-
PDF
- Split View
-
Views
-
Cite
Cite
Song Zhang, Kuerbannisha Amahong, Chenyang Zhang, Fengcheng Li, Jianqing Gao, Yunqing Qiu, Feng Zhu, RNA–RNA interactions between SARS-CoV-2 and host benefit viral development and evolution during COVID-19 infection, Briefings in Bioinformatics, Volume 23, Issue 1, January 2022, bbab397, https://doi.org/10.1093/bib/bbab397
- Share Icon Share
Abstract
Some studies reported that genomic RNA of SARS-CoV-2 can absorb a few host miRNAs that regulate immune-related genes and then deprive their function. In this perspective, we conjecture that the absorption of the SARS-CoV-2 genome to host miRNAs is not a coincidence, which may be an indispensable approach leading to viral survival and development in host. In our study, we collected five datasets of miRNAs that were predicted to interact with the genome of SARS-CoV-2. The targets of these miRNAs in the five groups were consistently enriched immune-related pathways and virus-infectious diseases. Interestingly, the five datasets shared no one miRNA but their targets shared 168 genes. The signaling pathway enrichment of 168 shared targets implied an unbalanced immune response that the most of interleukin signaling pathways and none of the interferon signaling pathways were significantly different. Protein–protein interaction (PPI) network using the shared targets showed that PPI pairs, including IL6-IL6R, were related to the process of SARS-CoV-2 infection and pathogenesis. In addition, we found that SARS-CoV-2 absorption to host miRNA could benefit two popular mutant strains for more infectivity and pathogenicity. Conclusively, our results suggest that genomic RNA absorption to host miRNAs may be a vital approach by which SARS-CoV-2 disturbs the host immune system and infects host cells.
Introduction
Coronavirus disease 2019 (COVID-19) caused by the syndrome coronavirus 2 (SARS-CoV-2) has emerged and continuously affected people around the world for over one year. Up to the July of 2020, more than 198 million cases including 4 million deaths have been reported by Coronavirus research center of the Johns Hopkins University of Medicine [1]. Recently, mutated viruses with more powerful infectivity have appeared in some countries [2]. Therefore, although some emerging drugs indicated potential curative effect, it is urgent to exploit effective prevention and treatment strategies due to their clinical risks and viral evolution [2–6]. Imbalanced host response to SARS-CoV-2, low levels of interferons (IFNs) and increased expression of chemokines and interleukin (IL)-6, is a typical characteristic in COVID-19-infected patients [7]. Low levels of type IFN-I and IFN-III in patients have been incapable of suppressing the viral replication and subsequently increasing viruses forces the host excessive inflammatory response via employing chemokine- and IL-6-induced leukocytes [7]. The cytokine storm frequently occurs in COVID-19-infected individuals [8, 9], which leads to deterioration and even death [7].
MicroRNAs (miRNAs) are small non-coding RNAs that regulate gene expression [10] and were found to modulate diverse cellular processes such as differentiation, proliferation, apoptosis, host immunity and viral infection [11–15]. MiRNAs have been found to participate in regulation of DNA virus and RNA virus [15, 16]. Both DNA virus and RNA virus can produce miRNAs [16, 17]. Accumulating evidence has indicated that virus- and host-produced miRNAs play crucial roles in viral infection and replication during COVID-19 infection [18, 19]. Satyam et al. [20] reported six SARS-CoV-2 encoded miRNAs by bioinformatics prediction, which can target immune-related genes that are involved in TNF signaling and chemokine signaling pathways. Regulation of the immune pathways induced by SARS-CoV-2 produced miRNAs may be an important cause for the emergence of cytokine storms during COVID-19 infection [20]. In addition, a cluster of studies used computational approaches and acquired several lists of miRNAs that can interact with RNA genome or transcript of SARS-CoV-2 [18, 21–24]. Some targets of these miRNAs were related to immune-signaling pathways [18, 21, 24], metabolism and defense system [23], and age-related signaling and diseases [22]. Although these results seem to be well explained the regulatory role of miRNA for COVID-19 infection, few systematic analyses are to understand the mechanisms of how miRNAs modulate the occurrence and development of COVID-19 and which specific pathways are induced by miRNAs are involved in the process.
