-
PDF
- Split View
-
Views
-
Cite
Cite
Xiao Song, Kevin K Dobbin, Evaluating biomarkers for treatment selection from reproducibility studies, Biostatistics, Volume 23, Issue 1, January 2022, Pages 173–188, https://doi.org/10.1093/biostatistics/kxaa018
- Share Icon Share
Summary
We consider evaluating new or more accurately measured predictive biomarkers for treatment selection based on a previous clinical trial involving standard biomarkers. Instead of rerunning the clinical trial with the new biomarkers, we propose a more efficient approach which requires only either conducting a reproducibility study in which the new biomarkers and standard biomarkers are both measured on a set of patient samples, or adopting replicated measures of the error-contaminated standard biomarkers in the original study. This approach is easier to conduct and much less expensive than studies that require new samples from patients randomized to the intervention. In addition, it makes it possible to perform the estimation of the clinical performance quickly, since there will be no requirement to wait for events to occur as would be the case with prospective validation. The treatment selection is assessed via a working model, but the proposed estimator of the mean restricted lifetime is valid even if the working model is misspecified. The proposed approach is assessed through simulation studies and applied to a cancer study.
1. Introduction
A biomarker used to predict the response to a treatment is called a predictive biomarker. For example, patients with colon cancer can be treated by surgery alone or surgery plus chemotherapy. Surgery alone is less expensive and has fewer side effects than surgery plus chemotherapy, but it may be less effective as well, at least for some patients. For an individual patient, it is desirable to identify whether or not the patient will benefit more from the extra chemotherapy based on a biomarker or a set of biomarkers. A possible useful biomarker in this context is the c-myc gene, which is over-expressed in approximately 70 percent of human colonic tumors. Based on a study conducted by the Eastern Cooperative Oncology Group (ECOG), Augenlicht and others (1997) suggested that the c-myc gene may be of clinically prognostic importance in patients with colon cancer. Using a subset of the cases from this clinical trial, Li and Ryan (2006) found that there is an interaction between the c-myc gene expression levels and the two treatments for the response of disease progression-free survival; Song and Zhou (2011) investigated using the observed c-myc gene expression level for treatment selection.
To evaluate the potential of biomarkers for treatment selection, approaches have been proposed that try to minimize the population event rate under (optimal) treatment selection criteria (Song and Pepe, 2004; Brinkley and others, 2010; Cai and others, 2011,Zhang and others, 2012,Janes and others, 2011, 2014) or maximize the population mean (restricted lifetime) (Song and Zhou, 2011).
However, biomarker measurements may contain measurement error, or the current biomarkers may not be very effective for treatment selection. In the ECOG study, the c-myc gene expression levels were measured with error (Li and Ryan, 2006). It is of interest to evaluate the amount of gain that could be achieved with respect to treatment selection if the biomarkers were accurately measured. In another aspect, a new technology may improve the measurement of biomarkers or a new biomarker may be identified with better predictability capacity. For example, with the development of polymerase chain reaction technique, the measurement of c-myc gene expression level may become more accurate. If we call the measurement of c-myc gene level in the original study the standard biomarker, and the improved measurement using advanced techniques the new biomarker, then we would like to assess the capacity of the new biomarker for making treatment selections. With a slight abuse of terminology, we refer both newly identified biomarkers and more accurately measured biomarkers as new biomarkers. Ideally, one would like to re-run the previous study, or perform a new study very similar to it, so that one could measure the new biomarkers using prospectively collected samples. But this is often not feasible. Such a procedure would entail large additional costs associated with obtaining samples of tumor tissues with measurement of disease progression-free survival through a multi-year randomized clinical trial. Moreover, it may not even be feasible to perform such a study. We propose a more efficient approach which requires only conducting a reproducibility study where the new biomarkers and the standard biomarkers will be measured on a set of patient samples or replicated measurements of the inaccurately measured standard biomarkers. Importantly, there is no need to re-run the clinical trial. This makes the study easier to conduct and much less expensive. In addition, it will make it possible to perform the estimation of the clinical performance of the new biomarkers quickly, since there will be no requirement to wait for events to occur. The idea of our approach is summarized in Figure 1. The outcome and the standard biomarkers are observed in the clinical trial, while the new biomarkers and the standard biomarkers are observed in the reproducibility study. We make inference on the new biomarkers versus the outcome to assess the capacity of the new biomarkers on treatment selection. There are related studies in the literature (Boostra and others, 2013a, 2013b) aiming to predict outcome with new biomarkers observed on a subset in the original study, but their objectives are different from what is considered in this article.
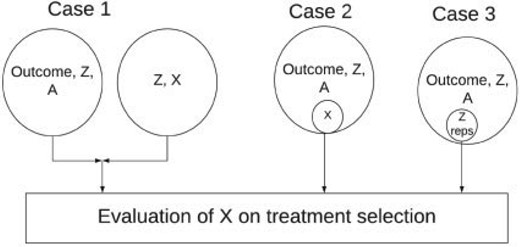
Overview of the three cases, where |$Z$| denotes the standard biomarkers, |$X$| denotes the new biomarkers, and |$A$| denotes the treatment.
In this article, we consider the setting in which the outcome is time to an event of interest (survival time), which may be subject to right censoring. To characterize the inter-relations between the biomarkers and the treatment arms, we adopt a working proportional hazards model with interactions between the treatment and the biomarkers. The relation between the standard markers and the new markers is modeled through a classical measurement error model. Various approaches have been proposed to estimate the regression coefficients in the presence of covariate measurement error under the proportional hazards model, however, most were derived under the assumption of linear covariate effects. These include regression calibration (Prentice, 1982; Dafni and Tsiatis, 1998; Wang and others, 2000), SIMEX (Greene and Cai, 2004), likelihood based approaches (Wulfsohn and Tsiatis, 1997; Faucett and Thomas, 1996; Henderson and others, 2000; Xu and Zeger, 2001; Song and others, 2002b), conditional score (Tsiatis and Davidian, 2001; Song and others, 2002a), and correction approaches (Huang and Wang, 2000), among others. Here, we extend the conditional score approach to the proportional hazards model with interactions. In addition, we propose to use the mean restricted lifetime to evaluate the performance of the predictive biomarkers and derive the optimal treatment selection strategy under the working model. To estimate the mean restricted lifetime, we propose a SIMEX estimator and establish the asymptotic properties using the empirical process and stochastic integral techniques.
The novelty of this article includes the following aspects. First, our idea of evaluating new biomarkers without re-running the clinical trial is novel, which could greatly reduce the study time and cost. Second, the adoption of the measurement error model and techniques under this circumstance is novel. Third, we propose well-justified resampling-based inference which extends the technique of Peng and Huang (2008). To the best of our knowledge, the overlay of the resampling-based inference with the already resampling-based SIMEX approach is new.
The article is organized as follows. In Section 1, we give the model definition. We derive an empirical estimator for the optimal treatment selection and propose an approach to evaluate and compare the new biomarkers and standard biomarkers on treatment selection in Section 2. We investigate the finite sample performance of the proposed approach in Section 3, and we apply the approach to the ECOG data in Section 4. Some discussions are given in Section 5. The regularity conditions and sketched proofs are given in the supplementary material available at Biostatistics online.
2. Model definition
Let |$T$| denote the survival time, and |$C$| denote the censoring time. The observed survival data are |$V=\min (T,C)$| and |$\Delta =I(T\leq C)$| , where |$ I(\cdot )$| is the indicator function. Let |$Z$| denote a vector of |$K$| continuous standard biomarkers, and |$X$| denote a vector of |$K$| continuous new biomarkers. Remember that, with a slight abuse of terminology, we refer both newly identified biomarkers and more accurately measured biomarkers as new biomarkers. Let |$A$| denote the treatment, where |$A=0$| denotes the control or standard treatment, and |$A=1$| denotes the new treatment. Suppose a randomized clinical trial has been conducted to evaluate the standard biomarkers for treatment selection with the observed data |$\{(V_{i},\Delta _{i},A_{i},Z_{i}):$||$i=1,\ldots ,n\}. $| We are interested in evaluating the treatment selection capacity of the new biomarkers |$X$|.
To ensure the identifiability of model (2.2), we need to have either validation data or replicated data on |$Z$|. We consider three cases. In case 1, a validation data set is available from an external reproducibility study. The observations in the reproducibility study are |$ \{(X_{i}^{\#},Z_{i}^{\#}):i=1,\ldots ,m\}$|. In case 2, an internal validation data set of a size |$m$| is available in the original data set. Although we may directly evaluate the new marker using the validation set in this case, it would be more efficient to use the whole data set. In case 3, replicated error-contaminated observations are available on some subjects in the original study. Case 3 is only feasible when the new biomarkers are obtained by improving the accuracy of measurement while cases 1 and 2 also cover the situation when the new biomarkers are truly different variables. To unify the notations in the three cases, the observed data in the original study is denoted by |$\{(V_{i},\Delta _{i},A_{i},Z_{ij},R_{i}X_{i}):$||$i=1,\ldots ,n,j=1,\ldots ,J_{i}\},$| where |$ J_{i}$| denotes the number of replicates for subject |$i,$| which always equals one in cases 1 and 2; |$R_{i}=1$| for a subset of |$i\in \Upsilon \subset \{1,\ldots ,n\}$|. The set |$\Upsilon $| contains |$m$| elements for case 2, while it is empty for cases 1 and 3.
3. Estimation
3.1. Estimation of the optimal treatment
If |$X$| were observed, an ideal estimator |$\hat{\beta}^{I}$| of |$\beta $| could be obtained by the standard partial likelihood approach, and the ideal estimator of |$A_{\rm opt}(X)$| is |$\hat{A}_{\rm opt}^{I}(X)=A(\hat{\beta}^{I},X)$|. Here and henceforth, we use the superscript “|$I$|” to denote the ideal approach. Since |$X$| is not observed in the original study or only observed in a subset |$\Upsilon $|, we may estimate |$\beta $| through measurement error approaches.
