-
PDF
- Split View
-
Views
-
Cite
Cite
Xing-Xing Shi, Zhi-Zheng Wang, Yu-Liang Wang, Guang-Yi Huang, Jing-Fang Yang, Fan Wang, Ge-Fei Hao, Guang-Fu Yang, PTMdyna: exploring the influence of post-translation modifications on protein conformational dynamics, Briefings in Bioinformatics, Volume 23, Issue 1, January 2022, bbab424, https://doi.org/10.1093/bib/bbab424
- Share Icon Share
Abstract
Protein post-translational modifications (PTM) play vital roles in cellular regulation, modulating functions by driving changes in protein structure and dynamics. Exploring comprehensively the influence of PTM on conformational dynamics can facilitate the understanding of the related biological function and molecular mechanism. Currently, a series of excellent computation tools have been designed to analyze the time-dependent structural properties of proteins. However, the protocol aimed to explore conformational dynamics of post-translational modified protein is still a blank. To fill this gap, we present PTMdyna to visually predict the conformational dynamics differences between unmodified and modified proteins, thus indicating the influence of specific PTM. PTMdyna exhibits an AUC of 0.884 tested on 220 protein–protein complex structures. The case of heterochromatin protein 1α complexed with lysine 9-methylated histone H3, which is critical for genomic stability and cell differentiation, was used to demonstrate its applicability. PTMdyna provides a reliable platform to predict the influence of PTM on protein dynamics, making it easier to interpret PTM functionality at the structure level. The web server is freely available at http://ccbportal.com/PTMdyna.
Introduction
As an important regulatory mechanism of protein function, post-translational modifications (PTMs) play vital roles in numerous cellular processes [1–3]. PTMs greatly increase the diversity of cellular proteome by covalently linking functional groups to polypeptide chains. While the human genome encodes ~30 000 genes, the number of proteins in the human proteome is estimated to exceed 1 million [4]. Statistics studies indicate that there is an average of five recognized PTM sites on each human protein, and over 20% of proteins are modified by multiple PTM types [5]. PTMs can occur on 15 natural amino acids, generating over 600 modified residues [6]. PTMs influence various aspects of normal cell biology, such as signal transduction [7], cytoskeletal regulation [8] and protein degradation [9]. The dysregulation of PTMs is associated with many etiologies and pathogenesis, such as monogenic disorders, diabetes and cancer [10–12]. The well-annotated genes and gene lists with PTM-enriched disease mutations are provided in ActiveDriverDB [13]. Therefore, it is of great significance to investigate the biological effect of specific PTM and its corresponding molecular mechanism.
Protein functions are fundamentally related to protein dynamics, and many cellular effects of PTMs are intrinsically dependent on the conformational changes [14–16]. For example, Querfurth et al. [17] demonstrate that phosphorylation of Neurospora circadian repressor protein induces a conformational switch, which results in its temporally gated degradation. During the process of PTM, dramatic changes are likely to be driven in protein structure and dynamics due to the covalent linking, thus affecting the interaction between protein and its binding partners as well as the signaling pathway. In many cases, PTM events are protein-dependent [18]. The type and extend of PTM-induced structural changes vary considerably with a series of elements, including protein sizes, modification types, the location of PTM sites and protein structure. Thus, when studying how specific PTM work to fulfill a related biological function, it is helpful to understand PTM-induced conformational changes at an atomic level, which might facilitate a further explanation of the molecular mechanism and disease treatment.
During the last 20 years, bioinformatics approaches for investigating PTM events have exploded. One class of representative tools are in silico predictors of PTM sites based on the analysis of protein sequence with machine learning methods, such as KinasePhos [19], PPSP [20], MeMo [21], CKSAAP-Palm [22] and pSumo-CD [23]. With the growth of experimentally identified PTM site data and the development of artificial intelligence technology, such tools have been continually improved and upgraded. Another class of tools is used for analyzing PTMs based on proteomics data to reduce the difficulty of PTM quantitation, including PhosphoPic [24], VEMS [25] and VIPER [26]. Recently, it has been reported that many PTM sites are located in defined structurally regions and surrounded by specific 3D structural environments [27–29]. The attention of analyzing PTM has also been paid on structural context information rather than exclusively on local sequence patterns. Further, a series of tools used for structure-based analysis of PTMs were developed, such as Phospho3D [30], mtcPTM [27], phos3D [31], DoS [32] and ReKINect [33]. Phosphos3D and mtcPTM are databases providing structural information on atomic models of modified proteins. Phos3D is an online server for phosphorylation site prediction, which adopted the support vector machine method using both sequences as well as structural information and performed better than sequence-only based prediction methods. Dos approach aims to uncover structural features of residues encoding substrate specificity within the kinase domain. ReKINect is a platform to identify mutations affecting signaling networks, including the changes in kinase modulation and dysregulation of phosphorylation sites. Additionally, there are some approaches based on multi-class features to analyze PTMs. Beltrao et al. [34] used machine learning to identify 59 features indicative of proteomic, structural, regulatory or evolutionary relevance and integrate them into a single functional score, which can be used to determine the functional importance of each phosphorylation site. These available tools or methods facilitate the theoretical study of protein PTM with less cost and higher efficiency. However, few tools are aimed to explore the structural and dynamic effects of specific PTM, which play a vital role in related protein function. The absence of modified protein structures and the lack of parameters describing modified residues are two main reasons for this situation. Although some web-servers, such as Vienna-PTM [35], allow for automatic parameterization of protein structures in PTM state for molecular dynamics (MD) simulations, they do not provide analysis services for simulation results. Therefore, an integrated strategy for predicting the change tendency of protein structural dynamics induced by PTM(s) appears to be appealing.
