-
PDF
- Split View
-
Views
-
Cite
Cite
Lotta-Katrin Pries, Agustin Lage-Castellanos, Philippe Delespaul, Gunter Kenis, Jurjen J Luykx, Bochao D Lin, Alexander L Richards, Berna Akdede, Tolga Binbay, Vesile Altinyazar, Berna Yalinçetin, Güvem Gümüş-Akay, Burçin Cihan, Haldun Soygür, Halis Ulaş, Eylem Şahin Cankurtaran, Semra Ulusoy Kaymak, Marina M Mihaljevic, Sanja Andric Petrovic, Tijana Mirjanic, Miguel Bernardo, Bibiana Cabrera, Julio Bobes, Pilar A Saiz, María Paz García-Portilla, Julio Sanjuan, Eduardo J Aguilar, José Luis Santos, Estela Jiménez-López, Manuel Arrojo, Angel Carracedo, Gonzalo López, Javier González-Peñas, Mara Parellada, Nadja P Maric, Cem Atbaşoğlu, Alp Ucok, Köksal Alptekin, Meram Can Saka, Genetic Risk and Outcome of Psychosis (GROUP) investigators , Celso Arango, Michael O’Donovan, Bart P F Rutten, Jim van Os, Sinan Guloksuz, Estimating Exposome Score for Schizophrenia Using Predictive Modeling Approach in Two Independent Samples: The Results From the EUGEI Study, Schizophrenia Bulletin, Volume 45, Issue 5, September 2019, Pages 960–965, https://doi.org/10.1093/schbul/sbz054
- Share Icon Share
Abstract
Exposures constitute a dense network of the environment: exposome. Here, we argue for embracing the exposome paradigm to investigate the sum of nongenetic “risk” and show how predictive modeling approaches can be used to construct an exposome score (ES; an aggregated score of exposures) for schizophrenia. The training dataset consisted of patients with schizophrenia and controls, whereas the independent validation dataset consisted of patients, their unaffected siblings, and controls. Binary exposures were cannabis use, hearing impairment, winter birth, bullying, and emotional, physical, and sexual abuse along with physical and emotional neglect. We applied logistic regression (LR), Gaussian Naive Bayes (GNB), the least absolute shrinkage and selection operator (LASSO), and Ridge penalized classification models to the training dataset. ESs, the sum of weighted exposures based on coefficients from each model, were calculated in the validation dataset. In addition, we estimated ES based on meta-analyses and a simple sum score of exposures. Accuracy, sensitivity, specificity, area under the receiver operating characteristic, and Nagelkerke’s R2 were compared. The ESMeta-analyses performed the worst, whereas the sum score and the ESGNB were worse than the ESLR that performed similar to the ESLASSO and ESRIDGE. The ESLR distinguished patients from controls (odds ratio [OR] = 1.94, P < .001), patients from siblings (OR = 1.58, P < .001), and siblings from controls (OR = 1.21, P = .001). An increase in ESLR was associated with a gradient increase of schizophrenia risk. In reference to the remaining fractions, the ESLR at top 30%, 20%, and 10% of the control distribution yielded ORs of 3.72, 3.74, and 4.77, respectively. Our findings demonstrate that predictive modeling approaches can be harnessed to evaluate the exposome.
Introduction
Several environmental exposures have been associated with psychosis spectrum disorder.1,2 Knowledge on this association has thus far been deduced from hypothesis-driven selective one-exposure to one-outcome studies, akin to the candidate-gene approach.3 However, each exposure constitutes a fraction of a dense network of exposures: the exposome.4 Here, we argue for embracing the exposome paradigm to investigate the sum of the nongenetic “risk” and show how a predictive modeling approach can be used to construct an exposome score (ES) for schizophrenia, a single metric of aggregated environmental load similar to polygenic risk score.5
Approach
Guided by the predictive modeling methods for constructing cumulative environmental exposure scores,6,7 we used 2 independent datasets to, first, build a predictive model in the training dataset (the Work-package 6 of the European Network of National Networks studying Gene-Environment Interactions in Schizophrenia [EUGEI]2) and, second, construct and test the ES in the validation dataset (the Genetic Risk and Outcome of Psychosis [GROUP] study8). We examined the following widely evaluated environmental factors that we also recently investigated individually within the context of gene-environment interaction9: hearing impairment, winter birth, cannabis use, and childhood adversities (bullying, emotional, physical, and sexual abuse along with emotional and physical neglect).10 Our analysis was limited to the environmental exposures that were reliably measured and equally available in both datasets. These environmental factors were defined according to previous studies.9 The detailed description of each environmental exposure is provided in the supplementary file. We used 4 prediction models to determine to what degree cumulative environmental exposure contributes to the liability for schizophrenia in a case-control design. Logistic regression (LR), Gaussian Naive Bayes (GNB), and penalized logistic regression (least absolute shrinkage and selection operator [LASSO] and Ridge) were applied to data with complete information on environmental exposures. The description of the models and the distribution of exposures are provided in the supplementary file. For each model, the dependent variable was the binary case-control status, whereas binary environmental exposures were features (independent variables). First, we estimated coefficients of binary exposures in the training dataset including 1241 healthy controls and 747 patients with a diagnosis of schizophrenia spectrum disorders. Second, we calculated the weighted sum of the exposures according to each predictive model in an independent validation dataset with 323 healthy controls, 463 patients with a diagnosis of schizophrenia spectrum disorders, and 542 unaffected siblings of the patients. To compare the performance of ES from each model, we also generated an environmental sum score by simply adding each binary exposure per individual as 0 = absent and 1 = present (the sum score is ranging from 0 to 9) and a cumulative environmental score weighted by the meta-analytical estimates for each exposure,11–14 conforming to a previous study.15 Finally, we tested the performance of ESs derived from each model by applying logistic regression in a case-control design in the independent validation dataset by evaluating the area under the receiver operating characteristic (ROC), accuracy (ACC), sensitivity, specificity, and Nagelkerke’s pseudo R2. In this regard, we prioritized models with better sensitivity than specificity as our main concern was to avoid misclassifying individuals diagnosed with schizophrenia.
