-
PDF
- Split View
-
Views
-
Cite
Cite
Cassandra M J Wannan, Barnaby Nelson, Jean Addington, Kelly Allott, Alan Anticevic, Celso Arango, Justin T Baker, Carrie E Bearden, Tashrif Billah, Sylvain Bouix, Matthew R Broome, Kate Buccilli, Kristin S Cadenhead, Monica E Calkins, Tyrone D Cannon, Guillermo Cecci, Eric Yu Hai Chen, Kang Ik K Cho, Jimmy Choi, Scott R Clark, Michael J Coleman, Philippe Conus, Cheryl M Corcoran, Barbara A Cornblatt, Covadonga M Diaz-Caneja, Dominic Dwyer, Bjørn H Ebdrup, Lauren M Ellman, Paolo Fusar-Poli, Liliana Galindo, Pablo A Gaspar, Carla Gerber, Louise Birkedal Glenthøj, Robert Glynn, Michael P Harms, Leslie E Horton, René S Kahn, Joseph Kambeitz, Lana Kambeitz-Ilankovic, John M Kane, Tina Kapur, Matcheri S Keshavan, Sung-Wan Kim, Nikolaos Koutsouleris, Marek Kubicki, Jun Soo Kwon, Kerstin Langbein, Kathryn E Lewandowski, Gregory A Light, Daniel Mamah, Patricia J Marcy, Daniel H Mathalon, Patrick D McGorry, Vijay A Mittal, Merete Nordentoft, Angela Nunez, Ofer Pasternak, Godfrey D Pearlson, Jesus Perez, Diana O Perkins, Albert R Powers, David R Roalf, Fred W Sabb, Jason Schiffman, Jai L Shah, Stefan Smesny, Jessica Spark, William S Stone, Gregory P Strauss, Zailyn Tamayo, John Torous, Rachel Upthegrove, Mark Vangel, Swapna Verma, Jijun Wang, Inge Winter-van Rossum, Daniel H Wolf, Phillip Wolff, Stephen J Wood, Alison R Yung, Carla Agurto, Mario Alvarez-Jimenez, Paul Amminger, Marco Armando, Ameneh Asgari-Targhi, John Cahill, Ricardo E Carrión, Eduardo Castro, Suheyla Cetin-Karayumak, M Mallar Chakravarty, Youngsun T Cho, David Cotter, Simon D’Alfonso, Michaela Ennis, Shreyas Fadnavis, Clara Fonteneau, Caroline Gao, Tina Gupta, Raquel E Gur, Ruben C Gur, Holly K Hamilton, Gil D Hoftman, Grace R Jacobs, Johanna Jarcho, Jie Lisa Ji, Christian G Kohler, Paris Alexandros Lalousis, Suzie Lavoie, Martin Lepage, Einat Liebenthal, Josh Mervis, Vishnu Murty, Spero C Nicholas, Lipeng Ning, Nora Penzel, Russell Poldrack, Pablo Polosecki, Danielle N Pratt, Rachel Rabin, Habiballah Rahimi Eichi, Yogesh Rathi, Avraham Reichenberg, Jenna Reinen, Jack Rogers, Bernalyn Ruiz-Yu, Isabelle Scott, Johanna Seitz-Holland, Vinod H Srihari, Agrima Srivastava, Andrew Thompson, Bruce I Turetsky, Barbara C Walsh, Thomas Whitford, Johanna T W Wigman, Beier Yao, Hok Pan Yuen, Uzair Ahmed, Andrew (Jin Soo) Byun, Yoonho Chung, Kim Do, Larry Hendricks, Kevin Huynh, Clark Jeffries, Erlend Lane, Carsten Langholm, Eric Lin, Valentina Mantua, Gennarina Santorelli, Kosha Ruparel, Eirini Zoupou, Tatiana Adasme, Lauren Addamo, Laura Adery, Munaza Ali, Andrea Auther, Samantha Aversa, Seon-Hwa Baek, Kelly Bates, Alyssa Bathery, Johanna M M Bayer, Rebecca Beedham, Zarina Bilgrami, Sonia Birch, Ilaria Bonoldi, Owen Borders, Renato Borgatti, Lisa Brown, Alejandro Bruna, Holly Carrington, Rolando I Castillo-Passi, Justine Chen, Nicholas Cheng, Ann Ee Ching, Chloe Clifford, Beau-Luke Colton, Pamela Contreras, Sebastián Corral, Stefano Damiani, Monica Done, Andrés Estradé, Brandon Asika Etuka, Melanie Formica, Rachel Furlan, Mia Geljic, Carmela Germano, Ruth Getachew, Mathias Goncalves, Anastasia Haidar, Jessica Hartmann, Anna Jo, Omar John, Sarah Kerins, Melissa Kerr, Irena Kesselring, Honey Kim, Nicholas Kim, Kyle Kinney, Marija Krcmar, Elana Kotler, Melanie Lafanechere, Clarice Lee, Joshua Llerena, Christopher Markiewicz, Priya Matnejl, Alejandro Maturana, Aissata Mavambu, Rocío Mayol-Troncoso, Amelia McDonnell, Alessia McGowan, Danielle McLaughlin, Rebecca McIlhenny, Brittany McQueen, Yohannes Mebrahtu, Martina Mensi, Christy Lai Ming Hui, Yi Nam Suen, Stephanie Ming Yin Wong, Neal Morrell, Mariam Omar, Alice Partridge, Christina Phassouliotis, Anna Pichiecchio, Pierluigi Politi, Christian Porter, Umberto Provenzani, Nicholas Prunier, Jasmine Raj, Susan Ray, Victoria Rayner, Manuel Reyes, Kate Reynolds, Sage Rush, Cesar Salinas, Jashmina Shetty, Callum Snowball, Sophie Tod, Gabriel Turra-Fariña, Daniela Valle, Simone Veale, Sarah Whitson, Alana Wickham, Sarah Youn, Francisco Zamorano, Elissa Zavaglia, Jamie Zinberg, Scott W Woods, Martha E Shenton, Accelerating Medicines Partnership® Schizophrenia (AMP® SCZ): Rationale and Study Design of the Largest Global Prospective Cohort Study of Clinical High Risk for Psychosis, Schizophrenia Bulletin, Volume 50, Issue 3, May 2024, Pages 496–512, https://doi.org/10.1093/schbul/sbae011
- Share Icon Share
Abstract
This article describes the rationale, aims, and methodology of the Accelerating Medicines Partnership® Schizophrenia (AMP® SCZ). This is the largest international collaboration to date that will develop algorithms to predict trajectories and outcomes of individuals at clinical high risk (CHR) for psychosis and to advance the development and use of novel pharmacological interventions for CHR individuals. We present a description of the participating research networks and the data processing analysis and coordination center, their processes for data harmonization across 43 sites from 13 participating countries (recruitment across North America, Australia, Europe, Asia, and South America), data flow and quality assessment processes, data analyses, and the transfer of data to the National Institute of Mental Health (NIMH) Data Archive (NDA) for use by the research community. In an expected sample of approximately 2000 CHR individuals and 640 matched healthy controls, AMP SCZ will collect clinical, environmental, and cognitive data along with multimodal biomarkers, including neuroimaging, electrophysiology, fluid biospecimens, speech and facial expression samples, novel measures derived from digital health technologies including smartphone-based daily surveys, and passive sensing as well as actigraphy. The study will investigate a range of clinical outcomes over a 2-year period, including transition to psychosis, remission or persistence of CHR status, attenuated positive symptoms, persistent negative symptoms, mood and anxiety symptoms, and psychosocial functioning. The global reach of AMP SCZ and its harmonized innovative methods promise to catalyze the development of new treatments to address critical unmet clinical and public health needs in CHR individuals.