In case 2, |$X$| is observed in a subset |$\Upsilon $|. The partial likelihood estimator |$\hat{\beta}^{\Upsilon }$| of |$\beta $| may be obtained using observations in |$\Upsilon $| only. But this approach is not efficient as the information not in |$\Upsilon $| is not used. To improve the efficiency, following Wang and Song (2016), an improved estimator can be obtained. Specifically, it is the best linear combination of |$\hat{\beta}^{\Upsilon }$| and |$\hat{\beta}^{\Upsilon ,N}$|, which equals |$ \hat{\beta}^{\Upsilon }-\hat{\Omega}(\hat{\beta}^{\Upsilon ,N}-\hat{\beta} ^{N}),$| where |$\hat{\beta}^{\Upsilon ,N}$| and |$\hat{\beta}^{N}$| are the naive estimates of |$\beta $| obtained by substituting |$W$| for |$X$| using the observations in |$\Upsilon $| and the whole data set, respectively, and |$\hat{ \Omega}$| is given in Appendix A in the supplementary material available on Biostatistics online. For simplicity, both this estimator and the conditional score estimator are referred to as error-corrected estimators henceforth.
Denote the error-corrected estimators of |$\beta $| by |$\hat{\beta}^{C}$|. The optimal treatment can be estimated by |$\hat{A}_{\rm opt}^{C}(X)=A(\hat{\beta} ^{C},X).$| Here and henceforth, we use the superscript “|$C$|” to denote the error-corrected approach. For now, assume model (3.1) holds.
Under the conditions C1–C4 given in Appendix B available on Biostatistics online, almost surely, |$\hat{ \beta}^{C}$| exists and converges to |$\beta $|. In addition, |$n^{1/2}(\hat{ \beta}^{C}-\beta )$| converges to a mean zero normal distribution.
If model (3.1) is not the true model, it is used as a working model to obtain the treatment selection criterion. Fine (2002) showed that even if model (3.1) does not hold, the partial likelihood estimator |$\hat{\beta}^{I}$| still converges to some constant |$\beta ^{\ast }=(\beta _{1}^{\ast T},\beta _{2}^{\ast },\beta _{3}^{\ast T}).$| It can be shown that the error-corrected estimators are consistent estimators of |$\beta ^{\ast }$|. Given the value of |$X,$||$A({\ \hat{\beta} ^{I},X})$| will converge to a valid treatment selection criterion |$A(\beta ^{\ast },X),$| which is equal to |$A_{\rm opt}(X)$| when model (3.1) is correctly specified. When model (3.1) is misspecified, |$A(\beta ^{\ast },X)$| is the optimal treatment under (3.1), but may not equal to the optimal treatment |$A_{\rm opt}(X).$| We consider evaluating |$A(\beta ^{\ast },X)$| and propose an empirical estimator of |$\Theta _{X}({A(\beta ^{\ast },X)})$|.
3.2. Estimation of |$\Theta _{X}$|
When |$\Sigma $| is not known, it can be replaced by the estimator |$\hat{ \Sigma}$| obtained from the validation data or the replicated data. Specifically, |$\hat{\Sigma}=m^{-1}\sum_{j=1}^{m}(Z_{j}^{\#}-X_{j}^{\#})^{ \otimes 2}$| in case 1, |$\hat{\Sigma}=\{\sum_{i=1}^{n}(J_{i}-1)\}^{-1} \sum_{i=1}^{n}I(J_{i}>1)\sum_{j=1}^{J_{i}}(Z_{ij}-\bar{Z}_{i})^{\otimes 2}$| in case 3 and |$\hat{\Sigma}=m^{-1}\sum_{i}^{n} R_i (Z_{i1}-X_{i})^{ \otimes 2}$| in case 2.
Under the regularity conditions C1–C3 given in Appendix B available on Biostatistics online, |$\hat{\Theta}_{X}^{I}$| is a consistent estimator of |$\Theta _{X}( {A(\beta ^{\ast },X)})$| and |$n^{1/2}({\hat{\Theta}_{X}^{I}-\Theta _{X}({ A(\beta ^{\ast },X)})})$| converges to a mean zero normal distribution. Further, with the additional conditions C4–C6, |$\hat{\Theta}_{X}^{C}$| is a consistent estimator of |$\Theta _{X}({A(\beta ^{\ast },X)})$| and |$n^{1/2}({ \hat{\Theta}_{X}^{C}-\Theta _{X}({A(\beta ^{\ast },X)})})$| converges to a mean zero normal distribution.
Proposition 2 indicates that even if the model (3.1) is misspecified, the empirical estimator |$\hat{\Theta}_{X}^{C}$| is still a valid estimator of the mean restricted lifetime under the treatment selection criterion constructed based on the working model.
If model (3.1) is misspecified, it can be shown that the model-based estimator |$\hat{\Theta}_{m,X}^{\ast }$| actually estimate |$\Theta _{\rm mis}=E[E\{\int_{0}^{L}S^{\ast }(t|\beta ,\Lambda _{0}^{\ast },A,X)\int {\rm d}t|A={ A(\beta ^{\ast },X)\}}]$| with |$S^{\ast }(t|\beta ,\Lambda _{0}^{\ast },A,X)=\exp \{-\Lambda _{0}^{\ast }(t)\exp (\beta _{1}^{\#}X+\beta _{2}^{\ast }A+\beta _{3}^{\ast }XA)\}$| and |$\Lambda _{0}^{\ast }(t)=\int_{0}^{L}{\rm d}E\{N(t)\}/E(Y(t)\exp \{\beta _{1}^{\ast }X+\beta _{2}^{\ast }A+\beta _{3}^{\ast }XA\}),$| which is different from the optimal mean restricted lifetime |$\Theta _{X}({A(\beta ^{\ast },X)})$| under the working model. For example, under the scenario considered in our simulation studies in Section 4, if the true model is |$\lambda (t|X,A)=\lambda _{0}(t)\exp (-2.5X-0.5X^{2}+0.2A+5.0XA+X^{2}A)$|, the optimal mean restricted lifetime is 6.82, the optimal mean restricted lifetime is 6.79 under working model (3.1), while |$\Theta _{\rm mis}=$| 6.60. Therefore the model-based estimator may not work well for estimation of the mean restricted lifetime under the working model in this case, while the empirical estimator is still a valid estimator.
We will focus on the empirical estimation for its robustness. This approach may be applied to the standard biomarkers as described in Section 3.4, which will facilitate the comparison of the standard biomarkers and the new biomarkers.
3.3. Marker-independent treatment selection
3.4. Compare the standard and new biomarkers
This approach is called the naive approach under the literature of measurement error models. Let |$\hat{\beta}^{N}$| be the partial likelihood estimator based on the working model (3.7), where the superscript “|$N$|” is used to denote naive estimators. It can be shown that |$\hat{\beta}^{N}$| converges to some constant |$\beta ^{\#}$| (Fine, 2002). Given |$Z$|, the optimal treatment selection criterion under the working model is |$\Theta_Z=A(\beta ^{\#},Z)=I(\beta _{2}^{\#}+\beta _{3}^{\#T}Z<0)$| and can be estimated by |$A({ \hat{\beta}^{N}},Z).$| The mean restricted lifetime |$E(T^{\ast }|A=A(\beta ^{\#},Z))$| can be estimated empirically by|$\ \hat{\Theta}_{Z}^{N}=\hat{E} _{e,Z}(T^{\ast }|A=A(\hat{\beta}^{N},Z);Z),$| which is obtained by substituting |$Z$| for |$X$| and |$\hat{\beta}^{N}$| for |$\hat{\beta}^I$| in (3.4).
The overall misclassification probability |$P(A)=P_{0}(A)+P_{1}(A)=P(A\neq A_{\rm opt}).$| Thus, we may compare the treatment selection rules |${A(\beta ^{\ast },X)}$| and |$A(\beta ^{\#},Z)$| based on (3.8) and (3.9).
In the simulation studies, if |$\beta $| is estimated by |$\hat{\beta},$| t he misassignment probabilities |$P_{0}(A(X))$| and |$P_{1}(A(X))$| of using biomarker |$X\,$|can be estimated by |$\hat{P}_{0}({A(\hat{\beta},X)} )=n^{-1}\sum_{i=1}^{n}I({A(\hat{\beta},X)=0}$| and |${A_{\rm opt}=1})$| and |$\hat{ P}_{1}({A(\hat{\beta},X)})=n^{-1}\sum_{i=1}^{n}I({A(\hat{\beta},X)=1}$| and |${ \ A_{\rm opt}=0})$|, and the estimated misassignment probabilities of using |$Z$| are obtained by substituting |$Z$| for |$X.$| Note that |$\hat{P}_{0}(A,\hat{\beta },X)$| and |$\hat{P}_{1}(A,\hat{\beta},X)$| converge to zero for a consistent estimator |$\hat{\beta}$| of |$\beta $|.
When the original data contain replicated observations, |$Z$| may be replaced by the mean |$\bar{Z}$| of the replicated observations, which has better performance than |$Z$| with reduced measurement error, and we may compare the treatment selection using |$\bar{Z}$| vs. |$X$|.