Here, we present PTMdyna, a web-based server for analyzing insightfully the influence of specific protein PTM on conformational dynamics. Given an inputted protein structure and user-defined PTM requirements, PTMdyna will automatically construct a post-translational modified structure and then predict protein dynamics in both unmodified and modified states. PTMdyna exhibits an AUC of 0.884 to predict the effect of PTM on conformational dynamics tested on 220 cases of protein–protein complex structures. A comprehensive case of heterochromatin protein 1α (HP1α) complexed with lysine 9-methylated histone H3 (H3K9me) was showed to prove its applicability. Consequently, PTMdyna provides a platform for molecular biologists, structural biologists and even non-specialists to easier explore the PTM-induced influences on protein dynamics and interpret PTM functionality at the structure level.
Results and Discussion
Overall workflow of PTMdyna
As shown in Figure 1, the overall workflow of PTMdyna can be roughly divided into two parts. The initial part is designed to generate conformation ensembles. In this part, energy minimization and MD simulations are firstly performed on the uploaded protein or protein–protein complex structure. Then, the dominant conformation is extracted from the equilibrated MD trajectory based on clustering analysis and is duplicated into two groups. One group is used to generate novel modified structures according to user-defined PTM requirement. Another group is treated as a control group without any modification. The two groups are further investigated by energy minimization and MD simulations to obtain equilibrated conformation ensembles of unmodified and modified structures. The second part of PTMdyna workflow is focused on the analysis of the PTM-induced effect. In this part, various dynamic properties of original and modified protein systems are analyzed and compared based on structural ensembles generated from the equilibrated MD trajectory. If the uploaded structure is a protein–protein complex or multi-chain protein, the qualitative change in the protein–protein binding induced by the PTM is predicted.

The overall workflow of PTMdyna. The workflow consists of an improved CMS protocol and PTM-induced effect analysis. In the improved CMS protocol, a dominant conformation is picked from equilibrated unmodified conformation ensembles based clustering analysis and novel modified structure is constructed based on this dominant conformation. MD simulations for unmodified and modified structure are further carried out to obtain their equilibrated conformation ensembles. Based on them, a series of dynamic properties of original and modified protein systems are analyzed to predict PTM-induced influence in protein dynamics. If the uploaded structure is a protein–protein complex, the PTM-induced protein–protein binding change is predicted.
Usage of the webserver
Input
The input data (Figure 2A) passed to PTMdyna server consist of two required and two optional parameters: (i) a protein/protein–protein structure file in PDB format or a PDB ID collected in the RCSB Protein Data Bank [36, 37], (ii) modification details in a format of ‘initial residue name _ residue number _ modified residue name,’ involving the residue name before modification in three-letter code, the corresponding residue number in structure file, and the desired residue name after modification in three-letter code, (iii) a pair of chain name for defining the receptor and ligand of the protein–protein complex in a format of ‘A;B’ or ‘A,B;C’ (this input is optional, and when it’s empty, the modified chain is defaulted as ligand and others are receptor) and (iv) other optional inputs, inducing job name, E-mail address for notifying job state, and password for that make job private. In our server, the input is relatively simple and easy for users to operate.
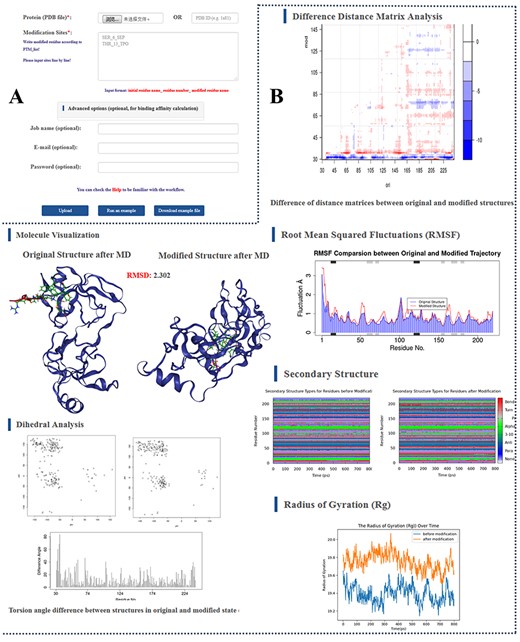
Screenshots of the PTMdyna webpages. (A) The input page for submitting job. The inputs include an upload PDB file, modification information and four optional contents (chain groups, task name, E-mail and password). (B) ‘Structure & Parameter’ tab of output page. This tab contains molecule visualization, dihedral analysis, distance difference analysis, secondary structure comparison and Rg comparison.
An initial checking module is developed in PTMdyna to avoid some common bugs in the input process. For example, if the name and number of inputted residue do not match, the web page will remind users with a message ‘The specific residue cannot be found in your PDB file.’ Only when all input parameters have been checked as acceptable, ‘submit’ button is allowed to start a job. A sample submission entry can run by pressing ‘run an example’ button, and all input data will be filled automatically for calculation. The details on the usage of this server are provided on the ‘Help’ webpage.
Output
In output pages, the results are displayed in four tabs: (i) structure & parameter tab (Figure 2B). In this tab, the original and modified structures that have undergone MD simulation are visualized. The Amber topology and parameter files of the two structures are available to download. The structures are shown visually in cartoon model and allowed to interactively manipulate. The modification sites are highlighted in stick model, and their surrounding residues within 5 angstroms are shown as line model. Basic Ramachandran plots of them are provided to evaluate its rationality. A series of residue-wise differences in these two structures are shown with diagrams, including dihedral angle, distance, root mean squared fluctuations (RMSF) and secondary structure. The radius of gyration (Rg) plot of these two structures and their pictures in B-factor putty style are also presented. (ii) Interaction analysis tab (Figure 3A). In this tab, the binding free energy difference value between the original and modified protein–protein complex is shown, along with the analysis of hydrogen bonds between modification sites and their surrounding residues. If there is only one chain in inputted protein, this tab will not exist. (iii) Dynamic conformation tab (Figure 3B). Herein, dynamic interpolated conformations of original and modified proteins are shown based on principal component analysis (PCA). The results of first three principal components during MD trajectories are also provided. Additionally, dynamical residue cross-correlation matrixes are displayed. (iv) Functional motion tab (Figure 3C). Herein, motion tendencies of original and modified proteins are displayed based on the first three principal components. Further, atomic movement similarity matrixes with domain annotation are graphically displayed. To sum up, the output results of PTMdyna are informative in various ways.
