-
PDF
- Split View
-
Views
-
Cite
Cite
Yu Ding, Hong Wang, Hewei Zheng, Lianzong Wang, Guosi Zhang, Jiaxin Yang, Xiaoyan Lu, Yu Bai, Haotian Zhang, Jing Li, Wenyan Gao, Fukun Chen, Shui Hu, Jingqi Wu, Liangde Xu, Evaluation of drug efficacy based on the spatial position comparison of drug–target interaction centers, Briefings in Bioinformatics, Volume 21, Issue 3, May 2020, Pages 762–776, https://doi.org/10.1093/bib/bbz024
- Share Icon Share
Abstract
The spatial position and interaction of drugs and their targets is the most important characteristics for understanding a drug’s pharmacological effect, and it could help both in finding new and more precise treatment targets for diseases and in exploring the targeting effects of the new drugs. In this work, we develop a computational pipeline to confirm the spatial interaction relationship of the drugs and their targets and compare the drugs’ efficacies based on the interaction centers. First, we produce a 100-sample set to reconstruct a stable docking model of the confirmed drug–target pairs. Second, we set 5.5 Å as the maximum distance threshold for the drug–amino acid residue atom interaction and construct 3-dimensional interaction surface models. Third, by calculating the spatial position of the 3-dimensional interaction surface center, we develop a comparison strategy for estimating the efficacy of different drug–target pairs. For the 1199 drug–target interactions of the 649 drugs and 355 targets, the drugs that have similar interaction center positions tend to have similar efficacies in disease treatment, especially in the analysis of the 37 targeted relationships between the 15 known anti-cancer drugs and 10 target molecules. Furthermore, the analysis of the unpaired anti-cancer drug and target molecules suggests that there is a potential application for discovering new drug actions using the sampling molecular docking and analyzing method. The comparison of the drug–target interaction center spatial position method better reflect the drug–target interaction situations and could support the discovery of new efficacies among the known anti-cancer drugs.
Introduction
Drug repositioning is the best way to discover new effective drugs for treating different diseases [1]. As key actors in pharmacology, protein targets combine with drugs by forming specific 3-dimensional conformation-dependent ligand–receptor interaction pairs [2, 3]. The spatial structures of a drug–target interaction, especially the drug’s action sites, have a decisive effect on how the drug works [4]. Deeply exploring the drug–target interactions and clarifying the relationship between the spatial position and drug efficacy will greatly improve our understanding of the action mechanism of single or multi-targeted drugs and shed light on the proceedings of new drug discovery [5].
With the rising cancer incidence and mortality, the demand for anti-tumor drugs is also growing [6, 7]. The traditional heterocyclic moieties, such as 5-fluorouracil, methotrexate and vinblastine, remain in the core group of anti-cancer drugs, especially for uncertain cancer subtypes [8]. Recently, many anti-cancer targeted drugs have been developed, such as itraconazole, nelfinavir and gefitinib; these drugs play important roles in treating some subtypes of cancer [9]. These targeted anti-cancer therapies completely change the treatment theory of cancer by transforming malignancies into long-term controllable conditions. Nevertheless, drug resistance and adverse effects limit their efficacy in long-term treatment, so the substitute drug use strategy is required for most cancer therapies [10]. By focusing on the development of targeted cancer therapy, a review introduces recent progresses and advances of computational tools and systems pharmacology approaches for identifying drug targets [11]. The rapid progress in genomics and bioinformatics and the wide variety of screening tests based on cellular and target genes have accelerated the pace of drug discovery [12, 13]. By incorporating similarities of drugs and cell lines shown from gene expression profiles, drug chemical structure as well as drug response similarity, a hybrid interpolation weighted collaborative filtering (HIWCF) method predicts anti-cancer drug responses of cell lines with higher performance [14]. With the successful clinical introduction of a number of non-cancer drugs for cancer treatment, drug repositioning has become a powerful strategy for discovering and developing novel anti-cancer drug candidates from the existing drugs [15].
With the increasing therapeutic effects of targeted drugs, computational methods for drug–target interactions have gained increasing attention [16, 17]. Ezzat et al. [18] introduced an overview and empirical evaluation on the computational drug–target interaction prediction techniques, which provide a comprehensive understanding for researchers who are working in the drug–target interaction field. Chen et al. [19] introduced some state-of-the-art computational models for drug–target interactions prediction. A novel network integration pipeline, DTINet, integrates information about drugs, proteins, diseases and side effects to develop a feature learning and vector space projection strategy and predict novel drug–target interactions [20]. KronRLS-MKL simulates the drug–target interaction as a link prediction task on bipartite networks and provides a new way to identify the drug–target binding sites [21]. Wang et al. [22] conclude that drug structure, drug profile and protein sequence similarities are the main types of similarity measures that are used for drug–target interaction prediction which inspired us to consider the drug structural information in our study. In addition, HotSpot3D identifies mutation–mutation and mutation–drug clusters using 3-dimensional drug–protein structures, which shows that the spatial structure plays an important role in cancer and other common diseases [23]. These studies provide new insights into drug discovery, repositioning and the further elucidation of the mechanisms of drug molecules.
The specific chemical structure of a drug molecule determines its efficacy, pharmacokinetics and pharmacodynamics. Drug transformation under different situations alters biological activities and influences its physical and chemical properties [24]. Once the drug binds to its target, the extent of the spatial complementarity between the groups has a significant impact on the drug efficacy. An increased drug–target spatial structure complementarity corresponds to a higher specificity, shorter distance and stronger interaction force of the drug target; thus, the drug will selectively bind to a class of structurally compatible targets with high affinity to perform its therapeutic efficacy [25]. It has been proposed that P-glycoprotein may have up to four distinct drug-binding sites and that each substrate has a distinct binding site [26–29]. Also, structurally different substrates could share the same residues during drug binding [30]. Therefore, it is of great significance to determine the precise binding sites and regions of a drug on its target proteins. Ulaganathan et al. [31] have identified and characterized a distinct allosteric pocket in Eg5 by using x-ray crystallography, kinetic and biophysical methods. This pocket may represent a novel site for drug development in cancer chemotherapy. Molecular docking methods can be used to fit the structure-dependent drug–target interactions, simulate the dynamic interaction and predict the binding mode between the drug and its target at the atomic level [32, 33].
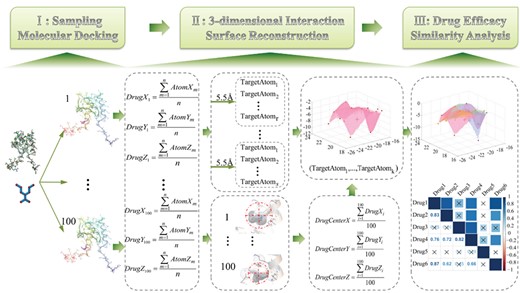
Pipeline of the SMDA method. The three main parts are as follows: (I) molecular docking—randomly select spatial conformations after performing drug–target docking; (II) three-dimensional interaction surface reconstruction—calculate the DTIC and determine the 3-dimensional interaction surface construction atoms; and (III) similarity analysis—drug efficacy analysis based on the spatial position of the DTIC.
In this research, we develop a sampling molecular docking and analyzing (SMDA) method to measure the relationship between the clinical efficacy and the spatial position concordance of the drug–target interaction (the detailed workflow is shown in Figure 1). We reconstruct a 3-dimensional interaction surface of the drug–target relationship by molecule docking, assess the similarity of the drug efficacy based on the similarity of the interaction centers and quantitatively analyze the similarities of the efficacy for the drug pairs. Furthermore, the efficacy of unconfirmed anti-cancer drugs is estimated by SMDA, which provides novel insights into anti-cancer drug repositioning. This study explores the drug–target interaction and implies a potential association between the spatial structure and functions, which help guide future drug development and innovation.
Materials and methods
Drug and target data acquisition
In this study, we focus on small molecular drugs, protein targets, their clinical efficacy and their inner relationships. The chemical and clinical information of the small molecule drugs and their spatial structure data are obtained from the DrugBank database [34]. The protein targets and their spatial structure data were obtained from the PDB database, including the atomic types, atomic spatial coordinates and atomic charge [35]. The approved small molecule drugs and protein target interactions are extracted from the DrugBank database (Version 4.5.0). The interaction data were filtered as follows:
(i) Those drugs and proteins with known 3-dimensional structure were reserved.
