-
PDF
- Split View
-
Views
-
Cite
Cite
Masood ur Rehman Kayani, Wanqiu Huang, Ru Feng, Lei Chen, Genome-resolved metagenomics using environmental and clinical samples, Briefings in Bioinformatics, Volume 22, Issue 5, September 2021, bbab030, https://doi.org/10.1093/bib/bbab030
- Share Icon Share
Abstract
Recent advances in high-throughput sequencing technologies and computational methods have added a new dimension to metagenomic data analysis i.e. genome-resolved metagenomics. In general terms, it refers to the recovery of draft or high-quality microbial genomes and their taxonomic classification and functional annotation. In recent years, several studies have utilized the genome-resolved metagenome analysis approach and identified previously unknown microbial species from human and environmental metagenomes. In this review, we describe genome-resolved metagenome analysis as a series of four necessary steps: (i) preprocessing of the sequencing reads, (ii) de novo metagenome assembly, (iii) genome binning and (iv) taxonomic and functional analysis of the recovered genomes. For each of these four steps, we discuss the most commonly used tools and the currently available pipelines to guide the scientific community in the recovery and subsequent analyses of genomes from any metagenome sample. Furthermore, we also discuss the tools required for validation of assembly quality as well as for improving quality of the recovered genomes. We also highlight the currently available pipelines that can be used to automate the whole analysis without having advanced bioinformatics knowledge. Finally, we will highlight the most widely adapted and actively maintained tools and pipelines that can be helpful to the scientific community in decision making before they commence the analysis.
Introduction
Shotgun metagenomics is one of the well-known applications of high-throughput sequencing that has enabled culture-independent genomic analysis of microbes directly from the collected samples [1]. In its first 15 years, metagenomics unveiled community composition and functional potential (collectively termed as microbiome) of various environmental settings. Human microbiome project [2], global ocean microbiome [3], identification of antibiotic resistance genes from the human gut [4] and association of gut microbiome with health and disease [5–7] are some of the major discoveries enabled by high-throughput sequencing. These studies mostly performed de novo assembly of contigs using the sequencing reads, followed by prediction of genes for taxonomic and functional annotations. Although metagenome assembly is considered computationally intensive, it provides better and precise taxonomic and metabolic inferences [8, 9].
In recent years, improved computational resources have significantly aided in the development of highly optimized and memory-efficient de novo assembly algorithms [10, 11]. These developments have been essential for the emergence of methods used for in silico reconstruction of microbial genomes from the assemblage of environmental samples. Since majority of the microbes are uncultivable [12], these ‘genome-resolved metagenome’ analyses allow bypassing this bottleneck and greatly expand microbial representatives in the reference genome database. In this avenue, the first-ever set of genomes was reconstructed in 2004 from an acid mine drainage that contained low microbial diversity [13]. Early success of genome-resolved metagenomics remained limited due to low sequencing depth of metagenomes [14, 15]. However, refined sequencing quality and decreasing costs have facilitated the generation of metagenomes with higher sequencing depths. With this, genome-resolved metagenome analyses became applicable to communities with relatively higher microbial complexities [16–18].
The application of genome-resolved metagenome analysis has now expanded massively and has been successfully utilized for metagenomes with differential microbial complexities (high, medium and low microbial diversities), low sequencing coverage or metagenomes containing strain-level variations. These efforts involve recovery of genomes from human gut microbiome [19], cow rumen [20–22], global oceans [23, 24], permafrost [25], biogas plants [26] and other environments [27]. In the last 2 years, several studies have performed genome-resolved metagenome analyses at an unparalleled scale: by recruiting thousands of publically-available metagenomes in a single study. Most notable examples include the recovery of thousands of genomes from human microbiome [28–30], environmental metagenomes and non-human gastrointestinal tracts [31], and the establishment of genomic catalogue of Earth’s microbiome [32]. These studies have provided first genome representatives of several uncultivable microbes and insights into previously unexplored metabolic potential of the microbes [31, 33]. Therefore, genome-resolved metagenome analyses offer a better exploitation of the metagenomic data to comprehensively understand microbial adaptation and association with different environments and hosts.
In this review, we discuss genome-resolved metagenome analysis as a series of four steps: (i) preprocessing of the sequencing reads, (ii) de novo assembly of the metagenomic data, (iii) genome binning and (iv) taxonomic and functional annotation of the reconstructed genomes (Figure 1). Preprocessing of the sequencing reads involves removal of poor-quality bases from the reads and adapter contamination whereas de novo metagenome assembly generally refers to joining these high-quality short reads into longer fragments or contigs. Genome binning can be defined as the process of identifying contigs corresponding to same organism and clustering them into groups or ‘genome bins’. Lastly, the taxonomic and functional analysis involves determining the taxonomic affiliation of the recovered genome, prediction of its genes and potential functions. Genome binning is not only the most critical step of genome-resolved metagenome analysis but also a difficult task to achieve computationally. This is largely due to the high sequence similarities between species and strain-level variations in the metagenome. Numerous computational resources have been developed to carry out genome binning and each of the other three steps, making the choice of the right tool slightly difficult. We discuss these currently available tools and methods for the above mentioned four stages and recommend best strategies for the genome-resolved metagenome analyses.
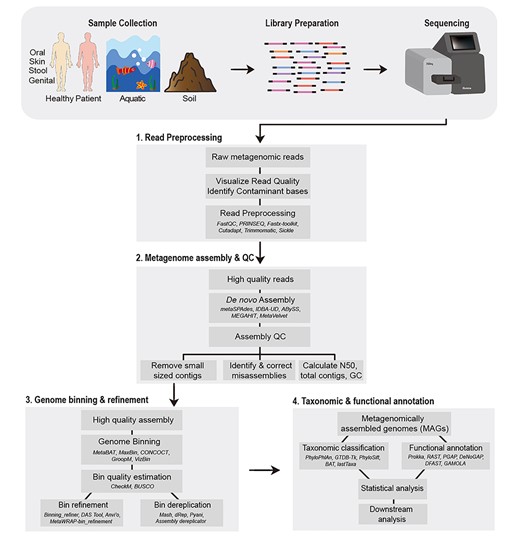
Schematic representation of the workflow for genome-resolved metagenomics analysis. The typical genome-resolved analysis of metagenomes, obtained through any source, typically involves four steps i.e. (1) read preprocessing, (2) metagenome assembly and QC, (3) genome binning and refinement and (4) taxonomic and functional annotation of the recovered MAGs. In the figure, examples of several tools for each of the steps is also provided (names italicized).
Preprocessing of the sequencing reads
The raw sequencing reads are typically accompanied by the quality scores (generally referred as PHRED Score or Q) in the FASTQ format [34]. Q is the representative of the probability of an incorrect base call by the sequencer [34]. In Illumina sequencing systems, Q10 represents base call accuracy of 90% and probability of incorrect base call of 1 in 10 whereas Q30 indicates 99.9% accuracy and probability of incorrect base call of 1 in 1000 [35]. The base call errors and insertion/deletions can arise in the sequencing data due to the digital nature of sequencing platforms [36, 37]. In addition, adapter sequences can also be present in the reads, which is attributed to the ligation of adapters to inserts during the preparation of sequencing library. Read duplication may also occur due to the emulsion polymerase chain reaction during library preparation or through optical duplicates [38, 39]. These problems in the raw sequences can easily become the source of suboptimal or erroneous results [40]. Therefore, the removal and trimming (generally referred as quality control or QC) of the problematic sequences may become necessary.
Fabbro et al. [40] performed extensive evaluation of different trimming algorithms and their effect on different types of datasets and analysis. Their results indicated that removal of low-quality portions of sequencing data not only improved genome assembly and variant calling but also reduced the execution time and the required computational resources. Recently, ~290–400% increase in computational time was observed when using trimmed reads for genome assembly [41]. However, the percentage of the assembled genome and the predicted number of genes did not significantly differ between raw and trimmed datasets [41]. Luo et al. [42] generated in silico metagenomes by spiking a publically available metagenome with Escherichia sp. strain TW10509 genomic reads [43]. Genes predicted from the recovered E. coli genome from this metagenome indicated presence of sequencing errors that led to the truncation of protein products or frameshift mutations [43]. Such gene products can lead to incorrect metabolic inferences. In genome-resolved metagenome analysis, trimming of the raw reads can be extremely useful even if it only reduces the computational time for metagenome assembly and not the overall quality of the recovered genomes. Therefore, it is highly recommended to carefully evaluate quality of the raw reads and perform QC accordingly before proceeding downstream to generate metagenome assembly and genome bins.
Visualization of the raw read quality scores is an effective way to overview the sequencing quality that enables inference of suitable trimming thresholds for base Q score, number of ambiguous bases per read (symbolized using N in the reads), minimum length of read and identification of adapter contaminated reads. Reads failing to meet these thresholds can then be discarded and adapters can be cut from reads using numerous different tools. FastQC [44] and PRINSEQ [45] can provide visual overview of the sequence quality and adapter contamination in the reads. Fastx-Toolkit [46] can also be used as an alternate to generate sequence quality and nucleotide distribution statistics. Both PRINSEQ and Fastx-Toolkit can also serve as the tools for QC. In addition to these, QC can be performed using Cutadapt [47], Trimmomatic [48], AdapterRemoval [49, 50], SOAPnuke [51], AlienTrimmer [52], Fastp [53], BBDuk [54] and multiple other tools [55–70] (Table 1). Most of these tools demonstrate high specificity and sensitivity for trimming and adapter removal. For instance, Cutadapt, Trimmomatic and AdapterRemoval exhibit sensitivity and specificity of approximately 0.999 when trimming paired-end data. Similarly, Trimmomatic shows better QC of the datasets contaminated with multiple adapters in contrast with several other tools [49].
Most commonly used tools for the evaluating the quality and preprocessing of raw metagenomics reads
Most commonly used tools for the evaluating the quality and preprocessing of raw metagenomics reads
Although, both Cutadapt and Trimmomatic have been widely adapted for QC, they require manual tweaking of the parameters e.g. the adapter sequences have to be provided manually. This can become cumbersome under certain scenarios, for instance, if no prior information about adapters is available. Another major limitation of Cutadapt and Trimmomatic is their inability to handle multiple datasets. TrimGalore [71], a wrapper script around FastQC and Cutadapt, can be highly useful in such scenarios as it can automate read QC of multiple datasets, estimate the possible adapter sequence and adjust the trimming parameters accordingly. Majority of these tools are restricted to utilize only the maximum number of processors in one computer server. However, this could only become a huge limitation if the datasets are too large and require extremely high-throughput performance [51]. In such cases, SOAPnuke, one of the recently developed tools, could be highly effective as it allows processing of the data through multiple working nodes for parallel computing. SOAPnuke has demonstrated ~5.37 times faster operation than tools including Trimmomatic and AlienTrimmer, without compromising the accuracy [51]. Fastp is another ultrafast all-in-one FASTQ preprocessor that is 2–5 times faster than majority of the above mentioned tools, including SOAPnuke, while maintaining high accuracy [53]. These benchmarking statistics are largely available through the original articles of the respective tools. Therefore, an unbiased and independent benchmarking will better demonstrate the best among these tools for QC.