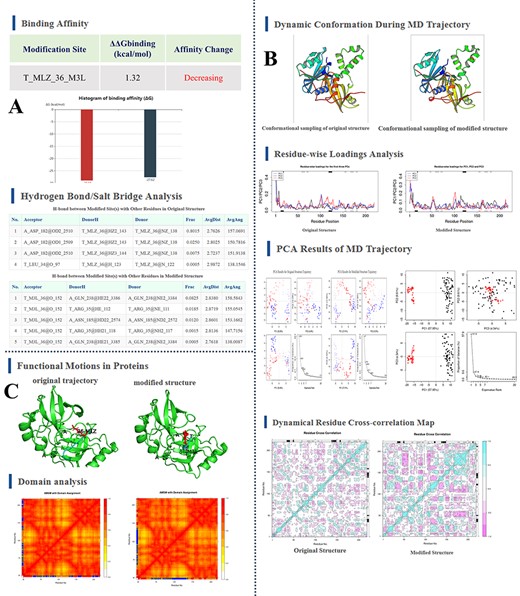
Screenshots of PTMdyna outputs. (A) ‘Interaction Analysis’ tab of output page. This tab presents binding free energy difference between unmodified and modified protein–protein complex, and hydrogen bonds between modification sites and their surrounding residues in two structures. (B) ‘Dynamic Conformation’ tab of output page. This tab shows dynamic interpolated conformations of original and modified proteins, an overview of PCA results, and their dynamical residue cross-correlation matrixes. (C) ‘Functional Motion’ tab of output page. This tab contains motion tendencies of original and modified proteins and their atomic movement similarity matrixes with domain annotation.
Performance of PTMdyna
The performance of PTMdyna in exploring the influence of PTM on conformational dynamics was evaluated by conformation-dependent protein–protein binding affinity change. The total test set contains 220 PTMs cases, including forwarding addition of PTM and reverse removal of PTM (Supplementary Table S1). For protein–protein complex structures, the change in conformational dynamics can simultaneously drive differences in protein–protein binding. The test dataset was carefully chosen from RCSB Protein Data Bank, consisting of protein–protein complexes crystal structures. According to the corresponding published experimental studies, the occurrence of specific PTM(s) in the complex structure would lead to a change of binding affinity between two proteins. The cases where the PTM(s) induced an experimentally measured increase in protein–protein binding affinity are considered as positive samples, while the cases where the PTM(s) induced an experimentally measured decrease are considered as negative samples. For each case, based on the known complex crystal structure, the influence of specific PTM(s) on binding affinity was also predicted using PTMdyna. The predictive and experimental qualitative changes in protein–protein binding affinity were compared. With flexibly dielectric constants in free energy calculation, PTMdyna achieved good performance with an area under the curve (AUC) value of 0.884, a sensitivity value of 0.822, a specificity value of 0.825 and an accuracy value of 0.823 (Figure 4A and B). As a result, the capability of PTMdyna to qualitatively predict the positive or negative effect of specific PTM on protein–protein binding is satisfactory, suggesting the effectiveness of PTMdyna for predicting PTM-induced changes in protein dynamics.
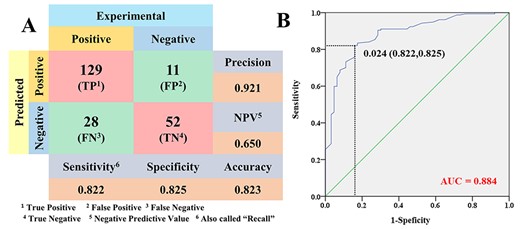
The performance of PTMdyna to qualitatively predict the change of protein–protein binding affinity induced by PTM(s). (A) The confusion matrix of predictive results in test set. (B) The ROC curve of classification of binding differences.
The performance of PTMdyna in predicting the impact of PTM on global conformational changes was evaluated by retrieving pair of experimentally obtained unmodified and modified protein crystal structures. About 25 pairs of conformers with PTMs were retrieved from CoDNaS database [38, 39]. Their crystal structures were collected from RCSB PDB and constituted 50 cases used as a test set (Supplementary Table S2). For each case, each structure was inputted into PTMdyna along with given specific modification details, and a predicted modified structure was outputted. The RMSD values between the experimentally observed and predictive modified structures were used as the criteria. When RMSD is no more than 4 Å, the prediction of PTM-induced global conformation change reflected by predictive modified structure is considered as successful [40]. About 82% of cases were predicted correctly, showing a good performance of PTMdyna in predicting global conformational changes. In addition, the performance of PTMdyna to prioritize potentially functional PTM sites was compared with the study of Beltrao’s team [34]. The cases with high phosphosite functional score (>0.5) were considered as positive samples, reflecting a higher importance of the PTM site for organismal fitness. While, the cases with low phosphosite functional score (<0.1) were considered as negative samples, reflecting a less importance of the PTM site for organismal fitness. An affinity change value (ΔΔGbinding) of 5 kcal/mol was defined as the threshold to distinguish whether the PTM site can induce a significant influence for the protein–protein interaction in each case. For positive samples, if the predicted absolute value of affinity change is more than 5 kcal/mol, the PTMdyna’s prediction by is considered as successful. For negative samples, if the predicted absolute value of affinity change is less than 5 kcal/mol, the PTMdyna’s prediction by is considered as successful. It was found that 81.1% of the cases exhibited consistent prediction results using these two methods (Supplementary Table S3 and Figure S1).
