic cholecystectomies per year |
Humm et al.37 | Unknown | Resident (unspecified) | Unknown | 1 | Unknown |
Kitaguchi et al.38 | Unknown | Unknown | Novice <10 operations, intermediate 10–30 operations | Unknown | >30 operations performed |
Lee et al.39 | Unknown | Fellow | Unknown | Unknown | Unknown |
Smith et al.40 | 10 | Resident (unspecified) | <200 operations performed | 10 | >1000 operations performed |
Wawrzynski et al.41 | 10 | Resident (unspecified) | <20 operations performed | 10 | >100 operations performed |
Wu et al.42 | 14 | Resident (unspecified) | Unknown | 58 | Unknown |
Yang et al.43 | Unknown | PGY3–PGY7 | Unknown | Unknown | Unknown |
PGY, postgraduate year. *Definition used in each study to quantify experience of participants. In all studies, except one, reporting baseline, the number of operations previously performed was used.
Method of automated video analysis
The most common method of automated video analysis was computer vision tracking (7 studies). This was used in all of the studies that assessed open surgery and one of the studies that assessed endoscopic procedures; three of these studies required manual identification of the region of interest (ROI) before computer vision tracking could be used for the completion of the assessment automatically31,34,35. More advanced AI-based approaches to video analysis were used in the remaining studies, with convolutional neural networking (CNN) being the most common method (4 studies) (Table 3). CNN relies on recognizing patterns to process images. Pyramid scene parsing networking (PSPN), based on image segmentation, was also used to generate heat maps in laparoscopic cholecystectomy36.
Study . | Method of analysis . | Metric(s) produced . | Application to training . |
---|---|---|---|
Azari et al.31 | Computer vision tracking | Fluidity of motion Motion economy Tissue handling | Metric correlated with alternative assessment tool |
Balal et al.32 | Computer vision tracking | Intraoperative phase duration Instrument distance travelled Instrument use | To differentiate between different skill sets |
Din et al.33 | Computer vision tracking | Instrument distance travelled Instrument use | Metric correlated with alternative assessment tool + To differentiate between different skill sets |
Frasier et al.34 | Computer vision tracking | Speed and acceleration of movement | To differentiate between different skill sets |
Glarner et al.35 | Computer vision tracking | Instrument use Bimanual dexterity Displacement, velocity, and acceleration | To differentiate between different skill sets |
Hameed et al.36 | PSPN | Heat maps | Qualitative study |
Humm et al.37 | CNN | Total operative duration Intraoperative phase duration | To differentiate between different skill sets |
Kitaguchi et al.38 | CNN | Generated suturing score | To differentiate between different skill sets |
Lee et al.39 | CNN | Classification of skill (novice, skilled, and expert) | Metric correlated with alternative assessment tool + To differentiate between different skill sets |
Smith et al.40 | Computer vision tracking | Total operative duration Instrument distance travelled Instrument use | To differentiate between different skill sets |
Wawrzynski et al.41 | Computer vision tracking | Total operative duration Instrument distance travelled Instrument use | To differentiate between different skill sets |
Wu et al.42 | TSM | CVS achievement | Metric correlated with years of experience |
Yang et al.43 | CNN | Instrument distance travelled Bimanual dexterity Depth perception | Metric correlated with alternative assessment tool + To differentiate between different skill sets |
Study . | Method of analysis . | Metric(s) produced . | Application to training . |
---|---|---|---|
Azari et al.31 | Computer vision tracking | Fluidity of motion Motion economy Tissue handling | Metric correlated with alternative assessment tool |
Balal et al.32 | Computer vision tracking | Intraoperative phase duration Instrument distance travelled Instrument use | To differentiate between different skill sets |
Din et al.33 | Computer vision tracking | Instrument distance travelled Instrument use | Metric correlated with alternative assessment tool + To differentiate between different skill sets |
Frasier et al.34 | Computer vision tracking | Speed and acceleration of movement | To differentiate between different skill sets |
Glarner et al.35 | Computer vision tracking | Instrument use Bimanual dexterity Displacement, velocity, and acceleration | To differentiate between different skill sets |
Hameed et al.36 | PSPN | Heat maps | Qualitative study |
Humm et al.37 | CNN | Total operative duration Intraoperative phase duration | To differentiate between different skill sets |
Kitaguchi et al.38 | CNN | Generated suturing score | To differentiate between different skill sets |
Lee et al.39 | CNN | Classification of skill (novice, skilled, and expert) | Metric correlated with alternative assessment tool + To differentiate between different skill sets |
Smith et al.40 | Computer vision tracking | Total operative duration Instrument distance travelled Instrument use | To differentiate between different skill sets |
Wawrzynski et al.41 | Computer vision tracking | Total operative duration Instrument distance travelled Instrument use | To differentiate between different skill sets |
Wu et al.42 | TSM | CVS achievement | Metric correlated with years of experience |
Yang et al.43 | CNN | Instrument distance travelled Bimanual dexterity Depth perception | Metric correlated with alternative assessment tool + To differentiate between different skill sets |
PSPN, pyramid scene parsing networking; CNN, convolutional neural networking; TSM, temporal shifting module; CVS, critical view of safety.