Introduction
The clinical high risk (CHR) for psychosis approach is used to prospectively identify people who are at increased clinical risk for developing psychotic disorders, ie, in the putatively prodromal phase of psychotic disorders.1,2 CHR criteria, which are based on a combination of attenuated symptoms, brief psychotic symptoms, and trait risk factors, are well-validated, reaching a good group-level prognostic accuracy (AUC = 0.85 at 34 months).3 Approximately one-quarter of CHR individuals convert to a psychotic disorder within a 3-year period, and 35% within 10 years.4 This rate is considerably higher than in the general population and other clinical populations, who have 0.5% and 3.9% likelihood of developing psychotic disorders over 3 years, respectively.1,2,4–6 The prevalence of CHR is 1.7% in the general population and 19.2% in clinical samples of youth.7 The CHR for psychosis criteria have been remarkably influential,8 with the Diagnostic and Statistical Manual of Mental Disorders-5 (DSM-5) including “Attenuated Psychosis Syndrome,” based on CHR criteria, as a condition for further study.9,10
Research to date, however, indicates substantial heterogeneity in clinical, neurocognitive, and neurobiological presentation, and trajectories and outcomes in the CHR population.11 Of CHR individuals who do not convert to psychosis, 48% experience full CHR remission at 12 months, and this number increases to 50% remission after 36 months.12 The remainder show progressing or persisting attenuated psychotic symptoms (APS),13 along with impaired functioning,14 cognition,15 and other symptoms.16 This high degree of heterogeneity in clinical course makes stratification of CHR individuals or prediction of individual-level outcomes using clinical information and/or biomarkers difficult but nonetheless of great importance. For example, it is challenging to match existing one-size-fits-all CHR interventions to the individual needs and phenotypes presented by CHR individuals and/or to develop new treatments that target individual pathoetiological mechanisms underlying variable CHR course. Reliable prognostic and predictive biomarkers are thus urgently required to meet these goals. In the current context, the term “biomarker” is used to refer broadly to cognitive, neuroimaging, digital health technology-derived, genetic, and biological markers.
Despite progress in identifying and validating predictive biomarkers across major international consortia,17–19 and good prognostic accuracy at the group level,3 current CHR prediction models do not reliably predict clinical course with the sufficient individual-level precision needed to yield clinically personalized approaches.20 Furthermore, the available individualized clinical prediction models are difficult to implement in real-world settings (fewer than 1% are being implemented in clinical care),21 largely because of heterogeneous assessment measurements. Thus, there is a need for well-powered, internationally coordinated efforts to better evaluate prospectively collected markers that can potentially inform interventional studies via state-of-the-art behavioral, neurobiological, and genetic measures. Such approaches would have 3 major benefits: (1) enhanced individual-level prediction of the full spectrum of outcomes in the CHR population; (2) harmonized assessment measurements that can facilitate transportability across global clinical settings and, therefore, support real-world implementation of precision psychiatry; and (3) identification and refinement of etiological mechanisms driving psychosis and psychiatric disorders more broadly. These elements are needed to develop novel and targeted precision therapeutics, to support the selection of primary clinical endpoints for future clinical trials, and to stratify patient samples optimally for clinical trials targeting those outcomes.
Accelerating Medicines Partnership Schizophrenia
The Accelerating Medicines Partnership (AMP) is a public-private partnership between the National Institutes of Health (NIH), the U.S. Food and Drug Administration (FDA), the European Medicines Agency (EMA), and multiple public and private organizations. Managed through the Foundation for the NIH (FNIH), the AMP program aims to accelerate new and effect therapies to patients. AMP projects work toward this goal by identifying clinically relevant disease targets, improving identification of patients most likely to respond to particular treatment, and safely reducing the development timelines for life-saving therapies and improvements in patient outcomes.22
AMP Schizophrenia (AMP SCZ) marks the first AMP initiative directed towards a neuropsychiatric disorder. AMP SCZ partners include government (NIH, FDA, EMA), industry (Boehringer-Ingelheim, Janssen, Otsuka), and nonprofit (American Psychiatric Association Foundation, National Alliance on Mental Illness, One Mind, Schizophrenia, and Psychosis Action Alliance, Wellcome)23 partners. The approach is precompetitive, ie, research is conducted cooperatively without potential marketing activities or patenting, with the ultimate goal of accelerating the development of new therapeutics.
The goals of AMP SCZ are multifaceted, including: (1) developing measures that further define early stages of risk and prediction of the likelihood of progression to psychosis and other clinical endpoints; (2) generating tools that will facilitate the development of early-stage interventions to attenuate, delay, or prevent transition to psychosis in high-risk individuals; and (3) improving CHR clinical and functional outcomes. Such tools will be utilized in clinical trials to test new interventions for preventing or reversing various adverse outcomes in this population. AMP SCZ harnesses the power of open science to accelerate the research and development process and to advance promising therapies for CHR populations. As such, the planning and design of AMP SCZ addresses current recommendations for CHR prediction research, which include: (1) harmonizing CHR definition and assessment measures; (2) providing a data capture and aggregation infrastructure to support multiple data types and provide quality assessment; (3) harmonizing study design, measures, and infrastructure across research networks; (4) extending the scope of prediction beyond transition to psychosis; (5) creating collaborative, open-science research networks; and (6) creating common modeling platforms and open-source model libraries.24 The purpose of the current article is to provide a high-level overview of the aims and structure of AMP SCZ, with a summary of study design and methodology. Subsequent articles will be published providing further methodological detail about each data domain.
Project Aims
The specific aims of AMP SCZ are to:
1. Establish a global research network
AMP SCZ has established an international research network focused on recruiting young people at CHR for psychosis from 43 international study sites (see figure 1). The project’s large, international scope will ensure the generalizability of AMP SCZ findings.
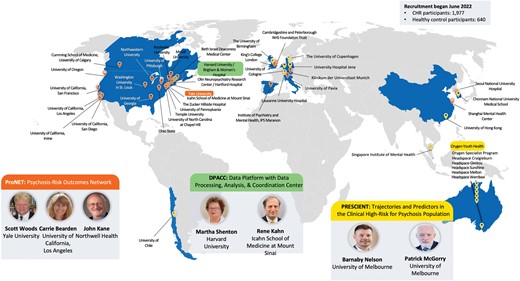
Accelerating Medicines Partnership Schizophrenia research networks and study sites.
2. Develop and validate tools for predicting individual outcomes
AMP SCZ will identify multimodal biomarkers that can be used to predict individualized clinical trajectories, including the likelihood of progression to psychosis, and illuminate heterogeneity within the CHR syndrome.
3. Set the stage for evaluating preventive as well as symptom and functioning-focused treatments
AMP SCZ will create a research framework that can improve clinical trial design and implementation, develop better approaches for measuring treatment response, and create more accurate tools for detecting early-stage risk. This will lay the foundation for the faster development of more effective treatments.
4. Create an accessible data repository
Data structures and dictionaries for each data type will be created to enable broad sharing. Data from AMP SCZ will be available to the scientific community through the National Institute of Mental Health (NIMH) Data Archive (NDA).
Research Networks and Data Processing Analysis and Coordination Center
Two international networks have been established under the framework of AMP SCZ: Psychosis-Risk Outcomes Network (ProNET) and Prediction Scientific Global Consortium (PRESCIENT). Additionally, the Data Processing, Analysis, and Coordination Center (DPACC) will integrate and analyze the data generated in AMP SCZ and will utilize other key existing clinical high-risk cohorts to inform the AMP SCZ analyses. New tools developed for infrastructure pipelines and analyses will be made available to the scientific research community through the NDA. An overview of the AMP SCZ networks and study sites is provided in figure 1.
ProNET.
ProNET is led by S.W. Woods (Yale), C.E. Bearden (University of California, Los Angeles), and J.M. Kane (Zucker School of Medicine). This network comprises 28 international sites, including 19 in the United States, 2 in Canada, 3 in continental Europe, 2 in the United Kingdom, and 2 in Asia. ProNET is managed via a hub centered at Yale and Zucker.
PRESCIENT.
The PRESCIENT network is led by B. Nelson and P. McGorry (Orygen and University of Melbourne). The PRESCIENT network comprises 15 international sites, including the hub site in Melbourne, Australia (Orygen, consisting of the Orygen Specialist Program and 5 headspace clinics, n = 6), 3 in Asia, 4 in Europe, 1 in the United Kingdom, and 1 in South America.
DPACC.
DPACC is led by M.E. Shenton (Brigham & Women’s Hospital and Harvard Medical School) and R.S. Kahn (Icahn School of Medicine at Mount Sinai). The center is responsible for the data capture of information from ProNET and PRESCIENT networks, and setting up the infrastructure for data flow and data quality control, in addition to integration and analysis of the data, the latter in conjunction with members of the research networks and AMP SCZ partners. Members of DPACC are also leading analyses of key preexisting CHR-related datasets prior to applying them to AMP SCZ data. The DPACC team is divided into 4 Cores: (1) Coordination and Monitoring; (2) Data Management, Processing, and Archiving; (3) Data Analysis and Visualization; and (4) Dissemination of Resources, which includes developing and maintaining a website (ampscz.org) and making the tools developed available to the general research community.