In addition, metagenomes obtained from a host such as human or mice, may be contaminated with reads corresponding to the host’s genome. For such samples, it is necessary to perform removal of host reads, not only to avoid suboptimal results but also to ensure subject privacy (especially for human host). To this end, raw reads could be mapped to the host genome using any read alignment tool, e.g. Bowtie2 [72], and the mapped reads can be identified and removed. DeconSeq [73] and BMTagger [74] have specially been developed to achieve removal of host reads. However, DeconSeq suffers from high error rate whereas BMTagger requires large and complex indexing of the reference genome, thus making Bowtie2 a better choice [75].
De novo metagenomic assembly
For obtaining genomes or full-length coding sequences of the microbes (especially uncultured microbes), the short reads must be ‘assembled’ into contigs. Metagenome assembly is computationally much more challenging due to the presence of highly similar sequences (e.g. strains) in the investigated community. These challenges also include presence of microbes in heterogeneous abundance, conserved sequences of coexisting microbes or repetitive DNA from the same genomes. The quality of metagenome assembly can be greatly hampered by these issues and result in the production of highly fragmented and misassembled contigs [76–80]. Recently developed metagenome assemblers tend to address these problems and offer larger contig sizes (greater N50 values), improved gene prediction and lower assembly errors [10, 11, 81–83].
Current metagenome assemblers are based on two different types of approaches i.e. (i) overlap, layout, consensus (OLC) assembly and (ii) de Bruijn graph (dBg) assembly. OLC assembly involves identification of overlap between the reads and construction of overlap graph, using the overlap information, reads are then formatted as contigs, followed by construction of consensus sequence of the contigs. In contrast, dBg assemblers involve identification of subsequences of length k (termed k-mers). The k-mers are overlapping sequences and represent the vertices in the dBg while the overlapping k-mers are connected through edges. Furthermore, the count of each k-mer is also maintained. Lastly, the assembler walks through the edges of dBg and constructs contig sequences. Both methods have several advantages and disadvantages and have been described in details elsewhere [84–86]. Here we will discuss several popular OLC- and dBg-based assemblers.
OLC-based assemblers
One of the first developed metagenome assemblers, Genovo [87, 88], utilized the OLC strategy. Genovo has been instrumental in recovering several viral and bacterial genomes from human gut metagenomic data [79, 89]. MAP [90] and Omega [91] are two other OLC-based assemblers that use paired-end information for metagenomic assembly. Viral quasispecies, which generally represent the diverse pool of viral species, could be assembled using a specifically designed tool i.e. SAVAGE [92], that shows superior ability to recover viral quasispecies in contrast with other related assemblers. Furthermore, it also provides an option to carry out reference-guided assembly [92]. Similarly, the iterative virus assembler (IVA) was designed for specially to assemble RNA viruses [93] and has been used for the assembly of Zika virus and H1N1 influenza virus assemblies [94, 95]. Both SAVAGE and IVA are capable of handling the variations in sequencing depth that is a major concern during the de novo metagenomic assembly.
dBg-based assemblers
In contrast with the OLC-based assemblers, the dBg-based assemblers are currently more popular. However, these assemblers require parameter declaring k-mer of specific length to be used for assembly, which can have significant impact on the assembly quality. For instance, smaller k can result in high occurrence of repetitive k-mers, and poor and unreliable quality of the assembled contigs [96]. Meta-IDBA [97], and its extension IDBA-UD [83], utilize multiple k-mers during assembly to tackle problems associated with using suboptimal k values. IBDA-UD is highly efficient while dealing with the uneven sequencing depth in the metagenomes.
The SPAdes assembler [98], initially designed for handling data originating from the single-cell experiments, has widely been adapted in assembling metagenomes due to its ability to tackle uneven coverage. SPAdes has recently been updated as a metagenome assembler i.e. metaSPAdes [10], which outperforms its predecessor in terms of quality, time and memory required to complete the assembly. metaSPAdes is also capable of handling multiple k-mers, and it has the ability to add hypothetical k-mers to keep the graph connected that ultimately improves the quality of assembled contigs [10]. However, one of the main pitfalls of metaSPAdes is its inability to handle single-end reads. Recently, metaviralSPAdes [99] has also been developed for the identification of viral genomes from metagenomic assemblies. However, its ability to identify complete viruses remains to be tested using real metagenomes.
Certain metagenomes may be highly complex with hundreds of strains and sequenced at much higher depths. The time and memory required to assemble such samples could be enormous. In such cases, parallel assemblers, such as ABySS [100] and Ray Meta [82], can be highly effective. In the development of Ray Meta, much attention has been paid to computational efficiency, scalability and distribution in the standard computational clusters. Therefore, it can be used without the requirement of having specialized computational resources with very high dedicated memories [82].
Velvet [101], well known for its suitability for genome assemblies, has received several updates to become suitable for metagenomic data: (i) MetaVelvet [81] and (ii) MetaVelvet-SL [102]. MetaVelvet uses paired-end information and differences in coverage to identify chimeric and repetitive contigs. Further modifications in MetaVelvet, using supervised learning (SL), improved the decision making to identify chimeric contigs in MetaVelvet-SL [102].
Although most of these tools have been used in numerous metagenome studies (Table 2), but MEGAHIT [11] has gained increasing attention in the recent years. MEGAHIT employs increasing k-mer along with computationally more efficient dBgs, which are the major reasons behind its success, extreme speed and usage of significantly less amount of memory to finish the metagenome assembly. Although several other tools [103–108] have also been developed for metagenome assembly, no single tool among the newly developed and the previously known tools can be argued to be the ‘best’, especially using the benchmarks provided in their original articles as they contain certain degree of bias toward their reported tool.
Tool . | Access link . | Performance scoring for Genome Binning . | First release . | Last updated . | Current citations . | Reference . | |||
---|---|---|---|---|---|---|---|---|---|
Differential complexity (H, M, L)* . | Required genome coverage (H, L)* . | Genome statistics (N50, # of Contigs) . | Memory requirements . | ||||||
metaSPAdes | http://cab.spbu.ru/software/spades | +,+,+ | +,+ | +,+ | − | 2012 | 2020 | 9760 | [10, 98] |
MEGAHIT | https://github.com/voutcn/megahit | +,+,+ | -,+ | +,- | + | 2015 | 2019 | 1500 | [11] |
IDBA-UD | https://github.com/loneknightpy/idba | -,+,+ | -,+ | +,- | − | 2011 | 2014 | ~2000 | [83, 97] |
Ray Meta | https://bioinformaticshome.com/tools/wga/descriptions/Ray-Meta.html | -,-,+ | -,+ | +,- | − | 2012 | − | 484 | [82] |
MetaVelvet | http://metavelvet.dna.bio.keio.ac.jp/ | -,-,+ | +,- | +,- | − | 2008 | 2014 | >10,000 | [81, 101, 102] |
ABySS | https://www.bcgsc.ca/resources/software/abyss | Not benchmarked | 2009 | 2017 | 3233 | [100] | |||
Genovo/ Xgenovo | http://xgenovo.dna.bio.keio.ac.jp/ | Not benchmarked | 2012 | 2013 | 124 | [87, 88] | |||
MAP | http://bioinfo.ctb.pku.edu.cn/MAP/ | Not benchmarked | 2012 | − | 57 | [90] | |||
Omega | https://omega.omicsbio.org/ | Not benchmarked | 2014 | − | 74 | [77] | |||
SAVAGE | https://bitbucket.org/jbaaijens/savage | Not benchmarked | 2017 | − | 43 | [92] | |||
IVA | http://sanger-pathogens.github.io/iva/ | Not benchmarked | 2015 | 2020 | 114 | [93] | |||
MetaQUAST | http://bioinf.spbau.ru/metaquast | Not applicable | 2016 | 2018 | 188 | [111] | |||
DeepMAsED | https://github.com/leylabmpi/DeepMAsED | Not applicable | 2020 | − | 3 | [112] |
Tool . | Access link . | Performance scoring for Genome Binning . | First release . | Last updated . | Current citations . | Reference . | |||
---|---|---|---|---|---|---|---|---|---|
Differential complexity (H, M, L)* . | Required genome coverage (H, L)* . | Genome statistics (N50, # of Contigs) . | Memory requirements . | ||||||
metaSPAdes | http://cab.spbu.ru/software/spades | +,+,+ | +,+ | +,+ | − | 2012 | 2020 | 9760 | [10, 98] |
MEGAHIT | https://github.com/voutcn/megahit | +,+,+ | -,+ | +,- | + | 2015 | 2019 | 1500 | [11] |
IDBA-UD | https://github.com/loneknightpy/idba | -,+,+ | -,+ | +,- | − | 2011 | 2014 | ~2000 | [83, 97] |
Ray Meta | https://bioinformaticshome.com/tools/wga/descriptions/Ray-Meta.html | -,-,+ | -,+ | +,- | − | 2012 | − | 484 | [82] |
MetaVelvet | http://metavelvet.dna.bio.keio.ac.jp/ | -,-,+ | +,- | +,- | − | 2008 | 2014 | >10,000 | [81, 101, 102] |
ABySS | https://www.bcgsc.ca/resources/software/abyss | Not benchmarked | 2009 | 2017 | 3233 | [100] | |||
Genovo/ Xgenovo | http://xgenovo.dna.bio.keio.ac.jp/ | Not benchmarked | 2012 | 2013 | 124 | [87, 88] | |||
MAP | http://bioinfo.ctb.pku.edu.cn/MAP/ | Not benchmarked | 2012 | − | 57 | [90] | |||
Omega | https://omega.omicsbio.org/ | Not benchmarked | 2014 | − | 74 | [77] | |||
SAVAGE | https://bitbucket.org/jbaaijens/savage | Not benchmarked | 2017 | − | 43 | [92] | |||
IVA | http://sanger-pathogens.github.io/iva/ | Not benchmarked | 2015 | 2020 | 114 | [93] | |||
MetaQUAST | http://bioinf.spbau.ru/metaquast | Not applicable | 2016 | 2018 | 188 | [111] | |||
DeepMAsED | https://github.com/leylabmpi/DeepMAsED | Not applicable | 2020 | − | 3 | [112] |
*H=High, M = Medium, L = Low.
