-
PDF
- Split View
-
Views
-
Cite
Cite
Stefano Damiani, Grazia Rutigliano, Teresa Fazia, Sergio Merlino, Carlo Berzuini, Luisa Bernardinelli, Pierluigi Politi, Paolo Fusar-Poli, Developing and Validating an Individualized Clinical Prediction Model to Forecast Psychotic Recurrence in Acute and Transient Psychotic Disorders: Electronic Health Record Cohort Study, Schizophrenia Bulletin, Volume 47, Issue 6, November 2021, Pages 1695–1705, https://doi.org/10.1093/schbul/sbab070
- Share Icon Share
Abstract
Acute and transient psychotic disorders (ATPDs) include short-lived psychotic episodes with a high probability of developing psychotic recurrences. Clinical care for ATPD is currently limited by the inability to predict outcomes. Real-world electronic health record (EHR)-based retrospective cohort study STROBE/RECORD compliant included all individuals accessing the South London and Maudsley NHS Trust between 2006 and 2017 and receiving a first diagnosis of ATPD (F23, ICD-10). After imputing missing data, stepwise and LASSO Cox regression methods employing a priori predictors (n = 23) were compared to develop and internally validate an individualized risk prediction model to forecast the risk of psychotic recurrences following TRIPOD guidelines. The primary outcome was prognostic accuracy (area under the curve [AUC]). 3018 ATPD individuals were included (average age = 33.75 years, 52.7% females). Over follow-up (average 1042 ± 1011 days, up to 8 years) there were 1160 psychotic recurrences (events). Stepwise (n = 12 predictors) and LASSO (n = 17 predictors) regression methods yielded comparable prognostic accuracy, with an events per variable ratio >100 for both models. Both models showed an internally validated adequate prognostic accuracy from 4 years follow-up (AUC 0.70 for both models) and good calibration. A refined model was adapted in view of the new ICD-11 criteria on 307 subjects with polymorphic ATPD, showing fair prognostic accuracy at 4 years (AUC: stepwise 0.68; LASSO 0.70). This study presents the first clinically based prediction model internally validated to adequately predict long-term psychotic recurrence in individuals with ATPD. The model can be automatable in EHRs, supporting further external validations and refinements to improve its prognostic accuracy.
Introduction
Acute and transient psychotic disorders (ATPDs) are characterized by the abrupt onset and rapid remission of symptoms (polymorphic, schizophrenic, or predominantly delusional syndromes) within 1–3 months.1 The ICD-10 included 6 ATPD subtypes: acute polymorphic psychotic disorder without (F23.0) or with (F23.1) schizophrenic symptoms; acute schizophrenia-like psychotic disorder (F23.2); acute predominantly delusional psychotic disorder (F23.3); “other” (F23.8) and “unspecified” (F23.9) ATPD. The forthcoming ICD-11 revision is expected to narrow the ATPD to the category of acute polymorphic disorder without schizophrenic symptoms (ie, F23.0).2 ATPD lies on the unclear border between Clinical High Risk for Psychosis (CHR-P)3,4 conditions (68% of CHR-P individuals meeting Brief and Limited Intermittent Psychotic symptoms would also meet ICD-10 ATPD criteria)5,6 and more persistent, chronic psychotic disorders.7,8 For example, the risk of psychotic recurrence after the initial index episode is similar to the DSM-5 category of Brief Psychotic Disorders (BPD) (0.494, 95% CI 0.347–0.642 at 3 years),6 but substantially lower than first episode remitted schizophrenia (0.84, 95% CI 0.70–0.94 at 3 years).9 Prospective meta-analyses showed that 56% (95% 0.52–0.60) of the initial ATPD cases retain the initial diagnosis after 4.5 years.10 These findings suggest that a prognostic, as opposed to static ICD/DSM diagnostic, framework is essential to capture the intrinsic dynamic and fluctuating nature of these disorders.11
In light of these challenges, there are no evidence-based guidelines for the clinical management of ATPD. Although there are no antipsychotic molecules specifically licensed for ATPD, these are frequently employed in ATPD patients,12 while only a very few (8%) of these individuals receiving psychotherapy.13 Even if about half of ATPD patients would not develop any psychotic recurrence even after a follow-up of 3 years,9 it is not possible to predict the psychotic recurrences at the individual subject level. Therefore, many people with ATPD are exposed to unnecessary antipsychotic treatment and avoidable side effects, while others may not be offered relapse prevention treatments.
These arguments call for individualized risk estimation models to forecast psychotic recurrence in ATPD. Conducting this type of research is particularly challenging, given the epidemiological rarity of ATPD and challenging long-term follow-up. This study fills these gaps by leveraging state-of-the-art clinical prediction modeling methods, in combination with electronic health records (EHRs). The primary aim was to develop and internally validate an individualized risk prediction model that can leverage clinical information routinely acquired in EHRs and predict the risk of psychotic recurrences in ATPD.
Materials and Methods
Study Design
Retrospective, real-world, EHRs clinical case-cohort study relating to secondary mental healthcare (South London and Maudsley, SLaM NHS Trust) in the United Kingdom.