AMP SCZ Working Groups
Several working groups have been organized within AMP SCZ to oversee each assessment domain, including data capture and processing, clinical ascertainment and outcomes, electrophysiology, neuroimaging, cognition, genetics and fluid biomarkers, digital health technology-derived measures, and speech/facial expression (see table 1). Working groups for data analysis, co-enrollment, and outreach/dissemination of resources have also been established. The perspective of individuals with lived experience has been included throughout the AMP SCZ project, including the conception, protocol design, and ongoing conduct of the study.25 The co-chair of the AMP SCZ Steering Committee brings lived experience to the study, which is further supported by advocacy group members who serve on the AMP SCZ Steering Committee. Together, they supported the development of the AMP SCZ research plan and reviewed the study protocol yielding insight into assessment measures and perceived burden from the perspective of the study participant. Lived experience partners are involved in reviewing recruitment and the demographics of the sample to be consistent with the CHR population. Also, they present at AMP SCZ consortium meetings and ensure that the patient voice is incorporated in the project priorities to bridge the gap between science and real-world needs.25
Working Group . | Leadership . | Function . |
---|---|---|
Team A: Data capture and processing pipelines | Sylvain Bouix | Develop overall architecture for data flow and management across research networks, DPACC, and NDA. |
Team B: CHR ascertainment and clinical outcomes | Jean Addington Alison Yung | Develop ascertainment and clinical outcome measures. Monitor clinical training, ascertainment, and clinical assessments. Provide advice for data collection and preparation of data for the analysis of clinical measures. |
Team C: Electrophysiology (EEG) | Daniel Mathalon Gregory Light | Develop and program EEG tasks, create training materials and Standard Operating Procedures (SOP) for set up and running of EEG sessions; create automated data processing pipelines that generate quality control measures, ERP waveforms, and topographic maps; develop web-based dashboard for display and review of each participant’s data to support QC and site-specific concerns, and to troubleshoot problems or challenges with EEG acquisition. |
Team D: Neuroimaging | Michael Harms Ofer Pasternak | Develop MRI protocol for all MRI platforms, create training materials and SOP documents. Monitor ongoing data acquisition and work with sites to bring acquisitions into compliance following protocol deviations. |
Team E: Cognition | Kelly Allott Bill Stone | Develop cognition assessment protocols; training and certification of assessors in cognitive assessments; monitoring and quality control; advise on outcome data and analyses. |
Team F: Genetics and fluid biomarkers | Diana Perkins Scott Clark | Develop biosampling protocols and metadata; develop and monitor laboratory credentialing program; develop and review process quality benchmarks; provide technical advice for quality issues; develop genomic and fluid marker-related hypotheses and recommendations for biomarker selection. |
Team G: Digital biomarkers | Justin Baker, John Torous | Develop and maintain digital phenotyping pipelines; train sites in mindLAMP (smartphone app) and use of a wearable device; ensure data quality, transform raw data into behavioral features. |
Team H: Speech | Phillip Wolff, Guillermo Cecchi, Cheryl Corcoran | Create and automate a processing pipeline to extract linguistic, cognitive, emotional, and behavioral features from speech and facial data. Provide site training and certification for interviewing. Safeguard data quality at each step in the analysis. |
Co-enrollment | John Kane | Review studies co-enrolling with AMP SCZ across recruitment sites to ensure AMP SCZ data integrity and minimize participant and rater burden. |
Dissemination and Outreach | Tina Kapur, Eve Lewandowski | Develop and maintain processes and products to disseminate information from the AMP SCZ program to the broader community of researchers, clinicians, and families. |
Working Group . | Leadership . | Function . |
---|---|---|
Team A: Data capture and processing pipelines | Sylvain Bouix | Develop overall architecture for data flow and management across research networks, DPACC, and NDA. |
Team B: CHR ascertainment and clinical outcomes | Jean Addington Alison Yung | Develop ascertainment and clinical outcome measures. Monitor clinical training, ascertainment, and clinical assessments. Provide advice for data collection and preparation of data for the analysis of clinical measures. |
Team C: Electrophysiology (EEG) | Daniel Mathalon Gregory Light | Develop and program EEG tasks, create training materials and Standard Operating Procedures (SOP) for set up and running of EEG sessions; create automated data processing pipelines that generate quality control measures, ERP waveforms, and topographic maps; develop web-based dashboard for display and review of each participant’s data to support QC and site-specific concerns, and to troubleshoot problems or challenges with EEG acquisition. |
Team D: Neuroimaging | Michael Harms Ofer Pasternak | Develop MRI protocol for all MRI platforms, create training materials and SOP documents. Monitor ongoing data acquisition and work with sites to bring acquisitions into compliance following protocol deviations. |
Team E: Cognition | Kelly Allott Bill Stone | Develop cognition assessment protocols; training and certification of assessors in cognitive assessments; monitoring and quality control; advise on outcome data and analyses. |
Team F: Genetics and fluid biomarkers | Diana Perkins Scott Clark | Develop biosampling protocols and metadata; develop and monitor laboratory credentialing program; develop and review process quality benchmarks; provide technical advice for quality issues; develop genomic and fluid marker-related hypotheses and recommendations for biomarker selection. |
Team G: Digital biomarkers | Justin Baker, John Torous | Develop and maintain digital phenotyping pipelines; train sites in mindLAMP (smartphone app) and use of a wearable device; ensure data quality, transform raw data into behavioral features. |
Team H: Speech | Phillip Wolff, Guillermo Cecchi, Cheryl Corcoran | Create and automate a processing pipeline to extract linguistic, cognitive, emotional, and behavioral features from speech and facial data. Provide site training and certification for interviewing. Safeguard data quality at each step in the analysis. |
Co-enrollment | John Kane | Review studies co-enrolling with AMP SCZ across recruitment sites to ensure AMP SCZ data integrity and minimize participant and rater burden. |
Dissemination and Outreach | Tina Kapur, Eve Lewandowski | Develop and maintain processes and products to disseminate information from the AMP SCZ program to the broader community of researchers, clinicians, and families. |
Working Group . | Leadership . | Function . |
---|---|---|
Team A: Data capture and processing pipelines | Sylvain Bouix | Develop overall architecture for data flow and management across research networks, DPACC, and NDA. |
Team B: CHR ascertainment and clinical outcomes | Jean Addington Alison Yung | Develop ascertainment and clinical outcome measures. Monitor clinical training, ascertainment, and clinical assessments. Provide advice for data collection and preparation of data for the analysis of clinical measures. |
Team C: Electrophysiology (EEG) | Daniel Mathalon Gregory Light | Develop and program EEG tasks, create training materials and Standard Operating Procedures (SOP) for set up and running of EEG sessions; create automated data processing pipelines that generate quality control measures, ERP waveforms, and topographic maps; develop web-based dashboard for display and review of each participant’s data to support QC and site-specific concerns, and to troubleshoot problems or challenges with EEG acquisition. |
Team D: Neuroimaging | Michael Harms Ofer Pasternak | Develop MRI protocol for all MRI platforms, create training materials and SOP documents. Monitor ongoing data acquisition and work with sites to bring acquisitions into compliance following protocol deviations. |
Team E: Cognition | Kelly Allott Bill Stone | Develop cognition assessment protocols; training and certification of assessors in cognitive assessments; monitoring and quality control; advise on outcome data and analyses. |
Team F: Genetics and fluid biomarkers | Diana Perkins Scott Clark | Develop biosampling protocols and metadata; develop and monitor laboratory credentialing program; develop and review process quality benchmarks; provide technical advice for quality issues; develop genomic and fluid marker-related hypotheses and recommendations for biomarker selection. |
Team G: Digital biomarkers | Justin Baker, John Torous | Develop and maintain digital phenotyping pipelines; train sites in mindLAMP (smartphone app) and use of a wearable device; ensure data quality, transform raw data into behavioral features. |
Team H: Speech | Phillip Wolff, Guillermo Cecchi, Cheryl Corcoran | Create and automate a processing pipeline to extract linguistic, cognitive, emotional, and behavioral features from speech and facial data. Provide site training and certification for interviewing. Safeguard data quality at each step in the analysis. |
Co-enrollment | John Kane | Review studies co-enrolling with AMP SCZ across recruitment sites to ensure AMP SCZ data integrity and minimize participant and rater burden. |
Dissemination and Outreach | Tina Kapur, Eve Lewandowski | Develop and maintain processes and products to disseminate information from the AMP SCZ program to the broader community of researchers, clinicians, and families. |
Working Group . | Leadership . | Function . |