(ii) Those target proteins and their corresponding drugs were excluded if the target proteins only targeted by one drug.
(iii) Those target proteins existed in human were reserved.
We also collect the clinical and targeted information about the anti-cancer drugs by searching the DrugBank database and published literature.
Drug–target sampling molecular docking
The molecular docking principle in this research is based on a semi-flexible docking strategy that uses the iterated local search global optimizer model to keep the situation of the target atoms unchanged while fine-tuning the drug’s spatial conformation to achieve a relatively reliable and stable molecular interaction simulation (Figure 1, part I). To obtain relatively reliable docking results, this process is performed in the AutoDockVina software [36]. To reduce the heterogeneity of the docking system and the disturbance of the embedded algorithms, we propose a sampling molecular docking model. Generally, AutodockVina produces fewer than nine molecular docking conformations for each drug–target pair at one time. Experimental results of molecular docking show that drug–target spatial conformation produced in each experiment is variable, and it has subtle changes in different rounds. The structure of drug–target conformation may change slightly when the drug binding to the target, for example, axitinib and vascular endothelial growth factor receptor 1 have 3 different binding structures from PDB database (PDB ID: 4AG8, 4AGC, 4TWP). So the multiple docking results can satisfy the polymorphism of drug’s conformation transformation. Here, due to the characteristics of the AutoDockVina software, we perform the docking 20 times to obtain approximately 180 docking conformations for a certain drug–target pair. Then, we randomly select 100 spatial conformations from the molecular docking results and we thought that these conformations are sufficient to reflect the state of multiple conformations. The atom type and coordinate position for the drug and target protein amino acid residues were collected for use in the subsequent analysis. In the process, we exclude the drug–target interaction pairs with fewer than 100 docking conformations after sampling.
Drug–target 3-dimensional interaction surface reconstruction
Here, |$\Big({DrugX}_i,{DrugY}_i,{DrugZ}_i\Big)$| is the drug center coordinate of one sampling, |$\Big({AtomX}_m,{AtomY}_m,{AtomZ}_m\Big)$| indicates the drug coordinate of one sampling and |$n$| is the number of atoms of the docking drug. Second, we set a threshold as the maximum distance for the drug center to target and extract the amino residue atoms in the defined scope as the candidate interaction atoms. Considering the extent of Van der Waals force and the relative references, the threshold is assigned as 5.5 Å [37]. Third, we select the atoms with an occurrence rate 0.6 or more in the 100 samplings as valid atoms for reconstructing the drug–target 3-dimensional interaction surfaces and realize the visualization.
Interaction center-based drug efficacy similarity measurement
Effect assessment and expanded anti-cancer drug repositioning analysis
The effect assessment of the interaction center similarity comparison is based on the drug efficacy semantic similarity from DrugBank and the published reference annotation. We acquired drug indications from DrugBank databases which show the description or common names of diseases that the drug is used to treat. The keywords mean disease name or their synonyms mentioned in descriptions of drug indications. Disease-related terms or vocabularies are mainly referenced from the ICD-10 database, then we compare their pharmacodynamic similarities by manual annotation process. We assign a weight score of 1 for the drug pairs that they used to treat the same diseases or have common keywords in their descriptions; otherwise, a score of −1 is given (here, |${w}_1$| for short). Previous studies have shown that 0.6 is often used as the threshold of correlation coefficient [38, 39]. For drug pairs with a similarity measurement score above 0.6, we set 1 as the spatial distance similarity estimation and use −1 for the others (here, |${w}_2$| for short). The product (|$w$|) of |${w}_1$| and |${w}_2$| is used to denote the relationship of the similarity of the spatial distance and drug efficacy. In the annotation process, we focus mainly on the clinical targeted anti-cancer drugs and their targets. We collect a wide range of anti-cancer drug indications by database searching and literature mining and further improve the pharmacology and biological annotations of these drugs. Then, we use this method for the anti-cancer drug repositioning analysis. For the collected 3-dimensional structures of the known targeted anti-cancer drug target proteins, we perform sampling docking and calculate a similarity score for the combination of the known and unknown drug pairs to get a candidate set for drug repositioning.
Drug–target crystal structure-based drug efficacy similarity measurement
We also compare drug-pairs efficacies similarity based on drug–target crystal structures. For the stationary drug–target crystal structures stored in PDB database, we applied the similar analysis processes description in `Interaction center-based drug efficacy similarity measurement’. We determine the docking drug center coordinate using the drug atom coordinate obtained from each crystal conformation. Then, we set a threshold 5.5 Å as the maximum distance for the drug center to target and extracted the amino residue atoms in the defined scope as the |$t- th$| drug’s interaction atom set |${\Big\{{TargetAtom}_{tj}\Big\}}_{j=1}^k$|. For the same target, the union of the interacted atom set of any two docked drugs, |$x$| and |$y$|, is recorded as |${\Big\{ UnionAtom\Big\}}_{xy}$|. According to Equation 4, we calculate the similarity score based on the spatial position of the DTICs.
Distribution of interaction surfaces atom-based drug efficacy similarity measurement
where |${(IS)}_l^p$| and |${(IS)}_l^q$| are the vector of shape descriptors for |$p- th$| and |$q- th$| query interaction surface, respectively.
Results
Overview of small molecule drug and target data
In this research, we obtain information for 1053 approved small molecule drugs and 714 drug targets, corresponding to 2742 drug–target interactions, after the filter process of DrugBank database. By random sampling docking and interaction surface atom selection, finally, we acquire 649 drugs and 355 targets, corresponding to the information of 1199 drug–target interactions (Supplementary Table S1). On average, each drug has approximately 2 (|$1.85\pm 1.43$|) targeted proteins, and each protein is targeted by almost 3 (|$3.38\pm 6.88$|) drugs. The extended information, including the drug’s 3-dimensional structure, drug indications and drug efficacy, is kept, and the data from 355 target protein 3-dimensional structures are extracted by the DrugBank crosslink of the PDB database. We also get the clinical anti-cancer drug information and the target molecule relationships. Table 1 lists information on the currently approved 15 anti-cancer targeted drugs, 10 target proteins and 37 drug–target interactions, including their annotated data sources. A total of 10 of the 15 drugs have more than 1 target protein, and all 10 of the corresponding targets have 2 or more drugs targeting them.