Study . | Method of analysis . | Metric(s) produced . | Application to training . |
---|---|---|---|
Azari et al.31 | Computer vision tracking | Fluidity of motion Motion economy Tissue handling | Metric correlated with alternative assessment tool |
Balal et al.32 | Computer vision tracking | Intraoperative phase duration Instrument distance travelled Instrument use | To differentiate between different skill sets |
Din et al.33 | Computer vision tracking | Instrument distance travelled Instrument use | Metric correlated with alternative assessment tool + To differentiate between different skill sets |
Frasier et al.34 | Computer vision tracking | Speed and acceleration of movement | To differentiate between different skill sets |
Glarner et al.35 | Computer vision tracking | Instrument use Bimanual dexterity Displacement, velocity, and acceleration | To differentiate between different skill sets |
Hameed et al.36 | PSPN | Heat maps | Qualitative study |
Humm et al.37 | CNN | Total operative duration Intraoperative phase duration | To differentiate between different skill sets |
Kitaguchi et al.38 | CNN | Generated suturing score | To differentiate between different skill sets |
Lee et al.39 | CNN | Classification of skill (novice, skilled, and expert) | Metric correlated with alternative assessment tool + To differentiate between different skill sets |
Smith et al.40 | Computer vision tracking | Total operative duration Instrument distance travelled Instrument use | To differentiate between different skill sets |
Wawrzynski et al.41 | Computer vision tracking | Total operative duration Instrument distance travelled Instrument use | To differentiate between different skill sets |
Wu et al.42 | TSM | CVS achievement | Metric correlated with years of experience |
Yang et al.43 | CNN | Instrument distance travelled Bimanual dexterity Depth perception | Metric correlated with alternative assessment tool + To differentiate between different skill sets |
Study . | Method of analysis . | Metric(s) produced . | Application to training . |
---|---|---|---|
Azari et al.31 | Computer vision tracking | Fluidity of motion Motion economy Tissue handling | Metric correlated with alternative assessment tool |
Balal et al.32 | Computer vision tracking | Intraoperative phase duration Instrument distance travelled Instrument use | To differentiate between different skill sets |
Din et al.33 | Computer vision tracking | Instrument distance travelled Instrument use | Metric correlated with alternative assessment tool + To differentiate between different skill sets |
Frasier et al.34 | Computer vision tracking | Speed and acceleration of movement | To differentiate between different skill sets |
Glarner et al.35 | Computer vision tracking | Instrument use Bimanual dexterity Displacement, velocity, and acceleration | To differentiate between different skill sets |
Hameed et al.36 | PSPN | Heat maps | Qualitative study |
Humm et al.37 | CNN | Total operative duration Intraoperative phase duration | To differentiate between different skill sets |
Kitaguchi et al.38 | CNN | Generated suturing score | To differentiate between different skill sets |
Lee et al.39 | CNN | Classification of skill (novice, skilled, and expert) | Metric correlated with alternative assessment tool + To differentiate between different skill sets |
Smith et al.40 | Computer vision tracking | Total operative duration Instrument distance travelled Instrument use | To differentiate between different skill sets |
Wawrzynski et al.41 | Computer vision tracking | Total operative duration Instrument distance travelled Instrument use | To differentiate between different skill sets |
Wu et al.42 | TSM | CVS achievement | Metric correlated with years of experience |
Yang et al.43 | CNN | Instrument distance travelled Bimanual dexterity Depth perception | Metric correlated with alternative assessment tool + To differentiate between different skill sets |
PSPN, pyramid scene parsing networking; CNN, convolutional neural networking; TSM, temporal shifting module; CVS, critical view of safety.
Metrics produced
Metrics generated varied between studies and can be classified as either process or outcome based. Process-based metrics uniformly analysed motion and, in all but one study, were generated using computer vision tracking. Distance travelled and total movement of hands/instruments were the most common motions measured, although bimanual dexterity, speed, acceleration, and fluidity of movements were also reported. These metrics were generated for entire procedures, specific intraoperative phases, or individual tasks.