---|---|---|
Team A: Data capture and processing pipelines | Sylvain Bouix | Develop overall architecture for data flow and management across research networks, DPACC, and NDA. |
Team B: CHR ascertainment and clinical outcomes | Jean Addington Alison Yung | Develop ascertainment and clinical outcome measures. Monitor clinical training, ascertainment, and clinical assessments. Provide advice for data collection and preparation of data for the analysis of clinical measures. |
Team C: Electrophysiology (EEG) | Daniel Mathalon Gregory Light | Develop and program EEG tasks, create training materials and Standard Operating Procedures (SOP) for set up and running of EEG sessions; create automated data processing pipelines that generate quality control measures, ERP waveforms, and topographic maps; develop web-based dashboard for display and review of each participant’s data to support QC and site-specific concerns, and to troubleshoot problems or challenges with EEG acquisition. |
Team D: Neuroimaging | Michael Harms Ofer Pasternak | Develop MRI protocol for all MRI platforms, create training materials and SOP documents. Monitor ongoing data acquisition and work with sites to bring acquisitions into compliance following protocol deviations. |
Team E: Cognition | Kelly Allott Bill Stone | Develop cognition assessment protocols; training and certification of assessors in cognitive assessments; monitoring and quality control; advise on outcome data and analyses. |
Team F: Genetics and fluid biomarkers | Diana Perkins Scott Clark | Develop biosampling protocols and metadata; develop and monitor laboratory credentialing program; develop and review process quality benchmarks; provide technical advice for quality issues; develop genomic and fluid marker-related hypotheses and recommendations for biomarker selection. |
Team G: Digital biomarkers | Justin Baker, John Torous | Develop and maintain digital phenotyping pipelines; train sites in mindLAMP (smartphone app) and use of a wearable device; ensure data quality, transform raw data into behavioral features. |
Team H: Speech | Phillip Wolff, Guillermo Cecchi, Cheryl Corcoran | Create and automate a processing pipeline to extract linguistic, cognitive, emotional, and behavioral features from speech and facial data. Provide site training and certification for interviewing. Safeguard data quality at each step in the analysis. |
Co-enrollment | John Kane | Review studies co-enrolling with AMP SCZ across recruitment sites to ensure AMP SCZ data integrity and minimize participant and rater burden. |
Dissemination and Outreach | Tina Kapur, Eve Lewandowski | Develop and maintain processes and products to disseminate information from the AMP SCZ program to the broader community of researchers, clinicians, and families. |
Methods
Establishing Harmonized Assessment Protocols Across Research Networks
The assessment domains, instruments, and measurement time points to be used across the AMP SCZ consortium were decided via Working Group discussions involving representatives from all stakeholder groups (members from the 2 research networks, DPACC, NIMH, and the AMP SCZ private and public partners). A consensus-based harmonized assessment battery was developed for use across sites in both research networks. This allows data to be pooled across all AMP SCZ sites, thereby increasing statistical power and generalizability of findings. The selection of assessment domains, measures, and time points was guided by the following principles: robust existing evidence regarding their relevance for CHR outcomes; capturing data that allows for both static and dynamic predictive modeling; timing of repeat assessments that would facilitate clinical trial design; balancing comprehensive data collection with participant and assessor burden; and innovative methods.
Study Design
AMP SCZ is an observational longitudinal study examining clinical trajectories and predictors of clinical endpoints in the CHR population. Supplementary table S3 presents the schedule of assessments. The timing of outcome assessments was selected to span the breadth of clinical trial-relevant CHR endpoints. This includes attenuated positive, negative, and affective symptoms, general psychopathology, social and role functioning, CHR persistence/remission, transition to psychosis, and cognition over both the short (4 and 8 weeks) and longer term (12–104 weeks). A 2-month interval was chosen for a repeat of the biomarker assessments as there is evidence that change in biomarkers over this time period may be useful for outcome prediction.26 Additionally, change over this reasonably short time period would facilitate early stratification into subgroups for clinical trials.
Sample
The AMP SCZ research network is recruiting a large cohort of CHR young people aged 12–30 years (n = 1977) and healthy control (HC) participants (n = 640) across 43 participating sites from 13 countries (figure 1). HC are matched to the sex, age, and parental socioeconomic status level of CHR participants enrolled at each site. HC participants complete screening, baseline, 12-month, and 24-month assessments, with a subset (5 of approximately 15 on average per site) completing additional key assessments at month 2 (see supplementary table S3).
Inclusion and Exclusion Criteria
Inclusion and exclusion criteria are provided in table 2.
Inclusion Criteria General inclusion criteria: a) Aged 12–30 years inclusive, b) Ability to give informed consent (parental/guardian consent is obtained for participants aged <18 years), and c) Meeting either CHR or healthy control criteria. CHR inclusion criteria: meet CAARMS-defined (Trait Vulnerability; Attenuated Psychotic Symptoms; Brief Limited Intermittent Psychotic Symptoms)12 or Structured Interview for Prodromal Syndromes18-defined (Brief Intermittent Psychotic Syndrome Current Progression; Attenuated Positive Symptom Syndrome Current Progression; Genetic Risk and Deterioration Current Progression) diagnostic criteria for CHR determined using a newly developed instrument, the Positive SYmptoms and Diagnostic Criteria for the CAARMS Harmonized with the SIPS (PSYCHS). Healthy control inclusion criteria: a) Do not meet CHR criteria or have a current or past Cluster A personality disorder, b) Not receiving any current treatment with psychotropic medication, and c) Do not have a family history (in first-degree relatives) of psychotic spectrum disorders. |
Exclusion Criteria a) Antipsychotic medication exposure equivalent to a total lifetime haloperidol dose of >50 mg, estimated based on available information, or current antipsychotic medication at time of baseline assessment, b) Documented history of intellectual disability, c) Past or current clinically relevant central nervous system disorder, d) Traumatic brain injury rated 7 or above on the Traumatic Brain Injury screening instrument,19 or e) Current or past psychotic disorder. |
Conversion Criteria a) At least 1 full threshold positive psychotic symptom as operationalized using the PSYCHS18 for 1 week or longer and occurring (i) for more than an hour a day, 3–6 days per week OR (ii) daily for less than 1 h, or b) At least 1 full threshold positive psychotic symptom with the above frequency but lasting less than 1 week in the context of newly prescribed or newly increased antipsychotic medication, or c) At least 1 full threshold positive symptom that is imminently dangerous (physically or to personal dignity or to social/family networks). |
Inclusion Criteria General inclusion criteria: a) Aged 12–30 years inclusive, b) Ability to give informed consent (parental/guardian consent is obtained for participants aged <18 years), and c) Meeting either CHR or healthy control criteria. CHR inclusion criteria: meet CAARMS-defined (Trait Vulnerability; Attenuated Psychotic Symptoms; Brief Limited Intermittent Psychotic Symptoms)12 or Structured Interview for Prodromal Syndromes18-defined (Brief Intermittent Psychotic Syndrome Current Progression; Attenuated Positive Symptom Syndrome Current Progression; Genetic Risk and Deterioration Current Progression) diagnostic criteria for CHR determined using a newly developed instrument, the Positive SYmptoms and Diagnostic Criteria for the CAARMS Harmonized with the SIPS (PSYCHS). Healthy control inclusion criteria: a) Do not meet CHR criteria or have a current or past Cluster A personality disorder, b) Not receiving any current treatment with psychotropic medication, and c) Do not have a family history (in first-degree relatives) of psychotic spectrum disorders. |
Exclusion Criteria a) Antipsychotic medication exposure equivalent to a total lifetime haloperidol dose of >50 mg, estimated based on available information, or current antipsychotic medication at time of baseline assessment, b) Documented history of intellectual disability, c) Past or current clinically relevant central nervous system disorder, d) Traumatic brain injury rated 7 or above on the Traumatic Brain Injury screening instrument,19 or e) Current or past psychotic disorder. |
Conversion Criteria a) At least 1 full threshold positive psychotic symptom as operationalized using the PSYCHS18 for 1 week or longer and occurring (i) for more than an hour a day, 3–6 days per week OR (ii) daily for less than 1 h, or b) At least 1 full threshold positive psychotic symptom with the above frequency but lasting less than 1 week in the context of newly prescribed or newly increased antipsychotic medication, or c) At least 1 full threshold positive symptom that is imminently dangerous (physically or to personal dignity or to social/family networks). |