Tool . | Access link . | Performance scoring for Genome Binning . | First release . | Last updated . | Current citations . | Reference . | |||
---|---|---|---|---|---|---|---|---|---|
Differential complexity (H, M, L)* . | Required genome coverage (H, L)* . | Genome statistics (N50, # of Contigs) . | Memory requirements . | ||||||
metaSPAdes | http://cab.spbu.ru/software/spades | +,+,+ | +,+ | +,+ | − | 2012 | 2020 | 9760 | [10, 98] |
MEGAHIT | https://github.com/voutcn/megahit | +,+,+ | -,+ | +,- | + | 2015 | 2019 | 1500 | [11] |
IDBA-UD | https://github.com/loneknightpy/idba | -,+,+ | -,+ | +,- | − | 2011 | 2014 | ~2000 | [83, 97] |
Ray Meta | https://bioinformaticshome.com/tools/wga/descriptions/Ray-Meta.html | -,-,+ | -,+ | +,- | − | 2012 | − | 484 | [82] |
MetaVelvet | http://metavelvet.dna.bio.keio.ac.jp/ | -,-,+ | +,- | +,- | − | 2008 | 2014 | >10,000 | [81, 101, 102] |
ABySS | https://www.bcgsc.ca/resources/software/abyss | Not benchmarked | 2009 | 2017 | 3233 | [100] | |||
Genovo/ Xgenovo | http://xgenovo.dna.bio.keio.ac.jp/ | Not benchmarked | 2012 | 2013 | 124 | [87, 88] | |||
MAP | http://bioinfo.ctb.pku.edu.cn/MAP/ | Not benchmarked | 2012 | − | 57 | [90] | |||
Omega | https://omega.omicsbio.org/ | Not benchmarked | 2014 | − | 74 | [77] | |||
SAVAGE | https://bitbucket.org/jbaaijens/savage | Not benchmarked | 2017 | − | 43 | [92] | |||
IVA | http://sanger-pathogens.github.io/iva/ | Not benchmarked | 2015 | 2020 | 114 | [93] | |||
MetaQUAST | http://bioinf.spbau.ru/metaquast | Not applicable | 2016 | 2018 | 188 | [111] | |||
DeepMAsED | https://github.com/leylabmpi/DeepMAsED | Not applicable | 2020 | − | 3 | [112] |
Tool . | Access link . | Performance scoring for Genome Binning . | First release . | Last updated . | Current citations . | Reference . | |||
---|---|---|---|---|---|---|---|---|---|
Differential complexity (H, M, L)* . | Required genome coverage (H, L)* . | Genome statistics (N50, # of Contigs) . | Memory requirements . | ||||||
metaSPAdes | http://cab.spbu.ru/software/spades | +,+,+ | +,+ | +,+ | − | 2012 | 2020 | 9760 | [10, 98] |
MEGAHIT | https://github.com/voutcn/megahit | +,+,+ | -,+ | +,- | + | 2015 | 2019 | 1500 | [11] |
IDBA-UD | https://github.com/loneknightpy/idba | -,+,+ | -,+ | +,- | − | 2011 | 2014 | ~2000 | [83, 97] |
Ray Meta | https://bioinformaticshome.com/tools/wga/descriptions/Ray-Meta.html | -,-,+ | -,+ | +,- | − | 2012 | − | 484 | [82] |
MetaVelvet | http://metavelvet.dna.bio.keio.ac.jp/ | -,-,+ | +,- | +,- | − | 2008 | 2014 | >10,000 | [81, 101, 102] |
ABySS | https://www.bcgsc.ca/resources/software/abyss | Not benchmarked | 2009 | 2017 | 3233 | [100] | |||
Genovo/ Xgenovo | http://xgenovo.dna.bio.keio.ac.jp/ | Not benchmarked | 2012 | 2013 | 124 | [87, 88] | |||
MAP | http://bioinfo.ctb.pku.edu.cn/MAP/ | Not benchmarked | 2012 | − | 57 | [90] | |||
Omega | https://omega.omicsbio.org/ | Not benchmarked | 2014 | − | 74 | [77] | |||
SAVAGE | https://bitbucket.org/jbaaijens/savage | Not benchmarked | 2017 | − | 43 | [92] | |||
IVA | http://sanger-pathogens.github.io/iva/ | Not benchmarked | 2015 | 2020 | 114 | [93] | |||
MetaQUAST | http://bioinf.spbau.ru/metaquast | Not applicable | 2016 | 2018 | 188 | [111] | |||
DeepMAsED | https://github.com/leylabmpi/DeepMAsED | Not applicable | 2020 | − | 3 | [112] |
*H=High, M = Medium, L = Low.
The critical assessment of metagenome interpretation (CAMI) is a community-driven initiative that is aimed at comprehensive and objective benchmarking of the metagenomics software [78]. The CAMI metagenome assembly challenge was aimed at evaluation of the performance of several state-of-the-art metagenome assemblers using high-complexity datasets, simulated from ~600 microbial genomes ~500 circular elements. Compared with the gold standard assembly of 2.80 Gbp contained in 39,140 contigs, MEGAHIT produced the highest assembly of 1.97 Gbp, with 587 607 contigs and recovered >69% fraction of the genomes [78]. Furthermore, MEGAHIT also demonstrated relatively better performance in handling strain-level diversity in contrast with other assemblers. However, in the CAMI metagenome assembly challenge, benchmarking for several assemblers (e.g. metaSPAdes and MetaVelvet) was not performed.
Vollmers et al. [109] evaluated the performance of majority of the assemblers mentioned here using two real metagenomes. The efficiency of the assemblers was represented with two parameters: assembly performance and assembly cost. Assembly performance was estimated from the product of N50 length of contigs and the percentage of reads mapped to the assembly. In contrast, the assembly cost was calculated through the sum of RAM (in Gigabytes) and runtime (in hours) per processing core (more details about these parameters available in [109]). metaSPAdes produced contigs with higher N50, which recruited a higher number of mapped reads from both of the metagenomes, thus it provided the best assembly performance. The next best performance was achieved by IDBA-UD and followed by MEGAHIT. This indicates that metaSPAdes was better at handling the variable microbial diversity in the two metagenomes. In terms of assembly cost, MEGAHIT produced the best results whereas the cost efficiency for metaSPAdes showed reduction. MetaVelvet and Ray Meta showed the lowest assembly performance and cost efficiency whereas IDBA-UD also showed very poor cost efficiency. To mimic genome binning from the assembly and evaluate genome recovery performance, both metagenomes were spiked with artificial reads for Metanosarcina mazei (4.1 Mbp) and Methanothermobacter marburgensis (1.6 Mbp) genomes at variable coverages. metaSPAdes, IDBA-UD and MEGAHIT showed remarkable sensitivity by successfully reconstructing 50% of the genomes with only 3X read coverage whereas nearly complete lengths were reconstructed with 6X coverage. Ray Meta displayed the lowest sensitivity and required at least 24X read coverage for 50% genome reconstruction. To further elucidate it, Vollmers et al. [109] used a genome ‘recovery performance’ parameter for each of the assemblers that was defined as the product of the fraction of the recovered genome (in percent) and the N50 length of the contigs (in kilobases). metaSPAdes successfully reconstructed larger proportion of these two genomes from both of the metagenomes, in fewer contigs (hence better N50), and using lowest read coverage than other assemblers. Ray Meta also showed higher recovery performance but required relatively higher read coverages. MEGAHIT and IDBA-UD also performed well under low coverages, however, the performance of MEGAHIT was surprisingly reported to deteriorate at >24X coverage [109].
In another independent evaluation of the metagenome assemblers, van der Walt et al. [110] used nine publically available metagenomes (from soil, aquatic environment and human gut) and three simulated metagenomes. Their results also indicated that metaSPAdes produced the largest contigs and higher N50 lengths than other tools. In contrast, MEGAHIT produced assembly of nearly the same quality by utilizing ~6X less memory than metaSPAdes.
Collectively, these benchmarking studies suggest that metaSPAdes should clearly be the choice for genome-resolved metagenome analysis. However, MEGAHIT would be ideal for the computationally limited resources since it offers the best balance between performance and computational cost (Table 2).
Assembly quality assessment
Metagenomes are extremely complex due to the presence of unknown diversity of microbes in the analyzed sample. Therefore, the de novo metagenomic assembly quality can be highly compromised due to presence of inversions, relocations and interspecies translocations. MetaQUAST [111] has the ability to perform reference-based and de novo (uses the closest reference genome) assembly evaluations. Both of these approaches in MetaQUAST become less practical for quality assessment of the metagenomic assemblies. Deep Metagenome Assembly Error Detection (DeepMAsED) [112] is a recently developed tool that uses a deep learning approach for the reference-independent identification of misassembled contigs. DeepMAsED offers in silico simulation of realistic metagenomic assemblies for model training and testing. Although DeepMAsED developers have shown its high accuracy and sensitivity, it remains to be utilized elsewhere.
Genome Binning
Metagenome assembly results in the production of hundreds-to-thousands of fragmented contigs corresponding to different microbes. These contigs can be taxonomically classified and functionally annotated to understand the microbial diversity and metabolic potential of the analyzed environment. However, in certain studies, intended for instance on comparative genomics or evolutionary analyses, metagenome assembly becomes extremely complex and intricate. Therefore, it is necessary to deconvolute the metagenome assembly into individual genomes. To this end, contigs corresponding to the same organism are identified using different properties of their sequences (e.g. composition or abundance) and clustered into genome bins. This process, termed as genome binning, results in the recovery of bins of variable qualities (e.g. draft or high-quality), which require certain post-processing (e.g. refinement and dereplication) before downstream analyses. Hugerth et al. [113] proposed the term metagenome-assembled genomes (MAGs), which has now also been adapted by the Genomic Standards Consortium (GSC) [114] for referring to the bins recovered from metagenomes.
Recovery of MAGs allows us to gain substantial insights into the previously unexplored avenues of microbial life. The uncultivable nature of the overwhelming majority of microbes has been a major bottleneck in expanding the available genomes in the reference databases. Genome binning has allowed us to overcome this limitation. The first large-scale initiative to recover MAGs from the publically-available metagenomes proved highly conducive and provided the first representative genomes of various bacterial and archaeal phyla and also greatly expanded the phylogenetic diversity of their respective genome trees [31]. However, the major focus of this study was the analysis of environmental and non-human gastrointestinal metagenomes.