Settings
The SLaM NHS Trust is one of Europe’s largest secondary mental healthcare providers,14,15 covering 4 highly socially, economically, and ethnically diverse South London boroughs, alongside tertiary referrals from the rest of London and the United Kingdom. Such high variance is particularly suited to develop clinical prediction models that can remain valid across different populations.15 SLaM is paper-free, and all clinicians record their activity electronically on the Patient Journey System (PJS), as part of their clinical routine16 (eMethods 1). Anonymized information from PJS is subsequently used to create the Clinical Record Interactive Search (CRIS). The CRIS system developed at SLaM facilitates extraction and research consultation of deidentified EHRs held by the Trust, providing real-world clinical data from over 400 000 individuals.14,17,18 The CRIS data received ethical approval as an anonymized dataset for secondary analyses from Oxfordshire REC C.14
Study Population
All individuals accessing the SLaM NHS Trust between April 1, 2006 and June 15, 2017 and receiving a first index diagnosis of ATPD (F23, ICD-10) were considered eligible for the study. Individuals were then followed up until they developed a psychotic recurrence (defined below) or dropped from the cohort study (as documented by the last entry on CRIS). To reflect the temporal criteria of maximum 3 months to formulate an index ATPD diagnosis, we excluded individuals developing psychotic recurrences within the first 3 months, as well as those with less than 3 months follow-up (see below).
Data Source
All data employed in the current study were extracted from the local EHR through CRIS. Follow-up started at 3 months since the index diagnosis of ATPD, which indexed the first contact with SLaM (ie, the entry point into the local healthcare system). The 3-month window for initiating the follow-up was chosen to match the maximal duration of symptoms for an index episode of ATPD episode.19 This approach has already been validated in previous studies conducted on this population.18,20,21
Statistical Analyses
This clinical register-based observational study was conducted according to the STROBE guidelines22 and the REporting of studies Conducted using Observational Routinely-collected health Data (RECORD) Statement (see eTable 1).23 Model development and validation followed the guidelines of,24,25 and the Transparent Reporting of a multivariable prediction model for Individual Prognosis Or Diagnosis (TRIPOD, eTable 2).26
Missing data were quantified and then imputed with a single imputation method using SPSS 26 (Mersenne Twister pseudorandom active generator, starting point fixed value of 2 000 000).27,28T tests and exact chi-square tests were employed to test baseline differences in clinical and sociodemographic characteristics between the complete cases individuals and those with data missingness (a P value of <.01 was used to correct for multiple comparisons).
Descriptive analyses of baseline clinical and sociodemographic characteristics of the imputed sample with means and frequencies for continuous and categorical variables, respectively, are reported in table 1.
Sample Characteristics and Predictors of Psychotic Recurrence Considered for Model Building
. | All Subjects (n = 3018) . | |
---|---|---|
Categorical Predictors . | n . | % . |
Female gender | 1590 | 52.7 |
Black ethnicity | 1359 | 45.0 |
Presence of psychiatric comorbidity at baseline | 144 | 4.8 |
Family history positive for any psychotic disorder | 689 | 22.8 |
Presence of acute stress events | 33 | 1.1 |
Substance use (any) | 999 | 33.1 |
Alcohol | 644 | 21.3 |
Opioids | 133 | 4.4 |
Cannabinoids | 961 | 31.8 |
Cocaine | 164 | 5.4 |
Stimulants | 372 | 12.3 |
Hallucinogens | 94 | 3.1 |
Volatile solvents | 272 | 9.0 |
ATPD length >1 month | 903 | 29.9 |
Recurrent ATPD within 3 months | 72 | 2.4 |
Abrupt onset of ATPD episode | 347 | 11.5 |
Antidepressant treatment in the first month | 361 | 12.0 |
Antipsychotic treatment in the first month | 1676 | 55.5 |
Antidepressant treatment in the first 3 months | 256 | 8.5 |
Antipsychotic treatment in the first 3 months | 1034 | 34.3 |
Continuous Predictors | Mean | SD |
Age (years) | 33.75 | 14.01 |
Time since first observation of attenuated/psychotic symptoms to the ATPD index diagnosis (days) | 404.23 | 831.99 |
Number of substances used | 0.45 | 0.77 |
. | All Subjects (n = 3018) . | |
---|---|---|
Categorical Predictors . | n . | % . |
Female gender | 1590 | 52.7 |
Black ethnicity | 1359 | 45.0 |
Presence of psychiatric comorbidity at baseline | 144 | 4.8 |
Family history positive for any psychotic disorder | 689 | 22.8 |
Presence of acute stress events | 33 | 1.1 |
Substance use (any) | 999 | 33.1 |
Alcohol | 644 | 21.3 |
Opioids | 133 | 4.4 |
Cannabinoids | 961 | 31.8 |
Cocaine | 164 | 5.4 |
Stimulants | 372 | 12.3 |
Hallucinogens | 94 | 3.1 |
Volatile solvents | 272 | 9.0 |
ATPD length >1 month | 903 | 29.9 |
Recurrent ATPD within 3 months | 72 | 2.4 |
Abrupt onset of ATPD episode | 347 | 11.5 |
Antidepressant treatment in the first month | 361 | 12.0 |
Antipsychotic treatment in the first month | 1676 | 55.5 |
Antidepressant treatment in the first 3 months | 256 | 8.5 |
Antipsychotic treatment in the first 3 months | 1034 | 34.3 |
Continuous Predictors | Mean | SD |
Age (years) | 33.75 | 14.01 |
Time since first observation of attenuated/psychotic symptoms to the ATPD index diagnosis (days) | 404.23 | 831.99 |
Number of substances used | 0.45 | 0.77 |
Note: ATPD, acute and transient psychotic disorder.