Case study of the influence of phosphorylation on HP1α-H3K9me complex
Heterochromatin protein 1 (HP1), an important transcriptional repressor, is strongly implicated in genomic stability and cell differentiation [41–43]. The chromodomain (CD) of HP1α, an HP1 isoform, directly binds to lysine 9 methylated histone H3 (H3K9me), a hallmark of histone modification for transcriptionally silenced heterochromatin [44, 45]. HP1 has been known to be a dynamic protein that functions in transcriptional elongation, telomere maintenance, and DNA repair. [46, 47]. HP1 proteins are liable to be post-translational modified within the context of H3K9me, which probably relates to these functional regulations [48]. Hamada et al. [49] revealed that N-terminal phosphorylation (S11–14) of HP1α enhanced its affinity for H3K9me and played an essential role in properly targeting to heterochromatin. Shimojo et al. [50] examined the unmodified and phosphorylated HP1α-H3K9me complex structures using NMR, and they found that the phosphorylation induced obvious conformational changes in the N-terminal tail of HP1α and H3K9me peptide state. Hence, the application of HP1α-H3K9me complex in PTMdyna is used as a case to uncover the structural role of PTM to ensure protein–protein interactions.
In this case, the influences of successive phosphorylation at four serine residues to HP1α-H3K9me complex on conformational dynamics are explored. The crystal structure of a chromodomain of HP1α with a phosphorylated N-terminal tail (phos-NCD) complexed with an H3K9me3 peptide (PDB ID: 2RVN) was uploaded into PTMdyna. The four phosphorylated serine residues (pS11–14) were modified into a natural state so that unmod-NCD bound with H3K9me (unmod-NCD-H3K9me) was the original structure and phos-NCD bound with H3K9me (phos-NCD-H3K9me) was the modified structure. The computational results of this case from PTMdyna server are expected to interpret the phosphorylation-induced binding difference between HP1α and H3K9me by analyzing conformational dynamic changes.
Part A: Influence of phosphorylation on conformational dynamics of HP1α-H3K9me
To explore the structural differences between phos-NCD-H3K9me and unmod-NCD-H3K9me, a series of dynamic properties of their conformations were analyzed. PTMdyna renumbered the residue in structures. The new residue number is adopted in this section. The root mean square deviation (RMSD) value of phos-NCD-H3K9me (original structure) and unmod-NCD-H3K9me (modified structure) indicated that the two structures arrived an equilibrium stage in the last 10 ns (Figure 5A). Thus, the analysis of conformational dynamics based on this stage could continue.
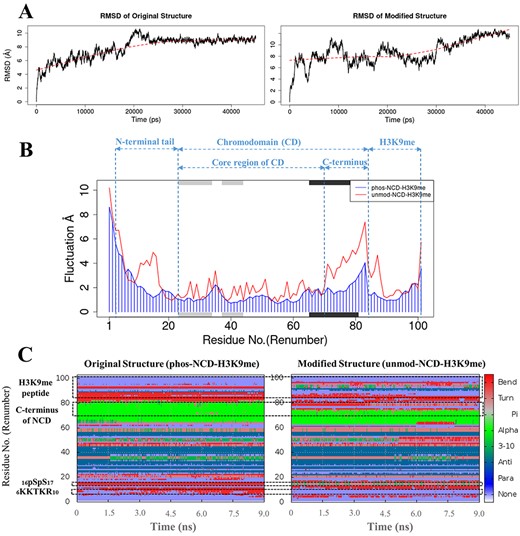
A comparison of some dynamic properties of HP1α-H3K9me without and with phosphorylation modifications (serine residues: 11–14). (A) RMSD of original (phos-NCD- H3K9me, left) and modified structure (unmod-NCD- H3K9me, right) during equilibrium MD trajectories. (B) RMSF of phos-NCD-H3K9me (blue line) and unmod-NCD-H3K9me (red line) during equilibrium stage. (C) Dynamic secondary structures of phos-NCD-H3K9me (left) and phos-NCD-H3K9me (right).
The RMSF comparison results could reflect flexibility change induced by serine phosphorylation (Figure 5B). In general, the fluctuation values of phos-NCD-H3K9me were less than that of umod-NCD-H3K9me, suggesting that phos-NCD-H3K9me system is not as flexible as unmod-NCD-H3K9me system. The three segments of umod-NCD-H3K9me, including N-terminal tail of HP1α (residues: 3–22), C-terminus of HP1α (residues: 71–84) and H3K9me peptide (residues: 85–101), exhibited significantly higher RMSF values than them of phos-NCD-H3K9me. This is consistent with the experimental observation that many NMR signals in the tail region for umod-NCD-H3K9me disappeared due to the large-amplitude conformational fluctuations while that for phos-NCD-H3K9me did not [50]. The changes in the flexibility of these portions might be direct effects of four phosphonate linkages, because phosphorylation occurs at N-terminal tail of HP1α which is near to H3K9me peptide.
The calculated secondary structures revealed protein stability in the residue level (Figure 5C). In C-terminus of NCD (residues: 71–84), phos-NCD-H3K9me formed a wide alpha helix (green bar), while unmod-NCD-H3K9me formed dominate none (light purple) and bend (red bar) secondary structures, indicating improved stability of this segment upon phosphorylation. The last two phosphorylation sites (residues:16–17) of N-terminal tail showed bend structures in phos-NCD-H3K9me, while they mainly showed turn structures in unmod-NCD-H3K9me, suggesting a local structural change caused by linking phosphate groups. The negatively charged segment (KKTKR, residue: 6–10) in N-terminal tail perpetually showed none secondary structure in unmod-NCD-H3K9me, while they exhibited bend structures in most of time in phos-NCD-H3K9me, suggesting that the phosphorylation of 14SSSS17 induces a stability reduction in the nearby basic segment. Also, the secondary structures of negatively charged H3K9me peptide were partially changed. The calculated changes of NCD-H3K9me in secondary structures could match with the changes in flexibility. Hence, these data suggested that the phosphorylation-induced extend of an acidic segment from 18EDEEE22 to 13DpSpSpSpSEDEEE22 alter the balance between interactions of this acidic segment with basic segment 6KKTKR10 of NCD and with basic residues of H3K9me peptide.