DrugBank ID . | Drug name . | UniProt ID of Target . | Target name . | PDB ID of Target . | Resourcea . |
---|---|---|---|---|---|
DB00317 | Gefitinib | P00533 | EGFR | 5SX4 | 10815932|11522647|11566608|11585753|11673690|11752352 |
DB00398 | Sorafenib | P17948 | VEGFR1 | 5ABD | 15466206|16507829|17016424 |
DB00398 | Sorafenib | P09619 | PDGFR | 2L6W | 16425993|17545544|17619763 |
DB00398 | Sorafenib | P07949 | RET | 5FM2 | 15466206|16507829|17016424 |
DB00530 | Erlotinib | P00533 | EGFR | 5SX4 | 12498017|12517254|12814826|12820772|12840797|11752352 |
DB00619 | Imatinib | P10721 | KIT | 4PGZ | 16865565|16087693|17458563|17369583|17559139 |
DB00619 | Imatinib | P00519 | ABL | 4ZOG | 15799618|15917650|15949566|16153117|16205964 |
DB01254 | Dasatinib | P00519 | ABL | 4ZOG | 17016423|17701954|11752352 |
DB01268 | Sunitinib | P36888 | FLT3 | 4RT7 | 12531805|14654525|14753710|15180525|15304385|11752352 |
DB01268 | Sunitinib | P35916 | VEGFR3 | 4BSJ | 17367763|17605814|16685460|17296815|15688612 |
DB01268 | Sunitinib | P17948 | VEGFR1 | 5ABD | 14654525|17367763 |
DB01268 | Sunitinib | P10721 | KIT | 4PGZ | 12748309|12873999|17545799|14753710|17367763|11752352 |
DB01268 | Sunitinib | P09619 | PDGFR | 2L6W | 12538485|12748309|14753710|15557593|16425993 |
DB01268 | Sunitinib | P07949 | RET | 5FM2 | HSDB |
DB04868 | Nilotinib | P10721 | KIT | 4PGZ | 17699867 |
DB04868 | Nilotinib | P00519 | ABL | 4ZOG | 17929114|17715389|16721371|19920925|19822896 |
DB05294 | Vandetanib | P35968 | VEGFR2 | 5EW3 | NCIt |
DB05294 | Vandetanib | P07949 | RET | 5FM2 | 10592235 |
DB06589 | Pazopanib | P17948 | VEGFR1 | 5ABD | 17288876 |
DB06589 | Pazopanib | P10721 | KIT | 4PGZ | 17288876 |
DB08865 | Crizotinib | P08581 | MET | 5HLW | 22594847|10592235 |
DB08875 | Cabozantinib | P10721 | KIT | 4PGZ | NCIt |
DB08875 | Cabozantinib | P08581 | MET | 5HLW | 21606412|21926191 |
DB08875 | Cabozantinib | P07949 | RET | 5FM2 | 21606412 |
DB08896 | Regorafenib | P35968 | VEGFR2 | 5EW3 | DrugBank |
DB08896 | Regorafenib | P35916 | VEGFR3 | 4BSJ | DrugBank |
DB08896 | Regorafenib | P17948 | VEGFR1 | 5ABD | DrugBank |
DB08896 | Regorafenib | P07949 | RET | 5FM2 | DrugBank |
DB08896 | Regorafenib | P00519 | ABL | 4ZOG | DrugBank |
DB08901 | Ponatinib | P36888 | FLT3 | 4RT7 | 23430109 |
DB08901 | Ponatinib | P35968 | VEGFR2 | 5EW3 | 19878872 |
DB08901 | Ponatinib | P07949 | RET | 5FM2 | 23526464 |
DB08901 | Ponatinib | P00519 | ABL | 4ZOG | 23409026 |
DB09078 | Lenvatinib | P35968 | VEGFR2 | 5EW3 | 17943726 |
DB09078 | Lenvatinib | P17948 | VEGFR1 | 5ABD | 17943726 |
DB09078 | Lenvatinib | P10721 | KIT | 4PGZ | 17943726 |
DB09330 | Osimertinib | P00533 | EGFR | 5SX4 | 26522274 |
DrugBank ID . | Drug name . | UniProt ID of Target . | Target name . | PDB ID of Target . | Resourcea . |
---|---|---|---|---|---|
DB00317 | Gefitinib | P00533 | EGFR | 5SX4 | 10815932|11522647|11566608|11585753|11673690|11752352 |
DB00398 | Sorafenib | P17948 | VEGFR1 | 5ABD | 15466206|16507829|17016424 |
DB00398 | Sorafenib | P09619 | PDGFR | 2L6W | 16425993|17545544|17619763 |
DB00398 | Sorafenib | P07949 | RET | 5FM2 | 15466206|16507829|17016424 |
DB00530 | Erlotinib | P00533 | EGFR | 5SX4 | 12498017|12517254|12814826|12820772|12840797|11752352 |
DB00619 | Imatinib | P10721 | KIT | 4PGZ | 16865565|16087693|17458563|17369583|17559139 |
DB00619 | Imatinib | P00519 | ABL | 4ZOG | 15799618|15917650|15949566|16153117|16205964 |
DB01254 | Dasatinib | P00519 | ABL | 4ZOG | 17016423|17701954|11752352 |
DB01268 | Sunitinib | P36888 | FLT3 | 4RT7 | 12531805|14654525|14753710|15180525|15304385|11752352 |
DB01268 | Sunitinib | P35916 | VEGFR3 | 4BSJ | 17367763|17605814|16685460|17296815|15688612 |
DB01268 | Sunitinib | P17948 | VEGFR1 | 5ABD | 14654525|17367763 |
DB01268 | Sunitinib | P10721 | KIT | 4PGZ | 12748309|12873999|17545799|14753710|17367763|11752352 |
DB01268 | Sunitinib | P09619 | PDGFR | 2L6W | 12538485|12748309|14753710|15557593|16425993 |
DB01268 | Sunitinib | P07949 | RET | 5FM2 | HSDB |
DB04868 | Nilotinib | P10721 | KIT | 4PGZ | 17699867 |
DB04868 | Nilotinib | P00519 | ABL | 4ZOG | 17929114|17715389|16721371|19920925|19822896 |
DB05294 | Vandetanib | P35968 | VEGFR2 | 5EW3 | NCIt |
DB05294 | Vandetanib | P07949 | RET | 5FM2 | 10592235 |
DB06589 | Pazopanib | P17948 | VEGFR1 | 5ABD | 17288876 |
DB06589 | Pazopanib | P10721 | KIT | 4PGZ | 17288876 |
DB08865 | Crizotinib | P08581 | MET | 5HLW | 22594847|10592235 |
DB08875 | Cabozantinib | P10721 | KIT | 4PGZ | NCIt |
DB08875 | Cabozantinib | P08581 | MET | 5HLW | 21606412|21926191 |
DB08875 | Cabozantinib | P07949 | RET | 5FM2 | 21606412 |
DB08896 | Regorafenib | P35968 | VEGFR2 | 5EW3 | DrugBank |
DB08896 | Regorafenib | P35916 | VEGFR3 | 4BSJ | DrugBank |
DB08896 | Regorafenib | P17948 | VEGFR1 | 5ABD | DrugBank |
DB08896 | Regorafenib | P07949 | RET | 5FM2 | DrugBank |
DB08896 | Regorafenib | P00519 | ABL | 4ZOG | DrugBank |
DB08901 | Ponatinib | P36888 | FLT3 | 4RT7 | 23430109 |
DB08901 | Ponatinib | P35968 | VEGFR2 | 5EW3 | 19878872 |
DB08901 | Ponatinib | P07949 | RET | 5FM2 | 23526464 |
DB08901 | Ponatinib | P00519 | ABL | 4ZOG | 23409026 |
DB09078 | Lenvatinib | P35968 | VEGFR2 | 5EW3 | 17943726 |
DB09078 | Lenvatinib | P17948 | VEGFR1 | 5ABD | 17943726 |
DB09078 | Lenvatinib | P10721 | KIT | 4PGZ | 17943726 |
DB09330 | Osimertinib | P00533 | EGFR | 5SX4 | 26522274 |
aThe drug indicates the annotation resources, including HSDB, NCIt, DrugBank and PubMed. The numbers under `Resourcea’ means PubMed ID.
