-
PDF
- Split View
-
Views
-
Cite
Cite
Diego Orcioli-Silva, Rodrigo Vitório, Victor Spiandor Beretta, Núbia Ribeiro da Conceição, Priscila Nóbrega-Sousa, Anderson Souza Oliveira, Lilian Teresa Bucken Gobbi, Is Cortical Activation During Walking Different Between Parkinson’s Disease Motor Subtypes?, The Journals of Gerontology: Series A, Volume 76, Issue 4, April 2021, Pages 561–567, https://doi.org/10.1093/gerona/glaa174
- Share Icon Share
Abstract
Parkinson’s disease (PD) is often classified into tremor dominant (TD) and postural instability gait disorder (PIGD) subtypes. Degeneration of subcortical/cortical pathways is different between PD subtypes, which leads to differences in motor behavior. However, the influence of PD subtype on cortical activity during walking remains poorly understood. Therefore, we aimed to investigate the influence of PD motor subtypes on cortical activity during unobstructed walking and obstacle avoidance. Seventeen PIGD and 19 TD patients performed unobstructed walking and obstacle avoidance conditions. Brain activity was measured using a mobile functional near-infrared spectroscopy–electroencephalography (EEG) systems, and gait parameters were analyzed using an electronic carpet. Concentrations of oxygenated hemoglobin (HbO2) of the prefrontal cortex (PFC) and EEG absolute power from alpha, beta, and gamma bands in FCz, Cz, CPz, and Oz channels were calculated. These EEG channels correspond to supplementary motor area, primary motor cortex, posterior parietal cortex, and visual cortex, respectively. Postural instability gait disorder patients presented higher PFC activity than TD patients, regardless of the walking condition. Tremor dominant patients presented reduced beta power in the Cz channel during obstacle avoidance compared to unobstructed walking. Both TD and PIGD patients decreased alpha and beta power in the FCz and CPz channels. In conclusion, PIGD patients need to recruit additional cognitive resources from the PFC for walking. Both TD and PIGD patients presented changes in the activation of brain areas related to motor/sensorimotor areas in order to maintain balance control during obstacle avoidance, being that TD patients presented further changes in the motor area (Cz channel) to avoid obstacles.
Parkinson’s disease (PD) is a heterogeneous neurodegenerative disease with distinct pathophysiological and clinical characteristics (1). Patients with PD can be classified into 2 subtypes according to clinical observation of motor symptoms: tremor dominant (TD) and postural instability gait disorder (PIGD) (1). Tremor dominant demonstrates slow disease progression and early onset of symptoms, being predominantly characterized by the presence of tremor (2,3). In contrast, PIGD presents bradykinesia, rigidity, postural instability, a higher risk of falling, marked cognitive decline, rapid disease progression, and late-onset symptoms (2,3). Patients with PIGD present distinct gait kinematics when compared to TD patients, such as shorter step length, slower gait velocity, and greater gait variability (4–6). In addition, PIGD patients underperform during obstacle avoidance, presenting slower velocity to overcome obstacles and shorter horizontal and vertical distance from foot to obstacle (4), which may increase the risk of falls. Therefore, it is evident that patients with PD present symptomatic and behavioral differences that vary according to PD subtype.
Previous studies suggested that the degeneration of subcortical/cortical pathways and structures is different between PD subtypes (7–10). Postural instability gait disorder is associated with increased gray matter atrophy in several cortical areas, including the prefrontal cortex (PFC), supplementary motor area (SMA), and primary motor cortex (M1), evoking abnormal neuronal activities in these areas (7,8). Postural instability gait disorder has also been associated with hypo-connectivity between striatum, thalamus, motor, and fronto-parietal areas (8,9). In addition, PIGD patients present hypoactivity in the cerebellum, hypo-connectivity between the putamen and the SMA, and hyper-connectivity between the pedunculopontine nucleus and cortical motor areas (10). These dysfunctions in communication between the subcortical and cortical regions have been related to greater gait impairment and freezing of walking in PIGD patients (7). The TD subtype has been associated with decreased cerebellar gray matter, the cerebello–thalamo–cortical circuit, and basal ganglia dysfunction (7,8,10). Tremor dominant patients show hyperactivity in the cerebellum, which might be a compensatory effect to overcome defective basal ganglia (10). In addition, these patients present hyper-connectivity in motor, somatosensory, and visual areas that have been associated with compensation for decreased movement automaticity (7,10). Therefore, alterations in subcortical and cortical levels and differences in neural circuits allow us to speculate that, during walking, patients with PD present differences in cortical activity according to PD subtype. However, the influence of PD subtypes on cortical activity during walking remains poorly understood.