The comparison of Rg indicated the influence of phosphorylation on molecular compactness (Figure 6A). The calculated average Rg values of phos-NCD-H3K9me and umod-NCD-H3K9me during the equilibrated MD trajectory were 17.11 ± 0.05 Å and 16.52 ± 0.09 Å, which were close with the corresponding Rg values of phos-NCD (18.9 Å) and unmod-NCD (17.2 Å) derived from small-angle X-ray scattering (SAXS) experiments [50]. This suggested that phos-NCD-H3K9me had a less compact structure than phos-NCD-H3K9me, which reflected the bulkiness and high charge of phosphorylated serine residues that easily lead to a series of electrostatic and steric repulsions.
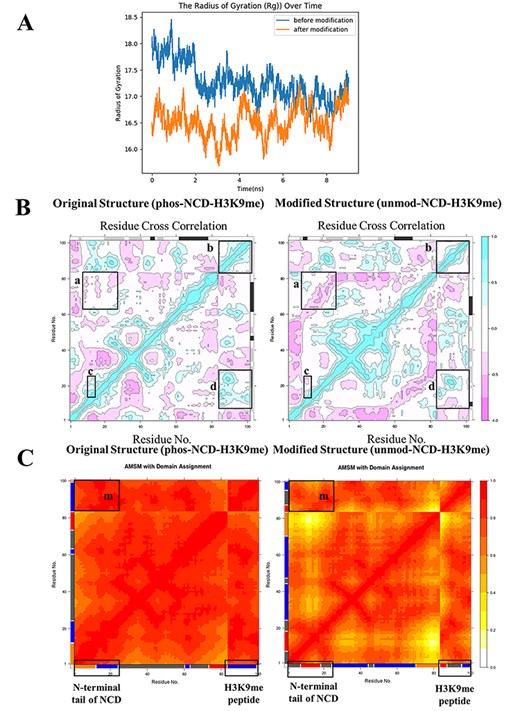
A comparison of unmod-HP1α-H3K9me and phos-HP1α-H3K9 in Rg, residue cross correlation and atomic movement similarity. (A) Rg of unmod-HP1α-H3K9m (organe) and phos-HP1α-H3K9 (blue). (B) Residue cross-correlation maps of phos-NCD- H3K9me (left) and unmod-NCD- H3K9 (right). Region a: the correlation between C-terminus of NCD (residues: 63–84) and N-terminal tail of NCD (residues: 3–22); Region b: the cross correlation of each residue in H3K9me peptide; Region c: the correlation between basic segment (residues: 6–10) of NCD and acidic segment plus four seine residues (residues: 14–22) of NCD; Region d: the correlation between H3K9me peptide and N-terminal tail (residues: 3–22) of NCD. (C) Atomic movement similarity matrixes (AMSM) with domain assignment of ophos-NCD- H3K9me (left) and unmod-NCD- H3K9me (right). Region m: atomic movement similarity between N-terminal tail of phos-NCD and H3K9me peptide.
The calculated residue cross-correlation map could exhibit motion tendency in proteins (Figure 6B). The magnitude of pairwise cross-correlation coefficients in NCD-H3K9me was changed greatly upon the phosphorylation, indicating a significant change in functional motion. Four regions in the correlation maps were selected for further analysis. The coefficients in the region a generally became less negative after the serine phosphorylation, indicating that phosphorylation induced the movement of NCD between C-terminus segment (residues: 63–84) and N-terminal tail segment (residues: 3–22) to be more irrelevant from opposite directions. This difference agreed with the calculated change in secondary structure and RMSF (Figure 5B and C). They were spatially diagonal and far away from each other. Originally, these two segments belonging to none or bend secondary structures fluctuated with high flexibility, making them move in opposite directions according to harmonic vibrational analysis. Upon phosphorylation, C-terminus segment currently belonging to alpha-helix fluctuated with low flexibility, making it move in small scale independent of flexible N-terminal tail. The comparison of region b showed that after phosphorylating NCD-H3K9me, there were more residues in H3K9me peptide that tended to move in the same directions. This difference implied that phosphorylation induced more intra-molecular interactions in H3K9me peptide. For N-terminal tail of NCD, the movement correlations between the basic segment (6KKTKR10) and acidic segment plus four seine residues (14SSSSEDEEE22) were displayed through region c. The movement of them was more positively correlated in phos-NCD-H3K9me, inferring that the phosphorylation induced more interactions between the 6KKTKR10 and 14SSSSEDEEE22. The inferred conclusions based on regions b and c agreed with the above conclusion of secondary structure analysis. As shown in region d, H3K9me peptide and N-terminal tail of NCD became more inclined to move in the same directions after the phosphorylation of NCD-H3K9me, inferring that phosphorylated N-terminal tail of NCD formed more inter-molecular interactions with H3K9me peptide than its unmodified state. This explanation corresponded well with available experimental data measured by fluorescence anisotropy that the N-terminal phosphorylation increased the affinity of HP1α NCD for H3K9me3 peptide by 4.3-fold [49].
The domain analysis based on atomic movement similarity was helpful to detect the rigid domains in protein structure (Figure 6C). The difference of color in region m indicated that N-terminal tail of phos-NCD has a higher movement similarity with H3K9me peptide than that of unmod-NCD. The domain assignment is annotated at the bottom and left sides of the two maps. For N-terminal tail of phos-NCD, there were two domains separated by a middle residue. However, for N-terminal tail of unmod-NCD, there were three segments separated by two middle residues but still two domains. The first and third segments belong to the same domain, while the middle segment belong to another domain. In phos-NCD-H3K9me, the entire H3K9me peptide belongs to the same domain as the last segment of N-terminal tail of phos-NCD. In unmod-NCD-H3K9me, H3K9me peptide can be distributed into three different domains. These differences showed that the phosphorylation induces N-terminal tail of NCD and the H3K9me peptide to be more likely to move as rigid bodies, which is consistent with the residue cross-correlation analysis. Therefore, taking these analysis results of dynamic properties together, it can be demonstrated that PTMdyna is a powerful tool for exploring the influence of PTM on protein conformational dynamics.