Recently, numerous genome-resolved studies have analyzed human microbiome samples and highlighted the previously unexplored microbial diversity. Most noticeably, the reconstruction of >150 000 MAGs, from ~10 000 human microbiome samples belonging to diverse geography, age and lifestyles, identified thousands of novel species and genes associated with conditions including infant development or lifestyle [28]. In addition, Almeida et al. [29, 115] and Nayfach et al. [30] have also cataloged thousands of MAGs from human gut microbiome, most of which lack cultured representatives in the genomic repositories. Furthermore, these studies also established association between MAGs and human diseases e.g. Nayfach et al., identified >2200 associations between the recovered MAGs and different diseases (including colorectal cancer, liver cirrhosis, type 2 diabetes). Interestingly, most of the significant associations involved novel MAGs. Furthermore, these MAGs were also characterized by significant genome reduction and loss of certain metabolic pathways [30]. Collectively, these and numerous other studies [20–22, 25, 28–30, 116] indicate that genome binning can provide novel insights into the microbial dark matter and allows better exploitation of the metagenomic data.
The recovery of MAGs has been a major challenge in metagenomic research. The number of the recovered MAGs is highly dependent on the sequencing depth of the assembled contigs (corresponding to an organism) across different samples [117]. Low sequencing depth can result in failed binning unless the genome size is too small. The quality of the recovered MAGs significantly correlates with the quality of the metagenome assembly. Highly fragmented assembly, obtained as a consequence of low coverage, strain-level variations or sequencing errors, is least suitable for genome-resolved analyses. Furthermore, these problems can also significantly increase the computational demands for successful genome binning [117, 118]. Although the current generation of genome binning tools excludes very small-sized or extremely low coverage contigs [117, 119], it is highly important to carefully consider the quality of metagenome assembly before proceeding to genome binning.
Computational methods for recovering MAGs
Majority of the computational methods developed for the recovery of MAGs are based on two different approaches: (i) Supervised binning or (ii) Unsupervised clustering. Supervised binning methods require a database of previously sequenced genomes to taxonomically classify the contigs. However, lack of reference genomes for most of the microbes in the reference databases is a major hindrance in application of supervised genome binning. In contrast, unsupervised clustering methods do not require reference genomes and rather perform self-comparison of the assembled contigs for genome binning. Therefore, unsupervised clustering methods have been widely adapted for the recovery of MAGs from metagenomes.
The unsupervised binning methods are further divided into three categories; (i) sequence composition (SC)-based, (ii) differential abundance (DA)-based and (iii) sequence composition and differential abundance (SCDA)-based methods [19, 117, 120–122]. These three subcategories differ fundamentally at the commencement of the process of genome binning. SC methods rely on the variations in the nucleotide frequencies whereas the DA methods are dependent on the differential abundance of contigs across multiple samples. In contrast, the SCDA methods combine both SC and DA analysis and create a hybrid or composite distance matrix for the process of genome binning. Among these three, SC-based methods were predominantly adapted in the initial genome-resolved metagenomic studies [123–129]. However, with the production of multi-sample metagenomes, DA-based methods emerged as a better alternate for recovering MAGs [17, 130]. Numerous MAGs, encompassing microbes, phages as well as plasmids, have been recovered using the DA-based methods [17, 19, 130]. The initial DA-based methods, such as the extended self-organizing maps (ESOM) [17], also required certain manual data curation for genome binning that becomes impractical for larger number of MAGs. Furthermore, the human supervision also made these methods not fully reproducible or scalable [117]. For overcoming the limitation of these two methods, SCDA-based methods have been developed that are not only more robust but also optimal for handling larger datasets.
CONCOCT [117] was one of the first automated SCDA-based genome binning methods developed in 2014. CONCOCT demonstrated high accuracy using synthetic metagenomes as well as for the real human gut microbiome samples when compared with different SC-based methods [131, 132] available at that time. For instance, from the species mock containing 101 genomes, CONCOCT predicted 101 clusters with precision (purity of clusters) and recall (proportion of species binned to the same cluster) of 0.988 and 0.998, respectively. However, the accuracy decreased using datasets containing strain-level variations or decreased coverage [117]. Recently, CONCOCT has been demonstrated to be more advantageous for the recovery of eukaryotic genome bins [117, 133, 134]. GroopM [135] is another automated binning tool that can recover MAGs from related metagenomes. One key feature of GroopM is the ability to interactively visualize and edit the recovered bins in various different ways e.g. merge or split bins using composition, coverage or contig lengths. However, GroopM requires metagenomic data for at least three related samples to perform its operation. Another limitation is its inability to separate contigs of closely related genotypes, which are placed in ‘chimeric’ bins and require manual curation [135].
MaxBin [136] was also developed concurrently with CONCOCT and GroopM around 2014. Using low-complexity (containing 10 species only) simulated metagenomes, MaxBin showed precision of up to 97.01%. However, with coverage ~20X only 3 of the 10 genomes were recovered that indicates its poor performance under low coverage. Furthermore, MaxBin performed even more poorly using complex metagenomes, with precision as low as 65.07 [136]. Another drawback of this tool was its inability to handle multiple samples. These limitations have been addressed in the algorithm of MaxBin2.0 [137] that not only produced significantly higher number of bins but also achieved higher accuracy of classifying contigs into distinct genomes than CONCOCT and GroopM, when benchmarked using simulated and real metagenomic data. In addition, MaxBin2.0 also performed better and generated higher number of bins using co-assembled metagenomes than single sample binning [137]. The CAMI binning challenge [78] indicated that MaxBin2.0 outperformed all other tools for the medium and low complexity datasets [78].
MetaBAT [119] was initially developed for handling complex microbial communities and accurate reconstruction of MAGs. The initial algorithm of MetaBAT outperformed all three of CONCOCT, GroopM and MaxBin (first generation) in terms of computational efficiency as well as accuracy [119]. However, it was prone to inconsistent results between different datasets and usually required binning with multiple parameter settings and subsequent merging to obtain optimum sensitivity and specificity. This is demonstrated by Parks et al. [31] in the recovery of ~8000 MAGs that required merging of results from five different parameter settings of MetaBAT. These limitations were addressed in MetaBAT 2 [138] by incorporation of a new core binning algorithm. MetaBAT 2 demonstrated massively improved binning, especially when benchmarked using high complexity datasets, in contrast with CONCOCT, MaxBin2.0 and the more recently developed tools: BinSanity [139], MyCC [140] and COCACOLA [141] (Table 3) that themselves showed only marginally improved performance than MaxBin, MetaBAT (first versions of both tools), GroopM and CONCOCT using multiple types of datasets [139, 140]. Furthermore, MetaBAT 2 showed exceptionally superior computational efficiency as it achieved binning ≥90 times faster and by consuming the least amount of memory in contrast with the other tools. Using the CAMI high complexity datasets, MetaBAT 2 outperformed MaxBin2.0 by recovering 333 out of 753 genomes whereas MaxBin2.0 could recover only 195 genomes. Hence, MetaBAT 2 becomes an ideal choice for large datasets originating from unknown complex microbial communities and in computationally limited settings [138].
Tool . | Access link . | Function . | First release . | Last updated . | Current citations . | Reference . |
---|---|---|---|---|---|---|
MetaBAT/ MetaBAT2 | https://bitbucket.org/berkeleylab/metabat | Binning | 2015 | 2019 | 900 | [119] |
CONCOCT | https://github.com/BinPro/CONCOCT | Binning | 2013 | 2019 | 34 | [117] |
MaxBin/MaxBin2 | https://sourceforge.net/projects/maxbin/ | Binning | 2014 | 2020 | 800 | [137] |
GroopM | https://github.com/Ecogenomics/GroopM | Binning | 2014 | 2016 | 219 | [135] |
COCACOLA | https://github.com/younglululu/COCACOLA | Binning | 2017 | 2017 | 81 | [141] |
ABAWACA | https://github.com/CK7/abawaca | Binning | – | – | – | – |
Canopy | http://git.dworzynski.eu/mgs-canopy-algorithm | Binning | 2014 | – | 574 | [19] |
BMC3C | http://mlda.swu.edu.cn/codes.php?name=BMC3C | Binning | 2018 | – | 13 | [227] |
CheckM | https://ecogenomics.github.io/CheckM/ | Quality assessment | 2015 | 2020 | 2244 | [155] |
BUSCO | http://busco.ezlab.org/ | Quality assessment | 2015 | 2020 | 3654 | [156] |
Anvi’o | http://merenlab.org/software/anvio/ | Binning, Quality assessment, Refinement | 2015 | 2020 | 509 | [157] |
VizBin | http://claczny.github.io/VizBin/ | Binning, Quality assessment, Refinement | 2015 | – | 142 | [156] |
Binning_refiner | https://github.com/songweizhi/Binning_refiner | Binning, Quality assessment, Refinement | 2017 | 2019 | 28 | [158] |
DAS Tool | https://github.com/cmks/DAS_Tool | Binning, Quality assessment, Refinement | 2018 | 2020 | 173 | [159] |
IcoVeR | https://git.list.lu/eScience/ICoVeR | Refinement | 2017 | – | 11 | [160] |
MetaWRAP | https://github.com/bxlab/metaWRAP | Binning, Quality assessment, Refinement | 2018 | 2020 | 114 | [161] |
Pyani | https://github.com/widdowquinn/pyani | Dereplication | 2017 | 2020 | 192 | [162] |
Assembly dereplicator | https://github.com/rrwick/Assembly-Dereplicator/tree/v0.1.0 | Dereplication | – | 2019 | – | – |
Mash | https://github.com/marbl/Mash | Dereplication | 2016 | 2019 | 684 | [163] |
dRep | https://github.com/MrOlm/drep | Dereplication | 2017 | 2020 | 173 | [165] |