Combined functional near-infrared spectroscopy (fNIRS) and electroencephalography (EEG) are useful and reliable tools for monitoring cortical activity during walking in an ecological environment (11). Studies assessing brain activation in patients with PD while walking demonstrated increased PFC activation, which has been associated with compensatory mechanisms to control locomotion (12–14). This increase is higher when navigating in environments with obstacles (13). Moreover, previous studies have reported greater involvement of the sensorimotor cortex, expressed as reduced alpha and beta band spectral power, to control locomotion when walking stability is required (15–19), such as during obstacle avoidance. Despite the strong scientific evidence regarding the role of the PFC in gait modulation in patients with PD, there is very limited information regarding brain activity across cortical areas such as the SMA, M1, and parietal cortex (20). Moreover, studies assessing cortical activity during walking have not considered PD subtypes. Due to the heterogeneity of symptoms and walking kinematics, it is likely that patients with PD present distinct cortical activity depending on the PD subtype.
Therefore, using mobile fNIRS and EEG systems, this study aimed to investigate the influence of PD motor subtypes on cortical activity during unobstructed walking and obstacle avoidance. Due to the greater gait impairment and reduced automaticity of PIGD patients (4–6), it was expected that these patients would present higher PFC activation, demonstrating recruitment of additional cognitive resources, as a compensatory mechanism, to maintain gait performance (13,21,22). It was also expected that both TD and PIGD patients would present increased PFC activity during obstacle avoidance compared to unobstructed walking. In addition, we hypothesized that patients would reduce the alpha and beta band spectral power in motor and sensory areas regardless of the PD subtype during obstacle avoidance, as these bands are involved in the control of stability and gait adaptation (15,16). Overall, patients with PD present slowing cortical activity compared to healthy controls, including a widespread increase of power in the alpha band as well as a decrease of beta and gamma power (23). This can be exacerbated in PIGD patients that have hypoactivity in cortical and subcortical areas (10). Taken together, we also hypothesized that PIGD patients would present higher spectral power at low EEG frequency (alpha band: 9–13 Hz) and lower spectral power at high EEG frequency (beta band: 14–30 Hz; and gamma band: 31–50 Hz) than TD patients. Finally, based on previous studies (4–6), it was expected that PIGD patients would present shorter step length, slower step velocity, and higher gait variability than TD patients.
Method
Participants
Forty patients with PD were recruited from the database of the Posture and Gait Studies Laboratory (LEPLO, UNESP Rio Claro) and from geriatric/neurology clinics of the local community. Patients with PD were classified as PIGD (n = 17), TD (n = 19), or indeterminate (n = 4) based on sub-scores of the Movement Disorders Society United Parkinson’s Disease Rating Scale (MDS-UPDRS) parts II and III (24). The ratio of the average TD score (11 items) and the average PIGD score (5 items) was assessed. Patients with a ratio >1.15 were classified as TD and with a ratio <0.9 as PIGD. Additionally, patients with a positive mean in the numerator and zero in the denominator were classified as TD, while patients with a zero in the numerator and a positive mean in the denominator were classified as PIGD (24). If the scores were in between these values, they were considered as indeterminate and excluded from further analyses. Patients were included if diagnosed with PD according to the UK Brain Bank criteria. Exclusion criteria were cognitive impairment (Mini-Mental State Examination [MMSE] score <24), neurological disorders (other than PD), and musculoskeletal, orthopedic, and/or visual impairments that could affect performance. Patients were tested during the “ON state” of PD medication. This study was approved by the local research ethics committee. All patients provided written informed consent to participate in this study.
Clinical and Cognitive Evaluation
Motor severity was evaluated using the motor section (part III) of the MDS-UPDRS (25). The levodopa equivalent daily dose was calculated according to established methods (26). The MMSE was used to assess global cognitive function (27). The Trail Making Test (TMT) parts A and B were administered for screening speed of processing, mental flexibility, and executive function (28). The time difference between completing TMT-B and TMT-A (TMT B-A) was considered for analysis.