3.5. Resampling-based inference
Since the asymptotic variance for the empirical estimator depends on the unknown density and hazard functions, the estimation requires smoothing and may not work well when |$n$| is not large. We develop a resampling-based approach by analogy to that used in Peng and Huang (2008). We describe how to derive the variance estimator for |$\hat{\Theta}_{X}^{C}$| in case 3; the process is similar in the other two cases. Specifically, we generate |$\{\eta _{i}:i=1,\ldots ,n\}$| from a known nonnegative distribution with mean 1. Using |$\eta _{i}$| as weights in the method of moment estimating equation, we first obtain the perturbed estimator |$\hat{\Sigma}^{P}$| of |$\Sigma ,$| where the superscript “|$P$|” stands for the perturbed estimator. Then using |$\eta _{i}$| as weights and replacing |$\Sigma $| by |$\hat{\Sigma}^{P}$| in the conditional score estimating equation (3.2), we obtain the perturbed estimator |$\hat{\beta}^{C,P}$| of |$ \beta .$| Next, we obtain the perturbed estimator |${\hat{\Theta}_{e,X}^{C,P}}$| through the perturbed SIMEX process where for each |$\zeta $| and |$b,$| replace |$X$| by |$W_{i\zeta }^{b,P}=\bar{Z}_{i}^{P}+\sqrt{\zeta }J_{i}^{-1/2} \hat{\Sigma}^{P,1/2}\varepsilon _{i\zeta }^{b}$| in |$\hat{E}_{X}(T^{\ast }|A= \hat{A}_{\rm opt}^{I};X)$| with |$\bar{Z}_{i}^{P}=n^{-1}\sum_{i=1}^{n}\eta _{i}Z_{i}.$| By repeatedly generating |$\{\eta _{i}:i=1,\ldots ,n\}$|, we obtain a large number of realization of |${\hat{\Theta}_{X}^{C,P},}$| denoted by |$\{{\hat{\Theta}_{X,r}^{C,P}}\}_{r=1}^{R}.$| It can be shown that conditional on the observed data, |$n^{1/2}\{{\hat{\Theta}_{X,r}^{C,P}-\hat{ \Theta}_{X}^{C}}\}$| has asymptotically the same distribution as |$n^{1/2}\{{ \hat{\Theta}_{X}^{C}-\Theta _{X}}\}.$| Thus the variance of |${\hat{\Theta} _{X}^{C}}$| can be estimated by the sample variance of |$\{{\hat{\Theta} _{X,r}^{C,P}}\}_{r=1}^{R},$| and the confidence interval of |${\Theta _{X}}$| can be constructed through Wald method or by the percentiles of |${\hat{\Theta }_{X,r}^{C,P}.}$| When the error variance is estimated from the validation data in case 1, the perturbed estimator of |$\hat{\Sigma}^{P}$| of |$\Sigma $| is obtained from the validation data with a separately generated set of perturbation variables |$\{\eta _{j}^{R}:j=1,\ldots ,m\}$|.
4. Simulation studies
We conducted simulation studies to evaluate the performance of the proposed approaches. Mimicking the case of c-myc gene in the ECOG study, we consider treatment selection using one biomarker. The new biomarker |$X$| was generated from a standard normal distribution, and the measurement error was generated from a normal distribution with mean 0 and variance |$\Sigma =0.1,0.3,0.5,$| or |$0.7.$| The survival data contains observations of (|$V,\Delta ,A,Z$|) on |$ n=500$| or |$2000$| subjects, where |$A$| was generated from a Bernoulli distribution with probability |$0.5$|, mimicking treatment assignment in a randomized clinical trial. The survival time was generated based on model (3.1) with |$\beta _{1}=2.5,$||$\beta _{2}=-0.2,$||$\beta _{3}=-5.0,$| and |$\lambda _{0}(t)=0.4.$| The optimal mean restricted lifetime under |$ A_{\rm opt}$| equals 6.93. The censoring time was generated from an exponential distribution with mean 20 and truncated at 12. The censoring rate was 30%. We considered three cases: (i) the error variance is estimated from an external validation data set that contains observations of (|$X,Z$|) on 500 subjects; (ii) the error variance is estimated from replicated observations of |$Z$| in the original data with |$J_{i}=2$| for |$i=1,\ldots ,n$|; (iii) an internal validation data set is available with |$m=0.2n$|. For each setting, 500 simulated data sets were generated.
We obtained the error-corrected estimators and naive estimators of |$\beta $|, |$A_{\rm opt}$|, and |$E(T^{\ast }|A=A_{\rm opt})$| as described in Section 3 . As a benchmark, we also obtained the ideal estimators of |$\beta$|, |$A_{\rm opt}$| , and |$E(T^{\ast }|A=A_{\rm opt})$|, assuming |$X$| is observed in the survival data. When the error is large, the root to the conditional score estimating equation |$U_{n}(\beta )=0$| may not exist, and there may exist some “outliers.” We calculate the bias based on the median of the estimates, and the standard error by the normalized median absolute deviation (MAD) via the resampling method. The 95% Wald confidence intervals were calculated correspondingly. For the SIMEX approaches, we adopted the rational linear extrapolation, and used the quadratic extrapolation as a backup if the rational extrapolation failed (Carroll and others, 2006). The perturbation variable |$\eta$| was generated from a location-scale transformation of beta(|$\sqrt{2}-1,1$|) and we set |$R=500$| in the resampling process and |$B=500$| in the SIMEX process.
The results of estimating |$\beta $| for |$n=500$| and |$2000$| are shown in Tables S1 and S2 in the supplementary material available at Biostatistics online, respectively. Compared to the ideal estimates, the naive estimates show clear bias with the coverage probabilities well below the nominal level; the coverage probabilities worsen when the sample size increases and error variance gets larger. The error-corrected estimates perform reasonably well for |$n=500$|, and the performance improves when the sample size increases to |$n=2000.$|
We also estimated the misassignment probabilities using the standard biomarker and the new biomarker for |$A_{\rm opt}=0$| and |$1$| separately. The misassignment probabilities would be zero if the treatment assignment was based on the true model with the true regression coefficients. The estimated misassignment probabilities are shown in Table 1. The estimated misassignment probabilities of the error-corrected estimates based on the new marker are close to the ideal estimates, and they are much lower than those from the naive approach based on the standard biomarker. When |$ n=2000$| and error standard variance increases from 0.1 to 0.7, the estimated total misassignment rate increases from 0.49% to 2.03% in case 1, 0.64% to 0.84% in case 2, and 0.44% to 0.93% in case 2 from the error-corrected approaches based on the new biomarker, while from 9.76% to 22.21% in case 1, 9.76% to 22.14% in case 2, and 6.96% to 17% in case 3 from the naive approach based on the standard marker. This shows the advantage of adopting the new biomarker, especially when the measurement error is large.
. | . | . | . | |$n=500$| . | . | |$n=2000$| . | ||||||||