Inclusion Criteria General inclusion criteria: a) Aged 12–30 years inclusive, b) Ability to give informed consent (parental/guardian consent is obtained for participants aged <18 years), and c) Meeting either CHR or healthy control criteria. CHR inclusion criteria: meet CAARMS-defined (Trait Vulnerability; Attenuated Psychotic Symptoms; Brief Limited Intermittent Psychotic Symptoms)12 or Structured Interview for Prodromal Syndromes18-defined (Brief Intermittent Psychotic Syndrome Current Progression; Attenuated Positive Symptom Syndrome Current Progression; Genetic Risk and Deterioration Current Progression) diagnostic criteria for CHR determined using a newly developed instrument, the Positive SYmptoms and Diagnostic Criteria for the CAARMS Harmonized with the SIPS (PSYCHS). Healthy control inclusion criteria: a) Do not meet CHR criteria or have a current or past Cluster A personality disorder, b) Not receiving any current treatment with psychotropic medication, and c) Do not have a family history (in first-degree relatives) of psychotic spectrum disorders. |
Exclusion Criteria a) Antipsychotic medication exposure equivalent to a total lifetime haloperidol dose of >50 mg, estimated based on available information, or current antipsychotic medication at time of baseline assessment, b) Documented history of intellectual disability, c) Past or current clinically relevant central nervous system disorder, d) Traumatic brain injury rated 7 or above on the Traumatic Brain Injury screening instrument,19 or e) Current or past psychotic disorder. |
Conversion Criteria a) At least 1 full threshold positive psychotic symptom as operationalized using the PSYCHS18 for 1 week or longer and occurring (i) for more than an hour a day, 3–6 days per week OR (ii) daily for less than 1 h, or b) At least 1 full threshold positive psychotic symptom with the above frequency but lasting less than 1 week in the context of newly prescribed or newly increased antipsychotic medication, or c) At least 1 full threshold positive symptom that is imminently dangerous (physically or to personal dignity or to social/family networks). |
Inclusion Criteria General inclusion criteria: a) Aged 12–30 years inclusive, b) Ability to give informed consent (parental/guardian consent is obtained for participants aged <18 years), and c) Meeting either CHR or healthy control criteria. CHR inclusion criteria: meet CAARMS-defined (Trait Vulnerability; Attenuated Psychotic Symptoms; Brief Limited Intermittent Psychotic Symptoms)12 or Structured Interview for Prodromal Syndromes18-defined (Brief Intermittent Psychotic Syndrome Current Progression; Attenuated Positive Symptom Syndrome Current Progression; Genetic Risk and Deterioration Current Progression) diagnostic criteria for CHR determined using a newly developed instrument, the Positive SYmptoms and Diagnostic Criteria for the CAARMS Harmonized with the SIPS (PSYCHS). Healthy control inclusion criteria: a) Do not meet CHR criteria or have a current or past Cluster A personality disorder, b) Not receiving any current treatment with psychotropic medication, and c) Do not have a family history (in first-degree relatives) of psychotic spectrum disorders. |
Exclusion Criteria a) Antipsychotic medication exposure equivalent to a total lifetime haloperidol dose of >50 mg, estimated based on available information, or current antipsychotic medication at time of baseline assessment, b) Documented history of intellectual disability, c) Past or current clinically relevant central nervous system disorder, d) Traumatic brain injury rated 7 or above on the Traumatic Brain Injury screening instrument,19 or e) Current or past psychotic disorder. |
Conversion Criteria a) At least 1 full threshold positive psychotic symptom as operationalized using the PSYCHS18 for 1 week or longer and occurring (i) for more than an hour a day, 3–6 days per week OR (ii) daily for less than 1 h, or b) At least 1 full threshold positive psychotic symptom with the above frequency but lasting less than 1 week in the context of newly prescribed or newly increased antipsychotic medication, or c) At least 1 full threshold positive symptom that is imminently dangerous (physically or to personal dignity or to social/family networks). |
Study Endpoints and Outcome Measures
The time periods of interest are 12 and 24 months post-baseline.
Primary Clinical Endpoint
Transition to Psychotic Disorder
As assessed by the Positive SYmptoms and Diagnostic Criteria for the CAARMS Harmonized with the SIPS (PSYCHS)27 by 12-month and 24-month follow-up, operationalized as per the definition in table 2.
Secondary Clinical Endpoints
Sustained Remission of CHR Status11,13
As defined by the PSYCHS27 for ≥6 months and until the last available follow-up.
Persistent CHR Status
Cases who do not meet sustained remission or transition criteria as assessed by the PSYCHS.
Other clinical endpoints of interest include relapse of CHR status, functional outcome, severity of attenuated psychotic symptoms, persistent functional impairment, persistent cognitive impairment, psychosocial functioning impairment, persistent negative symptoms, and incident and persistent nonpsychotic disorders.
Measures
The full rationale and details of the measures across domains will be published elsewhere. A summary of key features is provided below.
Clinical Measures.
AMP SCZ represents the most unique, layered, and thorough clinical assessment to be included in a biomarker study, including multidimensional measures of clinical state at baseline, change over time, and clinical outcome. The key clinical measure is the newly developed PSYCHS,27,28 which is used to define CHR criteria, assess attenuated psychotic symptoms and determine the onset of a first-episode of psychosis. The PSYCHS was developed from the Comprehensive Assessment of At-Risk Mental States (CAARMS)29 and the Structured Interview for Psychosis-Risk Syndromes (SIPS)30 and will allow data from this project to be used in conjunction with legacy data that used these tools. The Structured Clinical Interview for DSM-5 (SCID-5)-Research Version (RV)31 is used to assess DSM-5 Mood and Substance Use disorders and the SCID-5 Personality Disorders32 to assess Schizotypal Personality disorder. If participants develop threshold psychosis, the Psychotic Disorder section of the SCID-5-RV is administered to determine the type of DSM psychotic disorder. Demographic information plus medical and psychiatric history, including prior and ongoing treatment, are also being collected.
A comprehensive assessment of psychopathology includes well-established measures such as the Brief Psychiatric Rating Scale,33 the Overall Anxiety Severity and Impairment Scale,34 the Columbia Suicide Severity Rating Scale,35 and the Alcohol, Smoking and Substance Involvement Screening Test.36 Of note are the Calgary Depression Scale for Schizophrenia37 to assess depression independently of negative symptoms, which has been validated for CHR,38 and the recently developed and tested Negative Symptom Inventory-Psychosis Risk (NSI-PR) to assess a range of negative symptoms.39
A focus on Patient Reported Outcomes (PROs) is rare in CHR longitudinal studies.40 However, several important PROs will be addressed: the Patient Global Impression of Severity to assess participants’ impression of the severity of their symptoms; the Patient Reported Outcomes Measurement Information System-Sleep Disturbance41 to measure sleep quality, depth, and restoration; the Perceived Stress Scale42; the Perceived Discrimination Scale43 to determine whether participants have experienced discrimination in their lifetime; the Pubertal Development Scale44 for determining the development of secondary sexual characteristics; and, lastly, the Psychosis Polyrisk Score45 to capture exposure to a range of environmental risk factors associated with psychosis.
Assessment of social and role functioning includes: The Social and Occupational Functioning Assessment Scale,46 the Premorbid Adjustment Scale,47 and the Global Functioning (GF): Social and Role Scales.48 The GF scales each provide a single summary score that accounts for age, reflects change over time, and avoids confounding with psychiatric symptoms.
Digital Health Technologies-Derived Measures.
Digital health technologies (DHT)-derived measures are an optional aspect of the study. Informed by feedback from young people with lived experience of CHR and co-designed with their input, the study offers the following DHT-derived measures:
[1] Daily surveys: a self-report questionnaire consisting of 30 short questions on thoughts, feelings, and behaviors of the past day (eg, “Today I felt down”) is assessed every evening for 1 year on participants’ smartphone via the open-source mindLAMP app. The daily questions capture multiple domains (eg, mood, psychotic experiences, social interactions, and events). Participants can also record up to 2 min of spoken voice diaries. The surveys are available in 9 languages and the app captures relevant metadata such as response latency. Survey responses are plotted and shared in real-time with participants via the app. All data are automatically and securely transferred to study servers to enable remote research.
[2] Passive sensing data: Participants can choose to share passive sensing data gathered from their smartphone through the same mindLAMP app, namely geolocation (GPS), accelerometer, and/or screen-state (on/off) data. Participants can choose to share all data listed above, opt out of GPS, or use the app only for daily surveys. mindLAMP data are uniquely supported by a flexible Application Programming Interface (API). Digital phenotyping data can also be shared back when processed into behavioral and clinical features including metrics related to hometime, steps, sleep duration, sedentary periods, duration of screen use, and environmental exposure (eg, green space, population, etc.).49
[3] Actigraphy: Participants wear Axivity AX3 (Newcastle upon Tyne, UK) watches (accelerometers assessing 24h gross motor activity) during the first year to measure rest-activity patterns and physical/sedentary behaviors. Data are transferred to institutional servers at study visits.