Protocol
Gait and cortical activation were assessed during 2 conditions (at self-selected speed): (i) unobstructed walking and (ii) obstacle avoidance. Four foam obstacles (60 cm width × 5 cm depth × 15 cm height) were evenly spaced along the walking path during the obstacle conditions. Each condition was performed 5 times. All 10 trials were fully randomized for each participant. Each trial started with the instruction to stand quietly, looking straight ahead, and thinking about nothing for 30 seconds. Next, a “start” instruction was given and the participants were required to walk around a 26.8-m circuit for 30 seconds. All participants completed the lap in the imparted time at least once.
Data Analysis
Functional near-infrared spectroscopy analysis
Changes in oxygenated hemoglobin (HbO2) concentration in the PFC were measured with a wireless fNIRS system (OctaMon, Artinis Medical Systems, The Netherlands), sampled at 10 Hz. The fNIRS sensors (8 channels) were placed over the forehead of the patients, following the international 10–20 EEG system. This location roughly targets left and right Brodmann’s areas 9 and 10, which represent the dorsolateral and anterior PFC. Oxysoft 3.0.52 software (Artinis Medical Systems) was used for data collection.
Data processing for fNIRS followed previous recommendations (20,29). On NIRS-SPM open-source toolbox for Matlab (The Mathworks Inc., Natick, MA) (30), filtering of the fNIRS signal was conducted using a low-pass filter (0.14 Hz) based on canonical hemodynamic response function (to reduce the high-frequency noise) (30,31) and a wavelet filter (to remove motion artifacts) (32–34). Next, using a customized script in Matlab, HbO2 concentration signals were averaged per region of interest (left and right PFC) and normalized by dividing them by the corresponding signal amplitude relative to the whole experiment within-participant (14,35). Each of the 5 trials was divided into 2 phases: (i) a “baseline” period, 15 seconds before the beginning of the walking instructions (the 5 seconds immediately before the instruction was excluded) and (ii) experimental task, period walking from 5 to 25 seconds after the instruction “start” (14). Baseline HbO2 concentration was subtracted from the experimental task to evaluate the relative change in HbO2 concentration in each experimental condition (13,14,36,37).
Electroencephalography analysis
Electroencephalography data were recorded using a wireless Mobita 32-channel system (TMSi, The Netherlands). The sampling rate was set to 500 Hz. This EEG system is a gel-based system with passive electrodes. All processing and analysis were performed in Matlab using an open-source toolbox for processing EEG data (EEGLAB 13.0.1b) (38). The EEG recordings were synchronized to the accelerometer data, which was coupled to the Mobita system and attached to the lower back (L5) of the participant to record vertical acceleration. We used the acceleration data to estimate the initial and final contact events within the gait cycle, following the previous recommendations (39). Both right and left heel strike were identified, as well as the crossing steps (in obstacle avoidance condition). Electroencephalography data were segmented into gait cycles (epochs). Subsequently, individual EEG datasets from both conditions were merged into a single dataset, which was band-pass filtered (1–100 Hz) and line noise removal (60 Hz). Substantial artifacts throughout the recordings were removed using the following methods: (i) channels with magnitude <30 or >2000 µV; (ii) channels with kurtosis >5 SDs from the mean; and (iii) channels with standard deviation substantially greater compared to neighboring channels (17,40). After rejecting channels, independent component analysis (RUNICA, from EEGLAB) was performed on the dataset to identify and remove eye blinks and artifacts related to muscle activity (41,42). In addition, epochs with visible motion artifacts were removed by visual inspection.
Four steps were selected before each of obstacle crossing performed during the 30 seconds, and a matching number of epochs from the unobstructed walking condition was randomly created to compare to the obstacle condition (24.97 ± 6.22 epochs). Due to the limited number of electrodes (32 channels), we analyzed the power spectrum of brain areas closest to the electrode locations following the 10–20 EEG system. A subset of 4 channels across all patients was selected (FCz, Cz, CPz, and Oz). These channels were chosen due to the information captured from the brain regions which are related to motor planning (SMA), motor execution (M1), sensorimotor processing (posterior parietal cortex), and visual information (visual cortex). The power spectrum from the remaining EEG channels was computed across the time series representing the 4 steps prior to obstacle crossing (Hanning windowing, 1024 ms FFT, 512 ms point window). The absolute power of the power spectrum from each channel was averaged in the alpha (9–13 Hz), beta (14–30 Hz), and low gamma (31–50 Hz) frequency bands across the epoch time course.