Sample Characteristics and Predictors of Psychotic Recurrence Considered for Model Building
. | All Subjects (n = 3018) . | |
---|---|---|
Categorical Predictors . | n . | % . |
Female gender | 1590 | 52.7 |
Black ethnicity | 1359 | 45.0 |
Presence of psychiatric comorbidity at baseline | 144 | 4.8 |
Family history positive for any psychotic disorder | 689 | 22.8 |
Presence of acute stress events | 33 | 1.1 |
Substance use (any) | 999 | 33.1 |
Alcohol | 644 | 21.3 |
Opioids | 133 | 4.4 |
Cannabinoids | 961 | 31.8 |
Cocaine | 164 | 5.4 |
Stimulants | 372 | 12.3 |
Hallucinogens | 94 | 3.1 |
Volatile solvents | 272 | 9.0 |
ATPD length >1 month | 903 | 29.9 |
Recurrent ATPD within 3 months | 72 | 2.4 |
Abrupt onset of ATPD episode | 347 | 11.5 |
Antidepressant treatment in the first month | 361 | 12.0 |
Antipsychotic treatment in the first month | 1676 | 55.5 |
Antidepressant treatment in the first 3 months | 256 | 8.5 |
Antipsychotic treatment in the first 3 months | 1034 | 34.3 |
Continuous Predictors | Mean | SD |
Age (years) | 33.75 | 14.01 |
Time since first observation of attenuated/psychotic symptoms to the ATPD index diagnosis (days) | 404.23 | 831.99 |
Number of substances used | 0.45 | 0.77 |
. | All Subjects (n = 3018) . | |
---|---|---|
Categorical Predictors . | n . | % . |
Female gender | 1590 | 52.7 |
Black ethnicity | 1359 | 45.0 |
Presence of psychiatric comorbidity at baseline | 144 | 4.8 |
Family history positive for any psychotic disorder | 689 | 22.8 |
Presence of acute stress events | 33 | 1.1 |
Substance use (any) | 999 | 33.1 |
Alcohol | 644 | 21.3 |
Opioids | 133 | 4.4 |
Cannabinoids | 961 | 31.8 |
Cocaine | 164 | 5.4 |
Stimulants | 372 | 12.3 |
Hallucinogens | 94 | 3.1 |
Volatile solvents | 272 | 9.0 |
ATPD length >1 month | 903 | 29.9 |
Recurrent ATPD within 3 months | 72 | 2.4 |
Abrupt onset of ATPD episode | 347 | 11.5 |
Antidepressant treatment in the first month | 361 | 12.0 |
Antipsychotic treatment in the first month | 1676 | 55.5 |
Antidepressant treatment in the first 3 months | 256 | 8.5 |
Antipsychotic treatment in the first 3 months | 1034 | 34.3 |
Continuous Predictors | Mean | SD |
Age (years) | 33.75 | 14.01 |
Time since first observation of attenuated/psychotic symptoms to the ATPD index diagnosis (days) | 404.23 | 831.99 |
Number of substances used | 0.45 | 0.77 |
Note: ATPD, acute and transient psychotic disorder.
The primary objective of this study was to develop and internally validate a clinically based, individualized, EHR-based prediction model to forecast the risk of developing persistent psychotic recurrences in ATPD (ie, “diagnostic shifts”). The latter were defined as the onset of the first ICD-10 diagnosis of nonorganic psychotic disorders since the initial ATPD index diagnosis, while subsequent ATPD episodes were not considered: schizophrenia-spectrum disorders (schizophrenia [F20.x, except F20.4/F20.5], schizoaffective disorder [F25.x], delusional disorders [F22.x, F24]); affective-spectrum psychoses (mania with psychotic symptoms [F30.2], bipolar affective disorder with psychotic symptoms [F31.2, F31.5], and depression with psychotic symptoms [F32.3/F33.3]); psychotic disorders due to psychoactive substance use ([F10–F19].5); unspecified nonorganic psychoses (F28/F29); and severe mental and behavioral disorders associated with the puerperium (puerperal psychosis NOS, F53.1). The primary outcome measure was the prognostic accuracy evaluated with areas under the curve (AUCs)29 at several prediction horizons. An AUC value of 0.9–1.0 is considered outstanding, 0.8–0.9 excellent and 0.7–0.8 acceptable.30 The secondary outcome was calibration (see below).
Model Development
Eligible predictors of psychotic recurrence in ATPD were selected on the basis of clinical knowledge5,9,31–33 and their availability in EHRs. The initial pool of potential predictors encompassed 23 factors. Demographical factors: age, gender, ethnicity, psychiatric comorbidities at baseline, family history for any psychiatric disorder (up to third-degree relatives), and presence of acute stress due to adverse life events.
Substance-related factors: reported use of substances (any, alcohol, opioids, cannabinoids, cocaine, stimulants, hallucinogens, volatile solvents) and number of substances used. Substance use does not represent per se an exclusion criterion for diagnosing ATPD, but it is subject to “clinical judgement.” Accordingly, substance use was operationalized by identifying any substance misuse prior to the index ATPD diagnosis, as reported by clinicians in the patients’ EHR clinical records. These predictors indexed the presence of illicit substances on the clinical records uploaded in the EHR and not necessarily substance-induced disorders.
ATPD-related factors: duration of ATPD index episode >1 month, abrupt onset of symptoms, single/recurrent presentation of ATPD in the first 3 months of follow-up, and time since first observation of attenuated/psychotic symptoms to the ATPD index diagnosis (see eMethods 2). The latter was measured as the temporal intercourse between the first occurrence of attenuated/psychotic symptoms as documented by the clinicians in their clinical notes (EHR) and the first index diagnosis of ATPD, as formulated by clinicians. Notably, symptoms recorded before the ATPD index diagnosis may not necessarily have reached a sufficient intensity or duration to meet the diagnostic threshold of a full-blown ATPD episode or may have been highly infrequent (eg, lasting only a few hours on a single occasion), thus representing attenuated and not frank psychotic symptoms. In both cases, there was no assumption that symptoms preceding ATPD were ongoing and persistent.