Prediction in the Training Dataset
The coefficients of individual models (see figure 1a and supplementary table S2) indicate that cannabis use (coefficients ranging from 1.31 to 1.53), hearing impairment (coefficients: 1.10–1.19), and bullying (coefficients: 1.30–1.57) received the highest weights in the training dataset. The lowest weight was attributed to winter birth with coefficients between 0.01 and 0.06. In comparison with the GNB model, which assumes independence between predictors, the LR, Ridge, and LASSO models yielded lower weights for emotional abuse, sexual abuse, emotional neglect, physical neglect, and bullying. Further, although physical abuse was a strong positive predictor in the GNB model, its predictive value was lost and even yielded a negative weight when using predictive model approaches that account for dependence between the predictors. This is in line with evidence that exposures are weakly to moderately correlated with each other.3,16–18 Consequently, coefficients are overestimated when independence is assumed.
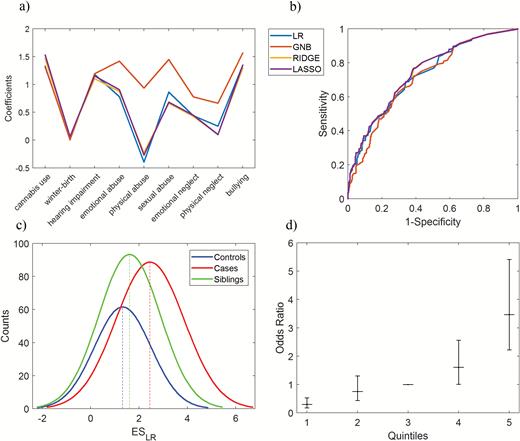
(a) Coefficients profile for each exposure derived from different classification methods in the training dataset, GNB: Gaussian Naive Bayes, LR: logistic regression. (b) The area under the receiver operating characteristic for the different exposome scores in the validation dataset. (c) The histogram of the ESLR (exposome score based on logistic regression) for patients, siblings, and controls in the validation dataset. For visualization, a Gaussian distribution was fit to histogram counts by adjusting mean and standard deviations. (d) The risk strata plot of the ESLR on case-control status: The ESLR was divided into 5 quintiles (X-axis) of the control distribution and logistic regression was applied to case-control status as the dependent variable. The third quintile includes the median and was used as reference. The Y-axis represents odds ratios and the error bars show confidence intervals.
Constructing and Testing the Performance of Exposome Score in an Independent Dataset
The ROC was used to estimate the performance of the calculated ESs in predicting the case-control status in the validation dataset (figure 1b and supplementary table S3). The ES based on meta-analytical estimates (the ESMeta-analyses), ESGNB, and the environmental sum score yielded the lowest ROC, 0.69, 0.71, and 0.71, respectively, whereas all other ESs (ESLR, ESRIDGE, and ESLASSO) had ROC ranging from 0.73 to 0.74. With a chance level of 0.5 (as patients and controls were in balance in the training sample; see supplementary file), all ESs indicated an ACC above chance level (ACC: 0.62–0.68) with specificity between 0.42 and 0.72 and sensitivity between 0.56 and 0.86. Compared to the ESLR, ESRIDGE, and ESLASSO, the ESs derived from the models assuming independence between exposures (ESGNB, environmental sum score, and ESMeta-analyses) performed worse on sensitivity and had more false negatives as they incorrectly classified patients as healthy. Given that our priority was reducing false negatives rather than reducing false positives and that the ESLR, ESRIDGE, and ESLASSO performed similarly well (figure 1b and supplementary table S3), we reported further analyses with the ESLR, which was constructed on the basis of a widely available and commonly used statistical model, logistic regression.