During virus infection, the virus is first uncoated and releases its genome into the cytoplasm of the host cell when the capsid is removed [25, 26]. Emerging evidence has shown that some miRNAs were related to COVID-19 development [18, 27]. For SARS-CoV-2, exposed genomic RNA in cytoplasm contributes to its interaction with intracellular small RNA molecules, especially miRNAs. To understand the role of host cellular miRNAs for the molecular mechanism of SARS-CoV-2 pathogenesis, we employed five datasets containing a series of miRNAs that were predicted to interact with the genome RNA of SARS-CoV-2 from 5 publications [18, 21–24]. Comprehensive databases and analysis tools have benefited the fields of multiple omics [28–34], biomolecule function prediction [35, 36], medical research [4–6] and drug design [37, 38]. Therefore, in this study, several tools are employed to analyze the data [39, 40]. These miRNAs were first identified their host target genes by miRTarBase [39], then the target genes were performed pathway and disease enrichment analysis by KOBAS [40]. Taken together, the results indicated that the miRNAs that were interacted with SARS-CoV-2 genomic RNA were closely related to the processes of viral infection and host immune response. Besides, the special RNA–RNA interaction mode can benefit the viral evolution for more infectivity and pathogenicity. Conclusively, this study provides novel insight that the genomic RNA absorbs the host functional miRNAs may explain how SARS-CoV-2 infects the host immune system.
Materials and methods
Extraction of host miRNAs targeted by SARS-CoV-2’s genome
Datasets are employed from five studies in PubMed database by searching for the keywords ‘COVID-19’, ‘SARS-CoV-2’ and ‘MicroRNAs’, ‘miRNA’. All miRNAs in five groups range from 100 to 800 in number are collected in these five independent datasets, which include human miRNAs that can be targeted by genomic RNA of the SARS-CoV-2.
Obtaining of targets of host miRNAs and functional enrichment analysis of target genes
There are also many miRNA-target interaction databases, which can be divided into two categories. Some of these databases, such as miRDB [41], mirDIP [42], miRbase [43] and miRPathDB [44], provided predicted interaction information. Some others databases (including miRTarBase [39], miRTargetLink [45] and DIANA-TarBase [46]) provided experimentally validated interaction information. As the most accurate and reliable data, the experimentally validated information is incorporated in this study. Among these experimentally validated databases, miRTarBase provides largest amount of data and therefore is used to obtain the targets of miRNAs in our work. Therefore, we selected miRTarBase for analysis the targets of miRNA in this study. Targets of host miRNAs were extracted by miRTarBase, in which only the strong experimentally validated-target genes were included (http://miRTarBase.cuhk.edu.cn/) [39]. Functional enrichment analysis of acquired target genes is performed by KOBAS 3.0 web-based tools (http://kobas.cbi.pku.edu.cn) [40] that cover different enriched results by a cluster of databanks including KEGG pathway [47], Reactome [48], BioCyc [49] and so on.
Protein–protein interaction analysis
STRING database (https://string-db.org/) [50] was employed to analyze protein–protein interactions (PPIs) among common target genes of these human miRNAs and profiled a sketch to visualize the interactions. We selected the highest confidence coefficient (>0.9) as standard in PPI network to obtain more credible interactions that play impact roles in COVID-19 [50].
Analysis of interaction patterns between mutated SARS-CoV-2 and human miRNAs
Interaction patterns of SARS-CoV-2 genome and human miRNAs were obtained by miRDB (http://mirdb.org/) [41], in which all interacting miRNAs and their binding sites are provided. We selected the miRNA that binds the mutated region of SARS-CoV-2 and to analyze the interaction pattern in wide-type and mutated status.