Medication-related factors: antidepressant medications at 1 and 3 months after the index episode and antipsychotic medications at 1 and 3 months after the index episode. Given that the outcomes are detected in the long term (up to 8 years since onset), we assumed that medication exposure taken within 3 months from the index diagnosis of ATPD was unlikely to influence the outcomes.
The predictors and time to events were entered in a Cox regression model after checking proportionality of hazards, in order to predict the psychotic recurrence over time. Selection of predictors was performed through 2 different approaches: (1) stepwise Cox regression procedure using a combination of a forward and backward procedure (implemented by the R package MASS)34–36 and (2) LASSO regularized Cox regression (implemented by the R packages glmnet).37,38 For the LASSO procedure, selection of predictors was automatic.
A valid prognostic model should retain good calibration (ie, the agreement between the observed and predicted risk of psychotic recurrence) and discrimination (ie, separating ATPD with and without psychotic recurrences).39 We adopted 2 variable selection approaches because the LASSO technique tends to maximize the discrimination at the price of calibration, while stepwise regression tends to maximize both. Two standard Cox models using as covariate, respectively, the variables selected by stepwise and LASSO regression were therefore fitted using the R package survival.40 Furthermore, to monitor the event per variable (EPV) ratio, we reported the number of psychotic recurrences divided by the degrees of freedom of the predictors for each model.
Internal Validation
Optimism in prognostic performance of the 2 models was assessed via 5-fold cross-validated receiver operating curve and dynamic AUC statistics (AUC measured for each year of follow-up). Chambless and Diao’s estimator of cumulative/dynamic AUC for time-to-event data was computed implementing R package survAUC.41
The calibration analysis of the 2 models was graphically inspected using the R package CalibrationCurves.39 All the analyses were performed using R statistical software.42
Sensitivity Analyses
To test our model within the forthcoming ICD-11 operationalization of ATPD, our analyses were repeated including only the individuals meeting the ATPD polymorphic subtype (only F23.0, F23.1).
We further conducted sensitivity analyses to control the impact of predictors that could be specifically affected by ecological biases, excluding the “time since first observation of attenuated/psychotic symptoms to ATPD index diagnosis” and the “use of substances (any, alcohol, opioids, cannabinoids, cocaine, stimulants, hallucinogens, volatile solvents, number of substances used)” predictors and reestimating the prognostic accuracy of the model.
Finally, a decision curve analysis has been performed to test potential clinical utility of both LASSO and stepwise prediction models using the R package dca.43
Results
Sociodemographic and Clinical Characteristics
Data from a total of 3286 subjects who had experienced an ATPD episode from April 1, 2006 to June 15, 2017 were extracted from CRIS (figure 1). Across the final sample (n = 3018), the mean age was 33.75 ± 14.01 years, 52.7% were females, and 45.0% were of black ethnicity. Table 1 details the sociodemographic and clinical information of the sample, as well as the potential predictors considered. Information for all predictors (complete cases) was available for 1871 subjects, while 1147 individuals had at least 1 missing variable. The overall missing data cells were low (7.86%) and were mainly related to the abrupt onset, time since first observation of attenuated/psychotic symptoms to the ATPD index diagnosis, and substance use data (eTable 4). Comparisons between missing and complete cases data are presented in eTable 5.
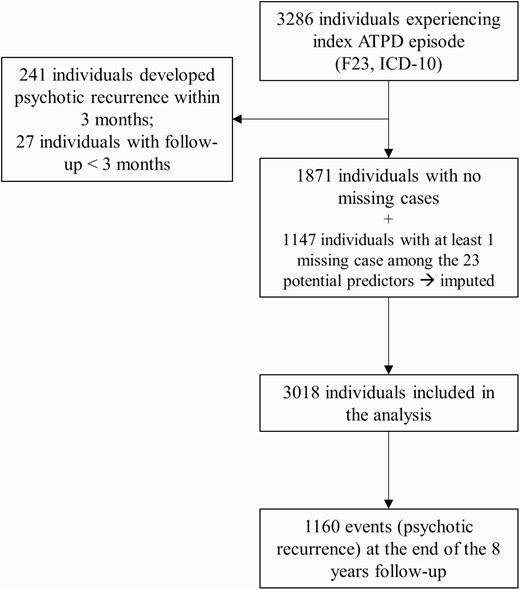
The mean follow-up was 1042 ± 1011 days. The mean time to psychotic recurrence was 661.82 ± 609.98 days, with the first event (psychotic recurrence) observed at 91 days and the last at 2907 days. There were 1160 events; their ICD-10 diagnoses are appended in eTable 3.
Individualized Prognostic Models to Forecast Psychotic Recurrence in ATPD
Across the eligible pool of predictors selected on the basis of a priori knowledge (n = 23), the stepwise and LASSO regression models selected 12 and 17 predictors, respectively, with a corresponding EPV of 251.5 and 177.5. All the predictors selected by the stepwise regression were also selected by the LASSO regression. Table 2 reports selected predictors and characteristics of the internally validated Cox regression model fitted on the predictors selected by both models. Across both models, predictors were ranked according to their decreased prognostic impact, indexed by the absolute magnitude of their LASSO regression coefficients (which selected more predictors, table 2): the top 4 predictors associated with an increased risk of psychotic recurrence were antipsychotics at 3 months, black ethnicity, recurrent ATPD episode within 3 months, and antipsychotics at 1 month. The top 4 predictors associated with a reduced likelihood of psychotic recurrence were substance use (opioids, stimulants, and volatile solvents) and abrupt onset. The AIC obtained by fitting the internally validated Cox models was 17308 for stepwise and 17315 for LASSO. Despite the lower number of variables selected by the stepwise analysis (12 vs. 17), its internally validated AIC was almost identical to the LASSO one, thus indicating similar estimates of the out-of-sample prediction error and fit. Internally validated AUC values increased with length of the prediction horizon for both stepwise and LASSO, peaking to 0.71 at 8 years in each model. Both models showed adequate (ie, greater than 0.7) AUCs at 4 years follow-up. Graphical inspection of the calibration plots showed that both models have good calibration (see figure 2).
