-
PDF
- Split View
-
Views
-
Cite
Cite
Christopher N Kaufmann, Chen Bai, Brianne Borgia, Christiaan Leeuwenburgh, Yi Lin, Mamoun T Mardini, Taylor McElroy, Clayton W Swanson, Keon D Wimberly, Ruben Zapata, Rola S Zeidan, Todd M Manini, ChatGPT’s Role in Gerontology Research, The Journals of Gerontology: Series A, Volume 79, Issue 9, September 2024, glae184, https://doi.org/10.1093/gerona/glae184
- Share Icon Share
Abstract
Chat Generative Pre-trained Transformer (ChatGPT) and other ChatBots have emerged as tools for interacting with information in manners resembling natural human speech. Consequently, the technology is used across various disciplines, including business, education, and even in biomedical sciences. There is a need to better understand how ChatGPT can be used to advance gerontology research. Therefore, we evaluated ChatGPT responses to questions on specific topics in gerontology research, and brainstormed recommendations for its use in the field.
We conducted semistructured brainstorming sessions to identify uses of ChatGPT in gerontology research. We divided a team of multidisciplinary researchers into 4 topical groups: (a) gero-clinical science, (b) basic geroscience, (c) informatics as it relates to electronic health records, and (d) gero-technology. Each group prompted ChatGPT on a theory-, methods-, and interpretation-based question and rated responses for accuracy and completeness based on standardized scales.
ChatGPT responses were rated by all groups as generally accurate. However, the completeness of responses was rated lower, except by members of the informatics group, who rated responses as highly comprehensive.
ChatGPT accurately depicts some major concepts in gerontological research. However, researchers have an important role in critically appraising the completeness of its responses. Having a single generalized resource like ChatGPT may help summarize the preponderance of evidence in the field to identify gaps in knowledge and promote cross-disciplinary collaboration.
OpenAI’s Chat Generative Pre-trained Transformer (ChatGPT) and other artificial intelligence “ChatBots” have emerged as tools with tremendous potential to transform how society consumes information. These ChatBots enable individuals to interact with information on the internet in a manner resembling natural human speech, facilitating new and diverse methods of accessing information never before seen in modern society. Although some critics of the technology warn of the ethical implications and the potential for disseminating misinformation, there is overall immense excitement about how these new tools can contribute to various disciplines, including business, education, and technology development.
Recently, there has been increased attention on the burgeoning applications of ChatGPT in health sciences, with studies demonstrating ways the technology can assist in patient care and medical research. Cascella et al. (1) investigated the manifold possibility of utilization of ChatGPT in healthcare and found that ChatGPT holds significant promise in advancing science and enhancing scientific literacy by bolstering various research facets. Moreover, there have been several applications for distinct medical specialties, such as genetics and oncology. For example, Johnson et al. (2) evaluated the reliability of cancer-related information identified by ChatGPT and found that it can provide accurate information about common cancer myths and misconceptions, thus preventing the dissemination of incorrect information. In a study comparing ChatGPT and respondents on human genetics questions, Duong et al. (3) found that the performance between the 2 was largely equivalent. Finally, Ayers et al. (4) assessed the responses from both physicians and ChatGPT to medical questions posted on a public social media forum, revealing that ChatGPT’s responses were consistently higher in quality and more empathetic compared to those provided by physicians.
Although past studies have evaluated ChatGPT uses in medical practice and research, surprisingly little attention has been paid to how ChatGPT can be used specifically in gerontological research. The field of gerontology is multidisciplinary, with research aimed at studying clinical, basic/mechanistic science, informatics, and healthcare technology perspectives, amongst others. As such, ChatGPT may be useful for generating gerontology-related research questions, brainstorming approaches for common scientific issues in studying aging populations, and identifying methods for exploring specific research questions. Therefore, it is necessary to identify the ways and appropriateness of approaches to using ChatGPT in gerontological research.