Five datasets that explored the information of human miRNAs interacted with SARS-CoV-2’s RNA genome
Datasets . | Contents (miRNA) . | Platform . | Isolate resources . | References . |
---|---|---|---|---|
Dataset 1 | 106 | IntaRNA v2.0; microRNA.org; psRNATarget; miRTarBase | 67 whole-genome sequences of SARS-CoV-2 isolates covering 24 different countries were retrieved from NCBI-Virus (2020) and Shu and McCauley (2017). | Front Genet. 2020, 11:765 [21] |
Dataset 2 | 873 | miRDB | Genomes of 29 SARS-CoV-2 isolates from different regions from the GenBank database. | Aging Dis. 2020, 11:509–522 [22] |
Dataset 3 | 479 | psRNATarget | The complete genome of SARS-CoV-2 isolates Wuhan-Hu-1 form GenBank MN908947.3. | PeerJ. 2020, 8:1–17 [23] |
Dataset 4 | 375 | multimiR | SARS-CoV-2 isolate from Huo Shen Shan Hospital of Wuhan during standard diagnostic tests. | Clin Transl Med. 2020, 10:1–18 [24] |
Dataset 5 | 462 | RNA22 v2 | The complete genome of SARS-CoV-2 isolates Wuhan-Hu-1 from GenBank NC_045512.2. | Am J Physiol Lung Cell Mol Physiol. 2020, 319:L444-L455 [18] |
Datasets . | Contents (miRNA) . | Platform . | Isolate resources . | References . |
---|---|---|---|---|
Dataset 1 | 106 | IntaRNA v2.0; microRNA.org; psRNATarget; miRTarBase | 67 whole-genome sequences of SARS-CoV-2 isolates covering 24 different countries were retrieved from NCBI-Virus (2020) and Shu and McCauley (2017). | Front Genet. 2020, 11:765 [21] |
Dataset 2 | 873 | miRDB | Genomes of 29 SARS-CoV-2 isolates from different regions from the GenBank database. | Aging Dis. 2020, 11:509–522 [22] |
Dataset 3 | 479 | psRNATarget | The complete genome of SARS-CoV-2 isolates Wuhan-Hu-1 form GenBank MN908947.3. | PeerJ. 2020, 8:1–17 [23] |
Dataset 4 | 375 | multimiR | SARS-CoV-2 isolate from Huo Shen Shan Hospital of Wuhan during standard diagnostic tests. | Clin Transl Med. 2020, 10:1–18 [24] |
Dataset 5 | 462 | RNA22 v2 | The complete genome of SARS-CoV-2 isolates Wuhan-Hu-1 from GenBank NC_045512.2. | Am J Physiol Lung Cell Mol Physiol. 2020, 319:L444-L455 [18] |
Five datasets that explored the information of human miRNAs interacted with SARS-CoV-2’s RNA genome
Datasets . | Contents (miRNA) . | Platform . | Isolate resources . | References . |
---|---|---|---|---|
Dataset 1 | 106 | IntaRNA v2.0; microRNA.org; psRNATarget; miRTarBase | 67 whole-genome sequences of SARS-CoV-2 isolates covering 24 different countries were retrieved from NCBI-Virus (2020) and Shu and McCauley (2017). | Front Genet. 2020, 11:765 [21] |
Dataset 2 | 873 | miRDB | Genomes of 29 SARS-CoV-2 isolates from different regions from the GenBank database. | Aging Dis. 2020, 11:509–522 [22] |
Dataset 3 | 479 | psRNATarget | The complete genome of SARS-CoV-2 isolates Wuhan-Hu-1 form GenBank MN908947.3. | PeerJ. 2020, 8:1–17 [23] |
Dataset 4 | 375 | multimiR | SARS-CoV-2 isolate from Huo Shen Shan Hospital of Wuhan during standard diagnostic tests. | Clin Transl Med. 2020, 10:1–18 [24] |
Dataset 5 | 462 | RNA22 v2 | The complete genome of SARS-CoV-2 isolates Wuhan-Hu-1 from GenBank NC_045512.2. | Am J Physiol Lung Cell Mol Physiol. 2020, 319:L444-L455 [18] |
Datasets . | Contents (miRNA) . | Platform . | Isolate resources . | References . |
---|---|---|---|---|
Dataset 1 | 106 | IntaRNA v2.0; microRNA.org; psRNATarget; miRTarBase | 67 whole-genome sequences of SARS-CoV-2 isolates covering 24 different countries were retrieved from NCBI-Virus (2020) and Shu and McCauley (2017). | Front Genet. 2020, 11:765 [21] |
Dataset 2 | 873 | miRDB | Genomes of 29 SARS-CoV-2 isolates from different regions from the GenBank database. | Aging Dis. 2020, 11:509–522 [22] |
Dataset 3 | 479 | psRNATarget | The complete genome of SARS-CoV-2 isolates Wuhan-Hu-1 form GenBank MN908947.3. | PeerJ. 2020, 8:1–17 [23] |
Dataset 4 | 375 | multimiR | SARS-CoV-2 isolate from Huo Shen Shan Hospital of Wuhan during standard diagnostic tests. | Clin Transl Med. 2020, 10:1–18 [24] |
Dataset 5 | 462 | RNA22 v2 | The complete genome of SARS-CoV-2 isolates Wuhan-Hu-1 from GenBank NC_045512.2. | Am J Physiol Lung Cell Mol Physiol. 2020, 319:L444-L455 [18] |
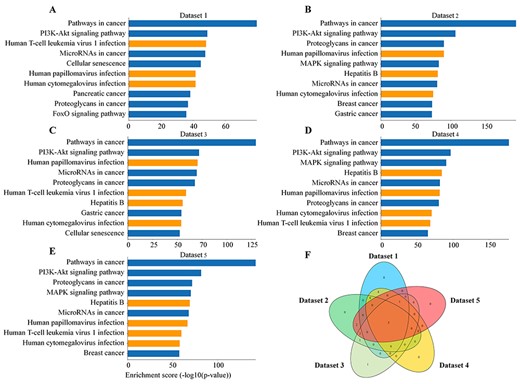
Enrichment analysis of KEGG pathways in five datasets. (A–E) The bar graphs show top-ten pathways of enrichment using targets of selected host miRNA that interacted with SARS-CoV-2 in five datasets according to Enrichment score (−log10 (P-value)). The orange bars represent virus-related diseases. (F) The Venn diagram shows the common and unique pathways in five datasets.