Predictors of Psychotic Recurrence in ATPD Selected by Stepwise and LASSO Cox Regression Models
Cox Regression . | Stepwise . | LASSO . | ||||
---|---|---|---|---|---|---|
Starting Variables . | Selected Variables . | Regress. Coeff. . | SE . | Selected Variables . | Regress. Coeff. . | SE . |
Antipsychotic treatment in the first 3 months | X | 0.739 | 0.066 | X | 0.723 | 0.070 |
Opioids | X | −0.593 | 0.193 | X | −0.524 | 0.197 |
Volatile solvents | X | −0.393 | 0.191 | X | −0.387 | 0.192 |
Ethnicity black | X | 0.378 | 0.060 | X | 0.378 | 0.061 |
Recurrent ATPD within 3 months | X | 0.350 | 0.155 | X | 0.346 | 0.156 |
Stimulants | X | −0.296 | 0.149 | X | −0.316 | 0.154 |
Antipsychotic treatment in the first month | X | 0.302 | 0.072 | X | 0.299 | 0.074 |
Hallucinogens | . | X | −0.278 | 0.201 | ||
Abrupt onset of ATPD episode | X | −0.163 | 0.096 | X | −0.159 | 0.096 |
Family history positive for any psychotic disorder | X | 0.145 | 0.067 | X | 0.138 | 0.067 |
Substance use (any) | X | 0.190 | 0.063 | X | 0.133 | 0.105 |
ATPD length >1 month | X | −0.109 | 0.068 | X | −0.110 | 0.068 |
N of substances used | . | X | 0.056 | 0.069 | ||
Antidepressant treatment in the first 3 months | . | X | 0.055 | 0.111 | ||
Antidepressant treatment in the first month | . | X | 0.041 | 0.101 | ||
Age (years) | . | X | −0.002 | 0.002 | ||
Time since first observation of attenuated/psychotic symptoms to the ATPD index diagnosis (days) | X | <0.001 | <0.001 | X | <0.001 | <0.001 |
Gender | . | . | ||||
Presence of psychiatric comorbidity at baseline | . | . | ||||
Acute stress events | . | . | ||||
Alcohol | . | . | ||||
Cannabinoids | . | . | ||||
Cocaine | . | . | ||||
n = 23 | n = 12 | n = 17 |
Cox Regression . | Stepwise . | LASSO . | ||||
---|---|---|---|---|---|---|
Starting Variables . | Selected Variables . | Regress. Coeff. . | SE . | Selected Variables . | Regress. Coeff. . | SE . |
Antipsychotic treatment in the first 3 months | X | 0.739 | 0.066 | X | 0.723 | 0.070 |
Opioids | X | −0.593 | 0.193 | X | −0.524 | 0.197 |
Volatile solvents | X | −0.393 | 0.191 | X | −0.387 | 0.192 |
Ethnicity black | X | 0.378 | 0.060 | X | 0.378 | 0.061 |
Recurrent ATPD within 3 months | X | 0.350 | 0.155 | X | 0.346 | 0.156 |
Stimulants | X | −0.296 | 0.149 | X | −0.316 | 0.154 |
Antipsychotic treatment in the first month | X | 0.302 | 0.072 | X | 0.299 | 0.074 |
Hallucinogens | . | X | −0.278 | 0.201 | ||
Abrupt onset of ATPD episode | X | −0.163 | 0.096 | X | −0.159 | 0.096 |
Family history positive for any psychotic disorder | X | 0.145 | 0.067 | X | 0.138 | 0.067 |
Substance use (any) | X | 0.190 | 0.063 | X | 0.133 | 0.105 |
ATPD length >1 month | X | −0.109 | 0.068 | X | −0.110 | 0.068 |
N of substances used | . | X | 0.056 | 0.069 | ||
Antidepressant treatment in the first 3 months | . | X | 0.055 | 0.111 | ||
Antidepressant treatment in the first month | . | X | 0.041 | 0.101 | ||
Age (years) | . | X | −0.002 | 0.002 | ||
Time since first observation of attenuated/psychotic symptoms to the ATPD index diagnosis (days) | X | <0.001 | <0.001 | X | <0.001 | <0.001 |
Gender | . | . | ||||
Presence of psychiatric comorbidity at baseline | . | . | ||||
Acute stress events | . | . | ||||
Alcohol | . | . | ||||
Cannabinoids | . | . | ||||
Cocaine | . | . | ||||
n = 23 | n = 12 | n = 17 |
Note: ATPD, acute and transient psychotic disorder.