Part B: Influence of phosphorylation on the protein–protein binding of HP1α-H3K9me
To investigate the influence of successive serine phosphorylation on interactions between HP1α and H3K9me, the binding free energies of them in the different states were predicted. The predictive binding free energy difference between phos-NCD-H3K9me and unmod-NCD-H3K9me was a positive value, indicating a stronger binding affinity after the phosphorylation, which could agree with experimental thermodynamic data. In particular, it has been known that phos-NCD bound to H3K9me with stronger affinity (Kd = 0.17 μM) than unmod-NCD (Kd = 1.77 μM) [50]. Based on the destination structure after MD simulation, the RMSD value between phos-NCD-H3K9me and unmod-NCD-H3K9me was estimated at 12.739 Å. This suggested that sequential phosphorylation induced a significant change in both in structure and protein interactions of NCD-H3K9me.
To explore the dynamic interactions in HP1α-H3K9me system more explicitly, the interactions between charged residues were further analyzed. As shown in Figure 7A and B, the negatively charged acidic segment (red spheres) of NCD could interact with either basic segments (blue spheres) of NCD or basic residues of H3K9me. In phos-NCD-H3K9me, there were more and complicated inter-molecular interactions. The representative interactions between NCD and H3K9me included the hydrogen bonds between pS14 and R17, and pS13 and K4, between pS12 and R2, between pS11 and K14, and between E19 and R2 (Figure 7A). In unmod-NCD-H3K9me, glutamate rather than phosphorylated serine served as an acid residue to participate in these interactions, leading to fewer inter-molecular interactions. The representative interactions are ones between E19 of NCD and R2 of H3K9me, as well as between E19 of NCD and Q5 of H3K9me (Figure 7B). The phosphorylation of the four serine residues created an extension of the acid segment in NCD from 15EDEEE19 to 10DpSpSpSpSEDEEE19, which might contribute to stronger electrostatic interactions between NCD and positively charged H3K9me peptide.
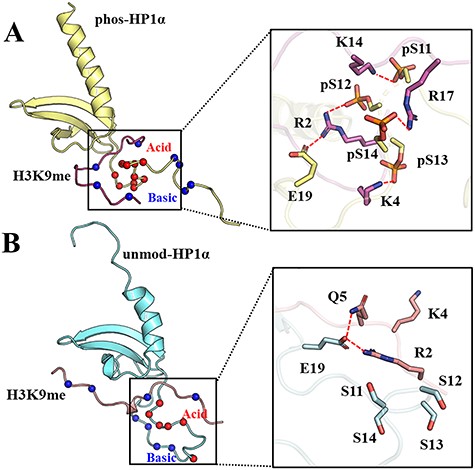
The destination structures of phos-HP1α-H3K9me and unmod-HP1α-H3K9me after MD simulations. (A) Phos-HP1α-H3K9me structure and representative interactions between phos-HP1α and H3K9me. Yellow: phos-HP1α. Magenta: H3K9me. After HP1α was phosphorylated, there were more inter-molecular interactions, including representative interactions between pS14 of HP1α and R17 of H3K9me, between pS13 of HP1α and K4 of H3K9me, between pS12 of HP1α and R2 of H3K9me, between pS11 of HP1α and K14 of H3K9me, and between E19 of HP1α and R2 of H3K9me. (B) Unmod-HP1α-H3K9me structure and representative interactions in unmod-HP1α-H3K9me. Cyan: unmod-HP1α. Pink: H3K9me. Compared to phosphorylated HP1α-H3K9me, there are less inter-molecular interactions between HP1α and H3K9me when N-terminal tail of HP1α is unmodified. Representative interactions contained the one between E19 of HP1α and Q5 of H3K9me, and the one between E19 of HP1α and R2 of H3K9me.
To figure out what specific residue contributed to the change in HP1α-H3K9me binding, the hydrogen bonds between modification sites and its surrounding residues were further analyzed (Supplementary Tables S4 and S5). In unmod- HP1α-H3K9me system, the top six hydrogen bonds with high fractions were all intra-molecular ones of HP1α. In the intermolecular hydrogen bonds between 11SSSS14 of HP1α and H3K9me peptide, the one between SER12 of HP1α and ARG2 of H3K9me had the largest fraction (0.2573). However, in phos-HP1α-H3K9me system, 16 hydrogen bonds had fractions of over 20%, and 11 of them were inter-molecular hydrogen bonds between 11pSpSpSpS14 of HP1α and H3K9me peptide. Two salt bridges between pS14 of HP1α and R17 of H3K9me were formed with fractions of 58.84% and 41.06%, indicating that there existed stable interactions between these two residues. Their differences in hydrogen bond distribution also supported the experimental fact that N-terminal phosphorylation of HP1α promoted its H3K9me binding [47, 49, 50]. Furthermore, the key role of pS14 in enhancing the interaction between NCD and H3K9me peptide was computationally revealed through PTMdyna, which corresponded well with Hamada et al.’s experimental data obtained from site-directed mutations of mouse HP1α. Their results showed that S14 phosphorylation is a prerequisite for or enhances the phosphorylation of its neighboring serine residues [49]. In general, the analysis results about HP1α-H3K9me binding based on the outputs of PTMdyna can agree well with experimental data, demonstrating the availability of PTMdyna.
Features and Limitations
As a web server to explore PTM-induced influence on protein conformational dynamics, the features of PTMdyna are concluded. First, the server contains 83 biologically relevant PTMs, allowing users to modify the inputted protein according to their own needs. Second, the original and modified structures after MD simulation and their Amber parameters are provided as job results for further MD analysis. Third, the outputs of PTMdyna are various, visual, and downloadable, covering multiple dynamic properties and interaction analysis.
However, it should be acknowledged that PTMdyna currently still has some limitations for further improvement. First, when predicting PTM-induced protein–protein interaction change, the changes in hydrogen bond networks between unmodified sites have not been intuitively presented on the results page. Second, the PTMs at terminal residues of peptides have not been covered yet. Third, in some cases, a relatively long computation time (several hours) is required to obtain predicted results, especially when a large protein is given. Finally, in a few cases, the calculated protein–protein binding free energy induced by PTM exhibited a relatively significant deviation, especially when there are many charged residues at protein–protein interfaces and they are aggregative distributed. In the future, we will be committed to overcoming these remaining limitations.