Tool . | Access link . | Function . | First release . | Last updated . | Current citations . | Reference . |
---|---|---|---|---|---|---|
MetaBAT/ MetaBAT2 | https://bitbucket.org/berkeleylab/metabat | Binning | 2015 | 2019 | 900 | [119] |
CONCOCT | https://github.com/BinPro/CONCOCT | Binning | 2013 | 2019 | 34 | [117] |
MaxBin/MaxBin2 | https://sourceforge.net/projects/maxbin/ | Binning | 2014 | 2020 | 800 | [137] |
GroopM | https://github.com/Ecogenomics/GroopM | Binning | 2014 | 2016 | 219 | [135] |
COCACOLA | https://github.com/younglululu/COCACOLA | Binning | 2017 | 2017 | 81 | [141] |
ABAWACA | https://github.com/CK7/abawaca | Binning | – | – | – | – |
Canopy | http://git.dworzynski.eu/mgs-canopy-algorithm | Binning | 2014 | – | 574 | [19] |
BMC3C | http://mlda.swu.edu.cn/codes.php?name=BMC3C | Binning | 2018 | – | 13 | [227] |
CheckM | https://ecogenomics.github.io/CheckM/ | Quality assessment | 2015 | 2020 | 2244 | [155] |
BUSCO | http://busco.ezlab.org/ | Quality assessment | 2015 | 2020 | 3654 | [156] |
Anvi’o | http://merenlab.org/software/anvio/ | Binning, Quality assessment, Refinement | 2015 | 2020 | 509 | [157] |
VizBin | http://claczny.github.io/VizBin/ | Binning, Quality assessment, Refinement | 2015 | – | 142 | [156] |
Binning_refiner | https://github.com/songweizhi/Binning_refiner | Binning, Quality assessment, Refinement | 2017 | 2019 | 28 | [158] |
DAS Tool | https://github.com/cmks/DAS_Tool | Binning, Quality assessment, Refinement | 2018 | 2020 | 173 | [159] |
IcoVeR | https://git.list.lu/eScience/ICoVeR | Refinement | 2017 | – | 11 | [160] |
MetaWRAP | https://github.com/bxlab/metaWRAP | Binning, Quality assessment, Refinement | 2018 | 2020 | 114 | [161] |
Pyani | https://github.com/widdowquinn/pyani | Dereplication | 2017 | 2020 | 192 | [162] |
Assembly dereplicator | https://github.com/rrwick/Assembly-Dereplicator/tree/v0.1.0 | Dereplication | – | 2019 | – | – |
Mash | https://github.com/marbl/Mash | Dereplication | 2016 | 2019 | 684 | [163] |
dRep | https://github.com/MrOlm/drep | Dereplication | 2017 | 2020 | 173 | [165] |
Tool . | Access link . | Function . | First release . | Last updated . | Current citations . | Reference . |
---|---|---|---|---|---|---|
MetaBAT/ MetaBAT2 | https://bitbucket.org/berkeleylab/metabat | Binning | 2015 | 2019 | 900 | [119] |
CONCOCT | https://github.com/BinPro/CONCOCT | Binning | 2013 | 2019 | 34 | [117] |
MaxBin/MaxBin2 | https://sourceforge.net/projects/maxbin/ | Binning | 2014 | 2020 | 800 | [137] |
GroopM | https://github.com/Ecogenomics/GroopM | Binning | 2014 | 2016 | 219 | [135] |
COCACOLA | https://github.com/younglululu/COCACOLA | Binning | 2017 | 2017 | 81 | [141] |
ABAWACA | https://github.com/CK7/abawaca | Binning | – | – | – | – |
Canopy | http://git.dworzynski.eu/mgs-canopy-algorithm | Binning | 2014 | – | 574 | [19] |
BMC3C | http://mlda.swu.edu.cn/codes.php?name=BMC3C | Binning | 2018 | – | 13 | [227] |
CheckM | https://ecogenomics.github.io/CheckM/ | Quality assessment | 2015 | 2020 | 2244 | [155] |
BUSCO | http://busco.ezlab.org/ | Quality assessment | 2015 | 2020 | 3654 | [156] |
Anvi’o | http://merenlab.org/software/anvio/ | Binning, Quality assessment, Refinement | 2015 | 2020 | 509 | [157] |
VizBin | http://claczny.github.io/VizBin/ | Binning, Quality assessment, Refinement | 2015 | – | 142 | [156] |
Binning_refiner | https://github.com/songweizhi/Binning_refiner | Binning, Quality assessment, Refinement | 2017 | 2019 | 28 | [158] |
DAS Tool | https://github.com/cmks/DAS_Tool | Binning, Quality assessment, Refinement | 2018 | 2020 | 173 | [159] |
IcoVeR | https://git.list.lu/eScience/ICoVeR | Refinement | 2017 | – | 11 | [160] |
MetaWRAP | https://github.com/bxlab/metaWRAP | Binning, Quality assessment, Refinement | 2018 | 2020 | 114 | [161] |
Pyani | https://github.com/widdowquinn/pyani | Dereplication | 2017 | 2020 | 192 | [162] |
Assembly dereplicator | https://github.com/rrwick/Assembly-Dereplicator/tree/v0.1.0 | Dereplication | – | 2019 | – | – |
Mash | https://github.com/marbl/Mash | Dereplication | 2016 | 2019 | 684 | [163] |
dRep | https://github.com/MrOlm/drep | Dereplication | 2017 | 2020 | 173 | [165] |
Tool . | Access link . | Function . | First release . | Last updated . | Current citations . | Reference . |
---|---|---|---|---|---|---|
MetaBAT/ MetaBAT2 | https://bitbucket.org/berkeleylab/metabat | Binning | 2015 | 2019 | 900 | [119] |
CONCOCT | https://github.com/BinPro/CONCOCT | Binning | 2013 | 2019 | 34 | [117] |
MaxBin/MaxBin2 | https://sourceforge.net/projects/maxbin/ | Binning | 2014 | 2020 | 800 | [137] |
GroopM | https://github.com/Ecogenomics/GroopM | Binning | 2014 | 2016 | 219 | [135] |
COCACOLA | https://github.com/younglululu/COCACOLA | Binning | 2017 | 2017 | 81 | [141] |
ABAWACA | https://github.com/CK7/abawaca | Binning | – | – | – | – |
Canopy | http://git.dworzynski.eu/mgs-canopy-algorithm | Binning | 2014 | – | 574 | [19] |
BMC3C | http://mlda.swu.edu.cn/codes.php?name=BMC3C | Binning | 2018 | – | 13 | [227] |
CheckM | https://ecogenomics.github.io/CheckM/ | Quality assessment | 2015 | 2020 | 2244 | [155] |
BUSCO | http://busco.ezlab.org/ | Quality assessment | 2015 | 2020 | 3654 | [156] |
Anvi’o | http://merenlab.org/software/anvio/ | Binning, Quality assessment, Refinement | 2015 | 2020 | 509 | [157] |
VizBin | http://claczny.github.io/VizBin/ | Binning, Quality assessment, Refinement | 2015 | – | 142 | [156] |
Binning_refiner | https://github.com/songweizhi/Binning_refiner | Binning, Quality assessment, Refinement | 2017 | 2019 | 28 | [158] |
DAS Tool | https://github.com/cmks/DAS_Tool | Binning, Quality assessment, Refinement | 2018 | 2020 | 173 | [159] |
IcoVeR | https://git.list.lu/eScience/ICoVeR | Refinement | 2017 | – | 11 | [160] |
MetaWRAP | https://github.com/bxlab/metaWRAP | Binning, Quality assessment, Refinement | 2018 | 2020 | 114 | [161] |
Pyani | https://github.com/widdowquinn/pyani | Dereplication | 2017 | 2020 | 192 | [162] |
Assembly dereplicator | https://github.com/rrwick/Assembly-Dereplicator/tree/v0.1.0 | Dereplication | – | 2019 | – | – |
Mash | https://github.com/marbl/Mash | Dereplication | 2016 | 2019 | 684 | [163] |
dRep | https://github.com/MrOlm/drep | Dereplication | 2017 | 2020 | 173 | [165] |
MAG quality assessment
Recent genome-resolved metagenomic surveys have produced thousands of MAGs from different environments and with continuous improvements in sequencing technologies, MAGs are expected to be recovered at even greater magnitude in the near future. The rapid recovery rate of MAGs also necessitates the availability of automated tools to assess and distinguish variations in the MAG quality and perform certain post-processing to refine or remove the contaminating sequences [142, 143]. Quality assessment and refinement will ensure that the quality of the public genome repositories, like NCBI, is not compromised due to submission of suboptimal MAGs [144].
The quality of isolate genomes is typically assessed using assembly statistics such as the N50 length [145], which is not applicable for genomes recovered from metagenomes. Recently, the GSC developed two standards for reporting bacterial and archaeal genome sequences. These include the minimum information about a single amplified genome (MISAG) and the minimum information about a metagenome-assembled genome (MIMAG) [114]. The MIMAG standards define three important parameters to assess the MAG quality: (i) assembly quality, (ii) completeness and (iii) contamination. Due to lack of reference genomes for majority of MAGs, assembly quality becomes non-trivial. However, statistics including but not limited to N50 length, largest contig, number of contigs, length of the assembled MAG, can provide necessary overview of the MAGs. Additionally, information regarding presence and completion of the encoded ribosomal and transfer RNAs can be used to complete the MAG quality metric.
For estimating the completeness and contamination of a MAG, no standard criteria have been defined. However, using ‘marker’ genes has been widely adapted for this purpose [17, 146–148], which assumes that the given marker gene should be present in genomes of nearly all taxa in single copy and is not subject to horizontal transfer. Several sets of single-copy marker genes, corresponding to bacterial and archaeal genomes, have been identified and validated [148–154]. Using any of these single-copy marker gene sets, completeness can be defined as the ratio of observed single-copy marker genes to the total number of marker genes. Similarly, contamination can be defined as the ratio of observed single-copy marker genes in ≥2 copies to the total number of marker genes [114]. Post quality assessment, MAGs can be classified as: (i) finished MAG (single continuous sequence without gap or overall quality score equal to or above Q50), (ii) high-quality draft (containing multiple contigs, 23S, 16S and 5S ribosomal RNA (rRNA) genes and at least 18 transfer RNA (tRNAs), completeness ≥ 90% and contamination < 5) (iii) medium-quality draft (containing multiple contigs, completeness ≥ 50% and contamination < 10%) and low-quality draft (containing multiple contigs, completeness < 50% and contamination < 10%) [114]. Majority of the downstream analyses tools recommend discarding the low-quality drafts/MAGs to avoid false conclusions. Therefore, it is highly essential to determine the quality of the MAGs before performing advanced analyses.
CheckM [155] is an automated tool that uses lineage-specific marker genes for bacteria and archaea to provide highly accurate quality estimates for MAGs. CheckM requires all MAGs within a single directory as the input and produces comprehensive tabular and graphic outputs presenting the quality statistics that can further be used to remove the contaminating sequences and refine the quality of the MAGs. BUSCO [156] depends on lineage-specific orthologs for estimating the quality of both prokaryotic and eukaryotic MAGs. However, it offers lower accuracy for eukaryotic MAGs. In contrast, EukCC [134] has been developed specifically to determine the quality of eukaryotic MAGs recovered from metagenomes. EukCC offers improved accuracy and increased sensitivity when compared with BUSCO. However, it remains to be tested thoroughly on large and complex metagenomic datasets. Similarly, Anvi’o [157] and VizBin [156] offer integrative workflows for determining the quality of the MAGs. However, both Anvi’o and VizBin require human assistance during their workflows. Therefore, CheckM remains most widely used tool in this category due to its ease-of-use, automated workflow and high accuracy.