Predictors of Psychotic Recurrence in ATPD Selected by Stepwise and LASSO Cox Regression Models
Cox Regression . | Stepwise . | LASSO . | ||||
---|---|---|---|---|---|---|
Starting Variables . | Selected Variables . | Regress. Coeff. . | SE . | Selected Variables . | Regress. Coeff. . | SE . |
Antipsychotic treatment in the first 3 months | X | 0.739 | 0.066 | X | 0.723 | 0.070 |
Opioids | X | −0.593 | 0.193 | X | −0.524 | 0.197 |
Volatile solvents | X | −0.393 | 0.191 | X | −0.387 | 0.192 |
Ethnicity black | X | 0.378 | 0.060 | X | 0.378 | 0.061 |
Recurrent ATPD within 3 months | X | 0.350 | 0.155 | X | 0.346 | 0.156 |
Stimulants | X | −0.296 | 0.149 | X | −0.316 | 0.154 |
Antipsychotic treatment in the first month | X | 0.302 | 0.072 | X | 0.299 | 0.074 |
Hallucinogens | . | X | −0.278 | 0.201 | ||
Abrupt onset of ATPD episode | X | −0.163 | 0.096 | X | −0.159 | 0.096 |
Family history positive for any psychotic disorder | X | 0.145 | 0.067 | X | 0.138 | 0.067 |
Substance use (any) | X | 0.190 | 0.063 | X | 0.133 | 0.105 |
ATPD length >1 month | X | −0.109 | 0.068 | X | −0.110 | 0.068 |
N of substances used | . | X | 0.056 | 0.069 | ||
Antidepressant treatment in the first 3 months | . | X | 0.055 | 0.111 | ||
Antidepressant treatment in the first month | . | X | 0.041 | 0.101 | ||
Age (years) | . | X | −0.002 | 0.002 | ||
Time since first observation of attenuated/psychotic symptoms to the ATPD index diagnosis (days) | X | <0.001 | <0.001 | X | <0.001 | <0.001 |
Gender | . | . | ||||
Presence of psychiatric comorbidity at baseline | . | . | ||||
Acute stress events | . | . | ||||
Alcohol | . | . | ||||
Cannabinoids | . | . | ||||
Cocaine | . | . | ||||
n = 23 | n = 12 | n = 17 |
Cox Regression . | Stepwise . | LASSO . | ||||
---|---|---|---|---|---|---|
Starting Variables . | Selected Variables . | Regress. Coeff. . | SE . | Selected Variables . | Regress. Coeff. . | SE . |
Antipsychotic treatment in the first 3 months | X | 0.739 | 0.066 | X | 0.723 | 0.070 |
Opioids | X | −0.593 | 0.193 | X | −0.524 | 0.197 |
Volatile solvents | X | −0.393 | 0.191 | X | −0.387 | 0.192 |
Ethnicity black | X | 0.378 | 0.060 | X | 0.378 | 0.061 |
Recurrent ATPD within 3 months | X | 0.350 | 0.155 | X | 0.346 | 0.156 |
Stimulants | X | −0.296 | 0.149 | X | −0.316 | 0.154 |
Antipsychotic treatment in the first month | X | 0.302 | 0.072 | X | 0.299 | 0.074 |
Hallucinogens | . | X | −0.278 | 0.201 | ||
Abrupt onset of ATPD episode | X | −0.163 | 0.096 | X | −0.159 | 0.096 |
Family history positive for any psychotic disorder | X | 0.145 | 0.067 | X | 0.138 | 0.067 |
Substance use (any) | X | 0.190 | 0.063 | X | 0.133 | 0.105 |
ATPD length >1 month | X | −0.109 | 0.068 | X | −0.110 | 0.068 |
N of substances used | . | X | 0.056 | 0.069 | ||
Antidepressant treatment in the first 3 months | . | X | 0.055 | 0.111 | ||
Antidepressant treatment in the first month | . | X | 0.041 | 0.101 | ||
Age (years) | . | X | −0.002 | 0.002 | ||
Time since first observation of attenuated/psychotic symptoms to the ATPD index diagnosis (days) | X | <0.001 | <0.001 | X | <0.001 | <0.001 |
Gender | . | . | ||||
Presence of psychiatric comorbidity at baseline | . | . | ||||
Acute stress events | . | . | ||||
Alcohol | . | . | ||||
Cannabinoids | . | . | ||||
Cocaine | . | . | ||||
n = 23 | n = 12 | n = 17 |
Note: ATPD, acute and transient psychotic disorder.
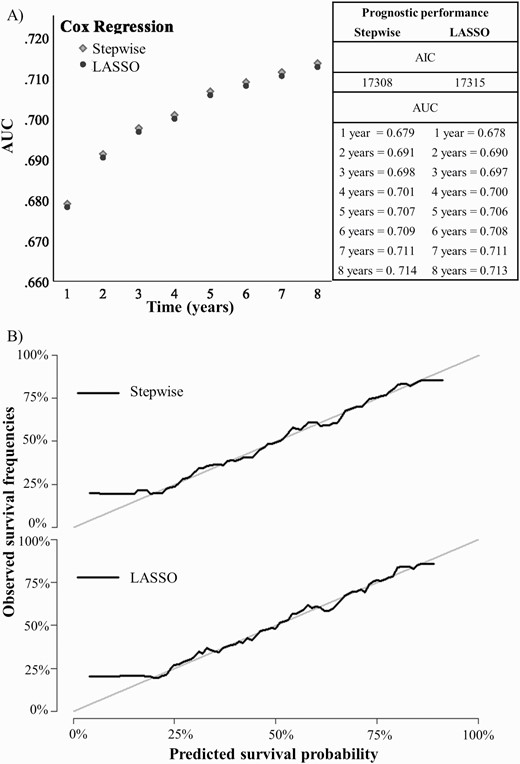
Internally validated (A) discrimination (AUC) and (B) calibration of the electronic health record-based, individualized prognostic model of psychotic recurrence for ATPD (n = 3018). Note: ATPD, acute and transient psychotic disorder; AUC, area under the curve.