Conclusion
PTMs play an important role in biological functions, and their dysregulations may result in many diseases. The understanding of PTM-induced conformational changes is helpful to study related cellular biological function, which might facilitate a further discovery of disease treatment. Here, PTMdyna is introduced, a freely available web server that offers users an opportunity to analyze the influence of specific PTMs on protein conformational dynamics. The output results are multidimensional and most of them are shown visually. PTMdyna achieved an AUC value of 0.844, a specificity value of 0.825, and an accuracy value of 0.823, for qualitatively predicting the effect of specific PTM on protein–protein binding, indicating its effectiveness for predicting PTM-induced changes in protein dynamics. The case of HP1α-H3K9me complex was studied using PTMdyna. Most of the output results of this case can correspond well with or interpret the experimental results, which demonstrated the applicability of the server. As a valuable tool for exploring PTMs, PTMdyna is expected to assist biomedical, chemical or pharmaceutical researchers to study PTM-dominated events and reveal the related molecular mechanism.
Materials and Methods
Force-field database for PTM
PTMdyna is aimed to cover known PTMs as completely as possible. We compiled an Amber-compatible parameter library for 83 post-translational modified amino acids, covering phosphorylation, acetylation, methylation, hydroxylation, nitration, carboxylation, carbonylation, palmitoylation, chlorination, iodination and many others. The force field parametrization of the 83 PTM types was prepared according to the details of Floudas’ method [51], but the initial structures for each modified amino acid were derived from RCSB PDB [36, 37] rather than constructed manually. Currently, all available PTMs in this server are obtained by covalently adding chemical groups on natural residues, but terminal modifications are not included. Except for glycine and valine, the reaming 18 natural amino acids all have corresponding modifications. Further, PTMdyna can support simultaneous modifications of multiple residues on a protein. Similar to the PDB format file, the newly introduced modified residues are represented using a three-letter code. For example, ‘ALA’ equals to alanine and ‘MAA’ equals to N-methyl alanine. The complete relation list of provided PTMs in this server and the modified residue structures are shown on the PTM_list page.
Computational mutation scanning
Our previously developed computational mutation scanning (CMS) protocol [52–55] was optimized and integrated into PTMdyna. The improved CMS was briefly described as following (Figure 1): (i) minimization and MD simulation for the starting protein structure was carried out to generate the equilibrium conformation ensembles, and a dominant conformation was picked using clustering analysis, (ii) novel modified protein structure was constructed by covalently modifying specific residue of original dominant structure according to expected PTM detail, (iii) original and modified structures were further parallelly performed MD simulations to obtain their reasonable conformation clusters and (iv) the binding free energy difference between original and modified states was calculated if the starting inputted structure is a protein–protein complex.
MD simulation
The inputted starting protein or protein–protein complex and its modified form were performed energy minimization and MD simulation using explicit-solvent particle mesh Ewald (PME) model [56], as implemented in AMBER16 package [57]. Topology and coordinate files were created in the tleap module based on the Amber ff99SB force field [58], general AMBER force field (GAFF) [59] and self-built PTM parameter library. The protein system was solvated in an octahedron box of TIP3P water, with at least 10 Å between the solute and each box edge [60]. Counter ions, Na + or Cl−, was added to neutralize the net charges of the system.
The energy minimization was achieved through three stages: (i) only water molecules and ions were allowed to move, (ii) backbone atoms of protein are restrained and the remaining atoms were allowed to move, (iii) all atoms in the system could move freely. In each stage, 3000 steepest descent steps and 3000 conjugated gradient steps were carried out in a vacuum. The MD simulation process was achieved through three stages: (i) the system was heated from 0 to 300 K over 500 ps in the NVT ensemble with restraints on the solute, (ii) 1 ns of MD with restraints on the main chain of protein system in the NTP (T = 300 K and P = 1 atm) ensemble, (iii) 5 ns or longer of MD with all atoms relaxed. As the following description (see the “Cluster analysis” section), the structural cluster analysis based on an RMSD cutoff value of 2 Å was regarded as an evaluation criterion to decide whether the simulation should continue after 5 ns. Of note, the MD time of protein structure in unmodified group would be always consistent with that in modified group. To avoid the situation where too much computing resources and time is spent for a single task, the allowable running time of a task in the backend and the total number of residues in a protein system were limited. When the number of residues exceeds 2000, the MD simulation would not be performed and only the Amber topology and parameter files for the original and modified structures will be provided. Once the running time exceeds 5 days or MD simulation time exceeds 5 days without the arrival of an equilibrated MD, the task will be interrupted and the latest Amber topology and parameter files will be provided for a user to continue the MD simulation by themselves.
Cluster analysis
An average-linked hierarchical clustering algorithm was used for the cluster analysis [61], which was implemented using the CPPTRAJ module in Amber 16 package. The RMSD cutoff for the neighboring cluster set was given as 2 Å. Protein structures were extracted from MD trajectories at 1 picosecond intervals for analysis. The dominant conformations were obtained from the major cluster emerged from the MD trajectories.
A cluster-based analysis method was used to assess the convergence of MD simulation trajectories [62, 63]. In general, the conformational change in dynamics equilibrium stage exhibits a specific stable trend, and the number of clusters in equilibrium stage tends to converge [64]. The number of conformational clusters was used to judge the convergence of MD simulation, and a growth rate of 10% was defined as the threshold. Starting from the third ns, every 1 ns simulation was completed, cluster analysis was performed on all protein structures extracted from entire merged MD trajectories and the number of clusters was counted. If the number of clusters increases by less than 10% in successive 2 ns, it is considered that the convergence of MD simulations has reached. For example, the number of clusters based on 3000 protein structures was N3 when 3 ns simulation was completed, while it were N4 based on 4000 structures and N5 based on 5000 structures when 4 ns and 5 ns simulation was completed. If (N4 – N3)/N4 is less than 10% and (N5 – N4)/N5 is less than 10%, the simulation was regarded to converge.