---|---|---|---|---|---|---|---|---|---|---|---|---|---|---|
. | . | . | . | A|$_{\rm opt}=0$| . | . | A|$_{\rm opt}=1$| . | . | A|$_{\rm opt}=0$| . | . | A|$_{\rm opt}=1$| . | ||||
|$\Sigma ^{1/2}$| . | Marker . | Method . | . | Est . | SD . | . | Est . | SD . | . | Est . | SD . | . | Est . | SD . |
New | Ideal | 0.35 | 0.61 | 0.41 | 0.64 | 0.19 | 0.30 | 0.18 | 0.28 | |||||
Case 1 | ||||||||||||||
0.1 | New | EC | 0.49 | 0.79 | 0.56 | 0.89 | 0.26 | 0.38 | 0.23 | 0.36 | ||||
Standard | Naive | 4.91 | 1.06 | 4.93 | 1.07 | 4.94 | 0.54 | 4.82 | 0.52 | |||||
[0.05in]0.3 | New | EC | 0.77 | 1.24 | 0.75 | 1.21 | 0.40 | 0.62 | 0.38 | 0.57 | ||||
Standard | Naive | 8.00 | 1.27 | 7.99 | 1.26 | 8.12 | 0.64 | 7.84 | 0.61 | |||||
[0.05in]0.5 | New | EC | 1.02 | 1.62 | 1.07 | 1.81 | 0.69 | 1.17 | 0.54 | 0.87 | ||||
Standard | Naive | 10.00 | 1.40 | 9.63 | 1.39 | 9.98 | 0.73 | 9.61 | 0.71 | |||||
[0.05in]0.7 | New | EC | 1.31 | 2.33 | 1.92 | 3.62 | 1.04 | 2.04 | 0.99 | 1.97 | ||||
Standard | Naive | 11.46 | 1.51 | 10.85 | 1.48 | 11.37 | 0.77 | 10.84 | 0.71 | |||||
Case 2 | ||||||||||||||
0.1 | New | EC | 0.60 | 0.95 | 0.66 | 0.96 | 0.32 | 0.47 | 0.32 | 0.48 | ||||
Standard | Naive | 4.91 | 1.08 | 4.83 | 1.03 | 4.91 | 0.54 | 4.85 | 0.53 | |||||
0.3 | New | EC | 0.71 | 1.07 | 0.75 | 1.08 | 0.38 | 0.55 | 0.38 | 0.57 | ||||
Standard | Naive | 8.05 | 1.26 | 7.82 | 1.24 | 8.09 | 0.67 | 7.88 | 0.64 | |||||
0.5 | New | EC | 0.73 | 1.11 | 0.78 | 1.12 | 0.41 | 0.59 | 0.41 | 0.61 | ||||
Standard | Naive | 9.93 | 1.41 | 9.54 | 1.33 | 9.95 | 0.70 | 9.63 | 0.71 | |||||
0.7 | New | EC | 0.74 | 1.13 | 0.80 | 1.14 | 0.42 | 0.61 | 0.42 | 0.63 | ||||
Standard | Naive | 11.30 | 1.45 | 10.78 | 1.43 | 11.30 | 0.74 | 10.84 | 0.75 | |||||
Case 3 | ||||||||||||||
0.1 | New | EC | 0.47 | 0.73 | 0.43 | 0.71 | 0.21 | 0.33 | 0.23 | 0.35 | ||||
Standard | Naive | 3.59 | 0.96 | 3.47 | 0.88 | 3.50 | 0.48 | 3.46 | 0.47 | |||||
[0.05in]0.3 | New | EC | 0.60 | 0.94 | 0.57 | 0.91 | 0.25 | 0.40 | 0.31 | 0.45 | ||||
Standard | Naive | 5.96 | 1.13 | 5.80 | 1.08 | 5.92 | 0.56 | 5.80 | 0.55 | |||||
[0.05in]0.5 | New | EC | 0.75 | 1.27 | 0.71 | 1.09 | 0.31 | 0.49 | 0.39 | 0.56 | ||||
Standard | Naive | 7.43 | 1.23 | 7.26 | 1.19 | 7.44 | 0.62 | 7.25 | 0.59 | |||||
[0.05in]0.7 | New | EC | 0.78 | 1.28 | 0.96 | 1.56 | 0.47 | 0.76 | 0.46 | 0.72 | ||||
Standard | naive | 8.60 | 1.26 | 8.30 | 1.34 | 8.64 | 0.68 | 8.36 | 0.65 |
. | . | . | . | |$n=500$| . | . | |$n=2000$| . | ||||||||
---|---|---|---|---|---|---|---|---|---|---|---|---|---|---|
. | . | . | . | A|$_{\rm opt}=0$| . | . | A|$_{\rm opt}=1$| . | . | A|$_{\rm opt}=0$| . | . | A|$_{\rm opt}=1$| . | ||||
|$\Sigma ^{1/2}$| . | Marker . | Method . | . | Est . | SD . | . | Est . | SD . | . | Est . | SD . | . | Est . | SD . |
New | Ideal | 0.35 | 0.61 | 0.41 | 0.64 | 0.19 | 0.30 | 0.18 | 0.28 | |||||
Case 1 | ||||||||||||||
0.1 | New | EC | 0.49 | 0.79 | 0.56 | 0.89 | 0.26 | 0.38 | 0.23 | 0.36 | ||||
Standard | Naive | 4.91 | 1.06 | 4.93 | 1.07 | 4.94 | 0.54 | 4.82 | 0.52 | |||||
[0.05in]0.3 | New | EC | 0.77 | 1.24 | 0.75 | 1.21 | 0.40 | 0.62 | 0.38 | 0.57 | ||||
Standard | Naive | 8.00 | 1.27 | 7.99 | 1.26 | 8.12 | 0.64 | 7.84 | 0.61 | |||||
[0.05in]0.5 | New | EC | 1.02 | 1.62 | 1.07 | 1.81 | 0.69 | 1.17 | 0.54 | 0.87 | ||||
Standard | Naive | 10.00 | 1.40 | 9.63 | 1.39 | 9.98 | 0.73 | 9.61 | 0.71 | |||||
[0.05in]0.7 | New | EC | 1.31 | 2.33 | 1.92 | 3.62 | 1.04 | 2.04 | 0.99 | 1.97 | ||||
Standard | Naive | 11.46 | 1.51 | 10.85 | 1.48 | 11.37 | 0.77 | 10.84 | 0.71 | |||||
Case 2 | ||||||||||||||
0.1 | New | EC | 0.60 | 0.95 | 0.66 | 0.96 | 0.32 | 0.47 | 0.32 | 0.48 | ||||
Standard | Naive | 4.91 | 1.08 | 4.83 | 1.03 | 4.91 | 0.54 | 4.85 | 0.53 | |||||
0.3 | New | EC | 0.71 | 1.07 | 0.75 | 1.08 | 0.38 | 0.55 | 0.38 | 0.57 | ||||
Standard | Naive | 8.05 | 1.26 | 7.82 | 1.24 | 8.09 | 0.67 | 7.88 | 0.64 | |||||
0.5 | New | EC | 0.73 | 1.11 | 0.78 | 1.12 | 0.41 | 0.59 | 0.41 | 0.61 | ||||
Standard | Naive | 9.93 | 1.41 | 9.54 | 1.33 | 9.95 | 0.70 | 9.63 | 0.71 | |||||
0.7 | New | EC | 0.74 | 1.13 | 0.80 | 1.14 | 0.42 | 0.61 | 0.42 | 0.63 | ||||
Standard | Naive | 11.30 | 1.45 | 10.78 | 1.43 | 11.30 | 0.74 | 10.84 | 0.75 | |||||
Case 3 | ||||||||||||||
0.1 | New | EC | 0.47 | 0.73 | 0.43 | 0.71 | 0.21 | 0.33 | 0.23 | 0.35 | ||||
Standard | Naive | 3.59 | 0.96 | 3.47 | 0.88 | 3.50 | 0.48 | 3.46 | 0.47 | |||||
[0.05in]0.3 | New | EC | 0.60 | 0.94 | 0.57 | 0.91 | 0.25 | 0.40 | 0.31 | 0.45 | ||||
Standard | Naive | 5.96 | 1.13 | 5.80 | 1.08 | 5.92 | 0.56 | 5.80 | 0.55 | |||||
[0.05in]0.5 | New | EC | 0.75 | 1.27 | 0.71 | 1.09 | 0.31 | 0.49 | 0.39 | 0.56 | ||||
Standard | Naive | 7.43 | 1.23 | 7.26 | 1.19 | 7.44 | 0.62 | 7.25 | 0.59 | |||||
[0.05in]0.7 | New | EC | 0.78 | 1.28 | 0.96 | 1.56 | 0.47 | 0.76 | 0.46 | 0.72 | ||||
Standard | naive | 8.60 | 1.26 | 8.30 | 1.34 | 8.64 | 0.68 | 8.36 | 0.65 |
EC, error corrected; Est, estimate; SD, empirical standard deviation.
. | . | . | . | |$n=500$| . | . | |$n=2000$| . | ||||||||
---|---|---|---|---|---|---|---|---|---|---|---|---|---|---|
. | . | . | . | A|$_{\rm opt}=0$| . | . | A|$_{\rm opt}=1$| . | . | A|$_{\rm opt}=0$| . | . | A|$_{\rm opt}=1$| . | ||||
|$\Sigma ^{1/2}$| . | Marker . | Method . | . | Est . | SD . | . | Est . | SD . | . | Est . | SD . | . | Est . | SD . |
New | Ideal | 0.35 | 0.61 | 0.41 | 0.64 | 0.19 | 0.30 | 0.18 | 0.28 | |||||
Case 1 | ||||||||||||||
0.1 | New | EC | 0.49 | 0.79 | 0.56 | 0.89 | 0.26 | 0.38 | 0.23 | 0.36 | ||||
Standard | Naive | 4.91 | 1.06 | 4.93 | 1.07 | 4.94 | 0.54 | 4.82 | 0.52 | |||||
[0.05in]0.3 | New | EC | 0.77 | 1.24 | 0.75 | 1.21 | 0.40 | 0.62 | 0.38 | 0.57 | ||||
Standard | Naive | 8.00 | 1.27 | 7.99 | 1.26 | 8.12 | 0.64 | 7.84 | 0.61 | |||||
[0.05in]0.5 | New | EC | 1.02 | 1.62 | 1.07 | 1.81 | 0.69 | 1.17 | 0.54 | 0.87 | ||||
Standard | Naive | 10.00 | 1.40 | 9.63 | 1.39 | 9.98 | 0.73 | 9.61 | 0.71 | |||||
[0.05in]0.7 | New | EC | 1.31 | 2.33 | 1.92 | 3.62 | 1.04 | 2.04 | 0.99 | 1.97 | ||||
Standard | Naive | 11.46 | 1.51 | 10.85 | 1.48 | 11.37 | 0.77 | 10.84 | 0.71 | |||||
Case 2 | ||||||||||||||
0.1 | New | EC | 0.60 | 0.95 | 0.66 | 0.96 | 0.32 | 0.47 | 0.32 | 0.48 | ||||
Standard | Naive | 4.91 | 1.08 | 4.83 | 1.03 | 4.91 | 0.54 | 4.85 | 0.53 | |||||
0.3 | New | EC | 0.71 | 1.07 | 0.75 | 1.08 | 0.38 | 0.55 | 0.38 | 0.57 | ||||
Standard | Naive | 8.05 | 1.26 | 7.82 | 1.24 | 8.09 | 0.67 | 7.88 | 0.64 | |||||
0.5 | New | EC | 0.73 | 1.11 | 0.78 | 1.12 | 0.41 | 0.59 | 0.41 | 0.61 | ||||
Standard | Naive | 9.93 | 1.41 | 9.54 | 1.33 | 9.95 | 0.70 | 9.63 | 0.71 | |||||