Gait analysis
Spatiotemporal gait parameters were measured using a 5.74-m GAITRite system (CIR Systems Inc., Clifton, NJ), sampled at 200 Hz, and placed over one straight segment of the circuit. This system was connected to a computer using GAITRite software version 4.7.5 (CIR Systems Inc.) for recording, initial processing, and storing data. A customized Matlab script was used to calculate gait parameters. The last 4 steps prior to the obstacle (approach phase) and the 4 steps of unobstructed walking registered by the carpet were considered for analysis. Means and variability (equal to the coefficient of variation: CV = [SD/mean]*100) were calculated for step length, step duration, and velocity.
Statistical Analysis
Subject characteristics between groups were compared using independent T tests for demographic, clinical, and cognitive variables, and the chi-squared test for sex. Relative HbO2, EEG dependent variables, and gait parameters were analyzed by 2-way analyses of variance (ANOVAs), with group (PIGD vs TD) and condition (unobstructed walking vs obstacle avoidance) as factors, with repeated measures for condition. Tukey post hoc tests were used to localize the differences between groups. All statistical analyses were run using SPSS 22.0 (IBM Corporation, Armonk, NY). The level of significance was set at p <.05.
Results
Table 1 summarizes the characteristics of the participants. As expected, the PIGD presented higher PIGD -scores and lower TD scores compared to the TD. No patients presented any episode of freezing during the gait protocol.
. | PIGD . | TD . | p Value . |
---|---|---|---|
Demographic | |||
Sex (male/female) | 11/6 | 12/7 | .923 |
Age (y) | 69.4 ± 6.6 | 70.8 ± 6.8 | .523 |
Height (cm) | 166.8 ± 10.0 | 164.8 ± 11.0 | .559 |
Weight (kg) | 73.2 ± 10.9 | 71.5 ± 9.0 | .607 |
Clinical | |||
Disease duration (y) | 4.5 ± 2.5 | 5.2 ± 2.8 | .453 |
MDS-UPDRS III (0–132) | 35.6 ± 16.1 | 27.6 ± 10.0 | .080 |
PIGD score (0–20) | 4.4 ± 1.6 | 1.8 ± 1.2 | <.001* |
TD score (0–44) | 5.7 ± 3.7 | 9.8 ± 4.6 | .006* |
Levodopa equivalent dose (mg/d) | 530.5 ± 384.0 | 633.7 ± 326.3 | .390 |
Cognitive function | |||
Mini-Mental State Examination (0–30) | 26.1 ± 2.5 | 25.9 ± 2.5 | .894 |
Trail Making Test (B-A) (s) | 103.2 ± 85.4 | 140.6 ± 98.3 | .234 |
. | PIGD . | TD . | p Value . |
---|---|---|---|
Demographic | |||
Sex (male/female) | 11/6 | 12/7 | .923 |
Age (y) | 69.4 ± 6.6 | 70.8 ± 6.8 | .523 |
Height (cm) | 166.8 ± 10.0 | 164.8 ± 11.0 | .559 |
Weight (kg) | 73.2 ± 10.9 | 71.5 ± 9.0 | .607 |
Clinical | |||
Disease duration (y) | 4.5 ± 2.5 | 5.2 ± 2.8 | .453 |
MDS-UPDRS III (0–132) | 35.6 ± 16.1 | 27.6 ± 10.0 | .080 |
PIGD score (0–20) | 4.4 ± 1.6 | 1.8 ± 1.2 | <.001* |
TD score (0–44) | 5.7 ± 3.7 | 9.8 ± 4.6 | .006* |
Levodopa equivalent dose (mg/d) | 530.5 ± 384.0 | 633.7 ± 326.3 | .390 |
Cognitive function | |||
Mini-Mental State Examination (0–30) | 26.1 ± 2.5 | 25.9 ± 2.5 | .894 |
Trail Making Test (B-A) (s) | 103.2 ± 85.4 | 140.6 ± 98.3 | .234 |
Notes: MDS-UPDRS III = Movement Disorders Society–Unified Parkinson’s Disease Rating Scale, motor part; PIGD = postural instability gait disorder; TD = tremor dominant.