DrugBank ID . | Drug name . | UniProt ID of Target . | Target name . | PDB ID of Target . | Resourcea . |
---|---|---|---|---|---|
DB00317 | Gefitinib | P00533 | EGFR | 5SX4 | 10815932|11522647|11566608|11585753|11673690|11752352 |
DB00398 | Sorafenib | P17948 | VEGFR1 | 5ABD | 15466206|16507829|17016424 |
DB00398 | Sorafenib | P09619 | PDGFR | 2L6W | 16425993|17545544|17619763 |
DB00398 | Sorafenib | P07949 | RET | 5FM2 | 15466206|16507829|17016424 |
DB00530 | Erlotinib | P00533 | EGFR | 5SX4 | 12498017|12517254|12814826|12820772|12840797|11752352 |
DB00619 | Imatinib | P10721 | KIT | 4PGZ | 16865565|16087693|17458563|17369583|17559139 |
DB00619 | Imatinib | P00519 | ABL | 4ZOG | 15799618|15917650|15949566|16153117|16205964 |
DB01254 | Dasatinib | P00519 | ABL | 4ZOG | 17016423|17701954|11752352 |
DB01268 | Sunitinib | P36888 | FLT3 | 4RT7 | 12531805|14654525|14753710|15180525|15304385|11752352 |
DB01268 | Sunitinib | P35916 | VEGFR3 | 4BSJ | 17367763|17605814|16685460|17296815|15688612 |
DB01268 | Sunitinib | P17948 | VEGFR1 | 5ABD | 14654525|17367763 |
DB01268 | Sunitinib | P10721 | KIT | 4PGZ | 12748309|12873999|17545799|14753710|17367763|11752352 |
DB01268 | Sunitinib | P09619 | PDGFR | 2L6W | 12538485|12748309|14753710|15557593|16425993 |
DB01268 | Sunitinib | P07949 | RET | 5FM2 | HSDB |
DB04868 | Nilotinib | P10721 | KIT | 4PGZ | 17699867 |
DB04868 | Nilotinib | P00519 | ABL | 4ZOG | 17929114|17715389|16721371|19920925|19822896 |
DB05294 | Vandetanib | P35968 | VEGFR2 | 5EW3 | NCIt |
DB05294 | Vandetanib | P07949 | RET | 5FM2 | 10592235 |
DB06589 | Pazopanib | P17948 | VEGFR1 | 5ABD | 17288876 |
DB06589 | Pazopanib | P10721 | KIT | 4PGZ | 17288876 |
DB08865 | Crizotinib | P08581 | MET | 5HLW | 22594847|10592235 |
DB08875 | Cabozantinib | P10721 | KIT | 4PGZ | NCIt |
DB08875 | Cabozantinib | P08581 | MET | 5HLW | 21606412|21926191 |
DB08875 | Cabozantinib | P07949 | RET | 5FM2 | 21606412 |
DB08896 | Regorafenib | P35968 | VEGFR2 | 5EW3 | DrugBank |
DB08896 | Regorafenib | P35916 | VEGFR3 | 4BSJ | DrugBank |
DB08896 | Regorafenib | P17948 | VEGFR1 | 5ABD | DrugBank |
DB08896 | Regorafenib | P07949 | RET | 5FM2 | DrugBank |
DB08896 | Regorafenib | P00519 | ABL | 4ZOG | DrugBank |
DB08901 | Ponatinib | P36888 | FLT3 | 4RT7 | 23430109 |
DB08901 | Ponatinib | P35968 | VEGFR2 | 5EW3 | 19878872 |
DB08901 | Ponatinib | P07949 | RET | 5FM2 | 23526464 |
DB08901 | Ponatinib | P00519 | ABL | 4ZOG | 23409026 |
DB09078 | Lenvatinib | P35968 | VEGFR2 | 5EW3 | 17943726 |
DB09078 | Lenvatinib | P17948 | VEGFR1 | 5ABD | 17943726 |
DB09078 | Lenvatinib | P10721 | KIT | 4PGZ | 17943726 |
DB09330 | Osimertinib | P00533 | EGFR | 5SX4 | 26522274 |
DrugBank ID . | Drug name . | UniProt ID of Target . | Target name . | PDB ID of Target . | Resourcea . |
---|---|---|---|---|---|
DB00317 | Gefitinib | P00533 | EGFR | 5SX4 | 10815932|11522647|11566608|11585753|11673690|11752352 |
DB00398 | Sorafenib | P17948 | VEGFR1 | 5ABD | 15466206|16507829|17016424 |
DB00398 | Sorafenib | P09619 | PDGFR | 2L6W | 16425993|17545544|17619763 |
DB00398 | Sorafenib | P07949 | RET | 5FM2 | 15466206|16507829|17016424 |
DB00530 | Erlotinib | P00533 | EGFR | 5SX4 | 12498017|12517254|12814826|12820772|12840797|11752352 |
DB00619 | Imatinib | P10721 | KIT | 4PGZ | 16865565|16087693|17458563|17369583|17559139 |
DB00619 | Imatinib | P00519 | ABL | 4ZOG | 15799618|15917650|15949566|16153117|16205964 |
DB01254 | Dasatinib | P00519 | ABL | 4ZOG | 17016423|17701954|11752352 |
DB01268 | Sunitinib | P36888 | FLT3 | 4RT7 | 12531805|14654525|14753710|15180525|15304385|11752352 |
DB01268 | Sunitinib | P35916 | VEGFR3 | 4BSJ | 17367763|17605814|16685460|17296815|15688612 |
DB01268 | Sunitinib | P17948 | VEGFR1 | 5ABD | 14654525|17367763 |
DB01268 | Sunitinib | P10721 | KIT | 4PGZ | 12748309|12873999|17545799|14753710|17367763|11752352 |
DB01268 | Sunitinib | P09619 | PDGFR | 2L6W | 12538485|12748309|14753710|15557593|16425993 |
DB01268 | Sunitinib | P07949 | RET | 5FM2 | HSDB |
DB04868 | Nilotinib | P10721 | KIT | 4PGZ | 17699867 |
DB04868 | Nilotinib | P00519 | ABL | 4ZOG | 17929114|17715389|16721371|19920925|19822896 |
DB05294 | Vandetanib | P35968 | VEGFR2 | 5EW3 | NCIt |
DB05294 | Vandetanib | P07949 | RET | 5FM2 | 10592235 |
DB06589 | Pazopanib | P17948 | VEGFR1 | 5ABD | 17288876 |
DB06589 | Pazopanib | P10721 | KIT | 4PGZ | 17288876 |
DB08865 | Crizotinib | P08581 | MET | 5HLW | 22594847|10592235 |
DB08875 | Cabozantinib | P10721 | KIT | 4PGZ | NCIt |
DB08875 | Cabozantinib | P08581 | MET | 5HLW | 21606412|21926191 |
DB08875 | Cabozantinib | P07949 | RET | 5FM2 | 21606412 |
DB08896 | Regorafenib | P35968 | VEGFR2 | 5EW3 | DrugBank |
DB08896 | Regorafenib | P35916 | VEGFR3 | 4BSJ | DrugBank |
DB08896 | Regorafenib | P17948 | VEGFR1 | 5ABD | DrugBank |
DB08896 | Regorafenib | P07949 | RET | 5FM2 | DrugBank |
DB08896 | Regorafenib | P00519 | ABL | 4ZOG | DrugBank |
DB08901 | Ponatinib | P36888 | FLT3 | 4RT7 | 23430109 |
DB08901 | Ponatinib | P35968 | VEGFR2 | 5EW3 | 19878872 |
DB08901 | Ponatinib | P07949 | RET | 5FM2 | 23526464 |
DB08901 | Ponatinib | P00519 | ABL | 4ZOG | 23409026 |
DB09078 | Lenvatinib | P35968 | VEGFR2 | 5EW3 | 17943726 |
DB09078 | Lenvatinib | P17948 | VEGFR1 | 5ABD | 17943726 |
DB09078 | Lenvatinib | P10721 | KIT | 4PGZ | 17943726 |
DB09330 | Osimertinib | P00533 | EGFR | 5SX4 | 26522274 |
aThe drug indicates the annotation resources, including HSDB, NCIt, DrugBank and PubMed. The numbers under `Resourcea’ means PubMed ID.
Characteristic analysis of the 3-dimensional interaction surface
On the interaction surface constructed by sampling docking, with a distance threshold of 5.5 Å, the number and type of amino acids and atoms vary across the different drug–target pairs. For the reconstructed surfaces that contain more than 2 atoms, the number of amino acids on the interaction surface is approximately 1~8 (|$2.77\pm 1.30$|) (Figure 2A). According to the reconstructed 3-dimensional surface from SMDA process, the inferred number of interacted target atoms on the surface is approximately 3~31 (|$7.71\pm 3.90$|) (Figure 2A). Among the 2528 amino acids on the interaction surfaces, the hydrophobic and basic amino acids are the most common (Figure 2B). For example, phenylalanine (PHE), arginine (ARG), leucine (LEU), histidine (HIS) and valine (VAL) are the top 5 amino acids, with the highest frequency of occurrence, in which PHE has the maximum value at 255 (10.13%). However, the sulfur-containing amino acids cysteine (CYS, 0.28%) and methionine (MET, 1.63%) rarely appear on the surface. By calculating the average coordinate of 100 sampling drug centers (|$DTIC$|), the simulated distance between the DTIC and its corresponding atom set on the interaction surface is approximately 0.40~15.26 Å (|$4.63\pm 1.41$|) (Figure 2C).
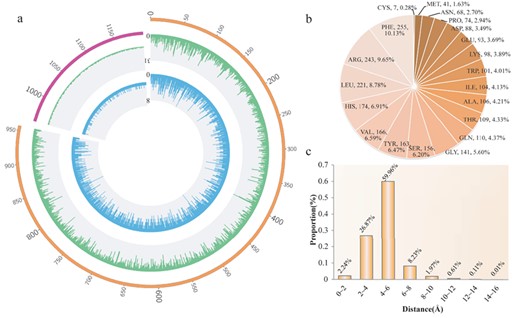
Amino acid and atom characteristic analysis of the 3-dimensional interaction surface. (A) Distribution of amino acid number and atom number on the interaction surface. The inner blue ring corresponds to the distribution of the amino acid number, and the outer green ring corresponds to the distribution of the atom number. The top left part indicates that there are less than three atoms on the interaction surface. (B) Distribution of amino acid type on the interaction surface. (C) Distribution of distance from the DTIC to the corresponding atom set on the interaction surface.