Sensitivity Analyses
When only the polymorphic subtypes of ATPD were considered (307 individuals), 7 predictors were selected by the stepwise approach and 8 by LASSO. Some factors were associated with an increased probability of psychotic recurrence (stepwise and LASSO: time since first observation of attenuated/psychotic symptoms to the ATPD index diagnosis, antipsychotics at 3 months, recurrent ATPD episodes within 3 months; stepwise only: cannabinoids; LASSO only: antidepressants at 1 month and antipsychotics at 1 month), while others were associated with a reduced likelihood of psychotic recurrence (stepwise and LASSO: use of stimulants or volatile solvents; stepwise only: number of substances; LASSO only: age). The AIC was 1337 for stepwise and 1339 for LASSO. Internally validated AUC values increased over time, peaking to 0.699 at 8 years for stepwise and 0.712 for LASSO. LASSO reached adequate AUC (ie, greater than 0.7), at 3 years follow-up. Graphical inspection of the calibration plots showed that both models have good calibration (see figure 3). The sensitivity analyses did not show substantial changes in prognostic accuracy after removing the predictors which may be particularly subject to ecological biases (eTable 6). Decision curve analyses showed that the model holds theoretical clinical utility to forecast psychotic recurrences for individuals with a predicted risk of psychotic recurrence above 20% within 96 months (eFigure 1).
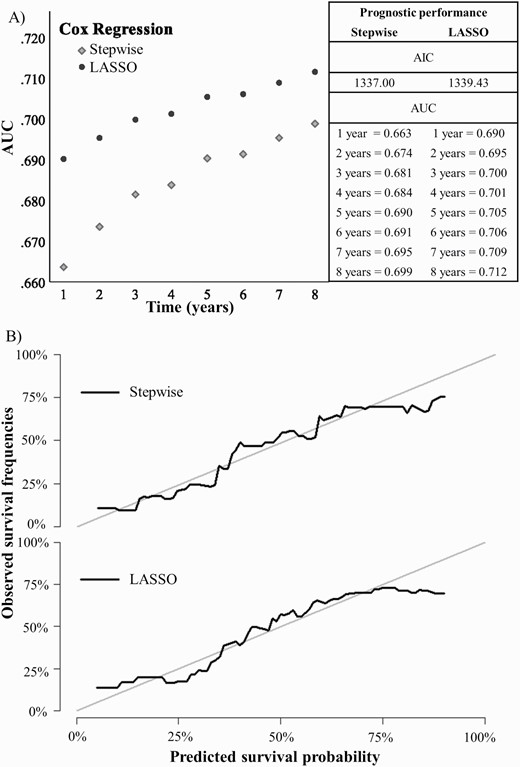
Internally validated (A) discrimination (AUC) and (B) calibration of the electronic health record-based, individualized prognostic model of psychotic recurrence for ATPD F23.0 and F23.1 (n = 307). Note: ATPD, acute and transient psychotic disorder; AUC, area under the curve.
Discussion
To our best knowledge, this is the first individualized clinical prediction model that has been developed and internally validated to forecast the risk of psychotic recurrence in ATPD. Across 3018 ICD-10 ATPD individuals, the prediction model reached an adequate 4 years level of prognostic accuracy and good calibration. A similar model developed and validated in view of the ICD-11 ATPD category retained adequate prognostic accuracy and calibration.
The main benefit of this study is to have employed state-of-the-art clinical prediction modeling methods and a large cohort of ATPD cases with a long follow-up, leveraging real-world EHR clinical data. This methodological approach holds the potential to overcome some of the main barriers of precision medicine to date.44 First, by using large cohorts with a substantial number of events (ie, psychotic recurrences), we were able to minimize the risk of reducing the EPV ratio, thus minimizing the risk of overfitting producing unstable risk prediction models.11 Accordingly, the EPV fell well beyond the recommended threshold of 10. Second, to further minimize the risk of overfitting our model to the data, we compared stepwise regression models with automatic selection of predictors via LASSO. Third, to estimate the out-of-sample generalizability, we performed an internal validation of the best model. A recent systematic review showed that only about 10% of risk prediction models developed and published in psychiatry is subjected to proper internal validation.44
A previous study has developed a multivariable prognostic model to ATPD cases to predict their diagnostic changes at follow-up, reporting a prognostic accuracy of 0.836 at 2 years. However, such prognostic performance was not validated internally or externally and may therefore reflect overfitting issues; the model was also developed on a small sample (n = 68) with few events (n = 30, corresponding to a suboptimal EPV = 5),45 which are known factors increasing overfitting. Fourth, we employed clinical predictors that can be available to clinicians as part of their clinical routine. Building simple prediction models that are not associated with logistic complexities and high costs is a prerequisite to facilitate subsequent implementation. Fifth, these predictors can be potentially automatable through their digital acquisition, exploiting EHR. We have recently demonstrated how individualized risk prediction algorithms can be implemented into EHR to screen large datasets and detect individuals that are more likely to develop severe mental disorders such as psychosis.46,47 Sixth, we have employed a long-term follow-up (up to 8 years) to fully characterize the dynamic nature of ATPD.