Results
Host miRNAs interacted with SARS-CoV-2 may induce and involve immune-related pathways
A recent study reported that some miRNAs, responsible for immune-related gene expressions, such as miR-34c-5p and miR-34a-5, could interact with the genome of SARS-CoV-2 and be deprived of their function to paralyze host immune system and facilitate viral development [18]. Therefore, we collected five miRNA datasets that genome of SARS-CoV-2 was predicted to interact with using different tools or algorithms in some studies (Table 1). The targets of all miRNAs in the five groups were first identified, which excluded those identified by high-throughput analysis in miRTarBase [39]. KOBAS is a comprehensive web server for integrated annotation and enrichment of pathways and diseases [40], which is employed to perform pathway enrichment analysis of these targets. First, the results of KEGG pathways [47] enrichment analysis showed that the miRNAs (or their targets) were related to some viral infections, including hepatitis B, human papillomavirus infection, human cytomegalovirus infection and human T-cell leukemia virus 1 infection in the top ten lists (Figure 1A–F). In addition, epstein–barr virus infection, herpes simplex virus 1 infection, human immunodeficiency virus 1 infection, human papillomavirus infection and kaposi sarcoma-associated herpesvirus infection had also great differences (Supplementary Table 1, see Supplementary Data available online at http://bib.oxfordjournals.org/). The results indicated that many of the miRNAs might be involved in process of virus infections. Next, Reactome pathways [48] enrichment analysis in five datasets captured three immune-related pathways in the top-ten list, which included immune system, signaling by interleukins and cytokine signaling in immune system (Supplementary Figure 1A–F, see Supplementary Data available online at http://bib.oxfordjournals.org/). We further checked all immune-related pathways and interleukin signaling pathways from enrichment results and found more than half of them had statistical significance (Supplementary Table 2, see Supplementary Data available online at http://bib.oxfordjournals.org/). Therefore, it can be deduced that SARS-CoV-2 employed host miRNA to affect host immune system-related pathways and the process of virus infection.
Host miRNAs interacted with SARS-CoV-2 might be related to cell metabolism pathways
Metabolic abnormalities, including glycolysis, gluconeogenesis and lipid metabolism, have been found in plasma or serum samples of COVID-19 patients [51, 52]. Followingly, we considered metabolic pathways regulated by host miRNAs during SARS-CoV-2 infection. The target genes in five groups of miRNAs were enriched in BioCyc database [49] and resulted in some datasets that can find glycolysis and gluconeogenesis in the top-ten list (Supplementary Figure 2A–E, see Supplementary Data available online at http://bib.oxfordjournals.org/). Overall, the five datasets shared three metabolic pathways on their top-ten lists, 3-phosphoinositide pathway, bone morphogenetic protein 2 (BMP2) and inositol pyrophosphates (IPPs) pathway (Supplementary Figure 2F, see Supplementary Data available online at http://bib.oxfordjournals.org/). Moreover, the three metabolic pathways have been reported to relate to virus-linked processes of immune-related pathways. Gain-of-function mutations in phosphatidylinositol 3-kinases (a member of 3-phosphoinositide pathway) catalytic subunit p110δ can lead to T cell senescence and human immunodeficiency in virus infection [53]. BMPs are dysregulated and play a crucial role in autoimmune disorders [54, 55]. IPPs pathways are major regulators of neutrophil function, which are critical players in innate immunity and host defense in infection and inflammation [56].