To preliminarily evaluate the utility of ChatGPT for research in gerontology, we conducted a structured brainstorming session to accomplish 2 main goals. First, we sought to explore the value of using ChatGPT in specific gerontological research domains. Second, we examined (a) the accuracy and (b) the completeness of ChatGPT responses to agreed-upon domain-specific scientific questions. Lastly, our team reflected on ways researchers in the gerontology field can use ChatGPT ethically and effectively.
Method
We created a working group with members of the Institute on Aging at the University of Florida. The working group comprised researchers in diverse fields at all levels of training. The team consisted of 5 faculty members (Drs. Kaufmann, Leeuwenburgh, Manini, Mardini, and Swanson), 5 postdoctoral fellows (Drs. Borgia, Lin, McElroy, Wimberly, and Zeidan), and 2 doctoral students (Mr. Bai and Mr. Zapata). The expertise and/or training background of evaluators included genetics, biological and physiological sciences, clinical research, population epidemiology, and informatics. In the first session, members set an a priori step-by-step action plan that is illustrated in Figure 1. Brainstorming sessions occurred over a 3-month period consisting of six 1-hour-long sessions.
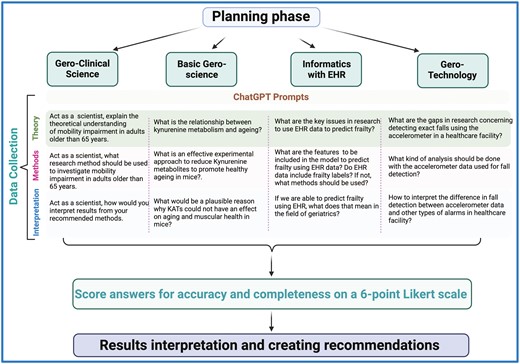
Four groups were self-formed based on individual research interests of members. Groups were self-identified as (a) gero-clinical science (eg, topics evaluated in clinical trials, intervention, and observational cohort studies), (b) basic geroscience (eg, mechanistic research using wet lab methodology), (c) informatics as it relates to EHRs (eg, analyses using healthcare big data and other innovative data sources), and (d) gero-technology (eg, use of mHealth and wearables). Each group created 3 questions to ask ChatGPT under the categories of content, methodology, and interpretation. Between sessions, teams entered each of their 3 questions individually into ChatGPT, and evaluated responses. Because of our focus on evaluating the accuracy and completeness of ChatGPT responses, each team rated responses for accuracy and completeness on a 6-point Likert scale. For the purposes of this exercise, we defined accuracy as “The quality of being correct or precise regardless of the details provided” (response options include 1 = “Completely Incorrect,” 2 = “Mostly Incorrect,” 3 = “Somewhat Incorrect,” 4 = “Somewhat Correct,” 5 = “Mostly Correct,” 6 = “Completely Correct”). Raters were instructed to disregard vagueness in their accuracy score. Raters were also asked to score completeness, defined as “The state or condition of having all the necessary or appropriate parts” (response options include 1 = ”Extremely Incomplete,” 2 = ”Mostly Incomplete,” 3 = ”Somewhat Incomplete,” 4 = ”Somewhat Comprehensive,” 5 = ”Mostly Comprehensive,” 6 = ”Extremely Comprehensive”) (Table 1). Please note that although ChatGPT can provide new responses each time the same prompt is entered, we chose to only evaluate the first response. We acknowledge that accuracy and completeness may differ with rephrasing of prompts. However, we chose to focus solely on the first response because it represents the most common use case of ChatGPT. Finally, we reconvened as a group to finalize ratings and develop recommendations about the use of ChatGPT. Please see Supplementary Material for a transcript of ChatGPT responses evaluated.