When characterizing the dynamic progression of prognostic accuracy (AUC) for ATPD, we found that levels of discrimination increased over time and, as depicted in figures 2 and 3, reached an adequate level (ie, AUC ≥0.70) at 4 years of follow-up. The model did not predict adequately in the shorter period, likely because of the reduced number of events. Overall, this level of accuracy is beyond chance and comparable to that of structural neuroimaging methods (ie, gray matter volume) to detect a first episode of psychosis at the individual level, with accuracies ranging from 0.5 to 0.63.48 A recent machine-learning study validating a risk calculator to predict treatment outcome in depression in 151 patients, reported a 1-year prognostic accuracy of 0.59 and concluded that, if implemented at scale, performance even only significantly above chance can be considered to be clinically useful.49 Risk calculators developed on real-world EHR data offer the additional potential for automatically screening large mental health populations. Psychiatry is undergoing a digital revolution,50 and there is an ongoing expansion of EHR adoption worldwide. Harnessing data from EHRs are more convenient than other methods such as patient recruitment because most of the predictors are available as part of clinical routine. Clinically based prognostic models can be theoretically transportable and tested for external generalizability in several settings including low/middle income countries, where ATPD appear particularly frequent.51,52
Several prognostic factors were identified by our analyses. Risk factors selected by both the stepwise and LASSO were (in decreasing strength of association with psychotic recurrences): antipsychotic exposure within the first 3 months, black ethnicity, recurrent episodes of ATPD within the first 3 months, antipsychotic exposure within the first month, family history and substance abuse (any), and antipsychotic exposure within the first 3 months. In Northern countries, black ethnicity is one of the most robust risk factors for psychosis with a convincing level of evidence (OR for association with psychosis = 4.87, 95% CI 3.96–6.00) in recent umbrella reviews.53 It likely acts as a spurious factor encompassing immigration-related distress and social discrimination, which mediate the risk for psychosis in these individuals. Recurrent episodes of ATPD may index a more insidious onset with shorter remission periods and therefore poorer prognosis. The association with exposure to antipsychotics within the first months may be a proxy of higher symptoms severity which indicates a poorer prognosis. As noted above, use of antipsychotic in this group is challenging for empirical and ethical considerations. Family history may index a shared genetic or environmental susceptibility, for which little research is available. Time-related factors such as the presence and recurrence of attenuated psychotic symptoms or frank psychotic before an index diagnosis may negatively influence the outcome among psychotic disorders54 (however, see eMethods 2 for limitations).
Protective factors selected by both stepwise and LASSO were (in decreasing strength of association with psychotic recurrences) the use of opioids, stimulants, volatile solvents, abrupt onset, and length of ATPD episode <1 month. The findings from the use of substances are partially contrasting and may be due to the type of substance considered. Overall, the better prognosis of exogenous ATPDs may be explained by the fact that in the absence of repeated use of these molecules, psychotic recurrences are less likely. There was no protective effect given by the duration of symptoms lasting less than 1 month (our models actually identified ATPD episodes lasting less than 1 month as a risk factor). This is clinically relevant because it suggests that redefining ATPD duration to 1 month (to better align it with the DSM-5 BPD) may not sensibly change the prognosis.
Surprisingly, while it has been suggested that female gender is an important factor determining outcomes in this patient group,55 we did not confirm this finding. However, the primary aim of this clinical prediction study was to forecast outcomes rather than explaining ATPD aetiopathology, and the above speculations should be considered cautiously.
Overall, the current study may advance clinical knowledge for individuals with ATPD. The next step would require external validation in independent datasets; the model’s coefficients presented here may facilitate this work. Our prognostic model was developed in a highly diverse population characterized by elevated ethnic and social variance: these features may support the generalizability of our model in other clinical settings, even outside the United Kingdom. At the same time, future refinements may involve the use of artificial intelligence (eg, natural language processing apps)18 to extract more fine grained predictors, or to develop dynamic prediction models that can update their prognosis over time.56 The ICD-11 compatible version of the current prognostic model (which offers comparable AUCs) will ensure that the current findings will be applicable to the next generation of research in this field. This would ultimately allow a personalized prognosis, and therefore tailored antipsychotics or relapse prevention strategies to ATPD individuals more in need.
The present study has some limitations. First, being the data retrospectively collected through EHR, the psychotic diagnoses in our analyses, and their predictors, are high in ecological validity (ie, they represent real-world clinical practice and pathways), but they have not been subjected to formal validation with research-based psychometric criteria. However, the use of structured diagnostic interviews or psychometric instruments to define ATPD and their predictors can lead to selection biases, decreasing the transportability of the prognostic model outside research frameworks (eg, in low/middle income countries which can access limited research infrastructures).57 There is also meta-analytical evidence indicating that within psychotic disorders, data recorded in clinical registers are indicative of truly validated diagnoses.58 As the primary aim of this study was to develop a clinical prediction model which could potentially be used in clinical practice, and not to test the reliability/validity of ATPD diagnoses per se, the ecological framework provided the ideal pragmatic asset. Furthermore, we have already externally replicated clinical prediction models that were developed in this EHR, including ATPD diagnoses. Such multiple replications demonstrate that the current ATPD diagnoses/predictors retain adequate generalizability outside their original setting.20,59,60 Second, the prognostic accuracy remains suboptimal and should be improved. There is scope for optimization of the current risk calculator through model refinement, which is a recommended method for advancing prognostic knowledge.11 For example, we did not consider additional factors that may play a role in modulating the likelihood of psychotic recurrences, such as catatonia-like psychomotor disturbances, hallucinations and first rank symptoms of schizophrenia, premorbid social and cognitive functioning, education years, and comorbid psychiatric diagnoses,61–64 mostly because these were not consistently recorded in the EHR. Third, as noted above, this model was not externally validated, and its international replicability should be confirmed.
Conclusions
This study presents the first clinical prediction model developed and internally validated to predict psychotic recurrence in individuals with ATPD. Its prognostic performance is adequate at/after 4 years, and the model can be automatable in EHRs. Further research should externally validate it and refine it.
Funding
This work was supported by the King’s College London Confidence in Concept award from the Medical Research Council (MRC) (MC_PC_16048) to P.F.-P.
Acknowledgments
The authors have declared that there are no conflicts of interest in relation to the subject of this study.