Host miRNAs interacted with SARS-CoV-2 are involved in some signaling pathways
Patients with COVID-19 have confirmed remarkable dysregulation of a series of signaling pathways, such as hyperactivation of IL-6 signaling pathway [7], JAK–STAT signaling pathway [57], silent type IFN signaling pathway [58] and so on. The interplay of crosslinked signaling pathways leads to the patients’ torture and even death. However, we wonder which signaling pathways are caused by host miRNAs interacted with SARS-CoV-2. Therefore, enriched analysis of these miRNAs was made using PANTHER pathway database [59], in which we listed top-ten pathways (Supplementary Figure 3A–E, see Supplementary Data available online at http://bib.oxfordjournals.org/) and also found that the five datasets shared three pathways, including CCKR signaling map, angiogenesis and apoptosis signaling pathway (Supplementary Figure 3F, see Supplementary Data available online at http://bib.oxfordjournals.org/). The obtained three pathways have been reported to be closely related to the immune system or virus infections. CCKR antagonists can enhance the efficacy of immune checkpoint antibody therapy in mice models [60]. Patients with COVID-19 presented a marked new vessel growth by intussusceptive angiogenesis in lung [61]. SARS-CoV-2-induced inflammatory responses including innate and adaptive immune responses can suppress lymphopoiesis and promote lymphocyte apoptosis [62].
Some related diseases are enriched by host miRNAs interacted with SARS-CoV-2
A study among 355 COVID-19 patients who died reported that only 0.8% of patients had no diseases, 25.1% had a single disease, 25.6% had 2 diseases and 48.5% had 3 or more potential diseases [63]. The most common diseases that were generally accompanied by COVID-19 are cancer, cardiovascular disease, diabetes, chronic respiratory disease and hypertension combine [64, 65]. Thus, the death rate in COVID-19 cases with these potential diseases was higher compared with the general population [66]. To get further insight into the diseases that the miRNAs may be involved in, KEGG DISEASE, a database that provided the function of enrichment for a series of diseases [67], therefore, was employed to analyze diseases that miRNAs potentially resulted in. The enrichment of the miRNAs’ target genes in all datasets is shown in the top-ten lists (Supplementary Figure 4A–E, see Supplementary Data available online at http://bib.oxfordjournals.org/) and the five datasets shared five types of cancer-related diseases including cancers, cancers of the digestive system, cancers of the breast and female genital organs, cancers of eye, brain and central nervous system as well as cancers of haematopoietic and lymphoid tissues (Supplementary Figure 4F, see Supplementary Data available online at http://bib.oxfordjournals.org/). Overall, the results indicated that each group of miRNAs in the datasets was important for various cancers in COVID-19 patients. A case study reported that patients with cancer were faced with higher risks of COVID-19 infection and had poorer outcomes than persons without cancers when suffering from COVID-19 [68]. We further investigated more diseases that miRNAs could potentially relate to by OMIM [69] and NHGRI GWAS Catalog [70] human disease databases. Results of disease enrichment included breast cancer, prostate cancer, type 2 diabetes, colorectal cancer, lung cancer, inflammatory bowel disease and rheumatoid arthritis, leukemia, acute myeloid and so on (Supplementary Figures 5 and 6, see Supplementary Data available online at http://bib.oxfordjournals.org/). Our results indicated that the existence of these host miRNA-related comorbidities may increase the risk of COVID-19 infection and morbidity of lung injury.
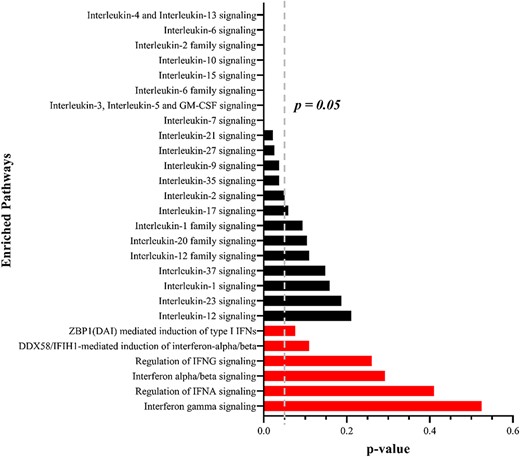
Pathway enrichment analysis by the shared target of host miRNAs. The black bars represent IL signaling pathways and the red bars represent IFN signaling pathways. P < 0.05 means a significant difference.