Domain-Specific Theory, Methods, and Interpretation Questions and Group Ratings on Response Accuracy and Completeness
Topics . | Question Type . | Questions . | Accuracy (Range: 1–6) . | Completeness (Range: 1–6) . |
---|---|---|---|---|
Gero-clinical science | Theory | Act as a role of scientist, explain the theoretical understanding of mobility impairment in adults older than 65 years. | 6 | 3 (average of 4, 4, 1) |
Methods | Act as a scientist, what research method should be used to investigate mobility impairment in adults older than 65 years. | 6 | 4 | |
Interpretation | Act as a scientist, how would you interpret results from your recommended methods. | 6 | 1 | |
Average: | 6 | 2.7 | ||
Basic geroscience | Theory | What is the relationship between kynurenine metabolism and aging? | 6 | 4 |
Methods | What is an effective experimental approach to reduce kynurenine metabolites to promote healthy aging in mice? | 4 | 4 | |
Interpretation | What would be a plausible reason why KATs could not have an effect on aging and muscular health in mice? | 5 | 4 | |
Average: | 5 | 4 | ||
Informatics with electronic health records | Theory | What are the key issues in research to use EHR data to predict frailty? | 6 | 6 |
Methods | What are the features we want to be included in the model to predict frailty using EHR data? Do EHR data include frailty labels? When there is no frailty label in EHR data, what methods should be used? | 6 | 6 | |
Interpretation | If we are able to predict frailty using EHR, what does that mean in the field of geriatrics? | 6 | 4 | |
Average: | 6 | 5.3 | ||
Gero-technology | Theory | What are the gaps in research concerning detecting exact falls using the accelerometer in a healthcare facility? | 4 | 4 |
Methods | What kind of analysis should be done with the accelerometer data used for fall detection? | 3 | 1 | |
Interpretation | How to interpret the difference in fall detection between accelerometer data and other types of alarms in healthcare facility? | 4 | 1 | |
Average: | 3.7 | 2 |
Topics . | Question Type . | Questions . | Accuracy (Range: 1–6) . | Completeness (Range: 1–6) . |
---|---|---|---|---|
Gero-clinical science | Theory | Act as a role of scientist, explain the theoretical understanding of mobility impairment in adults older than 65 years. | 6 | 3 (average of 4, 4, 1) |
Methods | Act as a scientist, what research method should be used to investigate mobility impairment in adults older than 65 years. | 6 | 4 | |
Interpretation | Act as a scientist, how would you interpret results from your recommended methods. | 6 | 1 | |
Average: | 6 | 2.7 | ||
Basic geroscience | Theory | What is the relationship between kynurenine metabolism and aging? | 6 | 4 |
Methods | What is an effective experimental approach to reduce kynurenine metabolites to promote healthy aging in mice? | 4 | 4 | |
Interpretation | What would be a plausible reason why KATs could not have an effect on aging and muscular health in mice? | 5 | 4 | |
Average: | 5 | 4 | ||
Informatics with electronic health records | Theory | What are the key issues in research to use EHR data to predict frailty? | 6 | 6 |
Methods | What are the features we want to be included in the model to predict frailty using EHR data? Do EHR data include frailty labels? When there is no frailty label in EHR data, what methods should be used? | 6 | 6 | |
Interpretation | If we are able to predict frailty using EHR, what does that mean in the field of geriatrics? | 6 | 4 | |
Average: | 6 | 5.3 | ||
Gero-technology | Theory | What are the gaps in research concerning detecting exact falls using the accelerometer in a healthcare facility? | 4 | 4 |
Methods | What kind of analysis should be done with the accelerometer data used for fall detection? | 3 | 1 | |
Interpretation | How to interpret the difference in fall detection between accelerometer data and other types of alarms in healthcare facility? | 4 | 1 | |
Average: | 3.7 | 2 |
Notes: Accuracy ratings range from 1 (“Mostly Incorrect”) to 6 (“Mostly Correct”). Completeness ratings range from 1 (“Extremely Incomplete”) to 6 (“Extremely Comprehensive”). Both scores are based on group consensus. EHR = electronic health record; KAT = kynurenine aminotransferase.