0.7 | New | EC | 0.74 | 1.13 | 0.80 | 1.14 | 0.42 | 0.61 | 0.42 | 0.63 | ||||
Standard | Naive | 11.30 | 1.45 | 10.78 | 1.43 | 11.30 | 0.74 | 10.84 | 0.75 | |||||
Case 3 | ||||||||||||||
0.1 | New | EC | 0.47 | 0.73 | 0.43 | 0.71 | 0.21 | 0.33 | 0.23 | 0.35 | ||||
Standard | Naive | 3.59 | 0.96 | 3.47 | 0.88 | 3.50 | 0.48 | 3.46 | 0.47 | |||||
[0.05in]0.3 | New | EC | 0.60 | 0.94 | 0.57 | 0.91 | 0.25 | 0.40 | 0.31 | 0.45 | ||||
Standard | Naive | 5.96 | 1.13 | 5.80 | 1.08 | 5.92 | 0.56 | 5.80 | 0.55 | |||||
[0.05in]0.5 | New | EC | 0.75 | 1.27 | 0.71 | 1.09 | 0.31 | 0.49 | 0.39 | 0.56 | ||||
Standard | Naive | 7.43 | 1.23 | 7.26 | 1.19 | 7.44 | 0.62 | 7.25 | 0.59 | |||||
[0.05in]0.7 | New | EC | 0.78 | 1.28 | 0.96 | 1.56 | 0.47 | 0.76 | 0.46 | 0.72 | ||||
Standard | naive | 8.60 | 1.26 | 8.30 | 1.34 | 8.64 | 0.68 | 8.36 | 0.65 |
. | . | . | . | |$n=500$| . | . | |$n=2000$| . | ||||||||
---|---|---|---|---|---|---|---|---|---|---|---|---|---|---|
. | . | . | . | A|$_{\rm opt}=0$| . | . | A|$_{\rm opt}=1$| . | . | A|$_{\rm opt}=0$| . | . | A|$_{\rm opt}=1$| . | ||||
|$\Sigma ^{1/2}$| . | Marker . | Method . | . | Est . | SD . | . | Est . | SD . | . | Est . | SD . | . | Est . | SD . |
New | Ideal | 0.35 | 0.61 | 0.41 | 0.64 | 0.19 | 0.30 | 0.18 | 0.28 | |||||
Case 1 | ||||||||||||||
0.1 | New | EC | 0.49 | 0.79 | 0.56 | 0.89 | 0.26 | 0.38 | 0.23 | 0.36 | ||||
Standard | Naive | 4.91 | 1.06 | 4.93 | 1.07 | 4.94 | 0.54 | 4.82 | 0.52 | |||||
[0.05in]0.3 | New | EC | 0.77 | 1.24 | 0.75 | 1.21 | 0.40 | 0.62 | 0.38 | 0.57 | ||||
Standard | Naive | 8.00 | 1.27 | 7.99 | 1.26 | 8.12 | 0.64 | 7.84 | 0.61 | |||||
[0.05in]0.5 | New | EC | 1.02 | 1.62 | 1.07 | 1.81 | 0.69 | 1.17 | 0.54 | 0.87 | ||||
Standard | Naive | 10.00 | 1.40 | 9.63 | 1.39 | 9.98 | 0.73 | 9.61 | 0.71 | |||||
[0.05in]0.7 | New | EC | 1.31 | 2.33 | 1.92 | 3.62 | 1.04 | 2.04 | 0.99 | 1.97 | ||||
Standard | Naive | 11.46 | 1.51 | 10.85 | 1.48 | 11.37 | 0.77 | 10.84 | 0.71 | |||||
Case 2 | ||||||||||||||
0.1 | New | EC | 0.60 | 0.95 | 0.66 | 0.96 | 0.32 | 0.47 | 0.32 | 0.48 | ||||
Standard | Naive | 4.91 | 1.08 | 4.83 | 1.03 | 4.91 | 0.54 | 4.85 | 0.53 | |||||
0.3 | New | EC | 0.71 | 1.07 | 0.75 | 1.08 | 0.38 | 0.55 | 0.38 | 0.57 | ||||
Standard | Naive | 8.05 | 1.26 | 7.82 | 1.24 | 8.09 | 0.67 | 7.88 | 0.64 | |||||
0.5 | New | EC | 0.73 | 1.11 | 0.78 | 1.12 | 0.41 | 0.59 | 0.41 | 0.61 | ||||
Standard | Naive | 9.93 | 1.41 | 9.54 | 1.33 | 9.95 | 0.70 | 9.63 | 0.71 | |||||
0.7 | New | EC | 0.74 | 1.13 | 0.80 | 1.14 | 0.42 | 0.61 | 0.42 | 0.63 | ||||
Standard | Naive | 11.30 | 1.45 | 10.78 | 1.43 | 11.30 | 0.74 | 10.84 | 0.75 | |||||
Case 3 | ||||||||||||||
0.1 | New | EC | 0.47 | 0.73 | 0.43 | 0.71 | 0.21 | 0.33 | 0.23 | 0.35 | ||||
Standard | Naive | 3.59 | 0.96 | 3.47 | 0.88 | 3.50 | 0.48 | 3.46 | 0.47 | |||||
[0.05in]0.3 | New | EC | 0.60 | 0.94 | 0.57 | 0.91 | 0.25 | 0.40 | 0.31 | 0.45 | ||||
Standard | Naive | 5.96 | 1.13 | 5.80 | 1.08 | 5.92 | 0.56 | 5.80 | 0.55 | |||||
[0.05in]0.5 | New | EC | 0.75 | 1.27 | 0.71 | 1.09 | 0.31 | 0.49 | 0.39 | 0.56 | ||||
Standard | Naive | 7.43 | 1.23 | 7.26 | 1.19 | 7.44 | 0.62 | 7.25 | 0.59 | |||||
[0.05in]0.7 | New | EC | 0.78 | 1.28 | 0.96 | 1.56 | 0.47 | 0.76 | 0.46 | 0.72 | ||||
Standard | naive | 8.60 | 1.26 | 8.30 | 1.34 | 8.64 | 0.68 | 8.36 | 0.65 |
EC, error corrected; Est, estimate; SD, empirical standard deviation.
We further estimated the mean restricted lifetime within 10 years using the new biomarker and the standard biomarker. The results are shown in Table 2. The optimal mean restricted lifetime is 6.93 using the new marker, whereas when |$\Sigma ^{1/2}$| increases from 0.1 to 0.7, the mean restricted lifetime based on the standard marker decreases from 6.72 to 6.02 in cases 1 and 2 and 6.82 to 6.35 in case 3. The estimates perform reasonably well for |$n=500$| and improves when |$n=2000$|.
Simulation results for estimation of |$\Theta _{X}$| and |$\Theta _{\bar{Z}}$|.
. | . | . | . | |$n=500$| . | . | |$n=2000$| . | ||||||
---|---|---|---|---|---|---|---|---|---|---|---|---|
|$\Sigma ^{1/2}$| . | True . | Method . | . | B . | MAD . | RMAD . | CP . | . | B . | MAD . | RMAD . | CP . |
Estimation of |$\Theta _{X}$| | ||||||||||||
[0.05in] | 6.931 | Ideal | |$\!\!\!\!-$|0.034 | 0.245 | 0.247 | 0.950 | |$\!\!\!\!-$|0.013 | 0.122 | 0.123 | 0.982 | ||
Case 1 | ||||||||||||
0.1 | 6.931 | EC | 0.007 | 0.379 | 0.474 | 0.948 | |$\!\!\!\!-$|0.007 | 0.168 | 0.197 | 0.978 | ||
0.3 | 6.931 | EC | |$\!\!\!\!-$|0.041 | 0.498 | 0.637 | 0.942 | |$\!\!\!\!-$|0.032 | 0.242 | 0.272 | 0.950 | ||
0.5 | 6.931 | EC | |$\!\!\!\!-$|0.101 | 0.582 | 0.805 | 0.913 | |$\!\!\!\!-$|0.104 | 0.283 | 0.345 | 0.948 | ||
0.7 | 6.931 | EC | |$\!\!\!\!-$|0.211 | 0.632 | 0.894 | 0.922 | |$\!\!\!\!-$|0.141 | 0.333 | 0.411 | 0.910 | ||
Case 2 | ||||||||||||
0.1 | 6.931 | EC | 0.013 | 0.402 | 0.452 | 0.906 | |$\!\!\!\!-$|0.007 | 0.176 | 0.195 | 0.964 | ||
0.3 | 6.931 | EC | |$\!\!\!\!-$|0.033 | 0.440 | 0.603 | 0.940 | |$\!\!\!\!-$|0.041 | 0.211 | 0.256 | 0.962 | ||
0.5 | 6.931 | EC | |$\!\!\!\!-$|0.016 | 0.578 | 0.776 | 0.920 | |$\!\!\!\!-$|0.064 | 0.286 | 0.324 | 0.936 | ||
0.7 | 6.931 | EC | |$\!\!\!\!-$|0.059 | 0.700 | 0.966 | 0.890 | |$\!\!\!\!-$|0.086 | 0.320 | 0.394 | 0.936 | ||
Case 3 | ||||||||||||
0.1 | 6.931 | EC | 0.001 | 0.353 | 0.412 | 0.934 | 0.015 | 0.149 | 0.179 | 0.952 | ||
0.3 | 6.931 | EC | |$\!\!\!\!-$|0.019 | 0.367 | 0.477 | 0.964 | |$\!\!\!\!-$|0.012 | 0.186 | 0.211 | 0.958 | ||
0.5 | 6.931 | EC | |$\!\!\!\!-$|0.026 | 0.425 | 0.549 | 0.970 | |$\!\!\!\!-$|0.025 | 0.228 | 0.249 | 0.958 | ||
0.7 | 6.931 | EC | |$\!\!\!\!-$|0.043 | 0.550 | 0.676 | 0.920 | |$\!\!\!\!-$|0.013 | 0.265 | 0.293 | 0.938 | ||
Estimation of |$\Theta _{\bar{Z}}$| | ||||||||||||
Case 1 | ||||||||||||
0.1 | 6.720 | Naive | |$\!\!\!\!-$|0.039 | 0.255 | 0.255 | 0.940 | |$\!\!\!\!-$|0.009 | 0.114 | 0.127 | 0.958 | ||
0.3 | 6.411 | Naive | |$\!\!\!\!-$|0.032 | 0.283 | 0.263 | 0.968 | |$\!\!\!\!-$|0.040 | 0.119 | 0.132 | 0.956 | ||
0.5 | 6.186 | Naive | |$\!\!\!\!-$|0.053 | 0.271 | 0.270 | 0.942 | |$\!\!\!\!-$|0.013 | 0.142 | 0.134 | 0.964 | ||
0.7 | 6.017 | Naive | |$\!\!\!\!-$|0.035 | 0.264 | 0.274 | 0.938 | |$\!\!\!\!-$|0.011 | 0.135 | 0.136 | 0.974 | ||
Case 2 | ||||||||||||
0.1 | 6.720 | Naive | |$\!\!\!\!-$|0.036 | 0.262 | 0.255 | 0.940 | |$\!\!\!\!-$|0.011 | 0.125 | 0.127 | 0.958 | ||
0.3 | 6.411 | Naive | |$\!\!\!\!-$|0.026 | 0.257 | 0.265 | 0.952 | |$\!\!\!\!-$|0.015 | 0.121 | 0.131 | 0.952 | ||
0.5 | 6.186 | Naive | |$\!\!\!\!-$|0.022 | 0.255 | 0.271 | 0.942 | |$\!\!\!\!-$|0.008 | 0.126 | 0.134 | 0.954 | ||