Different miRNAs regulated same COVID-19-related genes in five datasets
The enrichment results in five datasets shared some common pathways or diseases by diverse databases. We considered whether these datasets shared many miRNAs to regulate these common pathways. Therefore, to verify the reliability of these results, a Venn diagram was conducted to show the common miRNAs in five datasets. Interestingly, the five datasets shared no miRNAs and remained their unique miRNAs (dataset 1: 3, dataset 2: 453, dataset 3: 151, dataset 4: 147, dataset 5: 181) (Supplementary Figure 7A, see Supplementary Data available online at http://bib.oxfordjournals.org/). Due to different algorithms employed in these studies, we speculated that each of algorithms is limited to miRNAs of prediction. Given that a gene generally can be regulated by a list of miRNAs, therefore, we attempt to explore whether each of groups of miRNAs in five datasets executes a similar function for COVID-19 infection. We obtained target genes of these miRNAs from miRTarBase [39], which provided a large amount of experiment-validated miRNA-target interactions, and to acquire credible analysis, the high-throughput interactions are excluded (Supplementary Table 3, see Supplementary Data available online at http://bib.oxfordjournals.org/). The result showed targets of the five datasets shared 168 genes (Supplementary Figure 7B, see Supplementary Data available online at http://bib.oxfordjournals.org/, Supplementary Table 3, see Supplementary Data available online at http://bib.oxfordjournals.org/), including some proteins that were reported to be crucial regulators for COVID-19, such as IL6, IL6R, mTOR, AKT1 and so on.
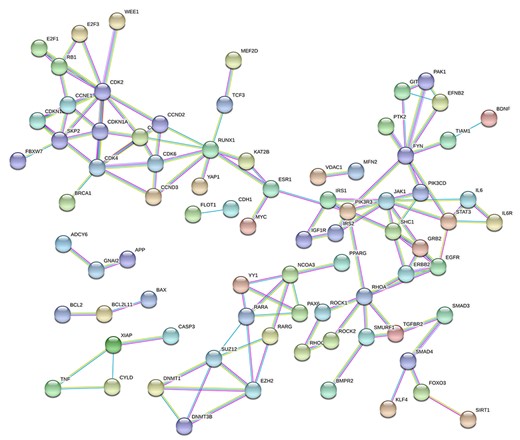
PPI network of the common genes in five datasets. The protein–protein network was set to the level of confidence score > 0.9 (highest confidence in STRING).
SARS-CoV-2 genome preference for miRNAs of interleukins genes not interferon genes
A ground-breaking study reported that COVID-19 patients generally had an imbalanced host response to SARS-CoV-2 that also can drive the development of COVID-19, which typically consisted of IL-6 signaling and silent IFN signaling [7]. The emergence of increasing leukocytes triggers not only a substantial response to the virus but also an inevitable attack of the host itself [7]. Suffering attacks from both the virus and leukocytes, patients may develop severe respiratory tract injuries such as respiratory distress syndrome and even death [7]. However, it is not understood why the imbalanced immune response emerges in COVID-19 patients. To get further insight, we used 168 common targets that were subjected to pathway enrichment analysis by KOBAS [40], in which we filtered the pathways of IL signaling and IFN signaling. Interestingly, the results suggested that more than half of the IL signaling pathways and none of the IFN signaling pathways were significantly different (Figure 2). It may reveal that an imbalanced host response in COVID-19 patients, such as excessive IL signaling and weak IFN signaling, was caused by viral genome absorption to the host miRNAs that bound and inhibited IL gene expression, therefore leading to the uncontrolled release of leukocytes.
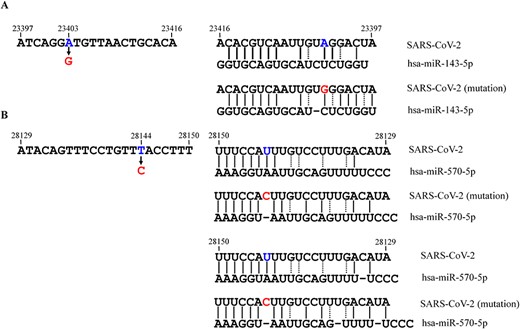
Binding details between the RNA genome of SARS-CoV-2 (in two popular mutated sites) and host miRNAs. The possible mode complementary base pairing between the regions that (A) covered the 23 403 site (gene) in wide-type and mutated strain and hsa-miR-143-5p and (B) covered 28 144 site (gene) in wide-type and mutated strain and hsa-miR-570-5p. The solid and dashed lines mean common base pairs (A-T and C-G) and wobble base pairs (G-U), respectively.
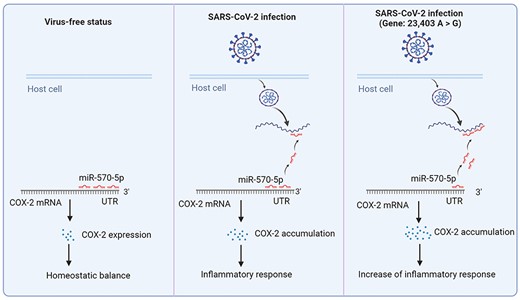
The mechanism how miRNA affect the process of virus infection in mutated SARS-CoV-2 infection. In virus-free status, miR-570-5p binding to the 3'-UTR of COX-2 mRNA leads to COX-2 normal expression and homeostatic balance in host cells; during SARS-CoV-2 infection, the virus releases its genome in host cells to captain intracellular miR-570-5p, which results in the decrease of the miRNA in COX-2 mRNA 3'-UTR and triggers to COX-2 accumulation-induced inflammatory response in lungs; during mutated SARS-CoV-2 (Gene: 23,403 A > G) infection, due to the stronger interactions between viral genome and miR-570-5p, it is followingly reduction of interactions, excessive COX-2 accumulation and enhance of inflammatory response in lungs which may cause more severe symptoms in patients.