In addition, strain heterogeneity, the proportion of polymorphic positions in a MAG, can be inferred to gain additional insights into MAG quality. CheckM [155] and CMSeq (https://github.com/SegataLab/cmseq) are both capable of estimating strain heterogeneity, with the latter being demonstrated to more accurately estimate the expected levels of strain mixtures [28]. Strain heterogeneity can effectively complement completeness and contamination metrics to identify contaminated MAGs and used to support high quality of MAGs [28, 29].
MAG refinement
By determining the quality of the MAGs, improvement in the overall quality of the MAGs becomes possible through manual curation or by automated tools. Different refinement approaches have been designed to increase the completeness and decrease the contamination in the MAGs before downstream analysis. One of these approaches relies on using different binning tools and generation of optimized, non-redundant set of MAGs from the same assembly. Binning_refiner [158] is a pipeline that merges the output of multiple binning programs that can significantly reduce the level of contamination and increase the total size of contamination-free and good-quality MAGs. However, the decrease in contamination is accomplished by splitting the contaminating contig into a newer MAG, which also decreases the completeness level. DAS Tool [159] uses flexible number of binning tools for producing MAGs that are aggregates using the predicted single-copy genes followed by extraction of significantly more complete (containing more single-copy genes) consensus MAG but also increases the chances of contamination. ICoVeR [160] also allows curation of MAGs obtained through multiple binning tools and allows their user-guided refinement to obtain highest quality MAGs. However, compared with the other two tools, ICoVeR is relatively less utilized in the currently published genome-resolved metagenomic studies. Alternatively, it is also possible to refine the MAGs by extracting the mapped reads for each MAG followed by independent reassembly but this approach remains to be properly tested and benchmarked.
Recently, MetaWRAP [161] has been developed as a collection of independent modules to address different aspects of metagenome analysis (details discussed in the last section of review). The Bin_refinement module of MetaWRAP can handle MAGs from three different binning tools and refine them to produce the highest quality MAGs. The Bin_refinement module uses Binning_refiner to produce hybrid MAG sets by ensuring that the two contigs, initially binned in different MAGs by any of the used binning tools, are not together in any hybrid MAG. In the next setup, the hybrid and original MAGs are compared and best version is chosen on the basis of completeness and contamination (estimated by CheckM in Bin_refinement module). Bin_refinement module has been proven to overcome the limitations of Binning_refiner and DAS Tool and is known to provide higher completeness and lower contamination in the refined set of MAGs [161].
MAG dereplication
Metagenomic assembly of individual samples is often performed rather than co-assembly for avoiding assembly fragmentation due to the presence of highly similar sequences in different samples. Subsequently, genome binning results in the recovery of highly similar MAGs across all the samples. Therefore, MAG dereplication is often recommended to make the downstream analyses computationally less intensive.
Generally, dereplication can be defined as the reduction of a given set of MAGs on the basis of sequence similarity among them. The process of removal of redundant MAGs typically requires calculation of average nucleotide identity (ANI) using pairwise MAG alignments. However, high number of MAGs in the dataset can make the process of pairwise alignments computationally too intensive. Pyani [162] computes ANIs using BLAST-based alignment of MAG contigs and requires significant amount of time for these calculations. However, BLAST-based alignment makes the ANI calculations highly inaccurate. Other tools, such as Mash [163] and its extension Mash Screen [164], offer an ultrafast grouping of MAGs. Both Mash and Mash Screen are based on computational concepts of creating ‘sketches’ using the MAGs (or any other genome sequences) and subsequently calculating the ‘distance’ between two sketches to provide an estimate of similarity between the two MAGs. However, the accuracy of Mash decreases significantly for partial or low-quality MAGs. Several tools have implemented Mash in their workflows to overcome its limitations and provide effective dereplication alternates. Assembly dereplicator (https://github.com/rrwick/Assembly-Dereplicator/tree/v0.1.0) is one such tool that has the ability to handle very large (e.g. >10 000) number of MAGs in a memory-efficient way. dRep [165] allows significantly faster and accurate comparisons between MAGs and dereplication using a bi-phasic approach that utilizes Mash and genome-wide ANI (gANI) [166]. In the first phase, Mash is used to create primary clusters of MAGs followed by their pairwise comparison using gANI to form secondary clusters that are then dereplicated. It is possible to perform MASH and gANI calculations without dRep. In such cases, MAGs can be dereplicated with MASH distance of 1e4 (parameter ‘-s 1e4’) or ANI ≥ 95%. However, dRep offers the convenience of automating these steps. The high accuracy and speed of dRep has been demonstrated using MAGs of variable completeness and contamination [165], which makes it an ideal choice for using in MAG dereplication.
Although, MAG dereplication can be highly important, there can be several reasons for not using it. For instance, MAGs with ≥99% ANI can be essential in obtaining information about single nucleotide variations or the variability in auxiliary genes present in same species and originating from different samples [167]. Therefore, the decision to dereplicate MAGs or not, should be made according to the goals of the study.
Taxonomic and functional analysis
Taxonomic analysis of MAGs
Once the representative set of MAGs has been reconstructed, the next step involves their taxonomic inference. Majority of the taxonomic classification tools are designed to work with short reads or contigs and consider each read or contig as an independent observation [168]. These tools usually estimate taxonomy through a best hit against a reference database [169, 170]. However, this approach cannot be applied to MAGs since they can be distantly related to any of the sequences in reference database and can encompass a high degree of novelty. Therefore, the classification of MAGs is typically performed using phylogenomics-based approaches. Phylogenomic approaches have become the de facto standard for inference of taxonomy of the complete genomes [171–173], which involve using the complete genome data to construct phylogeny. MAG classification has also benefitted greatly from the advancements in phylogenomics. However, the tools specifically designed for taxonomic classification of MAGs remain limited.
PhyloSift [159] allows phylogenetic analysis of raw metagenomic reads as well as isolate genomes. The core database in PhyloSift constitutes 37 universal and single copy ‘elite’ gene families [158], whereas the extended database includes 800 gene families in total that mostly correspond to viruses. PhyloSift works by identifying homology between the database sequences and the input sequence, generation and concatenation of RNA or protein multiple sequence alignment (MSA) for producing phylogenetic reference tree. Lastly, taxonomic affiliation is reported. PhyloSift has been successfully used in assigning taxonomy to metagenomic raw reads in various studies, several recent studies have also used it for classification of MAGs [33, 174, 175]. However, the output of PhyloSift may require subsequent manual curation and visual screening to obtain the final taxonomic affiliation of MAGs. lastTaxa (https://gitlab.com/jfroula/lasttaxa) uses National Center for Biotechnology Information’s (NCBI) non-redundant protein database and performs an alignment between the proteins predicted from MAGs and assigned to the taxonomic group with which the majority of proteins are annotated.
Genome taxonomy database tool kit (GTDB-Tk) [176] is a computationally efficient toolkit that uses 120 bacterial and 122 archaeal marker genes for the taxonomic classification of MAGs. First, genes are predicted from the MAGs and aligned with the reference marker gene sets, MAGs are then assigned to the domain with highest proportion of identified marker genes. The domain-specific marker gene alignments are concatenated into single MSA and MAGs are placed into domain-specific reference trees. The taxonomic classification is then determined either using placement of MAGs in the reference tree, by determining ANI with the reference genomes or relative evolutionary divergence (RED) value [177]. Both ANI and RED values are particularly useful when the topology of the tree cannot fully resolve the taxonomy. The current version GTDB-Tk implements a new complete domain-to-species taxonomy classifier for bacteria and archaea [178], which improves the classification of MAGs. Recently, GTDB-Tk has been used for taxonomic classification of 204 938 reference genomes from the human gut microbiome [29] and in several other studies [179–181].
Bin annotation tool (BAT) [168] is also based on a principle similar to lastTaxa. It performs the taxonomic classification of MAGs by predicting open reading frames (ORFs) from each MAG and annotating them using the NCBI non-redundant protein database. The annotated ORFs are individually classified using the last common ancestor (LCA) algorithm followed by summing the scores of all classified ORFs to assign a final taxonomic classification to the MAG [168]. Although, BAT authors report improved and rapid classification than lastTaxa and GTDB-Tk [168], its feasibility and application for determining the taxonomy of large-scale MAG sets remains to be tested. Microbial genome atlas (MiGA) [182] is a webserver-based classification tool for taxonomic analysis of bacteria and archaea at the whole genome levels, making it applicable to MAGs as well. Although the webserver-based nature of MiGA can make its application less practical for larger datasets, it can be used for determining gene content diversities, evolutionary relationships and pangenome analysis, which makes it a suitable choice for analyzing groups of highly similar genomes/MAGs [182].
PhyloPhlAn [183] is an automated, high-throughput pipeline that allows computationally efficient and rapid phylogenetic analysis of genomes and MAGs. The rapid classification, ease-of-use and multi-level phylogenetic resolution make it an appropriate choice for studies involving large-scale phylogenetic profiling. The generalized workflow of PhyloPhlAn is similar to the other tools in this category i.e. from identification of marker genes to generation and concatenation of MSAs, and reconstruction of phylogeny. The current release of PhyloPhlAn (PhyloPhlAn3) [184] allows different marker gene selection options e.g. 400 universal protein database can be used for high-diversity genomes or species-specific core genes from >18 000 sets of UniRef90 [185] gene families for vice versa. PhyloPhlAn3 also allows MASH-based comparisons and assignments of the new MAGs into species-level genome bins built from >230 000 publically available sequences. This can be extremely useful in identifying potentially novel MAGs. Recently, PhyloPhlAn was applied in a genome-resolved metagenomic analysis, which highlighted extensive unexplored diversity in the human microbiome through a catalogue of more than 150 000 MAGs [28]. PhyloPhlAn not only allows integration of publically available genomes and published sets of MAGs but can also be configured to obtain the MSA and the estimated mutation rates for advance phylogenetic and comparative genomic analyses. None of the previously described tools offers this functionality, therefore making PhyloPhlAn a unique choice for taxonomic analysis of MAGs.