0.7 | 6.017 | Naive | |$\!\!\!-$|0.019 | 0.261 | 0.274 | 0.940 | |$\!\!\!\!-$|0.011 | 0.121 | 0.136 | 0.962 | ||
Case 3 | ||||||||||||
0.1 | 6.818 | Naive | |$\!\!\!\!-$|0.023 | 0.251 | 0.253 | 0.958 | |$\!\!\!\!-$|0.001 | 0.121 | 0.126 | 0.940 | ||
0.3 | 6.629 | Naive | |$\!\!\!\!-$|0.023 | 0.243 | 0.258 | 0.958 | 0.000 | 0.135 | 0.129 | 0.942 | ||
0.5 | 6.476 | Naive | |$\!\!\!\!-$|0.016 | 0.258 | 0.262 | 0.954 | 0.003 | 0.131 | 0.131 | 0.954 | ||
0.7 | 6.347 | Naive | |$\!\!\!\!-$|0.018 | 0.274 | 0.267 | 0.938 | |$\!\!\!\!-$|0.005 | 0.136 | 0.132 | 0.948 |
. | . | . | . | |$n=500$| . | . | |$n=2000$| . | ||||||
---|---|---|---|---|---|---|---|---|---|---|---|---|
|$\Sigma ^{1/2}$| . | True . | Method . | . | B . | MAD . | RMAD . | CP . | . | B . | MAD . | RMAD . | CP . |
Estimation of |$\Theta _{X}$| | ||||||||||||
[0.05in] | 6.931 | Ideal | |$\!\!\!\!-$|0.034 | 0.245 | 0.247 | 0.950 | |$\!\!\!\!-$|0.013 | 0.122 | 0.123 | 0.982 | ||
Case 1 | ||||||||||||
0.1 | 6.931 | EC | 0.007 | 0.379 | 0.474 | 0.948 | |$\!\!\!\!-$|0.007 | 0.168 | 0.197 | 0.978 | ||
0.3 | 6.931 | EC | |$\!\!\!\!-$|0.041 | 0.498 | 0.637 | 0.942 | |$\!\!\!\!-$|0.032 | 0.242 | 0.272 | 0.950 | ||
0.5 | 6.931 | EC | |$\!\!\!\!-$|0.101 | 0.582 | 0.805 | 0.913 | |$\!\!\!\!-$|0.104 | 0.283 | 0.345 | 0.948 | ||
0.7 | 6.931 | EC | |$\!\!\!\!-$|0.211 | 0.632 | 0.894 | 0.922 | |$\!\!\!\!-$|0.141 | 0.333 | 0.411 | 0.910 | ||
Case 2 | ||||||||||||
0.1 | 6.931 | EC | 0.013 | 0.402 | 0.452 | 0.906 | |$\!\!\!\!-$|0.007 | 0.176 | 0.195 | 0.964 | ||
0.3 | 6.931 | EC | |$\!\!\!\!-$|0.033 | 0.440 | 0.603 | 0.940 | |$\!\!\!\!-$|0.041 | 0.211 | 0.256 | 0.962 | ||
0.5 | 6.931 | EC | |$\!\!\!\!-$|0.016 | 0.578 | 0.776 | 0.920 | |$\!\!\!\!-$|0.064 | 0.286 | 0.324 | 0.936 | ||
0.7 | 6.931 | EC | |$\!\!\!\!-$|0.059 | 0.700 | 0.966 | 0.890 | |$\!\!\!\!-$|0.086 | 0.320 | 0.394 | 0.936 | ||
Case 3 | ||||||||||||
0.1 | 6.931 | EC | 0.001 | 0.353 | 0.412 | 0.934 | 0.015 | 0.149 | 0.179 | 0.952 | ||
0.3 | 6.931 | EC | |$\!\!\!\!-$|0.019 | 0.367 | 0.477 | 0.964 | |$\!\!\!\!-$|0.012 | 0.186 | 0.211 | 0.958 | ||
0.5 | 6.931 | EC | |$\!\!\!\!-$|0.026 | 0.425 | 0.549 | 0.970 | |$\!\!\!\!-$|0.025 | 0.228 | 0.249 | 0.958 | ||
0.7 | 6.931 | EC | |$\!\!\!\!-$|0.043 | 0.550 | 0.676 | 0.920 | |$\!\!\!\!-$|0.013 | 0.265 | 0.293 | 0.938 | ||
Estimation of |$\Theta _{\bar{Z}}$| | ||||||||||||
Case 1 | ||||||||||||
0.1 | 6.720 | Naive | |$\!\!\!\!-$|0.039 | 0.255 | 0.255 | 0.940 | |$\!\!\!\!-$|0.009 | 0.114 | 0.127 | 0.958 | ||
0.3 | 6.411 | Naive | |$\!\!\!\!-$|0.032 | 0.283 | 0.263 | 0.968 | |$\!\!\!\!-$|0.040 | 0.119 | 0.132 | 0.956 | ||
0.5 | 6.186 | Naive | |$\!\!\!\!-$|0.053 | 0.271 | 0.270 | 0.942 | |$\!\!\!\!-$|0.013 | 0.142 | 0.134 | 0.964 | ||
0.7 | 6.017 | Naive | |$\!\!\!\!-$|0.035 | 0.264 | 0.274 | 0.938 | |$\!\!\!\!-$|0.011 | 0.135 | 0.136 | 0.974 | ||
Case 2 | ||||||||||||
0.1 | 6.720 | Naive | |$\!\!\!\!-$|0.036 | 0.262 | 0.255 | 0.940 | |$\!\!\!\!-$|0.011 | 0.125 | 0.127 | 0.958 | ||
0.3 | 6.411 | Naive | |$\!\!\!\!-$|0.026 | 0.257 | 0.265 | 0.952 | |$\!\!\!\!-$|0.015 | 0.121 | 0.131 | 0.952 | ||
0.5 | 6.186 | Naive | |$\!\!\!\!-$|0.022 | 0.255 | 0.271 | 0.942 | |$\!\!\!\!-$|0.008 | 0.126 | 0.134 | 0.954 | ||
0.7 | 6.017 | Naive | |$\!\!\!-$|0.019 | 0.261 | 0.274 | 0.940 | |$\!\!\!\!-$|0.011 | 0.121 | 0.136 | 0.962 | ||
Case 3 | ||||||||||||
0.1 | 6.818 | Naive | |$\!\!\!\!-$|0.023 | 0.251 | 0.253 | 0.958 | |$\!\!\!\!-$|0.001 | 0.121 | 0.126 | 0.940 | ||
0.3 | 6.629 | Naive | |$\!\!\!\!-$|0.023 | 0.243 | 0.258 | 0.958 | 0.000 | 0.135 | 0.129 | 0.942 | ||
0.5 | 6.476 | Naive | |$\!\!\!\!-$|0.016 | 0.258 | 0.262 | 0.954 | 0.003 | 0.131 | 0.131 | 0.954 | ||
0.7 | 6.347 | Naive | |$\!\!\!\!-$|0.018 | 0.274 | 0.267 | 0.938 | |$\!\!\!\!-$|0.005 | 0.136 | 0.132 | 0.948 |
EC, error corrected; B, empirical bias based on the median; MAD, empirical median absolute deviation; RMAD, resampling median absolute deviation; CP, empirical coverage probability of 95% confidence interval.
Simulation results for estimation of |$\Theta _{X}$| and |$\Theta _{\bar{Z}}$|.
. | . | . | . | |$n=500$| . | . | |$n=2000$| . | ||||||
---|---|---|---|---|---|---|---|---|---|---|---|---|
|$\Sigma ^{1/2}$| . | True . | Method . | . | B . | MAD . | RMAD . | CP . | . | B . | MAD . | RMAD . | CP . |
Estimation of |$\Theta _{X}$| | ||||||||||||
[0.05in] | 6.931 | Ideal | |$\!\!\!\!-$|0.034 | 0.245 | 0.247 | 0.950 | |$\!\!\!\!-$|0.013 | 0.122 | 0.123 | 0.982 | ||
Case 1 | ||||||||||||
0.1 | 6.931 | EC | 0.007 | 0.379 | 0.474 | 0.948 | |$\!\!\!\!-$|0.007 | 0.168 | 0.197 | 0.978 | ||
0.3 | 6.931 | EC | |$\!\!\!\!-$|0.041 | 0.498 | 0.637 | 0.942 | |$\!\!\!\!-$|0.032 | 0.242 | 0.272 | 0.950 | ||
0.5 | 6.931 | EC | |$\!\!\!\!-$|0.101 | 0.582 | 0.805 | 0.913 | |$\!\!\!\!-$|0.104 | 0.283 | 0.345 | 0.948 | ||
0.7 | 6.931 | EC | |$\!\!\!\!-$|0.211 | 0.632 | 0.894 | 0.922 | |$\!\!\!\!-$|0.141 | 0.333 | 0.411 | 0.910 | ||
Case 2 | ||||||||||||
0.1 | 6.931 | EC | 0.013 | 0.402 | 0.452 | 0.906 | |$\!\!\!\!-$|0.007 | 0.176 | 0.195 | 0.964 | ||
0.3 | 6.931 | EC | |$\!\!\!\!-$|0.033 | 0.440 | 0.603 | 0.940 | |$\!\!\!\!-$|0.041 | 0.211 | 0.256 | 0.962 | ||
0.5 | 6.931 | EC | |$\!\!\!\!-$|0.016 | 0.578 | 0.776 | 0.920 | |$\!\!\!\!-$|0.064 | 0.286 | 0.324 | 0.936 | ||
0.7 | 6.931 | EC | |$\!\!\!\!-$|0.059 | 0.700 | 0.966 | 0.890 | |$\!\!\!\!-$|0.086 | 0.320 | 0.394 | 0.936 | ||
Case 3 | ||||||||||||
0.1 | 6.931 | EC | 0.001 | 0.353 | 0.412 | 0.934 | 0.015 | 0.149 | 0.179 | 0.952 | ||
0.3 | 6.931 | EC | |$\!\!\!\!-$|0.019 | 0.367 | 0.477 | 0.964 | |$\!\!\!\!-$|0.012 | 0.186 | 0.211 | 0.958 | ||
0.5 | 6.931 | EC | |$\!\!\!\!-$|0.026 | 0.425 | 0.549 | 0.970 | |$\!\!\!\!-$|0.025 | 0.228 | 0.249 | 0.958 | ||
0.7 | 6.931 | EC | |$\!\!\!\!-$|0.043 | 0.550 | 0.676 | 0.920 | |$\!\!\!\!-$|0.013 | 0.265 | 0.293 | 0.938 | ||
Estimation of |$\Theta _{\bar{Z}}$| | ||||||||||||
Case 1 | ||||||||||||
0.1 | 6.720 | Naive | |$\!\!\!\!-$|0.039 | 0.255 | 0.255 | 0.940 | |$\!\!\!\!-$|0.009 | 0.114 | 0.127 | 0.958 | ||
0.3 | 6.411 | Naive | |$\!\!\!\!-$|0.032 | 0.283 | 0.263 | 0.968 | |$\!\!\!\!-$|0.040 | 0.119 | 0.132 | 0.956 | ||
0.5 | 6.186 | Naive | |$\!\!\!\!-$|0.053 | 0.271 | 0.270 | 0.942 | |$\!\!\!\!-$|0.013 | 0.142 | 0.134 | 0.964 | ||
0.7 | 6.017 | Naive | |$\!\!\!\!-$|0.035 | 0.264 | 0.274 | 0.938 | |$\!\!\!\!-$|0.011 | 0.135 | 0.136 | 0.974 | ||
Case 2 | ||||||||||||
0.1 | 6.720 | Naive | |$\!\!\!\!-$|0.036 | 0.262 | 0.255 | 0.940 | |$\!\!\!\!-$|0.011 | 0.125 | 0.127 | 0.958 | ||