*p < .05 at independent T tests.
. | PIGD . | TD . | p Value . |
---|---|---|---|
Demographic | |||
Sex (male/female) | 11/6 | 12/7 | .923 |
Age (y) | 69.4 ± 6.6 | 70.8 ± 6.8 | .523 |
Height (cm) | 166.8 ± 10.0 | 164.8 ± 11.0 | .559 |
Weight (kg) | 73.2 ± 10.9 | 71.5 ± 9.0 | .607 |
Clinical | |||
Disease duration (y) | 4.5 ± 2.5 | 5.2 ± 2.8 | .453 |
MDS-UPDRS III (0–132) | 35.6 ± 16.1 | 27.6 ± 10.0 | .080 |
PIGD score (0–20) | 4.4 ± 1.6 | 1.8 ± 1.2 | <.001* |
TD score (0–44) | 5.7 ± 3.7 | 9.8 ± 4.6 | .006* |
Levodopa equivalent dose (mg/d) | 530.5 ± 384.0 | 633.7 ± 326.3 | .390 |
Cognitive function | |||
Mini-Mental State Examination (0–30) | 26.1 ± 2.5 | 25.9 ± 2.5 | .894 |
Trail Making Test (B-A) (s) | 103.2 ± 85.4 | 140.6 ± 98.3 | .234 |
. | PIGD . | TD . | p Value . |
---|---|---|---|
Demographic | |||
Sex (male/female) | 11/6 | 12/7 | .923 |
Age (y) | 69.4 ± 6.6 | 70.8 ± 6.8 | .523 |
Height (cm) | 166.8 ± 10.0 | 164.8 ± 11.0 | .559 |
Weight (kg) | 73.2 ± 10.9 | 71.5 ± 9.0 | .607 |
Clinical | |||
Disease duration (y) | 4.5 ± 2.5 | 5.2 ± 2.8 | .453 |
MDS-UPDRS III (0–132) | 35.6 ± 16.1 | 27.6 ± 10.0 | .080 |
PIGD score (0–20) | 4.4 ± 1.6 | 1.8 ± 1.2 | <.001* |
TD score (0–44) | 5.7 ± 3.7 | 9.8 ± 4.6 | .006* |
Levodopa equivalent dose (mg/d) | 530.5 ± 384.0 | 633.7 ± 326.3 | .390 |
Cognitive function | |||
Mini-Mental State Examination (0–30) | 26.1 ± 2.5 | 25.9 ± 2.5 | .894 |
Trail Making Test (B-A) (s) | 103.2 ± 85.4 | 140.6 ± 98.3 | .234 |
Notes: MDS-UPDRS III = Movement Disorders Society–Unified Parkinson’s Disease Rating Scale, motor part; PIGD = postural instability gait disorder; TD = tremor dominant.
*p < .05 at independent T tests.
Prefrontal Activity During Walking
A main effect of Group was found for HbO2 level, showing that PIGD patients had higher PFC activity in both left (F1,34 = 8.528, p = .006, η p2 = .201) and right hemispheres (F1,34 = 5.590, p = .024, η p2 = .141) than TD patients, regardless of walking condition (Figure 1). In addition, a main effect of Condition was observed for HbO2 level in the right hemisphere (F1,34 = 4.795, p = .036, η p2 = .124). Participants presented increased right PFC activity during obstacle avoidance compared to unobstructed walking (Figure 1). No interaction was observed between Group and Condition.
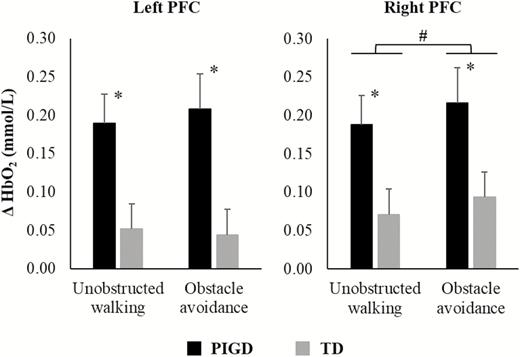
Means and standard errors of oxygenated hemoglobin (HbO2) levels in the left and right hemisphere during unobstructed walking and obstacle avoidance for PIGD and TD groups. *A main effect of group; #a main effect of condition.