PPI network construction using the shared miRNA in five datasets
We next performed PPI analysis on 168 shared genes in five datasets through the STRING web servers [50], in which the protein pairs were identified with the confidence coefficient > 0.9. Furthermore, 79 nodes and 123 edges are included in the formation of the PPI network in Figure 3. In this PPI network, IL6-IL6R has been included in the network that has been reported as potential indicators and therapeutic targets [71, 72]. And some of their inhibitors have been in clinical trials [73, 74]. Our results also suggested that many cell-cycle-related proteins (such as some CCNEs and CDKs), cell apoptosis-related proteins (such as TNF, XIAP and CASP3 as well as BCL2, BCL2L11 and BAX), insulin-related proteins (such as IGF1R, IRS1 and IRS2), and so on were important compartments in the network (Figure 3). Critically, host cell cycle arrest could be induced during coronavirus infection and serves for the viral survival and replication [75]; increasing lymphocyte apoptosis is a typical feature in the early phase of COVID-19 patients [62]; IGF-1 and IGF-1R in lung tissues were reported to increase in patients with fibroproliferative acute respiratory distress syndrome [76].
Interaction between host miRNA and viral genome benefits the evolution of SARS-CoV-2
Thus far, SARS-CoV-2 evolution has led to over 20 000 identified mutations, and these variants might enhance the infectivity and pathogenicity of the virus, for example by enhancing the ability to bind to S protein [2]. Herein, we raise a viewpoint that a mutation can affect the ratios of complementary base pairing between host miRNA and viral genome (enhance/weaken their interactions), in some cases, thus increasing immune- or virus-related proteins to enhance infectivity and pathogenicity of the virus. Notably, D614G (gene: 23,403 A > G) is one of the earliest S protein mutations that was with high transmissibility and led to higher viral loads in upper respiratory tract [77]; L84S of ORF8 (gene: 28,144 T > C) have shown a derived frequency of ≥70% across the SARS-CoV2 strains [78]. Using miRDB, a tool of miRNA target prediction, we found hsa-miR-143-5p (Figure 4A) and hsa-miR-570-5p (Figure 4B) can bind the two regions that covered A23403G and T28144C mutation sites, respectively. For these two mutations, we found more powerful binding force in a mutated mode compared with the wide-type mode (Figure 4A and B). For the T28144C mutation, two possible binding sites were provided to describe the interactions between the mutated genome and hsa-miR-570-5p (Figure 4B). Increased interactions between miRNAs and viral genome will lead to the dissociation of the miRNAs from their initial target mRNAs. In other words, the results showed that the two regions that covered A23403G and T28144C mutation sites had more powerful adsorption capacity to hsa-miR-143-5p and hsa-miR-570-5p than the wide-type regions, thus activating the expression of the miRNAs-related genes. Two studies reported that miR-143 could bind and downregulate cyclooxygenase-2 (COX-2) [79, 80]. Moreover, COX-2 has been reported to be highly expressed in SARS-CoV-2-infected individuals and was involved in lung inflammation observed in COVID-19 patients [81]. Therefore, the mutated SARS-CoV-2 (gene: 23,403 A > G) binding to hsa-miR-143-5p resulted in more severe inflammatory response in lungs due to mediating the reduction of hsa-miR-143-5p inhibition to COX-2 mRNA (Figure 5). Another study reported that miR-570 could bind and downregulate PD-L1 [82]. PD-L1 expression was found higher in SARS-CoV-2-infected epithelial cells [83] and PD-1/PD-L1 checkpoint inhibition would be a potential strategy to counteract cytokine storm in COVID-19 patients [84]. Therefore, in this sense, thousands of mutations in SARS-CoV-2 may evolve more adaptable strains using this way that benefit their survival and spread.