Functional annotation of MAGs
Functional annotation generally refers to predicting all genes for a genome and determining their potential role [186]. However, the process of annotation is multi-level and includes protein-coding genes, structural RNAs, tRNAs, small RNAs, repeats, insertion sequences, mobile genetic elements and pseudogenes. Several tools have been developed for identifying and annotating the above mentioned coding and non-coding features from genomes [187–196]. However, manual annotation can be cumbersome for large-scale genome and MAG sets, therefore, automatic annotation tools are more efficient and reliable for performing functional annotations.
The bacterial annotation system (BASys) [197] is one of the first tools that enabled in-depth automatic annotation of bacterial genomic sequences. It integrated more than 30 different programs and reported approximately 60 different annotations, including gene names, functions, possible paralogues and orthologues and reactions and pathways. However, BASys became available through a web server where genomes submitted for annotation could remain queued from days to several weeks. The rapid annotations using subsystems technology (RAST) server [198] was developed for automated annotations of bacterial and archaeal genomes. The accuracy and consistency in RAST-based annotations is derived from the use of a manually curated library of subsystems [199]. Annotation results from the server provide information regarding gene functions and metabolic reconstructions. Typically, annotation for the submitted genomic sequences becomes available within 12–24 h. The integrated microbial genome (IMG) expert review (ER) system [200] can perform annotation of bacterial and archaeal complete genomes and MAGs. IMG/ER processes the annotation through microbial genome annotation pipeline (MGAP) [201] that identifies both protein-coding genes, non-coding and regulatory RNAs and CRISPR elements. The annotated genes can further be assigned to clusters of orthologous groups (COG) [202], Pfam, TIGRfam and KEGG ortholog (KO) terms. Using the KO term assignments, metabolic pathways are also inferred according to MetaCyc pathway classifications [203]. Additionally, ANI, distance matrices, gene cassette region predictions [200] and prediction of biosynthetic clusters [204] as well as putatively horizontally transferred genes are also identified from the genomes. Furthermore, it is also possible to perform comparative genomic analyses with publically available genomes and MAGs in IMG database and visualize the results through the IMG interface. Although, IMG/ER provides extensive annotations, the substantial amount of time required to obtain the results makes it impractical for larger sets of MAGs. Bacterial genome annotation comparison (BEACON) [205], BG7 [206] and automatic annotation of microbial genomes (AAMG) [207] (Table 4) are similar automated annotation tools, however, their application in genome annotation is highly limited.
Tool . | Access link . | Function . | First release . | Last updated . | Current citations . | Reference . |
---|---|---|---|---|---|---|
PhyloSift | https://github.com/gjospin/PhyloSift | Taxonomic classification | 2014 | – | 438 | [159] |
GTDB-Tk | https://github.com/ecogenomics/gtdbtk | Taxonomic classification | 2019 | 2020 | 129 | [176] |
lastTaxa | https://gitlab.com/jfroula/lasttaxa | Taxonomic classification | – | – | – | – |
BAT | – | Taxonomic classification | 2019 | – | 15 | [168] |
MiGA | http://microbial-genomes.org/ | Taxonomic classification | 2018 | 2020 | 120 | [182] |
PhyloPhlAn | http://segatalab.cibio.unitn.it/tools/phylophlan/ | Taxonomic classification | 2013 | 2020 | 448 | [183] |
BASys | http://wishart.biology.ualberta.ca/basys | Genome annotation | 2005 | – | 333 | [197] |
RAST | https://rast.nmpdr.org/ | Genome annotation | 2008 | 2015 | 7630 | [198] |
IMG/ER | http://img.jgi.doe.gov/er | Genome annotation | 2007 | 2019 | 833 | [200] |
BG7 | https://github.com/bg7/BG7 | Genome annotation | 2012 | 2013 | 52 | [206] |
AAMG | http://www.cbrc.kaust.edu.sa/indigo | Genome annotation | 2013 | – | 70 | [207] |
BEACON | http://www.cbrc.kaust.edu.sa/BEACON/ | Genome annotation | 2015 | – | 14 | [205] |
PGAP | https://github.com/ncbi/pgap | Genome annotation | 2013 | 2020 | 1723 | [208] |
Prokka | https://github.com/tseemann/prokka | Genome annotation | 2014 | 2020 | 4794 | [210] |
DeNoGAP | https://sourceforge.net/projects/denogap/ | Genome annotation | 2016 | 2017 | 8 | [214] |
GAMOLA2 | https://drive.google.com/file/d/0B_fIEHIR2oaabVlzcF9NUTlnbjQ/view | Genome annotation | 2017 | – | 13 | [215] |
DFAST | https://github.com/nigyta/dfast_core/ | Genome annotation | 2016 | 2020 | 184 | [216] |
MicrobeAnnotator | https://github.com/cruizperez/MicrobeAnnotator | Genome annotation | 2020 | – | 0 | [217] |
MetaWRAP | https://github.com/bxlab/metaWRAP | Automated Pipeline | 2018 | 2020 | 114 | [161] |
SqueezeMeta | https://github.com/jtamames/SqueezeMeta | Automated Pipeline | 2019 | 2020 | 17 | [224] |
Tool . | Access link . | Function . | First release . | Last updated . | Current citations . | Reference . |
---|---|---|---|---|---|---|
PhyloSift | https://github.com/gjospin/PhyloSift | Taxonomic classification | 2014 | – | 438 | [159] |
GTDB-Tk | https://github.com/ecogenomics/gtdbtk | Taxonomic classification | 2019 | 2020 | 129 | [176] |
lastTaxa | https://gitlab.com/jfroula/lasttaxa | Taxonomic classification | – | – | – | – |
BAT | – | Taxonomic classification | 2019 | – | 15 | [168] |
MiGA | http://microbial-genomes.org/ | Taxonomic classification | 2018 | 2020 | 120 | [182] |
PhyloPhlAn | http://segatalab.cibio.unitn.it/tools/phylophlan/ | Taxonomic classification | 2013 | 2020 | 448 | [183] |
BASys | http://wishart.biology.ualberta.ca/basys | Genome annotation | 2005 | – | 333 | [197] |
RAST | https://rast.nmpdr.org/ | Genome annotation | 2008 | 2015 | 7630 | [198] |
IMG/ER | http://img.jgi.doe.gov/er | Genome annotation | 2007 | 2019 | 833 | [200] |
BG7 | https://github.com/bg7/BG7 | Genome annotation | 2012 | 2013 | 52 | [206] |
AAMG | http://www.cbrc.kaust.edu.sa/indigo | Genome annotation | 2013 | – | 70 | [207] |
BEACON | http://www.cbrc.kaust.edu.sa/BEACON/ | Genome annotation | 2015 | – | 14 | [205] |
PGAP | https://github.com/ncbi/pgap | Genome annotation | 2013 | 2020 | 1723 | [208] |
Prokka | https://github.com/tseemann/prokka | Genome annotation | 2014 | 2020 | 4794 | [210] |
DeNoGAP | https://sourceforge.net/projects/denogap/ | Genome annotation | 2016 | 2017 | 8 | [214] |
GAMOLA2 | https://drive.google.com/file/d/0B_fIEHIR2oaabVlzcF9NUTlnbjQ/view | Genome annotation | 2017 | – | 13 | [215] |
DFAST | https://github.com/nigyta/dfast_core/ | Genome annotation | 2016 | 2020 | 184 | [216] |
MicrobeAnnotator | https://github.com/cruizperez/MicrobeAnnotator | Genome annotation | 2020 | – | 0 | [217] |
MetaWRAP | https://github.com/bxlab/metaWRAP | Automated Pipeline | 2018 | 2020 | 114 | [161] |
SqueezeMeta | https://github.com/jtamames/SqueezeMeta | Automated Pipeline | 2019 | 2020 | 17 | [224] |
Tool . | Access link . | Function . | First release . | Last updated . | Current citations . | Reference . |
---|---|---|---|---|---|---|
PhyloSift | https://github.com/gjospin/PhyloSift | Taxonomic classification | 2014 | – | 438 | [159] |
GTDB-Tk | https://github.com/ecogenomics/gtdbtk | Taxonomic classification | 2019 | 2020 | 129 | [176] |
lastTaxa | https://gitlab.com/jfroula/lasttaxa | Taxonomic classification | – | – | – | – |
BAT | – | Taxonomic classification | 2019 | – | 15 | [168] |
MiGA | http://microbial-genomes.org/ | Taxonomic classification | 2018 | 2020 | 120 | [182] |
PhyloPhlAn | http://segatalab.cibio.unitn.it/tools/phylophlan/ | Taxonomic classification | 2013 | 2020 | 448 | [183] |
BASys | http://wishart.biology.ualberta.ca/basys | Genome annotation | 2005 | – | 333 | [197] |
RAST | https://rast.nmpdr.org/ | Genome annotation | 2008 | 2015 | 7630 | [198] |
IMG/ER | http://img.jgi.doe.gov/er | Genome annotation | 2007 | 2019 | 833 | [200] |
BG7 | https://github.com/bg7/BG7 | Genome annotation | 2012 | 2013 | 52 | [206] |
AAMG | http://www.cbrc.kaust.edu.sa/indigo | Genome annotation | 2013 | – | 70 | [207] |
BEACON | http://www.cbrc.kaust.edu.sa/BEACON/ | Genome annotation | 2015 | – | 14 | [205] |
PGAP | https://github.com/ncbi/pgap | Genome annotation | 2013 | 2020 | 1723 | [208] |
Prokka | https://github.com/tseemann/prokka | Genome annotation | 2014 | 2020 | 4794 | [210] |
DeNoGAP | https://sourceforge.net/projects/denogap/ | Genome annotation | 2016 | 2017 | 8 | [214] |
GAMOLA2 | https://drive.google.com/file/d/0B_fIEHIR2oaabVlzcF9NUTlnbjQ/view | Genome annotation | 2017 | – | 13 | [215] |
DFAST | https://github.com/nigyta/dfast_core/ | Genome annotation | 2016 | 2020 | 184 | [216] |
MicrobeAnnotator | https://github.com/cruizperez/MicrobeAnnotator | Genome annotation | 2020 | – | 0 | [217] |
MetaWRAP | https://github.com/bxlab/metaWRAP | Automated Pipeline | 2018 | 2020 | 114 | [161] |
SqueezeMeta | https://github.com/jtamames/SqueezeMeta | Automated Pipeline | 2019 | 2020 | 17 | [224] |
Tool . | Access link . | Function . | First release . | Last updated . | Current citations . | Reference . |
---|---|---|---|---|---|---|
PhyloSift | https://github.com/gjospin/PhyloSift | Taxonomic classification | 2014 | – | 438 | [159] |
GTDB-Tk | https://github.com/ecogenomics/gtdbtk | Taxonomic classification | 2019 | 2020 | 129 | [176] |
lastTaxa | https://gitlab.com/jfroula/lasttaxa | Taxonomic classification | – | – | – | – |
BAT | – | Taxonomic classification | 2019 | – | 15 | [168] |
MiGA | http://microbial-genomes.org/ | Taxonomic classification | 2018 | 2020 | 120 | [182] |
PhyloPhlAn | http://segatalab.cibio.unitn.it/tools/phylophlan/ | Taxonomic classification | 2013 | 2020 | 448 | [183] |
BASys | http://wishart.biology.ualberta.ca/basys | Genome annotation | 2005 | – | 333 | [197] |
RAST | https://rast.nmpdr.org/ | Genome annotation | 2008 | 2015 | 7630 | [198] |
IMG/ER | http://img.jgi.doe.gov/er | Genome annotation | 2007 | 2019 | 833 | [200] |
BG7 | https://github.com/bg7/BG7 | Genome annotation | 2012 | 2013 | 52 | [206] |
AAMG | http://www.cbrc.kaust.edu.sa/indigo | Genome annotation | 2013 | – | 70 | [207] |
BEACON | http://www.cbrc.kaust.edu.sa/BEACON/ | Genome annotation | 2015 | – | 14 | [205] |
PGAP | https://github.com/ncbi/pgap | Genome annotation | 2013 | 2020 | 1723 | [208] |