Domain-Specific Theory, Methods, and Interpretation Questions and Group Ratings on Response Accuracy and Completeness
Topics . | Question Type . | Questions . | Accuracy (Range: 1–6) . | Completeness (Range: 1–6) . |
---|---|---|---|---|
Gero-clinical science | Theory | Act as a role of scientist, explain the theoretical understanding of mobility impairment in adults older than 65 years. | 6 | 3 (average of 4, 4, 1) |
Methods | Act as a scientist, what research method should be used to investigate mobility impairment in adults older than 65 years. | 6 | 4 | |
Interpretation | Act as a scientist, how would you interpret results from your recommended methods. | 6 | 1 | |
Average: | 6 | 2.7 | ||
Basic geroscience | Theory | What is the relationship between kynurenine metabolism and aging? | 6 | 4 |
Methods | What is an effective experimental approach to reduce kynurenine metabolites to promote healthy aging in mice? | 4 | 4 | |
Interpretation | What would be a plausible reason why KATs could not have an effect on aging and muscular health in mice? | 5 | 4 | |
Average: | 5 | 4 | ||
Informatics with electronic health records | Theory | What are the key issues in research to use EHR data to predict frailty? | 6 | 6 |
Methods | What are the features we want to be included in the model to predict frailty using EHR data? Do EHR data include frailty labels? When there is no frailty label in EHR data, what methods should be used? | 6 | 6 | |
Interpretation | If we are able to predict frailty using EHR, what does that mean in the field of geriatrics? | 6 | 4 | |
Average: | 6 | 5.3 | ||
Gero-technology | Theory | What are the gaps in research concerning detecting exact falls using the accelerometer in a healthcare facility? | 4 | 4 |
Methods | What kind of analysis should be done with the accelerometer data used for fall detection? | 3 | 1 | |
Interpretation | How to interpret the difference in fall detection between accelerometer data and other types of alarms in healthcare facility? | 4 | 1 | |
Average: | 3.7 | 2 |
Topics . | Question Type . | Questions . | Accuracy (Range: 1–6) . | Completeness (Range: 1–6) . |
---|---|---|---|---|
Gero-clinical science | Theory | Act as a role of scientist, explain the theoretical understanding of mobility impairment in adults older than 65 years. | 6 | 3 (average of 4, 4, 1) |
Methods | Act as a scientist, what research method should be used to investigate mobility impairment in adults older than 65 years. | 6 | 4 | |
Interpretation | Act as a scientist, how would you interpret results from your recommended methods. | 6 | 1 | |
Average: | 6 | 2.7 | ||
Basic geroscience | Theory | What is the relationship between kynurenine metabolism and aging? | 6 | 4 |
Methods | What is an effective experimental approach to reduce kynurenine metabolites to promote healthy aging in mice? | 4 | 4 | |
Interpretation | What would be a plausible reason why KATs could not have an effect on aging and muscular health in mice? | 5 | 4 | |
Average: | 5 | 4 | ||
Informatics with electronic health records | Theory | What are the key issues in research to use EHR data to predict frailty? | 6 | 6 |
Methods | What are the features we want to be included in the model to predict frailty using EHR data? Do EHR data include frailty labels? When there is no frailty label in EHR data, what methods should be used? | 6 | 6 | |
Interpretation | If we are able to predict frailty using EHR, what does that mean in the field of geriatrics? | 6 | 4 | |
Average: | 6 | 5.3 | ||
Gero-technology | Theory | What are the gaps in research concerning detecting exact falls using the accelerometer in a healthcare facility? | 4 | 4 |
Methods | What kind of analysis should be done with the accelerometer data used for fall detection? | 3 | 1 | |
Interpretation | How to interpret the difference in fall detection between accelerometer data and other types of alarms in healthcare facility? | 4 | 1 | |
Average: | 3.7 | 2 |
Notes: Accuracy ratings range from 1 (“Mostly Incorrect”) to 6 (“Mostly Correct”). Completeness ratings range from 1 (“Extremely Incomplete”) to 6 (“Extremely Comprehensive”). Both scores are based on group consensus. EHR = electronic health record; KAT = kynurenine aminotransferase.