Outcome-based metrics were more diverse, largely generated from advanced AI-based analysis, and assessed either during specific phases of the operation or for individual tasks. Intraoperative phase durations were assessed in laparoscopic cholecystectomy and cataract surgery, whilst performance scores were generated for the quality of suturing in transanal total mesorectal excision (TaTME). Of the studies, two generated metrics that aimed to assess critical safety elements in laparoscopic cholecystectomy; these generated scores, to determine critical view of safety achievement, and heat maps, to demonstrate areas of safe and unsafe dissection. The full list of metrics produced is available in Table 3.
Application to training
The most common application to training was the use of metrics to discriminate between different skill levels (10 studies) for distinguishing trainees and attendings/experts. Of the studies, one compared different groups of trainees (novice versus intermediate). All studies were able to distinguish between groups with high levels of accuracy. In addition, five studies compared the metrics produced with an alternative assessment tool. These were based on expert assessment and included OSATS, Global Evaluative Assessment of Robotic Skills (GEARS), Objective Structured Assessment of Cataract Surgical Skill (OSACSS), and a performance rubric created by the study authors that had previously been validated using GEARS. All studies reported that the metrics produced from analysis correlated with the validated tool used. The number of human assessors ranged from one to six and the assessors were defined as being expert (2 studies) or board-certified surgeons (2 studies) (or no information was given (1 study)) and, of the studies relying on board-certified surgeons, one reported that the surgeons were experienced in the use of the AI model created to perform the video analysis. No study produced metrics for the assessment of non-technical skills or reported on how the metrics produced could be applied to assess non-technical skills. Furthermore, none of the included studies explored the impact on training outcomes (for example learning curves).
A qualitative study aimed to assess the educational value of using AI-generated heat maps to display areas of safe and unsafe dissection in laparoscopic cholecystectomy. Trainees and trainers strongly agreed (73%) that their use after surgery for coaching and feedback could be effective. Most suggested that heat maps should be incorporated in the surgical training curriculum, particularly for those at an earlier stage in training. However, 40% reported that, even if readily available, they would not use it routinely. Some raised concerns over potential dependence on it for making intraoperative decisions. Full results of the included studies are available in Table S3.
Discussion
This scoping review explores the current methods of automated analysis of intraoperative video in the context of surgical training. This is a rapidly evolving field, with most of the included studies being published within the last 5 years.
Several methods of automating the assessment of surgical video were identified. Computer vision, the broad term used to describe an algorithm’s ability to process and understand visual data20, was used to generate kinematic data. Early techniques utilized tracking of objects between individual frames extracted from video31,34,35 and others relied on the principle of point feature tracking32,33,40,41. The included studies were effective at tracking movements of the surgeon’s hand during suturing and knot tying31 and tracking surgical instruments during cataract surgery40. The need for manual identification of the ROI before automated tracking was a limitation in several studies31,34,35. Human-dependent steps in these studies presented potential barriers to the scalability of the methods used. Minimizing inputs required from trainees, and trainers, to collect and analyse operative video not only improves engagement but also likely reduces the impact on cognitive load during the operation.
Newer approaches, utilizing AI, aim to overcome some of these barriers. CNN was able to automatically detect and track surgical instruments to assess motion38,39,43. Surgical tool recognition is fundamental to this approach, with several studies reporting high accuracy with regard to identifying the presence or absence of instruments, as well as differentiating between instrument types44,45. Furthermore, tool usage determined using AI correlates strongly with that determined by human assessment46. Limitations of AI-based video analysis include the need for accurately annotated video for training AI models. However, the introduction of open data sets has diminished this obstacle47.
It is not surprising that the most commonly produced metrics reflect kinematic data. Traditionally collected using sensors applied to the surgeon or instrument, kinematic data have long been used as markers of technical proficiency, due to strong correlation with expert-based assessment scores48,49, even when deployed during complex operations50. Insights into operative performance can be gained by analysing motion throughout an entire operation (for example total distance travelled) or within an individual task. Of the five studies that assessed individual tasks, four were based on motion analysis and included critical steps, such as suturing of bowel anastomoses and TaTME. Importantly, this granular technique is now being automatically assessed in both laparoscopic and robotic simulated settings51,52.