![Drug efficacy similarity comparison of eight drugs acting on the glycine receptor subunit alpha-1. (A) Similarity scores for 28 pairs of 8 drugs. `×’ indicates a similarity score with no statistical significance ($P > 0.05$). The numbers [−1, 1] indicate the similarity scores of the drug efficacy. (B) Spatial configuration of the drugs and the target molecular (glycine receptor subunit alpha-1) docking.](https://oup.silverchair-cdn.com/oup/backfile/Content_public/Journal/bib/21/3/10.1093_bib_bbz024/3/m_bbz024f3.jpeg?Expires=1749362416&Signature=s1~UJGyvdToIzrfRrZu8JMQLNcl066auJIGwHI6ejs7~rdHcd~4GX~hoySbB~QzMaY3rwM~pEcVNrlS9Ya2jEJoj-AozLOB7q6~VkPMrjRXKFW9LfJ8eEaXWJxvaT~kJdF24GeiZYc6yXjbYVz4vAQJzsGnBq4kBpz4~L5hh-SO~neDYQhUP5JL4YXR9FrcBLgOsKh4hgMrNRyUJ7QI~~d6DpgHdP1oPziOL-L~yeLFVFp3lndCh2dLk-17gPbaqLRZka2B2cXpKHgsLZ5uoGvDIfHtCf45H~BgL3uGGTKb5CtUP-Qe8gWorHdufXss~QI8iMvfAdPMFMBCzL5z01Q__&Key-Pair-Id=APKAIE5G5CRDK6RD3PGA)
Drug efficacy similarity comparison of eight drugs acting on the glycine receptor subunit alpha-1. (A) Similarity scores for 28 pairs of 8 drugs. `×’ indicates a similarity score with no statistical significance (|$P > 0.05$|). The numbers [−1, 1] indicate the similarity scores of the drug efficacy. (B) Spatial configuration of the drugs and the target molecular (glycine receptor subunit alpha-1) docking.
Similarity analysis of drug efficacy based on the spatial position of the DTIC
According to the similarity measurement method, we calculate the drug efficacy similarity score for all the drugs with targets with at least two interaction drugs (Supplementary Table S2). Of the 6985 total drug pairs, 2316 have a similarity score above 0.6 and |$P<0.05$|. For those known drug pairs of the identical target, we acquire a measurement score (|${w}_1$|; Supplementary Table S2) based on the drug efficacy annotation extracted from the DrugBank database that is used to evaluate the semantic similarity. Then, the product of |${w}_1$| and |${w}_2$| (|$w$|, Materials and methods; Supplementary Table S2) is calculated to compare the relationship between the semantic similarity of the drug efficacy and the DTIC similarity. The result explains that 28.29% of the drug pairs with a similar semantic level efficacy tend to have a closer DTIC, which shows the high correlation of drug efficacy and spatial action sites. Further, approximately 78.97% of the drug pairs have no direct relationship between drug efficacy and the DTIC. This might be because the method based on semantic similarity could not identify synonyms precisely. Therefore, it remains a great challenge to develop a more comprehensive annotation of drug efficacies. On the other hand, the drug’s annotation information stored in databases is not complete, and the efficacy of many drugs has not been discovered yet. The lower consistence ratio is particularly helpful for understanding the potential pharmacological effects of existing drugs and identifying their potential new applications.
As an example, Figure 3A shows the similarity score matrix of eight small-molecule drugs (methoxyflurane, enflurane, halothane, isoflurane, desflurane, lindane, glycine, sevoflurane) stored in DrugBank that act on the target glycine receptor subunit alpha-1 (P23415). The similarity scores are listed in Supplementary Table S2 and are visualized by the color bar and the size of the square in Figure 3A. According to the efficacy description in DrugBank listed in Table 2, of the eight drugs, methoxyflurane, halothane and isoflurane are used for typical general anesthesia; enflurane, desflurane and sevoflurane are used for anesthesia with special applications; and glycine and lindane are not used as anesthesia in the clinic. The differences in the drug efficacies have strong correlations with the interaction center similarity score, especially when comparing the efficacies of lindane and glycine with those of the other drugs (the similarity score are all less than 0.4, and most are negative correlations). In Figure 3B, the spatial configuration of the docking eight drugs and target protein P23415 shows a consistent result with the similarity score. There are two diverse docking regions around the target, and the distribution follows the drug efficacies. Glycine has two binding pockets, while the other seven drugs only bind to pocket 1. Compared to the other six drugs binding to pocket 1, lindane binds far from this pocket.
DrugBank ID . | Drug name . | Drug indication . |
---|---|---|
DB01028 | Methoxyflurane | Used for the induction and maintenance of general anesthesia |
DB00228 | Enflurane | Used for the induction and maintenance of general anesthesia during surgery and cesarean section and used for analgesia during vaginal delivery |
DB01159 | Halothane | Used for the induction and maintenance of general anesthesia |
DB00753 | Isoflurane | Used for the induction and maintenance of general anesthesia |
DB01189 | Desflurane | Used as an inhalation agent for induction and/or maintenance of anesthesia for inpatient and outpatient surgery in adults |
DB01236 | Sevoflurane | Used for induction and maintenance of general anesthesia in adult and pediatric patients for inpatient and outpatient surgery |
DB00145 | Glycine | Supplemental glycine may have antispastic activity. Very early findings suggest that there may also be antipsychotic activity as well as antioxidant and anti-inflammatory activities. |
DB00431 | Lindane | Used for the treatment of patients infested with Sarcoptes scabiei or Pediculosis capitis who have either failed to respond to adequate doses or are intolerant of other approved therapies |
DrugBank ID . | Drug name . | Drug indication . |
---|---|---|
DB01028 | Methoxyflurane | Used for the induction and maintenance of general anesthesia |
DB00228 | Enflurane | Used for the induction and maintenance of general anesthesia during surgery and cesarean section and used for analgesia during vaginal delivery |
DB01159 | Halothane | Used for the induction and maintenance of general anesthesia |
DB00753 | Isoflurane | Used for the induction and maintenance of general anesthesia |
DB01189 | Desflurane | Used as an inhalation agent for induction and/or maintenance of anesthesia for inpatient and outpatient surgery in adults |
DB01236 | Sevoflurane | Used for induction and maintenance of general anesthesia in adult and pediatric patients for inpatient and outpatient surgery |
DB00145 | Glycine | Supplemental glycine may have antispastic activity. Very early findings suggest that there may also be antipsychotic activity as well as antioxidant and anti-inflammatory activities. |
DB00431 | Lindane | Used for the treatment of patients infested with Sarcoptes scabiei or Pediculosis capitis who have either failed to respond to adequate doses or are intolerant of other approved therapies |
DrugBank ID . | Drug name . | Drug indication . |
---|---|---|
DB01028 | Methoxyflurane | Used for the induction and maintenance of general anesthesia |
DB00228 | Enflurane | Used for the induction and maintenance of general anesthesia during surgery and cesarean section and used for analgesia during vaginal delivery |
DB01159 | Halothane | Used for the induction and maintenance of general anesthesia |
DB00753 | Isoflurane | Used for the induction and maintenance of general anesthesia |
DB01189 | Desflurane | Used as an inhalation agent for induction and/or maintenance of anesthesia for inpatient and outpatient surgery in adults |
DB01236 | Sevoflurane | Used for induction and maintenance of general anesthesia in adult and pediatric patients for inpatient and outpatient surgery |
DB00145 | Glycine | Supplemental glycine may have antispastic activity. Very early findings suggest that there may also be antipsychotic activity as well as antioxidant and anti-inflammatory activities. |
DB00431 | Lindane | Used for the treatment of patients infested with Sarcoptes scabiei or Pediculosis capitis who have either failed to respond to adequate doses or are intolerant of other approved therapies |
DrugBank ID . | Drug name . | Drug indication . |
---|---|---|
DB01028 | Methoxyflurane | Used for the induction and maintenance of general anesthesia |
DB00228 | Enflurane | Used for the induction and maintenance of general anesthesia during surgery and cesarean section and used for analgesia during vaginal delivery |
DB01159 | Halothane | Used for the induction and maintenance of general anesthesia |
DB00753 | Isoflurane | Used for the induction and maintenance of general anesthesia |
DB01189 | Desflurane | Used as an inhalation agent for induction and/or maintenance of anesthesia for inpatient and outpatient surgery in adults |
DB01236 | Sevoflurane | Used for induction and maintenance of general anesthesia in adult and pediatric patients for inpatient and outpatient surgery |
DB00145 | Glycine | Supplemental glycine may have antispastic activity. Very early findings suggest that there may also be antipsychotic activity as well as antioxidant and anti-inflammatory activities. |
DB00431 | Lindane | Used for the treatment of patients infested with Sarcoptes scabiei or Pediculosis capitis who have either failed to respond to adequate doses or are intolerant of other approved therapies |
We also get the similar results in carbonic anhydrase 4 (P22748) and gamma-aminobutyric acid receptor subunit beta-3 (P28472) (Supplementary Table S2). We obtained a drug pair with a higher similarity score (|${r}_{Cyclothiazide- Hydrochlorothiazide}=1.00$|) of carbonic anhydrase 4. Annotations from DrugBank show that both two drugs are used for the treatment of high blood pressure and management of edema. Five drug pairs acting on gamma-aminobutyric acid receptor subunit beta-3 exceed 0.6 (|${r}_{Isoflurane- Sevoflurane}=0.98$|, |${r}_{Enflurane- Desflurane}=0.82$|, |${r}_{Desflurane- Sevoflurane}=0.73$|, |${r}_{Isoflurane- Desflurane}=0.70$|, |${r}_{Enflurane- Isoflurane}=0.69$|). After efficacy search and annotation, we find that all of these drugs were used for induction and maintenance of anesthesia.