Cognition.
The Wide Range Achievement Test 5 Reading subtest50 is used to estimate premorbid IQ at English language sites. However, as some countries do not typically measure reading accuracy and given the task will vary based on language, some sites are using a local version of a reading task, and others do not measure premorbid IQ. In English-speaking countries, the 2-subtest version (Vocabulary and Matrix Reasoning subtests) of the Wechsler Abbreviated Scale of Intelligence-Second Edition51 (WASI-II) is used to measure current Full-Scale IQ. As the WASI-II is only available in English, non-English-speaking sites are administering, in their local language, the Vocabulary and Matrix Reasoning subtests of the Wechsler Adult Intelligence Scale–Fourth Edition52 (WAIS-IV) for participants aged ≥16 and the Wechsler Intelligence Scale for Children–Fifth Edition53 (WISC-V) for participants aged <16 years.
Using the Penn Computerized Neurocognitive Battery54 we are measuring the following specific cognitive domains: attention (Short Penn Continuous Performance Test), working memory (Short Fractal N-Back Test), processing speed (Digit-Symbol Test), relational memory (Digit-Symbol Recall), verbal learning (Short Penn List Learning Test), visual memory (Short Visual Object Learning Test), emotion recognition (Penn Emotion Recognition Test), motor function (Short Computerized Finger-Tapping Test), and sensorimotor speed (Motor Praxis Test).
Electrophysiology.
To minimize cross-site differences in EEG hardware, identical high-impedance active electrode EEG recording systems (BrainProducts actiChamp 64-Channel system55) were leased from a vendor (Neurosig, Inc.) that also: (1) custom engineered a stimulus delivery system dedicated to the AMP SCZ paradigms that presented auditory and visual stimuli with high temporal precision, and (2) developed a software interface for EEG acquisition laptops that included set-up instructions for EEG technicians and participant task instructions in the local language. EEG measures were selected based on their previously established sensitivity to schizophrenia and/or ability to predict CHR clinical endpoints. They include: (1) Auditory mismatch negativity to pitch + duration “double-deviant” tones56,57 recorded concurrently while the participant performs a primary visual oddball task, thereby allowing simultaneous assessment of (2) Visual P300 event-related potentials (ERPs)56,58 to infrequent target circles (P3b) and novel fractal images (P3a); (3) Auditory P300 ERPs58,59 to infrequent target tones (P3b) and novel sounds (P3a); (4) Gamma oscillations assessed with 40-Hz auditory steady-state responses60,61; and (5) 1/f periodic and aperiodic components of EEG power spectra62 assessed from resting EEG (eyes open/closed). See supplementary materials for further details.
Neuroimaging.
The multimodal neuroimaging protocol was designed for a 3T MR scanner and includes structural imaging, resting-state functional MRI (rfMRI), and multi-shell diffusion-weighted scans. There are currently 37 MR scanning sites with a variety of vendors and platforms: Siemens Prisma (n = 28), Siemens Skyra (n = 4), Siemens Vida (n = 1), General Electric MR750 (n = 3), and Philips Achieva DDAS (n = 1). All sites use either 32 or 64-channel head coils. The protocol represents a synthesis of aspects of the HCP-Lifespan and ABCD Study protocols,63,64 with a cumulative scan duration of approximately 50 min. Specifically, it includes 0.8 mm isotropic structural scans (3D T1-weighted and T2-weighted scans, each ~6–7 min); 2.4 mm isotropic resting-state BOLD (acquired as 4 × 5 min runs with TR = 900 ms, TE = 35 ms, and multiband-factor = 6), and a 1.8 mm isotropic multi-shell diffusion scan (9–10 min, multiband-factor = 3). The structural and rfMRI scans are relatively well harmonized in terms of basic scan parameters across vendors and platforms, which was made possible without sacrificing much performance on the Prisma scanners, since those modalities are not dramatically affected by peak gradient strength.
However, since the different 3T MR platforms in AMP SCZ have considerable differences in peak gradient strength (Siemens Prisma: 80 mT/m; Siemens Skyra: 45 mT/m; Siemens Vida: 60 mT/m; General Electric MR750: 50 mT/m; Philips Achieva DDAS: 40 mT/m), which is particularly important for diffusion imaging, we created a “two-tier” protocol to take full advantage of the Prisma’s higher peak gradient strength. Specifically, on the Prisma, we are collecting shells with b-values of 200, 500, 1000, 2000, and 3000 s/mm2 (6, 10, 50, 50, 50 directions, respectively; TR = 3200 ms; TE = 79.4 ms). On all the other platforms we are collecting the same diffusion directions, but only for the b = 200, 500, 1000, and 2000 s/mm2 shells. Thus the non-Prisma diffusion data represents an exact subset of the Prisma diffusion protocol (albeit acquired with a longer TR and TE as well; TR = 3970–4250 ms; TE = 96–97 ms). This provides several flexible options for how the diffusion data can be analyzed. To promote consistent acquisition of the protocol, the Siemens protocol is highly automated with field-of-view positioning and orientation handled automatically using Siemens “AutoAlign” feature on all platforms (Prisma, Skyra, and Vida). On the other platforms, positioning, and orientation are set manually by the scan operator and linked (copied) to later scans in the session where appropriate. See supplementary materials for details of neuroimaging data flow and quality assurance procedures.
Genetics and Fluid Biomarkers.
Blood and saliva samples are collected at baseline and at 2-month follow-up. Blood is processed using a standardized protocol to obtain aliquots of platelet-poor plasma, serum, and whole blood. In addition, the buffy coat is aliquoted for DNA extraction. Samples are stored in a minus 80 freezer within 90 min of collection. In addition, 3 saliva samples are collected over a 2h period. Relevant metadata includes height, weight, sleep and awakening times, time fasting, recent illnesses or inflammatory conditions (eg, fever, respiratory systems, acne, sunburn, white blood cell differential), and physical activity.
We intend to analyze potential biomarkers of psychosis risk, especially those biomarkers that might reflect 1 or more treatment targets or be useful in risk stratification. Salivary cortisol65–69 will be assessed and DNA will be analyzed to obtain polygenic risk scores (PRS) for schizophrenia and other conditions.70,71 As study participants are intended to be ethnically diverse we propose to generate PRS with the Blended Genome Exome (BGE) assay, recently developed at the Broad Institute. The BGE is a state-of-the-art, cost-effective approach to capturing genetic diversity that blends high-pass sequencing of protein-coding and low-pass sequencing of non-protein-coding regions. Additional promising biomarker candidates under consideration include those reflecting redox system function,63 immune system dysregulation,64,65 and fatty acid levels.70 While each of these groups of markers has been associated with risk of transition to psychosis, there is emerging evidence for interaction between genotype, aberrant immune response, redox status, and membrane fatty acid composition, with subsequent impact on brain connectivity and plasticity and on dopamine and glutamate systems related to the underlying pathology of psychotic illness.72,73
All remaining fluid and DNA biospecimens will be stored indefinitely at the NIMH Repository and Genomics Resource (NRGR) for future research. See supplementary materials for further details.
Spoken Language and Facial Expression Samples.
Spoken language and facial expression samples are obtained from open-ended conversations (~20 min) and semi-structured PSYCHS interviews using Zoom’s online meeting platform. Spoken language samples are also acquired from audio diaries captured over smartphones. Face processing uses the open-source software packages Py-Feat74 v0.5 and MediaPipe v0.9.3.075 to determine the number and location of faces in a video. The landmark detection algorithms in these packages support the extraction of facial action units,76 allowing for the detection of common expressions and emotions. Zoom is parameterized to create separate audio files for each speaker in an online meeting. The open-source software packages Praat v6.3.1077 and openSMILE v3.0.78 are used to extract acoustic features such as voice stability, noise measurements, pitch variations, spectral characterizations, vowel space, and timbre features.
The 3 kinds of spoken language samples are converted into text using the TranscribeMe!79 transcription service. The transcripts are full verbatim: they include everything that is said, including filler words, false starts, grammatical errors, and nonlinguistic expressions, such as sighs and laughs. The service’s HIPAA-compliant workflow ensures proper handling of sensitive medical information. Human-editors identify personal information (personal health information and personally identifying information) so that it can be redacted from the transcript. Sentence-level timestamping enables time-course analyses of linguistic features over the course of an interview. The same transcription service is used for all 8 language communities included in the study.
Language recordings reflecting people’s naturally occurring thoughts, temporally aligned with facial expressions, acoustics, and prosody, will enable recovery of the concepts behind the words, the semantics of the face, and the hidden meaning of speech sounds.