Prokka | https://github.com/tseemann/prokka | Genome annotation | 2014 | 2020 | 4794 | [210] |
DeNoGAP | https://sourceforge.net/projects/denogap/ | Genome annotation | 2016 | 2017 | 8 | [214] |
GAMOLA2 | https://drive.google.com/file/d/0B_fIEHIR2oaabVlzcF9NUTlnbjQ/view | Genome annotation | 2017 | – | 13 | [215] |
DFAST | https://github.com/nigyta/dfast_core/ | Genome annotation | 2016 | 2020 | 184 | [216] |
MicrobeAnnotator | https://github.com/cruizperez/MicrobeAnnotator | Genome annotation | 2020 | – | 0 | [217] |
MetaWRAP | https://github.com/bxlab/metaWRAP | Automated Pipeline | 2018 | 2020 | 114 | [161] |
SqueezeMeta | https://github.com/jtamames/SqueezeMeta | Automated Pipeline | 2019 | 2020 | 17 | [224] |
NCBI’s prokaryotic genome annotation pipeline (PGAP) [208] was initially available as an online service; however, its current issue has been updated to work as a standalone tool and can annotate MAGs and draft genomes. PAGP uses the universally conserved, clade-specific ribosomal genes (called as core genes) at species or higher levels to generate annotations of the genomic sequences. This (pan-genome approach) is extremely useful for comparative analysis of large groups of highly related genomes. RefSeq Targeted Loci collection [209] is used as the reference for identifying 16S and 23S rRNAs. Several other annotations, including tRNA and CRISPR, are also performed [208]. PGAP has the ability to annotate more than 1200 MAGs (or genomes) per day with high accuracy for both protein-coding and non-coding elements.
Prokka [210], a command line tool, is one of the most widely adopted annotation tools that performs rapid and highly accurate annotations of complete genomes and MAGs. The annotation involves integration of several databases, including ~16 000 validated UniProt proteins [211], genus-specific RefSeq proteins from finished bacterial genomes, and multiple Hidden Markov Model profile databases from Pfam [212] and TIGRFAMs [213]. Annotation against these databases is performed in hierarchical manner i.e. starting from UniProt proteins, followed by RefSeq entries and Pfam or TIGRfam. Using Prokka is straightforward and its output files are compatible with several downstream analyses and visualization tools. However, their discussion is beyond the scope of this review. With Prokka, it is possible to classify a single genome/MAG within 10 min using a standard desktop computer, which highlights its ultrafast speed [210].
In recent years, several other automated annotation tools have been developed for improved performance and increased accuracy. The de novo genome analysis pipeline (DeNoGAP) [214] has specially been designed for annotation and comparative analysis of large number of complete and draft genomes. It integrates multiple tools and databases for annotation and adopts an iterative clustering approach that reduces the computational complexity during comparative analysis. Furthermore, DeNoGAP has the ability to create local databases for storing the annotated data and graphical interface to explore and compare data for multiple genomes. However, currently no large scale study has included DeNoGAP in their analysis. GAMOLA2 [215] is another, less known, comprehensive annotation and curation tool for complete and draft genomes.
DDBJ fast annotation and submission Tool (DFAST) [216] supports annotation as well as submission of the genome to public database repositories. Although the database of DFAST is 20 times bigger than Prokka (417 922 sequences in DFAST versus 18,276 in Prokka), it is still able to complete single genome annotation within 10 min, which indicates its superiority in speed over Prokka. The annotations produced by DFAST are very comparable to Prokka and MiGAP in terms of the total annotated genes, noncoding RNAs and pseudogene counts [216]. MicrobeAnnotator [217] is the most recently developed tool in this category that also uses iterative approach similar to Prokka for annotating genomes and MAGs belonging to bacteria, archaea and viruses. The authors of the tool have reported better performance than Prokka and RAST but the capability of MicrobeAnnotator remains to be tested on real datasets (especially MAGs).
Automated Genome-resolved metagenomic analysis
The conventional analysis of metagenomes has been integrated into several pipelines that allow improved and automated execution of all or certain steps of the analysis. For instance, InteMAP [105], combines two dBg assemblers (ABySS, IDBA-UD) and one OLC assembler (Celera [218]) and generates optimal assembly by merging the outputs of the pairs of assemblers [86]. MetaCRAM [219] is an integrated pipeline that utilizes assembly via IDBA-UD, followed by compression of assemblies for storage. MOCAT [220] is a toolkit that allows read QC, removal of human or any other hosts’ reads via read mapping to the hosts’ reference genome, assembly, gene prediction and gene annotation. MetaAMOS [221] also has an integration of modules similar to MOCAT for metagenome analysis. Furthermore, MetaAMOS integrates ~20 different metagenomic assemblers and enables users to create customized workflows according to requirements and suitability to their data. In contrast, limited efforts have been carried out to integrate the whole workflow of genome-resolved metagenome analysis (i.e. read QC, assembly, binning, bin refinement and annotation) into an automated and customizable pipeline.
MetaWRAP [161] is a collection of independent modules that work from processing of raw reads and end with high- quality MAG sets and their annotations. The read QC module allows read quality visualization, trimming and removal of host reads. The metagenomic assembly can be performed using either metaSPAdes or MEGAHIT in the assembly module. Additionally, assembly quality assessment and taxonomic profiling and visualization of reads and contigs can also be performed. The binning module allows initial binning and extraction of MAGs using MetaBAT, MaxBin and CONCOCT, independently or in any combination. Bin refinement and reassembly modules can be applied to increase the MAG completeness, increase N50 and lower the contamination levels. Other modules allow quantification and visualization of MAGs across multiple samples, as well as taxonomic and functional visualization. MetaWRAP has shown improved performance, especially for bin refinement, and has already been used in various genome-resolved metagenomic studies [115, 222, 223].
SqueezeMeta [224] is another pipeline that allows automation of all necessary steps of genome-resolved metagenome analyses in a computationally efficient way. SqueezeMeta can be run in a fully automatic way, without the requirement of technical or bioinformatics knowledge. In contrast with MetaWRAP, several advanced features are available in Squeeze- Meta. For instance, it supports co-assembly of metagenome samples, with or without merging individual metagenomes. Other features include binning and MAG refinement, taxonomic annotation of contigs and MAGs with internal checks, and support for nanopore long reads and metatranscriptomic data. This pipeline also supports multiple different options to explore and visualize results. For example, the results can be stored in a local database for effective manipulation and visualization through a web-based interface. Furthermore, it also allows whole SqueezeMeta project to be exported to R (open-source programing language) through the SQMtools R package provided with the pipeline. SQMtools allows visualization of the results as well as generation of tables for multivariate analysis and differential abundance testing using third-party packages in R. These flexibilities make SqueezeMeta a better solution over MetaWRAP. However, in our opinion, the fully capability of the SqueezeMeta pipeline still needs to be thoroughly tested using large-scale metagenomic datasets.
Conclusions
Advances in high-throughput sequencing technologies and data analysis methods have increased the recovery of MAGs in last few years. The taxonomic identification and characterization of the metabolic potential of MAGs can provide essential insights for understanding microbial adaptations in different environmental settings. We believe this review will assist the researchers in developing a basic understanding of the genome-resolved metagenome analysis and assist them in performing the data analysis by providing a guide for correctly selecting the required tools for each step of the analysis.
Genome-resolved metagenomics enables the recovery of draft and high-quality microbial genomes of uncultivable and novel microorganisms.
Introduced the main steps and currently available tools for performing genome-resolved analysis from any metagenome sample, and post-processing, taxonomic classification and functional annotation of the recovered genomes.
Information on the most widely adapted and well-maintained tools to help the scientific community in choosing a suitable tool for their analysis.
Overview of the analysis pipelines to assist people lacking advanced bioinformatics and computational skills in performing automated genome-resolved analysis.
Authors’ contributions
M.R.K., H.W. and R.F. carried out the literature survey and prepared the manuscript. L.C. conceived the idea and supervised the manuscript preparation. All authors read and approved the manuscript.
Availability of data and material
Not applicable.
Acknowledgments
We are highly thankful to Dr. Samina Shaheen and Dr. Syed Shujaat Ali Zaidi for their feedback on the manuscript.
Funding
The Shanghai Jiao Tong University, School of Medicine New PI Startup Fund (No. 17X100040046).
Conflicts of interest
The authors declare that they have no conflicts of interests.
Masood ur Rehman Kayani is a postdoctoral researcher at Shanghai Institute of Immunology, School of Medicine, Shanghai Jiao Tong University, Shanghai, China.
Wanqiu Huang is a PhD student at Shanghai Institute of Immunology, School of Medicine, Shanghai Jiao Tong University, Shanghai, China.
Ru Feng is a PhD student at Shanghai Institute of Immunology, School of Medicine, Shanghai Jiao Tong University, Shanghai, China.
Lei Chen is a principle investigator and associate professor at Shanghai Institute of Immunology, School of Medicine, Shanghai Jiao Tong University, Shanghai, China.
References
Author notes
Masood ur Rehman Kayani and Huang Wanqiu contributed equally to this study.