Discussion
COVID-19 has been affected lives and health of people around the world for more than one year and has so far caused 10 million deaths. Some timely studies and policies have also partially mitigated the effects of COVID-19, with a series of therapeutics and tools to prevent and resist COVID-19. Now, a mass of studies has been uncovered how the coronaviruses infect, hurt and kill the host from different perspectives. For example, in May 2020, Blanco-Melo et al. [7] identified that imbalanced host immune response to SARS-CoV-2 is the defining and driving features of COVID-19. Low levels of interferons juxtaposed to high expression interleukin-6 and chemokines were found in COVID-19 individuals, excessive interleukins recruited plenty of leukocytes to defend the virus in lungs [7]. A fierce battle of leukocytes in the lungs eventually also spread to somatic cells. However, little is known what leads to silence of interleukins and activation of interleukins when SARS-CoV-2 infects the host cell.
Several studies reported that some host functional miRNA can be absorbed by the RNA genome of SARS-CoV-2, which may cause the dysregulation of some immune-related genes and facilitate the process of viral infection [18, 85]. Based on these findings, we suppose whether RNA genome of SARS-CoV-2 regulates disorder of host immune system by large-scale manipulation of host functional miRNAs or not. Now, bioinformatics has promoted the development of the field of omics studies and feature selection [28–30, 86]. We employed five datasets and bioinformatic tools and then identified that target genes of these miRNAs are involved in many immune-related signaling pathways and viral infectious diseases. Moreover, the enriched cell metabolic pathways and other diseases are also accorded with promotion of the virus infectious development. More importantly, we found these five groups shared no common miRNAs but these miRNAs shared 168 common target genes. Pathway enrichment analysis of these genes indicated excessive IL signaling and weak IFN signaling. Now, some therapies about interferons and IL-6 inhibitors have been in clinical trials [73, 74, 87, 88]. PPI network analysis also identified that RNA genome of SARS-CoV-2 can regulate IL6-IL6R, cell cycle-related proteins, apoptosis-related proteins and growth factor-related proteins to coordinate a series of processes of viral infection. Interestingly, our analysis also found that SARS-CoV-2 is evolved to more contagious and pathogenic mutations by regulating virus-related genes in the same way, such as A23403G and T28144C mutations. Therefore, in this study, we report that interaction between host miRNA and SARS-CoV-2 genome is a crucial approach for viral survival and evolution.
Host miRNAs that can interact with genomic RNA of SARS-CoV-2 were involved in immune-related pathways and virus-infectious diseases by enrichment analysis.
The function of host miRNAs was deprived by absorption of genomic RNA of SARS-CoV-2, which led to imbalanced immune responses featured by activated interleukin signaling pathways and silence interferon signaling pathways.
The RNA–RNA interactions benefited the evolution of SARS-CoV-2 in two popular mutated strains, which probably led to the virus with higher infectivity and transmissibility.
Data availability
Lists of miRNAs are collected from five independent studies which are available in PubMed, and the targets of miRNAs are obtained from miRTarBase as Supplementary Table 3, see Supplementary Data available online at http://bib.oxfordjournals.org/.
Funding
National Natural Science Foundation of China (81971982, 81872798, U1909208); Key R&D Program of Zhejiang Province (2020C03123, 2020C03010); Natural Science Foundation of Zhejiang Province (LR21H300001); Leading Talent of the ‘Ten Thousand Plan’ - National High-Level Talents Special Support Plan of China; Fundamental Research Fund for Central Universities (2018QNA7023); ‘Double Top-Class’ University Project (181201*194232101); Alibaba-Zhejiang University Joint Research Center of Future Digital Healthcare; Alibaba Cloud; Information Technology Center of Zhejiang University.
Song Zhang is a PhD student in the College of Pharmaceutical Sciences in Zhejiang University, and the First Affiliated Hospital of Zhejiang University School of Medicine, China. He is interested in bioinformatics and molecular biology.
Kuerbannisha Amahong, Chenyang Zhang and Fengcheng Li are PhD/MD students of the College of Pharmaceutical Sciences in Zhejiang University, China. They are interested in bioinformatics and molecular biology.
Jianqing Gao is a Professor of the College of Pharmaceutical Sciences in Zhejiang University, China. He is interested in drug delivery and molecular biology.
Yunqing Qiu is a Professor of First Affiliated Hospital in Zhejiang University, China. He is interested in precision medicine, diagnosis and treatment of liver disease and system biology.
Feng Zhu is a Professor of the College of Pharmaceutical Sciences in Zhejiang University, China. His research laboratory (https://idrblab.org/) has been working in the fields of bioinformatics, OMIC-based drug discovery, system biology and medicinal chemistry.
References
Author notes
Song Zhang, Kuerbannisha Amahong and Chenyang Zhang authors contributed equally to this work.