Data Flow and Quality Assessment.
Figure 2 shows data flow from acquisition to the NDA curated releases. The data flow for this project is unique in that it requires coordination between 4 entities: 2 research networks each managing separate data capture systems, the DPACC overseeing data processing, QC pipelines and data visualization, and the NDA where curated data is deposited and disseminated. ProNET manages 4 data capture systems: REDCap for form data, XNAT for MRI data, MINDLamp for phone data, and Box for other file transfers (video recordings, actigraphy data, EEG scans). PRESCIENT manages 3 systems: RPMS for form data, MINDLamp, and MediaFlux for all other data types. Both networks use the PennCNB system to administer neurocognitive tests to participants. Each network also maintains a data aggregation and de-identification server, which pulls data from their data capture systems, organizes the data files on a local filesystem, and then performs de-identification procedures. For the AMP SCZ project, de-identification primarily consists of extracting key features from audio/video recordings, including redacted transcripts as raw data cannot leave the networks. These include automated QC as well as visual inspection of all data types. Key QC metrics and study monitoring information are displayed in a custom study dashboard hosted by the DPACC.
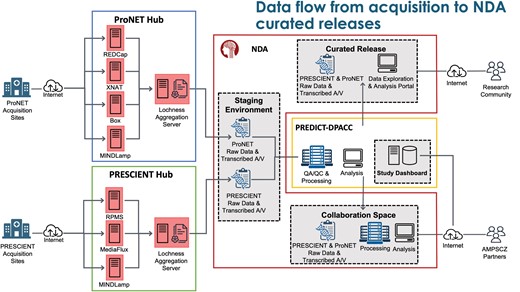
Overview of the data flow from acquisition to National Institute of Mental Health (NIMH) Data Archive (NDA) curated releases.
Aggregate and de-identified data are automatically transferred twice-daily to a staging area at the NDA, from which the DPACC then pulls the data for review, validation, quality checking, and further processing. Data are then further transformed to meet the standards established by the NDA data dictionaries and uploaded to the NDA collaboration space,13 where data can be explored and analyzed by AMP SCZ partners in Amazon workspaces virtual desktops. Data will also be shared with the wider community via traditional NDA data releases. Network-specific data flow and data quality checking is described briefly in supplementary materials.
Data quality is assessed in near real-time for all data types. Form data such as clinical interviews or cognitive tests are checked for data missingness and valid ranges. Automated and manual QC pipelines have been established for all complex data types including EEG, MRI, A/V, fluid, and digital biomarker data, monitored by DPACC and the relevant data type experts from the wider AMP SCZ community. Run sheets are checked for any protocol deviation or data quality issues observed by study staff during data acquisition. All QA/QC findings are reported back to the research networks management team for follow-up with the individual data collection sites. By assessing data quality in near real-time, missing or poor quality measures can often be corrected or re-collected. Additionally, deviations in protocols are caught quickly and can be amended. Finally, if the wrong protocol is run, or corrupted, because it is in near-time, the site can be contacted to rerun the participant for that measure.
Sample Size and Power.
The CHR sample size is primarily determined by the aim of developing a prediction model for transition to psychosis by 12 months and 24 months. We have used a recently described method80 for developing a clinical prediction model using a traditional likelihood-based approach (eg, logistic regression) to estimate an appropriate sample size. Specifically, sample sizes were derived for the scenarios of having low, medium, or high prediction performance and also for the number of parameters in the model being 10, 20, 30, 40, or 50. The expected transition rate used was 15% over 1 year. Assuming a transition rate of 15%, and a maximum of 30 parameters included in the predictive model, the minimum sample size for a model is estimated to be between 1100 and 1300 for a survival outcome (ie, an outcome that accounts for time to conversion and censoring; see supplementary material). Our projected sample size of 1977 CHR young people is therefore adequate for developing a prediction model with ~30 parameters that has high predictive performance, while still allowing a subset of the data to be “held back” for internal validation purposes. The adequacy of this sample size for machine-learning-based prediction models was confirmed in the simulation analyses of PRONIA data.81 The size of the HC sample was determined primarily to control for site effects, particularly for diffusion MRI data which is highly sensitive to scanner biases.82,83 Simulation analyses in the PRONIA18 dataset suggest that 15 HC per MRI scanner is recommended to control for site effects in diffusion data.
Data Analysis.
Data analysis for AMP SCZ will be performed by the Analysis and Visualization core of the DPACC in consultation with the Data Analytic Strategy Workgroup, which includes members from all consortium parties. The analyses will focus on 2 main goals: (1) prediction of clinical endpoints18,81,84–88 and (2) characterization and prediction of clinical trajectories.89,90 These goals will be achieved using state-of-the-art machine-learning methods that are robust, flexible, and clinically informed.91,92 Predictions will be based on data collected at baseline until and including the month 2 follow-up visit. This approach was chosen to optimize the usability for potential dynamic participant selection procedures in future clinical trials, while leveraging the expected stronger prediction value encapsulated in the longitudinal data.93–95 To ensure our ability to test the robustness of prediction models in an unbiased manner, 200 CHR individuals (roughly 10% of the entire data) will be held out from distribution for use as an unseen independent validation sample. This size of a held-out set has the power to validate predictors with area under the curve of 0.7 or higher, and with a prevalence of 10% or higher.96,97 Flexibility will be assured by applying data-fusion techniques81,98–101 to support the multimodal and longitudinal data. Our approaches will be clinically informed by implementing Explainable artificial intelligence (AI) methods102 that derive informative predictions that can be mapped back to individual features and biomarkers, as opposed to some deep learning and other “black-box” techniques that may not be appropriate for achieving interpretability. Clinical trajectory analyses, utilizing data from baseline, 12- and 24-month follow-up, will identify biomarkers and outcome measures that are associated with the endpoints so that they can inform future clinical trials, help construct risk calculators, and provide a more mechanistic understanding of the clinical endpoints using Explainable AI techniques.102 Trajectory analyses will include all information available from all time points, and will apply state-of-the-art clustering and latent variable analyses103–106 designed to find subtypes of CHR, and to identify measures that are informative regarding the endpoints, and that may be useful as biomarkers that will further facilitate population enrichment in future clinical trials. These analyses also lay the foundation for developing individual treatment paths, where prediction models in future clinical trials can be trained to identify individuals more likely to benefit from specific treatments, and trajectory models can facilitate dynamic models that could inform clinical decisions at each evaluation point.
Dissemination of Resources.
A website has been created (ampscz.org) that provides an overview of the aims and activities of the program for use by researchers, potential help-seeking youth and their caregivers, and clinicians looking for clinical trials that may be of help for their patients. The details for each study site in the networks are provided in the website, including contact information for all the principal and co-investigators, as well as the tools, protocols, standard operating procedures, and workflows. Tutorials will also be added to the website as they become available. The source code for the tools we have been building is available on GitHub (https://github.com/AMP-SCZ/). An annual meeting brings together AMP SCZ stakeholders across the networks and the DPACC, and includes presentations by senior scientists, junior researchers, public, and private partners, and experts with lived experience to disseminate information about progress toward project goals and other information relevant to stakeholders. Participation of AMP SCZ teams in an established international alliance of open-source software engineers and scientists will be organized to share best practices in the harmonization and analysis of multimodal data collected from multi-institutional international studies.
Discussion
With the goal of recruiting approximately 2000 CHR young people, AMP SCZ will be the largest and most diverse cohort study of individuals at CHR for psychosis to date, ensuring appropriate power and generalizability of findings. Identifying antecedents of psychosis and other outcomes has important implications for early detection, prevention, and treatment of psychotic disorders. Given its large projected sample size and geographical reach, AMP SCZ will develop and validate effective methods for dissecting the heterogeneity of CHR trajectories. This is critically needed in order to support the selection of primary outcome measures for future clinical trials, to stratify patients according to risk level for outcomes of interest, and to provide insight into pathoetiological mechanisms that can be targeted in future studies of novel treatments. This will accelerate targeted early intervention strategies, based on clinically relevant predictive models, which are critical to prevent psychosis onset and other adverse outcomes in CHR patients.