0.3 | 6.411 | Naive | |$\!\!\!\!-$|0.026 | 0.257 | 0.265 | 0.952 | |$\!\!\!\!-$|0.015 | 0.121 | 0.131 | 0.952 | ||
0.5 | 6.186 | Naive | |$\!\!\!\!-$|0.022 | 0.255 | 0.271 | 0.942 | |$\!\!\!\!-$|0.008 | 0.126 | 0.134 | 0.954 | ||
0.7 | 6.017 | Naive | |$\!\!\!-$|0.019 | 0.261 | 0.274 | 0.940 | |$\!\!\!\!-$|0.011 | 0.121 | 0.136 | 0.962 | ||
Case 3 | ||||||||||||
0.1 | 6.818 | Naive | |$\!\!\!\!-$|0.023 | 0.251 | 0.253 | 0.958 | |$\!\!\!\!-$|0.001 | 0.121 | 0.126 | 0.940 | ||
0.3 | 6.629 | Naive | |$\!\!\!\!-$|0.023 | 0.243 | 0.258 | 0.958 | 0.000 | 0.135 | 0.129 | 0.942 | ||
0.5 | 6.476 | Naive | |$\!\!\!\!-$|0.016 | 0.258 | 0.262 | 0.954 | 0.003 | 0.131 | 0.131 | 0.954 | ||
0.7 | 6.347 | Naive | |$\!\!\!\!-$|0.018 | 0.274 | 0.267 | 0.938 | |$\!\!\!\!-$|0.005 | 0.136 | 0.132 | 0.948 |
. | . | . | . | |$n=500$| . | . | |$n=2000$| . | ||||||
---|---|---|---|---|---|---|---|---|---|---|---|---|
|$\Sigma ^{1/2}$| . | True . | Method . | . | B . | MAD . | RMAD . | CP . | . | B . | MAD . | RMAD . | CP . |
Estimation of |$\Theta _{X}$| | ||||||||||||
[0.05in] | 6.931 | Ideal | |$\!\!\!\!-$|0.034 | 0.245 | 0.247 | 0.950 | |$\!\!\!\!-$|0.013 | 0.122 | 0.123 | 0.982 | ||
Case 1 | ||||||||||||
0.1 | 6.931 | EC | 0.007 | 0.379 | 0.474 | 0.948 | |$\!\!\!\!-$|0.007 | 0.168 | 0.197 | 0.978 | ||
0.3 | 6.931 | EC | |$\!\!\!\!-$|0.041 | 0.498 | 0.637 | 0.942 | |$\!\!\!\!-$|0.032 | 0.242 | 0.272 | 0.950 | ||
0.5 | 6.931 | EC | |$\!\!\!\!-$|0.101 | 0.582 | 0.805 | 0.913 | |$\!\!\!\!-$|0.104 | 0.283 | 0.345 | 0.948 | ||
0.7 | 6.931 | EC | |$\!\!\!\!-$|0.211 | 0.632 | 0.894 | 0.922 | |$\!\!\!\!-$|0.141 | 0.333 | 0.411 | 0.910 | ||
Case 2 | ||||||||||||
0.1 | 6.931 | EC | 0.013 | 0.402 | 0.452 | 0.906 | |$\!\!\!\!-$|0.007 | 0.176 | 0.195 | 0.964 | ||
0.3 | 6.931 | EC | |$\!\!\!\!-$|0.033 | 0.440 | 0.603 | 0.940 | |$\!\!\!\!-$|0.041 | 0.211 | 0.256 | 0.962 | ||
0.5 | 6.931 | EC | |$\!\!\!\!-$|0.016 | 0.578 | 0.776 | 0.920 | |$\!\!\!\!-$|0.064 | 0.286 | 0.324 | 0.936 | ||
0.7 | 6.931 | EC | |$\!\!\!\!-$|0.059 | 0.700 | 0.966 | 0.890 | |$\!\!\!\!-$|0.086 | 0.320 | 0.394 | 0.936 | ||
Case 3 | ||||||||||||
0.1 | 6.931 | EC | 0.001 | 0.353 | 0.412 | 0.934 | 0.015 | 0.149 | 0.179 | 0.952 | ||
0.3 | 6.931 | EC | |$\!\!\!\!-$|0.019 | 0.367 | 0.477 | 0.964 | |$\!\!\!\!-$|0.012 | 0.186 | 0.211 | 0.958 | ||
0.5 | 6.931 | EC | |$\!\!\!\!-$|0.026 | 0.425 | 0.549 | 0.970 | |$\!\!\!\!-$|0.025 | 0.228 | 0.249 | 0.958 | ||
0.7 | 6.931 | EC | |$\!\!\!\!-$|0.043 | 0.550 | 0.676 | 0.920 | |$\!\!\!\!-$|0.013 | 0.265 | 0.293 | 0.938 | ||
Estimation of |$\Theta _{\bar{Z}}$| | ||||||||||||
Case 1 | ||||||||||||
0.1 | 6.720 | Naive | |$\!\!\!\!-$|0.039 | 0.255 | 0.255 | 0.940 | |$\!\!\!\!-$|0.009 | 0.114 | 0.127 | 0.958 | ||
0.3 | 6.411 | Naive | |$\!\!\!\!-$|0.032 | 0.283 | 0.263 | 0.968 | |$\!\!\!\!-$|0.040 | 0.119 | 0.132 | 0.956 | ||
0.5 | 6.186 | Naive | |$\!\!\!\!-$|0.053 | 0.271 | 0.270 | 0.942 | |$\!\!\!\!-$|0.013 | 0.142 | 0.134 | 0.964 | ||
0.7 | 6.017 | Naive | |$\!\!\!\!-$|0.035 | 0.264 | 0.274 | 0.938 | |$\!\!\!\!-$|0.011 | 0.135 | 0.136 | 0.974 | ||
Case 2 | ||||||||||||
0.1 | 6.720 | Naive | |$\!\!\!\!-$|0.036 | 0.262 | 0.255 | 0.940 | |$\!\!\!\!-$|0.011 | 0.125 | 0.127 | 0.958 | ||
0.3 | 6.411 | Naive | |$\!\!\!\!-$|0.026 | 0.257 | 0.265 | 0.952 | |$\!\!\!\!-$|0.015 | 0.121 | 0.131 | 0.952 | ||
0.5 | 6.186 | Naive | |$\!\!\!\!-$|0.022 | 0.255 | 0.271 | 0.942 | |$\!\!\!\!-$|0.008 | 0.126 | 0.134 | 0.954 | ||
0.7 | 6.017 | Naive | |$\!\!\!-$|0.019 | 0.261 | 0.274 | 0.940 | |$\!\!\!\!-$|0.011 | 0.121 | 0.136 | 0.962 | ||
Case 3 | ||||||||||||
0.1 | 6.818 | Naive | |$\!\!\!\!-$|0.023 | 0.251 | 0.253 | 0.958 | |$\!\!\!\!-$|0.001 | 0.121 | 0.126 | 0.940 | ||
0.3 | 6.629 | Naive | |$\!\!\!\!-$|0.023 | 0.243 | 0.258 | 0.958 | 0.000 | 0.135 | 0.129 | 0.942 | ||
0.5 | 6.476 | Naive | |$\!\!\!\!-$|0.016 | 0.258 | 0.262 | 0.954 | 0.003 | 0.131 | 0.131 | 0.954 | ||
0.7 | 6.347 | Naive | |$\!\!\!\!-$|0.018 | 0.274 | 0.267 | 0.938 | |$\!\!\!\!-$|0.005 | 0.136 | 0.132 | 0.948 |
EC, error corrected; B, empirical bias based on the median; MAD, empirical median absolute deviation; RMAD, resampling median absolute deviation; CP, empirical coverage probability of 95% confidence interval.
Under this setting, the average survival time for treatment |$A=1$| is significantly longer than |$A=0$| without adjusted for the biomarker value. Therefore, marker-independent treatment selection will assign all subjects to treatment 1. The corresponding mean restricted lifetime within 10 years is 3.984, which is much shorter than marker based estimates. The corresponding misassignment rate is 48.4%, which is much higher than marker based estimates.
We also consider a simulation setting when the true model is misspecified as (3.1). The simulation setting is the same as case 1 above except that the true model is |$\lambda (t|X,A)=\lambda _{0}(t)\exp (-2.5X-0.5X^{2}+0.2A+5.0XA+X^{2}A)$| with |$\lambda _{0}(t)=0.4.$| In this case, the treatment selection based on the working model (3.1) is not optimal overall. Based on the working models (3.1) and (3.7), we estimated the misassignment probabilities and the mean restricted lifetime using the new biomarker and the standard biomarker, respectively. The results for |$n=2000$| are shown in Tables S3 and S4 in the supplementary material available at Biostatistics online. For estimation of the overall misassignment rate, the estimate is 3.53% from the ideal approach. When the error variance increases from 0.1 to 0.7, the estimates from the error-corrected approach based on the new biomarker are close to that from the ideal approach, while the estimates based on the standard biomarker increase considerably from 10.21% to 22.53% for the naive approach with the error variance increases from 0.1 to 0.7. The optimal mean restricted lifetime based on the new marker is 6.79 under model (3.1), while the mean restricted lifetime based on the standard marker decreases from 6.59 to 5.92 when |$\Sigma $| increases from 0.1 to 0.7. The estimates of the mean restricted lifetime perform well.
5. Application
We applied the proposed approach to a subset of the ECOG clinical trial, which was analyzed in Li and Ryan (2006), where c-myc expression level was measured via dot plots on 92 patients randomized to receive surgery alone or surgery plus chemotherapy, both progression-free survival and overall survival were recorded. The results for overall survival are presented as follows while the results for progression-free survival are presented in the supplementary material available at Biostatistics online.