Dynamics analysis with trajectory
A series of dynamic properties were analyzed based on equilibrium MD trajectory. In PTMdyna, the analysis of protein dynamics was multidimensional, including RMSD and Rg over time, RMSF and B-factor of each residue, secondary structure transition of each residue over time, major conformation change and motion nature, residue-wise dynamical cross-correlation, as well as atomic movement similarity. Through a comprehensive comparison of original and modified proteins in these properties, it is possible to track the influence of the PTM on protein dynamics.
RMSD was generally used in the analysis of MD trajectory to ensure the arrival of an equilibration period in which protein dynamics was more likely to exhibit an inherent pattern [65]. Rg indicated the degree of compactness and was often used to assess the folding states of protein [66]. RMSD and Rg values of each frame along MD trajectory were calculated using Cpptraj program [67]. RMSF was a measure of conformational variance and can highlight the portions of structure that fluctuated from their mean structure. RMSF value of each residue was computed with R package [68]. Similarly, B-factor described the attenuation of X-ray or neutron scattering, and could indicate the motion uncertainty of specific parts of the structure [69]. The calculated B-factor was positively correlated with RMSF. B-factor of each atom was computed with Cpptraj program [67]. The secondary structure in each frame was calculated using the DSSP algorithm [70] and their dynamic trend was represented using gnuplot program [71]. Based on atom atomic coordinates of each frame in MD trajectory, residue-wise cross-correlations coefficients were assessed and visualized to indicate regions that moved in a same or opposite direction. Additionally, residue-wise movement similarity matrix was calculated with GeoStaS algorithm [72] to identify rigid domains.
PCA [73, 74] was employed for different conformations sampled from MD trajectory using Bio3D package [75]. Along each of the first three principal components, the most dissimilar conformations were interpolated and assembled into multi-model PDB trajectories. Such trajectories were visualized through MolScript program [76] and PyMol [77] to provide insight into the nature of conformational differences and functional motion.
Free energy calculation
The solvation entropy (ΔSsol) was determined by the movement of water molecules to reduce their interactions with hydrophobic groups in protein. The conformational entropy (ΔSconf) was closely relevant to the change in the number of rotatable bonds during the binding process.
The ΔΔG value of all the equilibrium snapshots was averaged to obtain a final weighted value. A positive ΔΔG value shows a decrease in the binding affinity after occurring of specific PTM on the original protein–protein complex, while a negative value indicates an improved binding between proteins.
Hou et al.’s previous study [80] showed that the value of solute dielectric constant in MM-PB/GBSA method affects the accuracy of binding affinity prediction. In PTMdyna, the solute dielectric constant was automatically adjusted according to the number of charged residues in binding interfaces. The difference in computational solvent accessible surface area (ΔSASA) between complex-based and chain-only based states was used to define protein–protein interface residues with a cutoff value of 0.75. Three different solute dielectric constants (εin) were chosen flexibly according to the number of interfacial charged residue. εin = 1.0 was selected only when there was no charged interfacial residue. εin = 4.0 was selected when there are more than three charged interfacial residues and εin = 2.0 was selected in other cases.
Server configuration
PTMdyna protocol was implemented via a user-friendly freely available web server (http://ccbportal.com/PTMdyna). The web server was built on an Apache HTTP server running on a CentOS system. The webpage was achieved using PHP, and JavaScript. PTMdyna web server is compatible with most of the common web browsers without any plug-in installation, including Google Chrome, Mozilla Firefox, and Windows Edge. Jobs in the backend are managed by Maui and Torque. The Job information and task results are deposited in MySQL databases. NGL Viewer [81] is used to achieve molecular visualization, which runs with no additional plugins.
Knowing the influence of PTM on conformational dynamics is helpful to study how specific PTM work to fulfill related biological function, facilitating a further explanation of the related molecular mechanism and disease treatment.
We present PTMdyna, a server to visually predict the conformational dynamics differences between unmodified and modified proteins, thus indicating the influence of specific PTM.
Currently, PTMdyna contains 83 biologically relevant PTM types, and its outputs cover various dynamic properties and interaction analysis.
The predictive results of the case of HP1α-H3K9me complex produced by PTMdyna case can accord with or interpret the experimental data, suggesting the applicability of the server.
PTMdyna is free to access for the public without registration.
Data availability
The original crystal structures of proteins/protein-protein complexes were collected from RCSB Protein Data Bank (https://www.rcsb.rog/), and the information about post-translation modification sites were obtained from the literatures, see Supplementary Data available online.
Funding
The National Natural Science Foundation of China (21772059, 91853127, 31960548 and 21907036).
Xing-Xing Shi and Zhi-Zheng Wang are PhD students at College of Chemistry, Central China Normal University (CCNU), the direction of their thesis is structural bioinformatics.
Yu-Liang Wang and Guang-Yi Huang are Master students at College of Chemistry, Central China Normal University (CCNU). He is mainly engaged in bioinformatics and computational molecular simulation.
Jing-Fang Yang is postdoctoral associate at College of Chemistry of CCNU. She received his PhD in Pesticide Science from CCNU.
Fan Wang is an associate professor at College of Chemistry of CCNU. He received the PhD degree in Computational Chemistry from University of Amiens, France.
Ge-Fei Hao is a professor in Bioinformatics in Key Laboratory of Pesticide & Chemical Biologyand of CCNU and Key Laboratory of Green Pesticide and Agricultural Bioengineering of Guizhou University. He received his PhD in Pesticide Science from CCNU.
Guang-Fu Yang is Professor in Chemical Biology. He is the group leader and has been the Dean at College of Chemistry of CCNU. He received the PhD degree in Pesticide Science from Nankai University, Tianjin, China.
References
Author notes
Xing-Xing Shi and Zhi-Zheng Wang authors contributed equally to this work.