Anti-cancer drug analysis based on the similarity comparison of drug efficacy
We further analyze the efficacy similarity of the anti-cancer drugs used in the clinic. Based on the analysis results of approved drug–target interactions and the particularity and complexity of cancer in human disease, a strict threshold of 0.7 was chosen in order to get more accurate results in anti-cancer drug analysis. This threshold does not change the operation process and the overall result of the whole algorithm. Researchers can screen the results according to their own needs. For the 10 targets and 15 drugs, we construct the 37 interaction surfaces and calculate the interaction center similarity scores (Supplementary Table S3). Finally, we acquire 36 similarity scores, 11 of which exceed 0.7. After efficacy search and annotation, 11 drug pairs have a certain similar efficacy.
Figure 4 shows the drug pairs’ similarity score comparisons and the fitted interaction surfaces corresponding to the 4 targets. Figure 4A fits the 3-dimensional interaction surfaces of imatinib and ponatinib, acting on tyrosine-protein kinase (P00519). By calculating the distance distribution from the DTIC to atom set on the interaction surface, we find that these two drugs have a high similarity score (|${r}_{Imatinib- Ponatinib}=0.74$|, Figure 4B). The pharmacology and biological annotations from the DrugBank database show that these two drugs are both used to treat leukemia.
![Drug–target 3-dimensional interaction surface and efficacy similarity of the drug pairs. The left panel (a/c/e/g) shows different interaction surfaces. `+’ indicates different DTICs. `·’ indicates atoms on interaction surfaces. The right panel (b/d/f/h) shows similarity scores for drug pairs. `×’ indicates a similarity score with no statistical significance (P > 0.05). The numbers [−1, 1] indicate the similarity scores of the drug efficacy.](https://oup.silverchair-cdn.com/oup/backfile/Content_public/Journal/bib/21/3/10.1093_bib_bbz024/3/m_bbz024f4.jpeg?Expires=1749362416&Signature=jelsO23MLjg6P8kEddk~T-gtTLyAgPA20-7-YALkrd4mcCR71CGlidSihZPso0n0ANxVCSuU0KQckI6OhIXKefHt34gV4qvF~2bIUjjIA1BrqbCFKh82aizCksiv-IOU7BcVHEZgLeM-IbsvvxVwerC6f7lbQL395g4Km8E8rhLB6qsVJj8gqoglUggb9a3~d66VFfFQLgMyR3LAIetYre44~JwSecYHeuI0kdFYzBmIQc1p35jMmZLsSMpIeDoaUJsAWw88GgLEYDy4FsqYTencCSp70-WwjHEirEebcxjwO-S0KxuIUeqdQCmCkQsjROJBQKMeo4TbBFbZtfrMlg__&Key-Pair-Id=APKAIE5G5CRDK6RD3PGA)
Drug–target 3-dimensional interaction surface and efficacy similarity of the drug pairs. The left panel (a/c/e/g) shows different interaction surfaces. `+’ indicates different DTICs. `·’ indicates atoms on interaction surfaces. The right panel (b/d/f/h) shows similarity scores for drug pairs. `×’ indicates a similarity score with no statistical significance (P > 0.05). The numbers [−1, 1] indicate the similarity scores of the drug efficacy.
Vascular endothelial growth factor receptor 1 (P17948) is targeted by five anti-cancer drugs. After analyzing the efficacy similarity of drug pairs, we identify four similar small molecule drug pairs (Figure 4C, Supplementary Table S3). The DrugBank database annotation information showed that pazopanib and sorafenib have effects on renal cell carcinoma (|${r}_{Pazopanib- Sorafenib}=0.99$|; Figure 4D). Regorafenib is used to treat colorectal and gastrointestinal stromal tumors, but the DrugBank database does not record similar indications for regorafenib and pazopanib/sorafenib (|${r}_{Regorafenib- Pazopanib}=1.00$|, |${r}_{Regorafenib- Sorafenib}=0.98$|). Further study of the literature shows that regorafenib is a novel bi-aryl urea compound that has potential anti-tumor activity in renal cell cancer [42, 43]. The DrugBank database annotation information indicates that lenvatinib is used to treat thyroid cancer. Studies have shown that pazopanib monotherapy inhibits colony formation in anaplastic thyroid cancer cells and that combination of pazopanib and trametinib inhibit the growth of thyroid cancer cell lines (|${r}_{Lenvatinib- Pazopanib}=0.79$|) [44, 45]. This finding indicates that our strategy, based on a similarity analysis of drug–target 3-dimensional interaction centers, can supplement the current drug-related annotation information.
Figure 4E fits the 3-dimensional interaction surfaces of regorafenib and ponatinib, acting on vascular endothelial growth factor receptor 2 (P35968). Our results show that regorafenib and ponatinib have a higher similarity score (|${r}_{Regorafenib- Ponatinib}=0.71$|, Figure 4F). The spatial interaction surfaces of ponatinib and regorafenib acting on vascular endothelial growth factor receptor 2 are different, but they have close DTICs. Our methods consider the distribution of atoms on interaction surfaces and compare the drugs’ efficacies based on the interaction centers comprehensively. So the drug pairs have a higher similarity score. The annotation information in the database indicates that regorafenib can be used in the treatment of gastrointestinal stromal tumors, and relevant studies have shown that ponatinib (30 mg daily) displayed encouraging clinical activity in 2 of 3 patients with gastrointestinal stromal tumors [46].
The target proto-oncogene tyrosine-protein kinase receptor Ret (P07949) is related to five anti-cancer drugs. After screening and analyzing the efficacy similarity of the drug pairs, we identify five similar small molecule drug pairs (Figure 4G, H). The similarity score of Sunitinib and vandetanib is 0.82. Studies have shown that these two drugs were found to be associated with significant dose-dependent inhibition of proliferation and an enhancement of apoptosis in a panel of four human gastric and esophageal cancer cell lines [47]. The other four similar drug pairs can be validated in the database or related literature, indicating that any one drug pair can be used to treat the same kind of cancer (Supplementary Tables S3 and S4).
Repositioning study for targeting anti-cancer drugs
Based on the calculation and comparison of the spatial distance distribution from the average drug center to the atom set on the interaction surface, the possibility of a drug acting on the new target can be predicted and screened quantitatively. This study further analyzes the above candidate 10 anti-cancer targets. Based on the known paired interactions, in total, we analyze 141 drug pairs and calculate the spatial distance distribution for the paired drug–target interactions (known drug–target interaction pairs) and the unpaired drug–target interactions (unknown drug–target interaction pairs) to predict the possibility of new targeted relationships.