Operative phase recognition is an emerging field of AI workflow analysis53,54. The concept of surgical workflow analysis is based on the premise that an operation is rarely a singular event but rather a series of complex sequences that build upon one another. Variations in these sequences are more common for less experienced surgeons55 and, thus, being able to efficiently analyse phases has great potential for improving post-hoc feedback. Several applications of phase analysis, such as duration of hepatocystic triangle dissection in laparoscopic cholecystectomy37, tool usage in phacoemulsification during cataract surgery32, and skill determination during the first phase of robot-assisted thyroidectomy39, were identified in the present review. Analysis of surgical gestures, defined as granular interactions between surgical instruments and human tissue56, is another emerging field. Preliminary studies have identified that gesture type (for example to indicate ‘dissect’ or ‘coagulate’) and gesture efficiency (for example use of ineffective or erroneous gestures) can distinguish experts from novices57–59. Most studies in this field are in the simulated setting; therefore, the potential application of surgical gestures to improve trainee surgical performance in the operating room remains unclear.
The majority of the included studies used the metrics produced from their analysis to differentiate between skill sets, a finding not consistent in the broader literature60. A limitation of using this method in the included studies was that very few reported the level of training or the baseline skills of the participants. For example, the term ‘trainee’ is ambiguous and is equally applicable to someone in their first year of surgical training as to someone in their last. It may also be applied to fully certified surgeons learning a new procedure or approach (for example robotics).
Automatically generated metrics were also compared with validated, expert-based tools. Most studies compared metrics of similar properties (for example tool usage with bimanual dexterity); however, other extractable metrics from video, such as clearness of the operating field from blood, have also been shown to correlate with global expert-based assessments61. Although there is growing evidence to support the accuracy of automated assessments compared with expert-based methods48,62, video-based assessments can only provide metrics from the material provided. In certain scenarios, wider contextual factors need consideration to guide accurate training and assessment. This has led some to advocate a hybrid approach, incorporating expert assessment with objective data generated from AI63.
Currently evidence to explore the experiences of trainees and trainers using metrics generated from video analysis is lacking. Hameed et al.36 report mixed opinions from trainees and trainers on an AI-based assessment of safe dissection planes in laparoscopic cholecystectomy. Most agreed that there was potential for use in surgical training; however, up to 40% said that, even if readily available, they would not routinely use it. Reservations are also shared by patients, with a recent survey reporting that, although most recognized the educational benefit of having their procedure recorded for training64, concerns remained over non-maleficence, regarding data storage and access65. Robust protocols are needed to satisfy these legitimate concerns and frameworks should be established to manage potential data breaches.
The present scoping review identifies the methods and metrics generated from automated video analysis of operative surgery. Establishing robust validity of automated video analytics is essential if this innovation is to help shape future training. It is also crucial that consensus be reached on which metrics of operative performance are most relevant for surgical trainees. No study reported longitudinal use of metrics by trainees to aid in their skill development. Training is a continuous process, requiring regular review of performance and goal setting. Understanding the longer-term impact of new technologies on training is a priority, so they can be applied in the most effective way. A second research priority is to address the lack of automated assessment of non-technical skills in surgery. Exploration of this in simulated and non-simulated settings is in progress27,66.
The present review has some limitations. There were no restrictions with regard to surgical procedure or approach in the inclusion criteria, which likely increased heterogeneity in the findings. The metrics relevant for one procedure or skill are likely different from those required for another. It was surprising that MIS was not the most common surgical approach, given its video-based nature. Inclusion of only real-world operations may have resulted in under-representation of MIS, as research in MIS is still largely confined to the simulated setting, particularly in robotics.
The current landscape of automated video analytics in surgical training shows promise, both in terms of the metrics produced and the video analytics used. Additionally, there is a paucity of evidence with regard to understanding both the end user experience and the longitudinal benefit of automated video analysis for surgical trainees. To embrace this new technology, educators, curriculum developers, and professional bodies must support validation studies in training environments, before widespread adoption, for the benefit of trainees, trainers, and patients.
Funding
L.D. is supported by a Medical Education Fellowship funded by NHS Lothian. R.J.E.S. is funded by NHS Research Scotland (NRS) via a clinician post.
Acknowledgements
The authors would like to thank Marshall Dozier for assistance in generating the search criteria.
Author contributions
Lachlan Dick (Conceptualization, Data curation, Formal analysis, Methodology, Resources, Software, Visualization, Writing—original draft, Writing—review & editing), Connor P. Boyle (Data curation, Writing—review & editing), Richard J. E. Skipworth (Conceptualization, Supervision, Writing—review & editing), Douglas M. Smink (Writing—review & editing), Victoria R. Tallentire (Conceptualization, Supervision, Writing—review & editing), and Steven Yule (Conceptualization, Supervision, Writing—review & editing)
Disclosure
The authors declare no conflict of interest.
Supplementary material
Supplementary material is available at BJS Open online.
Data availability
Some data may be available upon reasonable request.
References
Author notes
Presented as a talking poster at the Association of Surgeons of Great Britain and Ireland Congress, Belfast, UK, 2024.