Electroencephalography Absolute Power During Walking
Data of 2 participants of the PIGD group and 3 of the TD group were removed due to substantial noise in the EEG recordings. Therefore, statistical analyses of EEG variables included data from 15 PIGD and 16 TD patients.
Alpha band
A main effect of Condition was observed, showing that both groups presented decreases in alpha power in the FCz (F1,29 = 8.309, p = .007, η p2 = .223) in the obstacle avoidance condition when compared to unobstructed walking (Figure 2). There was no interaction between Group and Condition.
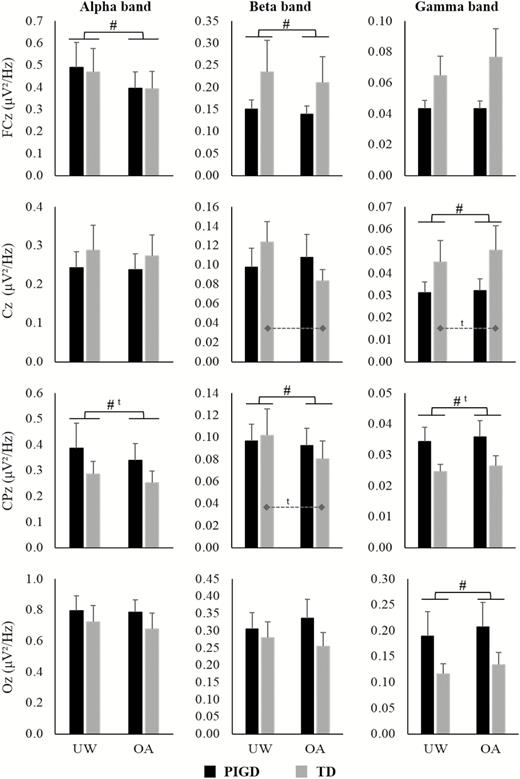
Means and standard errors of EEG absolute power of FCz, Cz, CPz, and Oz channels during unobstructed walking (UW) and obstacle avoidance (OA) condition for PIGD and TD groups. Dashed lines indicate the difference between conditions for the TD group in the interaction analysis. #Main effect of Condition; ta trend in the results (p < .08).
Beta band
A Group by Condition interaction was observed in the Cz (F1,29 = 6.473, p = .017, η p2 = .182) (Figure 2). The post hoc test showed that the TD group presented decreased beta power in the Cz (p = .007) during the obstacle avoidance compared to unobstructed walking condition, whereas no difference was observed for the PIGD group. A main effect of Condition was observed in the FCz (F1,29 = 4.290, p = .047, η p2 = .129) and CPz channels (F1,29 = 8.023, p = .008, η p2 = .217) (Figure 2). Compared to unobstructed walking, in the obstacle condition, both the PIGD and TD groups presented decreases in the absolute power of these channels.
Gamma band
A main effect of Condition was observed in the Cz (F1,29 = 7.786, p = .009, η p2 = .212) and Oz channels (F1,29 = 4.136, p = .05, η p2 = .125) (Figure 2). Regardless of the group, participants presented increased absolute power of these channels in the obstacle avoidance when compared to unobstructed walking. There was no interaction between Group and Condition.
Gait Behavioral Performance
Gait parameters are presented in Figure 3. Overall, participants presented decreased step length (F1,34 = 15.766, p < .001, η p2 = .317) and velocity (F1,34 = 17.682, p < .001, η p2 = .342), and increased variability of step length (F1,34 = 33.119, p < .001, η p2 = .493), step time (F1,34 = 14.603, p = .001, η p2 = .300) and step velocity (F1,34 = 7.111, p = .012, η p2 = .173) during obstacle avoidance when compared to unobstructed walking. No significant interactions were observed between Group and Condition.