A strict threshold of 0.7 is set to evaluate the efficacy similarities between the drug pairs, and 39 similar drug pairs were retained. Five of these drug pairs have similar annotations for drug efficacy according to the DrugBank database (Supplementary Table S5); the other similar drug pairs provide useful directional information for repositioning the anti-cancer drugs and discovering new protein targets. Figure 5 illustrates the distance distribution between the interaction center and the atoms on the interacted surface of the target protein receptor-type tyrosine-protein kinase FLT3 (P36888). The top five group in the figure indicates that five drugs, including crizotinib, lenvatinib, dasatinib, sorafenib, and regorafenib, are associated with lung cancer, thyroid carcinoma, leukemia, renal carcinoma and gastrointestinal stromal tumor, respectively, by their DrugBank annotations. In our study, we predict a new functional effect of these anti-cancer targeted drugs by utilizing the SMDA strategy and infer their underlying drug efficiencies upon renal carcinoma or gastrointestinal stromal tumors. This premise is certified by the database and literature annotations (Supplementary Table S5) [48–51]. These results may provide important hints for understanding the structural basis of the predicted drug–target interactions and can thus help reveal the underlying molecular mechanisms of drug action.
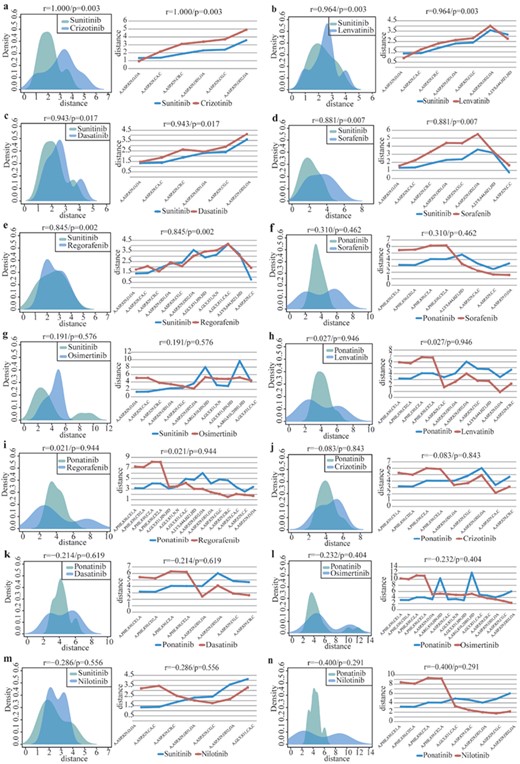
Distance distribution between the drug center and atoms on the 3-dimensional interaction surface of target P36888 (protein receptor-type tyrosine-protein kinase FLT3). |$r$| and |$p$| indicate similarity score and statistical significance of drug pairs, respectively. (A–E) presents two drugs with an identical target and similar efficacy (above threshold r > 0.7, P < 0.05). (F–N) presents two drugs with an identical target and a non-similar efficacy (below threshold).
Comparison of similarity scores based on the molecular docking conformation and drug–target crystal structure
We analyze and compare the similarity of drug pairs based on the molecular docking conformations and drug–target crystal structures of the target carbonic anhydrase 2 (P00918) (Supplementary Table S6). For those 15 drug pairs, we find that 11 of them are consistent with our calculation result. Further annotations from DrugBank database show that crystal-based approaches are more likely to reflect the consistence of drug indications and its similarity scores. While, we also find that there are 468 drug–target crystal structures stored in PDB database, which is much smaller than the numbers of total drug–target interactions (2742).
Comparison of similarity scores based on the DTIC and interaction surfaces atoms
For the two small-molecule drugs, adenosine monophosphate and ATP, that act on 5′-AMP-activated protein kinase catalytic subunit alpha-1 (Q13131), the similarity scores are 0.92 and 0.86 for the two methods, respectively (Supplementary Table S7). In addition, the pharmacology annotations from DrugBank database show that these two drugs are both used for nutritional supplementation. The target angiotensin-converting enzyme (P12821) is related to five small-molecule drugs. We identify five similar small molecule drug pairs based on the spatial position comparison of DTICs (Supplementary Table S7). The annotations show that these five small-molecule drugs used in the treatment of hypertension. However, the method based on the distribution of all the interaction surfaces atoms could not identify these drug pairs. Therefore, it is more meaningful to consider both drug center and the distribution of all the interaction surfaces atoms.
Discussion
Increasing evidence reveals that the 3-dimensional structure plays a critical role in the interaction between a drug and its target proteins [23]. The specific spatial structure and binding models determine how useful a drug will be in treating a given disease [52]. Recent advances in the computational prediction of drug–target interactions have gained increasing attention both in the drug repositioning process and in drug target identification. Compared to the computational-based approaches, our research focuses mainly on the influence that the 3-dimensional structure has on the molecular functions, discovering potential drug–target binding features and inferring the drug efficacy in disease treatment. Based on our method, we identify a promising drug list for drug repositioning and present a reduced drug–target pair sets that need to be validated.
According to the `guilt-by-association’ principle, similar ligands may bind with similar targets and vice versa [20, 53, 54]. A neighbor-based collaborative filtering with global effect removal (NCFGER) method estimates anti-cancer drug responses of cell lines by integrating cell line similarity networks and drug similarity networks based on the fact that similar cell lines and similar drugs exhibit similar responses [55]. In addition, a single target may have multiple distinct regions or domains that perform distinct functions [56]. A number of biological activities originate from the interaction between small-molecule ligands and their target proteins in the pockets of a protein’s 3-dimensional structure [57, 58]. There are two drug binding pockets in the AcrB protein of Escherichia coli. Large antibiotics (erythromycin and rifampin) bind to the proximal pocket. However, small antibiotics (doxorubicin and minocycline) are thought to bind to the distal binding pocket [59–61]. Therefore, it is of great significance to determine the precise binding sites and regions of a compound on its target proteins.
Recently, many computational strategies have been introduced to discover drug–target interactions. Peska et al. [62] focused on machine-learning algorithms aiming at drug-centric repositioning research. Rifaioglu et al. [63] examined and discussed the recent applications of machine-learning and deep-learning techniques in silico drug discovery which provided a novel insight into the field of computational drug discovery and developing novel bio-prediction methods. Zu et al. [64] proposed a statistical model, GIFT, which combined different chemical substructures into one unit to globally evaluate the substructure-domain interactions. In addition, much effort has been devoted to investigating the interactions between a drug and its targets based on different network modes [65–69]. Among these studies, Guney et al. [68] and Cheng et al. [69] provided new indications for studying drug–disease associations that could provide more direct indications for existing drugs [68, 69]. Our methods not only add novel insights about the drug–disease relationships but also provide 3-dimensional interaction information about a drug and its target proteins, which are important for understanding both the therapeutic and adverse effects. Furthermore, this work concentrates on the drug–target binding models at the spatial structure level, which accurately reflects the drug–target interaction status.
Recent years, many web-based drug repurposing tools have been proposed for drug repositioning/repurposing, such as ChemMapper, MeSHDD, CMap and so on [70]. In addition, many other studies apply network-based approaches (gene regulatory networks, metabolic networks, protein–protein interaction networks, etc) to drug repositioning [71]. These studies comprehensively investigated databases of drug–target interactions and highlighted the high-quality resources that have been broadly utilized; however, these researches lack specific evaluation on repositioning anti-cancer drugs. Our research focused on repositioning study for targeting anti-cancer drugs especially. We acquired 39 similar drug pairs from 141 drug pairs. The analysis of receptor-type tyrosine-protein kinase FLT3 (P36888) and its targeting drugs indicates that our results could help reveal the potential drug efficacy of existing anti-cancer drugs.
Moreover, some methods have been developed to decrease the number of drug combination experiments. NLLSS provides an efficient strategy to find potential drug combinations by integrating known synergistic drug combinations, drug–target interactions and drug chemical structures [72]. In our research, drug pairs with higher similarity score tend to have similar pharmacodynamics. We also find that our method could be used for predicting drug combinations for treating other diseases. For example, the similarity score of lenvatinib and pazopanib is 0.79. Lenvatinib is used to treat thyroid cancer. Pazopanib is used to treat renal cell cancer and advanced soft tissue sarcoma. DrugBank database does not record similar indications for these two drugs; however, studies have shown that combination of pazopanib and trametinib inhibit the growth of thyroid cancer cell lines [45].