Results
Accuracy
Table 1 summarizes ratings by theory-, methods-, and interpretation-based questions. Three of the 4 topic groups reported responses as highly accurate for all 3 question types (average of 5–6), with the gero-technology group giving moderate ratings with an average of 3.7. Likely due to the fact that responses were based on text collected over the internet and parsed using large language models, the responses were consistent with the generalized knowledge of the respective fields. However, a key issue among all groups was the inability to verify assertions made by ChatGPT, specifically within ChatGPT itself—when asked for citations to evaluate further information, ChatGPT “hallucinated” (eg, made up) citations. Furthermore, as the content used to generate responses was capped in 2021 at the time of our search, new findings in our field were not included in the output for various questions, which limited the accuracy of responses, especially with emerging research topics. Given the rapidly developing field for the gero-technology group, members of this group noted this as a significant reason for lower accuracy ratings.
Completeness
Groups rated completeness variably. For example, the informatics group scored responses to all questions as very complete, with an average score of 5.3. It is possible that because ChatGPT is more commonly used in engineering and data science fields, the large language models had more data to generate responses. For the groups in fields with broader generalized knowledge (eg, basic-geroscience, gero-clinical science, etc.), responses were less complete—perhaps due to the requirement for synthesizing a broader range of content specific to that field. For example, the gero-clinical science group reported the response to the theory-based question lacked the depth of detail needed for researchers aiming to have mechanistic insights.
It should be noted that the research methods response was deemed less complete by most groups. Specifically, groups reported that there were specifics of methods that were needed, including limitations and challenges of different approaches, a more extensive overview of data collection procedures and analysis, and the consideration of sample size and sampling techniques. Groups reported that responses helped identify specific methods, but more in-depth exploration was needed to apply such methods in their own research.
Finally, among all groups, the interpretation response was scored the lowest. ChatGPT acknowledged important factors for interpreting results, but significant parts were thought to be missing. These included delving deeper into confounding variables, effect sizes and clinical relevance, potential biases, and seamlessly merging quantitative and qualitative discoveries. The healthcare technology group scored completeness lower due to the requirement for follow-up prompts to obtain detailed information to be considered a complete answer.
Reflections on ChatGPT’s Use for Gerontology Research
As a whole, the experience with ChatGPT was positive. The working group members felt that the platform could be a phenomenal tool for brainstorming, idea generation, assistance with organizing thoughts, translating domain-specific technical language for communicating with those outside our sub-disciplines, and collating foundational information that is relatively accurate.
Our study should be interpreted in the context of its limitations. First, although this was a structured brainstorming exercise, it still was qualitative and therefore “accuracy” and “comprehensiveness” should be interpreted as such. However, we feel that the general insights gained from these ratings helped us make appropriate conclusions of ChatGPT’s use in gerontological research. Second, our conclusions are specific to the expertise of study group members, and given gerontology is a broad field, our exercise needs to be replicated in other disciplines as appropriate. Third, generative AI technology is rapidly evolving, and new features are added daily, making our results provisional.
Despite the limitations of our study, our group identified several recommendations for use of the technology in our field. First, we strongly urge researchers to obtain outside help from experts in respective fields to augment and contextualize ChatGPT’s completeness. Largely, our groups rated the responses from ChatGPT quite accurately; however, apart from questions from the informatics group (who rated most responses as comprehensive), the other topic groups reported missing key information from ChatGPT’s responses. It is worth noting that ChatGPT is evolving quickly—today while it is popularized by some media outlets, it is primarily being used by early adopters and those in the technology fields, and use cases for the tool are expanding daily. We also note that additional follow-up questions based on the initial response could increase the depth of responses. In a single-question framework as employed here, research-based users are encouraged to seek additional resources through traditional means (eg, synthesis of the literature, meta-analyses, and one-to-one interactions with experts) to obtain thorough answers.