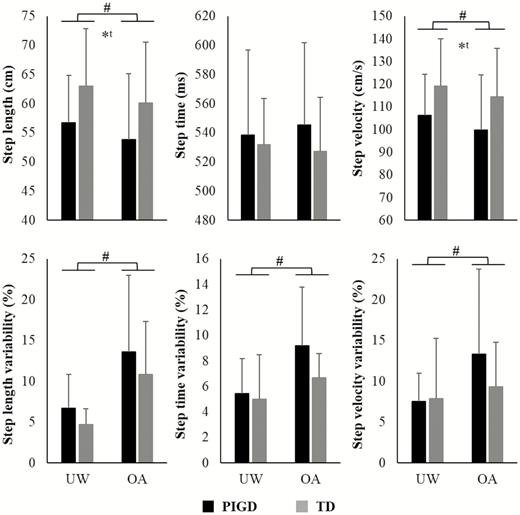
Means and standard deviation of gait parameters for PIGD and TD groups during unobstructed walking (UW) and obstacle avoidance (OA) conditions.*A main effect of group; #a main effect of condition; ta trend in the results (p < .065).
Discussion
Our main findings were that PIGD patients presented higher PFC activation when compared to TD patients, regardless of walking condition. Moreover, TD patients showed a reduction in the beta EEG absolute power in the Cz channel. Overall, our results demonstrated that cortical activity during walking is different between PD subtypes, in which PIGD patients need more recruitment of cognitive resources for walking, while TD patients present greater electrocortical modulation in the motor area during obstacle avoidance in order to maintain balance control and perform the task.
Parkinson’s disease subtype influences PFC activity during walking. Our results indicating that PIGD patients show greater PFC activity than TD patients in both walking conditions is in line with previous studies, which demonstrated increased use of executive-attentional resources to compensate for gait deficits (12,20–22,29). However, the PIGD group presented (trend) shorter step length (p = .062) and slower gait velocity (p = .058) (Figure 3), suggesting that the compensatory mechanism was not efficient in maintaining gait variables at the same level as the TD group. Previous studies have also observed more severe gait problems in PIGD patients (4–6), which may be associated with several structural and functional brain abnormalities (eg, altered connectivity between the striatum and fronto-parietal areas) (7–9). Our results are consistent with the neural inefficiency hypothesis, which states that a brain impaired due to aging and/or pathology does not efficiently allocate resources to support task demands (43). Thus, patients with greater cortical activation may present worse behavioral performance (12,21). Taken together, our findings suggest that PIGD patients increased PFC activation towards allocating more executive-attentional resources during walking. However, the motor output for PIGD patients is still inferior when compared to TD patients due to a more impaired neural system.
Prefrontal cortex resources contribute to control in the obstacle avoidance task. This task requires the use of cognitive resources to examine the environment, motor planning, attention to relevant stimuli, and visual-spatial ability to successfully navigate in a challenging environment (44,45). Our results corroborate these suggestions, by demonstrating that both PIGD and TD patients presented increased HbO2 levels in the right hemisphere during obstacle avoidance. Interestingly, the right hemisphere has been associated with the processing of spatial stimuli (46). Indeed, obstacle avoidance is a predominantly spatial task. Together, our results demonstrate that right PFC activity plays an essential role in controlling the locomotor task during navigation in the environment with obstacles.
Walking in environments with obstacles requires the activation of additional cortical areas, such as motor/sensorimotor and visual areas (15–17,19,47,48). For instance, tasks with higher balance demand, such as active walking compared to robot-assisted walking (18), walking on a balance beam (15), and normal walking compared to stabilized walking (16), may reduce alpha and beta power in motor/sensorimotor cortical areas. Our findings of reduced EEG power during obstacle avoidance in the alpha and beta bands in the FCz and CPz channels, which are related to the SMA and parietal cortex, corroborate these previous studies. In addition, we found a higher gamma band at Cz, CPz (trend), and Oz in obstacle avoidance compared to unobstructed walking. These channels are associated with the M1, parietal, and visual cortex, respectively. The visual cortex has been associated with the processing of environment information (relevant stimuli) and identification of object attributes (48). The parietal cortex is related to planning gait modifications during approaching obstacles (19,47,48), and M1 is involved in the execution of these modifications (48). Therefore, our findings corroborate previous studies by indicating that the processing of sensorimotor and visual information is highly relevant to a successful motor adjustment to avoid obstacles (17,19,48).