Based on the log-rank test, surgery plus chemotherapy is better than surgery alone for overall survival (p-value = 0.0186). Thus marker-independent treatment selection would assign all patients to surgery plus chemotherapy. Using this subset of data, Li and Ryan (2006) found marginally significant interaction between treatment |$A=I($|surgery plus chemotherapy|$)$| and log c-myc gene expression level |$X$| for progression-free survival (|$p$|-value |$ =0.073$|) and overall survival (|$p$|-value |$=0.112$|) under model (3.1 ), and Song and Zhou (2011) evaluated bisecting the observed c-myc gene expression level |$Z$| for treatment selection. It is conjectured that a more accurate measurement of c-myc gene expression level might improve its capacity for treatment selection. In this subset, 26 subjects have replicated c-myc gene expression measurements with the estimated measurement error standard deviation equal to |$0.20$|. The plot of the residuals |$Z_{ij}- \bar{Z}_{i}$| and the corresponding Q–Q plot indicate that it is reasonable to assume the error is normal with constant variance (Figure 2).
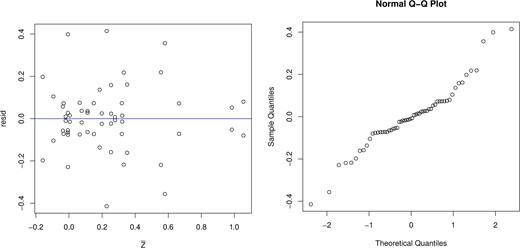
We checked the proportional hazards assumption in model (3.7 ) using the method in Therneau and Grambsch (2006, Chapter 6.2). To check the proportional hazards assumption in model (3.1), as |$X$| is not available, we adopted SIMEX approach to obtain the error-corrected p-values. There is no evidence of violation of the proportional hazards assumptions in both models.
We would like to assess the amount of gain of improving c-myc gene expression level measurement on treatment selection and to compare treatment selection based on the true value |$X$| and the error-contaminated observation. To reduce variation from SIMEX and bootstrap, we took larger values of |$R=5000$| in the resampling process and |$B=5000$| in the SIMEX process. Under the working proportional hazards model (3.1) and (3.7), the estimated coefficients and standard errors are shown in Table 3. The error-corrected estimates of log c-myc level and the interaction are larger in magnitude. Among the 92 subjects, 48 (47.8%) patients were assigned to surgery alone and 44 to surgery plus chemotherapy. An estimate of 87% patients would be assigned to surgery plus chemotherapy if the treatment is selected using |$Z$|, and 82% if the treatment is selected using |$X$|. This suggested that 10% patients might be assigned to the wrong treatment if model (3.1) is true and the treatment is selected using |$Z$|. The estimated mean restricted lifetime within 5 years is 3.85 if all patients are assigned to surgery alone, and 4.10 if all are assigned to surgery plus chemotherapy. The estimated mean restricted lifetime within 5 years is 4.40 when the treatment is selected using |$X$| while 4.13 when the treatment is selected using |$Z$|. There is no significant difference between the mean restricted life times (95% confidence interval |$-0.07$|, 0.61). With the consideration of the possible side effects of chemotherapy, the treatment selection based on the new marker seems to be better as less patients are assigned to surgery plus chemotherapy.
Estimation of Cox model coefficients . | ||||||||
---|---|---|---|---|---|---|---|---|
. | log(c-myc) . | . | TRT . | . | log(c-myc)|$\times $|TRT . | |||
. | Est (RMAD) . | . | Est (RMAD) . | . | Est (RMAD) . | |||
Model based on |$X$| | 0.715 (0.548) | |$-$|0.027 (0.488) | |$-$|1.355 (0.975) | |||||
Model based on |$Z$| | 0.582 (0.396) | |$-$|0.105 (0.428) | |$-$|1.126 (0.691) | |||||
Estimation of 5-year restricted lifetime | ||||||||
Est | CI | |||||||
All assigned to surgery alone | 3.85 | (3.41–4.29) | ||||||
All assigned to surgery|$+$|chemotherapy | 4.10 | (3.66–4.53) | ||||||
Treatment selection using |$X$| | 4.39 | (3.79–5.00) | ||||||
Treatment selection using |$Z$| | 4.13 | (3.71–4.55) |
Estimation of Cox model coefficients . | ||||||||
---|---|---|---|---|---|---|---|---|
. | log(c-myc) . | . | TRT . | . | log(c-myc)|$\times $|TRT . | |||
. | Est (RMAD) . | . | Est (RMAD) . | . | Est (RMAD) . | |||
Model based on |$X$| | 0.715 (0.548) | |$-$|0.027 (0.488) | |$-$|1.355 (0.975) | |||||
Model based on |$Z$| | 0.582 (0.396) | |$-$|0.105 (0.428) | |$-$|1.126 (0.691) | |||||
Estimation of 5-year restricted lifetime | ||||||||
Est | CI | |||||||
All assigned to surgery alone | 3.85 | (3.41–4.29) | ||||||
All assigned to surgery|$+$|chemotherapy | 4.10 | (3.66–4.53) | ||||||
Treatment selection using |$X$| | 4.39 | (3.79–5.00) | ||||||
Treatment selection using |$Z$| | 4.13 | (3.71–4.55) |
Est, estimate; RMAD, resampling median absolute deviation; CI, 95% confidence interval.
Estimation of Cox model coefficients . | ||||||||
---|---|---|---|---|---|---|---|---|
. | log(c-myc) . | . | TRT . | . | log(c-myc)|$\times $|TRT . | |||
. | Est (RMAD) . | . | Est (RMAD) . | . | Est (RMAD) . | |||
Model based on |$X$| | 0.715 (0.548) | |$-$|0.027 (0.488) | |$-$|1.355 (0.975) | |||||
Model based on |$Z$| | 0.582 (0.396) | |$-$|0.105 (0.428) | |$-$|1.126 (0.691) | |||||
Estimation of 5-year restricted lifetime | ||||||||
Est | CI | |||||||
All assigned to surgery alone | 3.85 | (3.41–4.29) | ||||||
All assigned to surgery|$+$|chemotherapy | 4.10 | (3.66–4.53) | ||||||
Treatment selection using |$X$| | 4.39 | (3.79–5.00) | ||||||
Treatment selection using |$Z$| | 4.13 | (3.71–4.55) |
Estimation of Cox model coefficients . | ||||||||
---|---|---|---|---|---|---|---|---|
. | log(c-myc) . | . | TRT . | . | log(c-myc)|$\times $|TRT . | |||
. | Est (RMAD) . | . | Est (RMAD) . | . | Est (RMAD) . | |||
Model based on |$X$| | 0.715 (0.548) | |$-$|0.027 (0.488) | |$-$|1.355 (0.975) | |||||
Model based on |$Z$| | 0.582 (0.396) | |$-$|0.105 (0.428) | |$-$|1.126 (0.691) | |||||
Estimation of 5-year restricted lifetime | ||||||||
Est | CI | |||||||
All assigned to surgery alone | 3.85 | (3.41–4.29) | ||||||
All assigned to surgery|$+$|chemotherapy | 4.10 | (3.66–4.53) | ||||||
Treatment selection using |$X$| | 4.39 | (3.79–5.00) | ||||||
Treatment selection using |$Z$| | 4.13 | (3.71–4.55) |
Est, estimate; RMAD, resampling median absolute deviation; CI, 95% confidence interval.
6. Discussion
We have proposed a novel method to evaluate a new biomarker based on data from a reproducibility study. Our approach assumes that the reproducibility study samples and the clinical trial samples are randomly drawn from a common target population. It is beyond the scope of this article to address the impact of violations of this assumption.
We have aimed on maximizing the population mean-restricted lifetime. This method can be extended to other statistical measures, for example, |$L$|-year survival rate, or a more flexible utility function that incorporates notions of cost and quality of life. Although we have focused on survival time as outcomes, the approach can be adapted to discrete and continuous outcomes with minor modifications.
For simplicity, we have adopted proportional hazards models as working models. The estimation of mean restricted lifetime under the treatment selection criterion based on the working model is still valid even if the model is misspecified. Our approaches may also be extended to more flexible models, for example, by allowing time-varying treatment or covariate effects, or including nonparametric functions of covariates in the survival model. Other types of survival models, such as the accelerated failure time model or the additive hazards model, may also be used. Such extensions may warrant further investigation.
In addition, we have assumed a classical measurement error model between the new marker and the standard marker. An more flexible model such as (2.3) with |$g_{1}$| and |$g_{2}$| unknown may be adopted and could be estimated using data from the reproducibility study with validation data based on parametric models or spline approximation. In some situations, both markers might involve measurement error while the measurement error for the new marker might be smaller. It would be of interest to extend our approaches to accommodate such complexity.
The model of equation (2.2) assumes that the new assay reduces error compared to the existing assay. This is motivated by the fact that often newer technologies provide better measurements than existing technologies. However, the new technologies are often more expensive as well. There is a cost–benefit tradeoff for many new assays. Our modeling approach can help in the quantification of how large the potential benefit might be from a new assay that is under consideration. This can be used to make the “go” versus “no go” decision on whether or not to switch to the new marker in the future. In other words, by quantifying the benefit, our method gives the information needed to make this decision. Another application of our approach is to the setting where a gold standard treatment-selection biomarker exists along with an approximation to the biomarker. The approximation may be based for example on pathological evaluations, like the Magee score as an approximation to the OncotypeDX score (Farrugia and others, 2017). Our approach provides a framework in which to understand the relationship between two such scores as predictors of a clinically important survival outcome.
7. Software
The R code and a sample data set are available on GitHub (https://github.com/xsong88/Evaluate-Biomarkers).
Supplementary material
Supplementary material is available online at http://biostatistics.oxfordjournals.org.
Acknowledgments
Conflict of Interest: None declared.
Funding
National Institutes of Health (CA201207 to X.S. and K.K.D.; R43GM134768 to X.S.); and NSF (DMS-1916411 to X.S.).