Second, using ChatGPT stimulated our diverse group to communicate across disciplines. Thus, it might be used to build collaborations. Members of the working group enjoyed learning about diverse research opportunities unique to each domain. Furthermore, they were enthusiastic about bridging research areas, content, and methodologies and developed an application for translational research. For example, in discussions, the basic geroscience group reported that ChatGPT responses from both the informatics and gero-technology groups helped them apply those domains to their own topic area. It also helped them generate new or revised research questions that could bridge research methodologies across the disciplines.
Third, ChatGPT can aid researchers in conveying complex information to those outside our respective fields. ChatGPT could be a “translator” that promotes cross-field research. For example, opportunities for collaboration may include scholars in the liberal arts (such as history, literature, and philosophy), or workers in community organizations and local senior centers. In our view, these opportunities can make our gerontology research more applicable and relevant to broader audiences.
Fourth, we strongly urge that we educate the gerontology field in the burgeoning area of “prompt engineering.” Prompt engineering is the process of designing and refining prompts (ie, questions) that are used to generate responses from artificial intelligence systems like ChatGPT (5,6). In the field of gerontology, it is crucial for researchers to carefully consider the questions posed to ChatGPT to ensure accurate and complete responses. Specifically, we propose that researchers provide ChatGPT with key contextual information. For example, the researcher could specify the role that ChatGPT should play in responding; for example, “Act as if you are a research scientist studying age-related mobility decline.” Additionally, we urge researchers to provide additional background information or follow-up questions, to improve response accuracy and depth. Finally, we suggest users of ChatGPT clearly outline what the response should look like and what information would be useful in responses (eg, “Provide 3 examples of instruments for mobility assessment, and list the strength and limitations of each”). Indeed, several organizations have identified key best practices in prompt generation that are referenceable (7–9). Of note, OpenAI itself offers key recommendations including, among others, using the latest LLM model and reducing imprecise language (7). As the technology advances and becomes more common in use, it will be important to establish best practices across all industries including those for gerontology researchers.
Finally, we wish to offer a warning when using ChatGPT to identify references to published manuscripts. Our searches provided citations that, in some cases, were not even real. This is a serious issue that needs to be considered when using ChatGPT, and we strongly urge gerontology researchers to use the technology for general knowledge rather than specific examples of scientific content. Recent advancements to the technology have begun to address this issue. For example, OpenAI released a feature called PlugIns that allows ChatGPT to interface with services outside of the platform, which can facilitate searching scientific literature on, for example, PubMed. However, as of this writing, this functionality is only available for paid users and, therefore, is unavailable to those without a subscription.
Conclusion
This study evaluated the ways ChatGPT can be used in gerontology research and identified strategies for which members in the field can effectively use it. Overall, we strongly advocate exploring various uses of ChatGPT as fruitful discussions are clearly generated from the efforts. However, it is important to use our collective “brain power” and traditional resources to appraise responses and content generated in a critical manner. Importantly, ChatGPT-like technology is rapidly evolving, and the limitations and strengths of the tool are expanding daily. We suggest continued experimentation with platforms such as ChatGPT in the gerontology field to further elucidate potential benefits and limitations for gerontology research.
Funding
This work was supported by the National Institute on Aging (Grant #’s: T32AG062728, P30AG028740, K01AG061239, and R01AG079391). C.W.S. was also supported by a Career Development Award-1 #RX003954-01A1 from the United States (U.S.) Department of Veterans Affairs, Rehabilitation Research and Development Service. C.N.K. also received funding from the Sleep Research Society Foundation (Grant #: 23-FRA-001).
Conflict of Interest
None.
Acknowledgments
Resources were provided by the North Florida/South Georgia Veterans Health System and the VA Brain Rehabilitation Research Center. The contents of this article do not represent the views of the U.S. Department of Veterans Affairs or the United States Government.