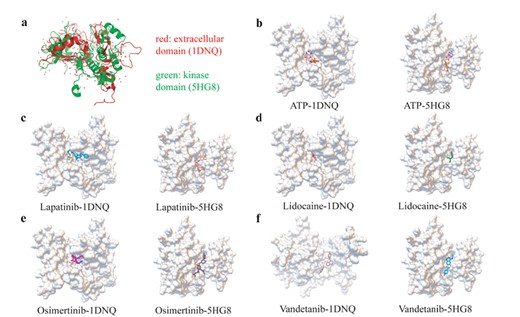
The extracellular and kinase domain comparison both by structure similarity and drug–target interactions. (A) The aligned structure of EGFR extracellular domain and kinase domain by SPalignNS. (B) The ATP molecule docking result with two EGFR domains. (C) The Lapatinib molecule docking result with two EGFR domains. (D) The lidocaine molecule docking result with two EGFR domains. (E) The osimertinib molecule docking result with two EGFR domains. (F) The vandetanib molecule docking result with two EGFR domains.
In this paper, we mainly focus on anti-cancer drug discovery. In fact, our method could extend to other domains. Based on the spatial position comparison of DTICs, we identify five and seven similar drug pairs for angiotensin-converting enzyme (P12821) and type-1 angiotensin II receptor (P30556), respectively (Supplementary Table S2). All of these drugs are used to treat hypertension. In addition, many researchers have indicated that RNA are a new type of small molecule drug targets that play an important role in the development of human complex diseases, especially for microRNAs [73–76]. We apply our method to evaluate of drug efficacy based on drug–microRNA associations. We obtained miR-21 and its targeted six drugs (fluorouracil, berberine, curcumin, epigallocatechin gallate, genistein, resveratrol) relationships from NRDTD database [77]. The miR-21 precursor’s 3-dimensional structure file is extracted from PDB databases (PDB ID: 5UZZ). By repeating the process based on the spatial position comparison of DTICs, we got a drug pair with a higher similarity score (|${r}_{Berberine- Genistein}=0.99$|; Supplementary Table S8). The DrugBank database annotation information shows that genistein is being studied in clinical trials as a treatment for prostate cancer. Liu et al. [78] suggest that berberine should be developed as a pharmacological agent for use in combination with other anti-cancer drug for treating metastatic prostate cancer. The annotation information are consistent with our results.
The SMDA method could also be used in exploring potential drug mechanisms by discovery new drug targeted domains. In the research of target protein epidermal growth factor receptor (EGFR), we find that the paired drugs had the potential to bind to the extracellular domain of EGFR, in addition to binding to the kinase domain. In order to certify this unexpected phenomenon, we perform domain structure similarity comparison, drug–target sampling docking analysis and comparison and literature retrieval annotation. For the domain structure similarity analysis, by using SPalignNS [79], we find that the overlap structure of the kinase domain and extracellular domain for EGFR reached 47.67% (Figure 6A). The structural regions associated with the drugs combination have larger spatial consistency, exert potential ability of lapatinib, lidocaine, osimertinib and vandetanib to interact with both the kinase domain and extracellular domain. For the drug–target sampling docking analysis and comparison, we find that for ATP and the other four paired drugs described above (besides the interaction of vandetanib with extracellular domain), they have a similar and stable docking position both in the kinase domain and the extracellular domain (Figure 6B–F). Moreover, the further literature annotation found that lapatinib could interact with the extracellular domain of HER2 protein, another member of ERBB family as well as EGFR, and lead to a better experimental and clinical outcome in breast cancer [80, 81].
This research provides an overview of targeted drug repositioning and development in relation to the drug’s 3-dimensional structure and highlights the challenges and future potential for targeted cancer therapies. In addition, the literature-validated drug–disease relationships of the anti-cancer drugs provide great opportunities for drug repositioning, finding new targets or functions for these drugs and offering new insights into the understanding of their molecular mechanisms of drug actions. We analyze and compare drug’s efficacy that targeting the same protein based on the SMDA method. For the inconsistency of space coordinate system and characteristics of AutoDockVina software, drug pairs that targeting different proteins were not analyzed in the SMDA process. In the future, we will develop methods to analyze the drug efficacy among different targets. Although we find that our method will provide some false positive rates because of multi-targeted drugs or drug combination therapy cases, our findings are particularly helpful for understanding the unknown pharmacological effects of existing drugs and identifying their potential new applications.
In summary, this study highlights the potential value of 3-dimensional structure-based approaches for identifying potentially druggable targets and new anti-cancer indications for the existing drugs to accelerate the development of molecular-targeted cancer therapeutics.
The drug efficacy could be determined by spatial conformation of drug–target interaction, which inspires us to design a method of pharmacodynamic comparative analysis method (SMDA) based on docking sampling.
The SMDA method focuses on the relationship of spatial position between drug center and its target interaction surface, which better reflects the spatial interaction consistence of known effective drug and its target.
The analysis and application of SMDA method, especially in anti-cancer drugs, could shed light on landscape of identifying potentially novel targets and pharmic efficiency of targeted drugs, as well as accelerating the development of anti-cancer drug repositioning.
Acknowledgements
We thank all support from the Training Center for Students Innovation and Entrepreneurship Education, Harbin Medical University, Harbin 150081, China.
Funding
National Natural Science Foundation of China (grant number 31501062 and 31801098); the Research Projects of Education Department of Heilongjiang Province (grant numbers 1254G041 and 12511273); the Research Project of Health Department of Heilongjiang Province (grant number 2011-249); Harbin Science and Technology Bureau project (grant number RC2013QN004112); the Fundamental Research Funds for the Provincial Universities (grant number 2017JCZX50); and the Internal Fund Project of Eye Hospital of Wenzhou Medical University (grant number YJGG20181001).
Yu Ding is a graduate student jointly trained by Harbin Medical University and Wenzhou Medical University. His main research interests are RNA and protein structural biology.
Hong Wang is an assistant professor at Harbin Medical University. Her research interests include complex disease bioinformatics and molecular genetics.
Hewei Zheng a graduate student jointly trained by Harbin Medical University and Wenzhou Medical University. His main research interests are RNA molecular genetics and structural bioinformatics.
Lianzong Wang is a graduate student jointly trained by Harbin Medical University and Wenzhou Medical University. His main research interests are lncRNA regulatory bioinformatics and system biology.
Guosi Zhang a graduate student jointly trained by Harbin Medical University and Wenzhou Medical University. His main research interests are RNA molecular genetics and structural bioinformatics.
Jiaxin Yang is a graduate student jointly trained by Harbin Medical University and Wenzhou Medical University. Her main research interests are microbiological genomics and computational biology.
Xiaoyan Lu is a graduate student jointly trained by Harbin Medical University and Wenzhou Medical University. Her main research interests are RNA molecular genetics and structural bioinformatics.
Yu Bai a graduate student jointly trained by Harbin Medical University and Wenzhou Medical University. Her main research interests are RNA molecular genetics and structural bioinformatics.
Haotian Zhang is an undergraduate student jointly trained by Harbin Medical University and Wenzhou Medical University. His main research interests include bioinformatics algorithm and structural bioinformatics.
Jing Li is a graduate student jointly trained by Harbin Medical University and Wenzhou Medical University. Her main research interests are RNA molecular genetics and structural bioinformatics.
Wenyan Gao is an undergraduate student jointly trained by Harbin Medical University and Wenzhou Medical University. Her main research interests are ncRNA regulation mechanism.
Fukun Chen is an undergraduate student jointly trained by Harbin Medical University and Wenzhou Medical University. His main research interests are RNA molecular genetics and structural bioinformatics.
Shui Hu is an undergraduate student jointly trained by Harbin Medical University and Wenzhou Medical University. His main research interests are ncRNA regulation mechanism.
Jingqi Wu is a graduate student jointly trained by Harbin Medical University and Wenzhou Medical University. Her main research interests are RNA molecular genetics and structural bioinformatics.
Liangde Xu is an associate professor at the School of Ophthalmology & Optometry and Eye Hospital, School of Biomedical Engineering at Wenzhou Medical University, and cooperates with Harbin Medical University. His main research interests include complex disease bioinformatics and molecular genetics. The College of Bioinformatics Science and Technology, of Harbin Medical University, is one of the largest computational biology research organizations in China, which has made valuable achievements in bioinformatics research of complex diseases. The School of Ophthalmology & Optometry and Eye Hospital, School of Biomedical Engineering of Wenzhou Medical University, is one of the most famous ophthalmic medical organizations in China, which has achieved a high level of research in ophthalmic diseasemechanism, biomedical big data and biomedical materials.
References
Wang C,
Author notes
Yu Ding, Hong Wang, and Hewei Zheng contribute equally to this work.