Considering PD subtypes, TD patients presented a significantly reduced beta band at Cz as well as a trend toward reduced CPz and increased gamma band at Cz during obstacle avoidance. As mentioned previously, oscillation in beta power in sensorimotor areas is related to balance demand for the task (15,16,18). In addition, previous studies suggested that gamma power oscillations in cortical motor areas facilitate movements in PD patients (49) and enhance motor performance (50). Increased gamma power is coupled with basal ganglia activity (49). However, as PIGD symptoms are related to hypo-connectivity between the motor area and the striatum (7,10), these patients may present difficulty increasing the gamma band in motor area when the task is required. Therefore, our findings showed that functional TD patients present further changes in electrocortical dynamics in order to maintain balance control and perform the obstacle avoidance.
This study presents some limitations. Firstly, the available sample size (PIGD: n = 17, TD: n = 19) and relatively high variability may have prevented us finding higher statistical power and/or other differences in fNIRS, EEG, and gait data, such as some interactions between Group and Condition. For example, we have observed some interesting trends, such as higher modulation in the Cz (gamma band; ANOVA: p = .076; post hoc test: p = .002) and CPz (beta band; ANOVA: p = .07; post hoc test: p = .002) channels (Figure 2) and larger step length (p = .062) and higher step velocity (p = .058) (Figure 3) for TD patients compared to PIGD. However, the small sample size may have limited the statistical power. In the processing data, we did not use short-separation channels to control the peripheral hemodynamic response. However, we applied Wavelet-MDL detrending to remove unknown global trends from our data, which has been shown to be acceptable (29,32). Additionally, we used a 32-channel EEG system, which may impose a limitation on our conclusions. The use of a high-density EEG (64 channels or more) would have allowed the extraction of independent components from participant datasets (15,16,18,19,50). Nonetheless, RUNICA was used to minimize the effects of artifacts on EEG data.
In conclusion, PD subtype influences the activity of multiple cortical areas during walking. Postural instability gait disorder patients need to recruit additional PFC executive-attentional resources for walking. Both PIGD and TD patients reduced alpha and beta power in FCz and CPz channels, which are related to the SMA and parietal cortex, to maintain the balance control during obstacle avoidance. In addition, TD patients present further change in the motor area activation to avoid obstacles, such as decreased beta power in the Cz. Therefore, these findings assist understanding of the neural mechanisms underlying PD subtype during walking, providing information for further interventions focused on gait in PD.
Funding
This study was supported by Coordenação de Aperfeiçoamento de Pessoal de Nível Superior (CAPES; Finance Code 001); Fundação de Amparo à Pesquisa do Estado de São Paulo (FAPESP; grant number 2017/19845-1, 2016/214991, 2014/22308-0); and Conselho Nacional de Desenvolvimento Científico e Tecnológico (CNPq; grant number #429549/2018-0).
Acknowledgments
The authors would like to thank Ellen Lirani-Silva, PhD, Vinicius Cavassano Zampier, MSc, Vinicius de Belli, BSc, and Lucas Meira Fiorio, BSc, for their assistance with data collection.
Conflict of Interest
None declared.
Author Contributions
D.O.-S. was involved in the conceptualization of the study, collecting, analysis, and interpretation of the data and drafting and revising the manuscript for intellectual content. R.V. was involved in the conceptualization of the study, data interpretation, and revising the manuscript for intellectual content. V.S.B. was involved in the data collection, EEG data analysis, data interpretation, and revising the manuscript for intellectual content. N.R.C. and P.N.-S. were involved in the data collection, fNIRS data analysis, data interpretation, and revising the manuscript for intellectual content. A.S.O. was involved in the EEG data analysis, data interpretation, and revising the manuscript for intellectual content. L.T.B.G. was involved in the conceptualization of the study, data interpretation, and revising the manuscript for intellectual content.
References
- gait
- parkinson disease
- electroencephalography
- gait disorders
- hemoglobin
- hyperbaric oxygenation
- motor cortex
- prefrontal cortex
- spectroscopy, near-infrared
- tremor
- visual cortex
- brain
- parietal lobe
- avoidance behavior
- brain activity
- characteristics of gait
- tissue degeneration
- postural instability
- supplementary motor cortex
- subcortical
- sensorimotor cortex