AMP SCZ will establish a landmark dataset for current and future analyses related to psychosis risk. The design of the project was guided by a number of innovative approaches, including: (1) a large and diverse international CHR sample recruited across 16 countries, ascertained using a newly developed instrument (PSYCHS) that harmonizes the 2 most widely used instruments in the field (ie, CAARMS and SIPS); (2) inclusion of the perspective of individuals with lived experience in the protocol design phase and in the ongoing conduct of the study; (3) a repeatable core set of clinical outcome measures suitable for future clinical trials and empirical trajectory analyses; (4) real-time behavioral data from smartphone sensors and actigraphy; (5) symptom reports from surveys that offer novel longitudinal and dynamic data on clinical, functional, and cognitive outcomes that will provide a rich new data set to deconstruct clinical heterogeneity; (6) a novel neuropsychological assessment battery designed to combine computerized and paper-and-pencil measures in a manner that provides sensitive, remote, longitudinal, international assessment of at-risk cognitive domains across multiple languages and cultures, while also minimizing participant burden; (7) a panel of multimodal measures collected at 2 early timepoints suitable for use in patient stratification based on dynamic prediction of subsequent outcomes and trajectories; (8) modern “HCP-Style” MRI acquisition protocol harmonized across scanner platform for assessment of both structural and connectivity changes, with certification of sites in implementation of the protocol; (9) leasing of identical EEG acquisition systems and custom-engineered stimulus delivery systems dedicated exclusively to the AMP SCZ project, as well as implementation of an automated EEG processing pipeline and web-based dashboard displaying single subject data and quality control metrics that are updated daily and reviewed in weekly video calls with the EEG technicians and investigators from AMP SCZ sites; (10) partnership with the Broad Institute using state-of-the-art genotyping that is designed to better account for genetic diversity, addressing a critical issue in polygenic risk determination; (11) language samples, in sync with facial expressions and speech characteristics, are collected and analyzed using Artificial Intelligence, enabling the extraction of underlying concepts, emotional cues, and semantic information from both verbal and non-verbal communication; (12) close to real-time quality control of all collected data and reporting of issues back to sites so that errors can be quickly corrected and incorporated into future data collection, and in some instances an error in a measure can be rerun so that correct data replace the incorrect data; (13) incorporation of a novel poly-environmental risk score into multimodal analyses; and (14) a cutting-edge data capture and study management system that is flexible, scalable and customizable, and can support multiple data types, including traditional types such as questionnaires and imaging data, but also emerging data types from phones, personal tracking devices, and audio-visual recordings.
The global reach of AMP SCZ and its harmonized innovative methods promise to catalyze efforts to address critical unmet clinical and public health needs in CHR patients. This initiative will provide tools that will enable testing of more precise and mechanism-linked treatments and advance the goal of averting the onset of psychotic disorders in high-risk individuals.
Acknowledgments
The Accelerating Medicines Partnership Schizophrenia (AMP SCZ) is a public-private partnership managed by the Foundation for the National Institutes of Health. The AMP SCZ research program is supported by contributions from the AMP SCZ public and private partners, including NIMH (U24MH124629, U01MH124631, and U01MH124639) and Wellcome (220664/Z/20/Z and 220664/A/20/Z). The support of the NHMRC CRE PRE-EMPT (1198304) is acknowledged. The views expressed in this article are personal views of the authors and may not be understood or quoted as being made on behalf of or reflecting the position of the Department of Health and Human Services, including the National Institutes of Health and the US Food and Drug Administration, the United States Government, or the European Medicines Agency or one of its committees or working parties. AMP SCZ Steering Committee members include Loni Ajagbe, R. Reina Benabou, Lynsey Bilsland, Linda S. Brady, Terri Brister, Florence Butlen-Ducuing, Kenneth Duckworth, Gregory K. Farber, Tiffany Farchione, Bernard A. Fischer, Sophia Frangou, Suzanne T. Garcia, Nitin Gogtay, Joshua A. Gordon, Robert K. Heinssen, Brian R. Johnson, Pushkar S. Joshi, Carlos A. Larrauri, Sarah H. Lisanby, Gahan Pandina, Sharin E. Roth, Michael Sand, Adam Savitz, Vikas Sharma, Brandon Staglin, Alessio Travaglia, Eva Velthorst, and Glen Wunderlich. Other AMP SCZ members not listed elsewhere include Margaret C. Grabb, Daniel Janes, Sarah E. Morris, Jonathan Pevsner, S. Andrea Wijtenburg, Laura M. Rowland, Ming Zhan, Eline Appelmans, Noam I. Keren, and Steven Hyman. S.W.W. has received sponsor-initiated research funding support from Boehringer-Ingelheim, Amarex, and SyneuRx. He has been a paid consultant to Boehringer-Ingelheim, New England Research Institute, and Takeda. He has been granted US patent no. 8492418 B2 for a method of treating prodromal schizophrenia with glycine agonists. B.C.W. has been a paid consultant with Boehringer-Ingelheim and the Pier Institute. A.A. holds equity and is a member of the Technology Advisory Board for Neumora Therapeutics, Inc.; is a cofounder, serves as a member of the Board of Directors, as a scientific adviser, and holds equity in Manifest Technologies, Inc.; and is a coinventor on the following patent: Anticevic A, Murray JD, Ji JL: Systems and Methods for Neuro-Behavioral Relationships in Dimensional Geometric Embedding, PCT International Application No. PCT/US2119/022110, filed Mar 13, 2019. J.M.K has received honoraria for lectures or consulting from Alkermes. R.S.K. has consulted for Alkermes, Otsuka, and Sunovion. D.O.P reports consulting for Alkermes. S.R.C. reports Speaker’s Fees/ Honoraria: Janssen-Cillag Australia, Lundbeck-Otsuka Australia, Servier Australia Advisory Board: Lundbeck-Otsuka Australia (Maintena, Brexpiprazole) Investigator-initiated grants: Janssen-Cillag Australia, Lundbeck-Otsuka AustraliaTravel Support: Janssen-Cillag Australia. D.H.M. has served as a consultant for Neurocrine Biosciences. C.A. has been a consultant to or has received honoraria or grants from Acadia, Angelini, Biogen, Boehringer, Gedeon Richter, Janssen Cilag, Lundbeck, Medscape, Menarini, Minerva, Otsuka, Pfizer, Roche, Sage, Servier, Shire, Schering Plough, Sumitomo Dainippon Pharma, Sunovion and Takeda. E.Y.H.C. reports investigator-initiated grants: Janssen-Cillag. C.M.D.-C. reports grant support from Instituto de Salud Carlos III, Spanish Ministry of Science and Innovation (PI17/00481, PI20/00721, JR19/00024), and honoraria from Exeltis and Angelini. P.J.M. has received sponsor-initiated funding from Alkermes, Boehringer-Ingelheim, Roche, NeuroRX, and Otsuka and honorarium from Alkermes, Lundbeck, Otsuka, and BioXcel. B.H.E is part of the Advisory Board of Eli Lilly Denmark A/S, Janssen Cilag, Lundbeck Pharma A/S, and Takeda Pharmaceutical Company Ltd; and has received lecture fees from Bristol-Myers Squibb, Boehringer-Ingelheim, Otsuka Pharma Scandinavia AB, Eli Lilly Company, and Lundbeck Pharma A/S. Other authors report no conflict of interest. P.F.P has received research funds or personal fees from Lundbeck, Angelini, Menarini, Sunovion, Boehringer-Ingelheim, Proxymm Science, outside the current study. G.P.S. has consulted for Boehringer-Ingelheim, Acadia, Minerva, and Sunovion. J.T. is a scientific Advisor for Precision Mental Wellness. R.U. reports grant funding from Medical Research Council (MR/S037675/1), National Institute for Health Research: Health Technology Assessment (NIHR 127700), and speaker fees from Sunovion, Springer Heathcare, Otuska, and Vitaris outside the submitted work. R.U. holds unpaid officership with the British Association for Pharmacology- Honorary General Secretary 2021–2024 and is Deputy Editor, The British Journal of Psychiatry. J.J. is an employee of Manifest Technologies and has previously worked for Neumora (formerly BlackThorn Therapeutics) and a coinventor on the following patents: Anticevic A, Murray JD, Ji JL: Systems and Methods for Neuro-Behavioral Relationships in Dimensional Geometric Embedding (N-BRIDGE), PCT International Application No. PCT/US2119/022110, filed March 13, 2019. P.A.L. has received honoraria for talks presented at educational meetings organized by Boehringer-Ingelheim. V.H.S. has served on Advisory Boards to Takeda and Karuna Therapeutics. A.T. has been a consultant to or has received honoraria or grants from Janssen Cilag, Otsuka, Pfizer, and Servier. P.P. has received Speaker’s Fees from Angelini, Janssen, Lundbeck-Otsuka, and Roche.
References
Author notes
Cassandra M. J. Wannan and Barnaby Nelson Joint first author.
Scott W. Woods and Martha E. Shenton Joint senior author.