To examine whether the ESLR reflects schizophrenia liability in the validation dataset, we evaluated the ESLR in patients, siblings, and controls (see figure 1c for an illustration and supplementary table S4 for the other models). The ESLR discriminated patients from controls (odds ratio [OR] = 1.94; 95% confidence interval [CI] = 1.71–2.20; P < .001, Nagelkerke’s pseudo R2 = 0.21), also after adjusting for age and sex (OR = 1.87; 95% CI = 1.64–2.14; P < .001) in the validation dataset. Similarly, logistic regression analysis showed higher ESLR in patients compared to siblings (OR = 1.58; 95% CI = 1.43–1.74; P < .001; adjusted for age and sex: OR = 1.55; 95% CI = 1.40–1.72; P < .001) and in siblings compared to controls (OR = 1.21; 95% CI = 1.08–1.36; P = .001; adjusted for age and sex: OR = 1.23; 95% CI = 1.09–1.38; P < .001).
To visually represent the risk stratification properties of the ESLR, we categorized the ESLR using the quintiles of the control distribution and measured the case-control ORs using the middle quintile (median ESLR) as the reference. With an increase of the ESLR, we noticed a gradient increase in the risk for schizophrenia. In comparison with the median, the fifth quintile had a higher OR (OR = 3.47; 95% CI = 2.22–5.41; P < .001 and age- and sex-adjusted OR = 3.78; 95% CI = 2.34–6.09; P < .001) and the first quintile had a lower OR (OR = 0.30; 95% CI = 0.17–0.53; P < .001 and age- and sex-adjusted OR = 0.34; 95% CI = 0.19–0.62; P < .001; figure 1c). We then dichotomized the ESLR with cutoff points at 70%, 80%, and 90% of the control distribution. Comparing the top and the bottom part translated to ORs of 3.81, 3.96, and 5.11 (age- and sex-adjusted ORs of 3.72, 3.74, and 4.77) for 70%, 80%, and 90% of the distribution, respectively (supplementary table S5).
Discussion
For the first time, we applied a predictive modeling approach to construct the ES for schizophrenia by leveraging 2 large independent datasets (training and validation data) with similar assessment protocols for environmental exposures. Our findings suggest that predictive modeling can be used to estimate environmental loading of a range of exposures. We found that the ESLR, ESRIDGE, and ESLASSO performed similarly well, whereas the ESs derived from the models assuming independence performed worse. Of the ESGNB, ESMeta-analyses, and the simple summation of exposures, the ESMeta-analyses, relying on the external sources for extracting estimates for environmental exposures, showed the worst performance.
The low performance of the ES driven by meta-analyses might be related to the fact that meta-analytical estimates are derived from different studies that use different assessments, different definitions, and different cutoff points for exposures in different study populations,3 which might not be completely compatible with the dataset at hand. The availability of similar training and validation datasets plays a major role in prediction power—for instance, the predictive performance of polygenic scores for schizophrenia is considerably lower in non-Caucasian ancestry samples.19 Therefore, a similar situation exists in estimating genetic liability, which, however, has the advantage of using more concrete, uniformly measured genetic variation for prediction in comparison to environmental assessment. Generating a uniform “environmental risk score” is even more challenging. For instance, cannabis use could be scored positive if participants smoke daily, or at least weekly, or at least monthly for lifetime use or exposure during adolescence, whereas childhood adversities could similarly be measured by various methods. Therefore, as weights are determined by how strict or lenient the cutoff points are, it is likely that the inconsistency between sampling and measurement strategies would introduce bias. Further, when individual coefficients from meta-analyses are used for a weighted environmental score, correlations between exposures are ignored, and weights may be overestimated.3 In line with this, we also show that GNB, which assumes independence between predictors, produces higher weights for exposures than the other data-driven models.
Similar to current results, previous studies show that more contemporary algorithms do not necessarily translate into superior performance over logistic regression for clinical prediction modeling.20,21 However, it should be noted that our analysis did not involve a complex data structure with many predictors. Penalized classification models might have led to performance improvement if more complex structures had to be considered (eg, increasing the number of predictors and adding pairwise interactions). Researchers likewise need to be cautious about overfitting models and be aware that, if environmental exposures are correlated, the initial simple model with a few predictors will show the highest portion of improvement. However, each sequentially added predictor would result in less and less improvement in model performance.21
The ESs assuming independence between predictors (sum score, ESMeta-analyses, and ESGNB) had lower sensitivity than the rest. The ESMeta-analyses indicated the lowest sensitivity (56%). The sensitivity of an environmental score derived from meta-analytical estimates in a previous study was even lower, only around 7%–9%.15 In other words, predictive models that do not assume independence between exposures may more accurately classify patients as positive by decreasing false negatives. The ESs from the models assuming independence, however, had higher specificity and were better in decreasing false positives. As our main concern was to avoid misclassifying individuals diagnosed with schizophrenia, we chose sensitivity over specificity. Further, if more environmental exposures were to be included in the ES, thus introducing more correlation, the models not assuming independence between predictors (ESLR, ESRIDGE, and ESLASSO) would perform increasingly better than the models assuming independence (sum score, ESMeta-analyses, and ESGNB).
The ESLR, generated using an easily accessible method (logistic regression), achieved similar performance results compared with the ESRIDGE and ESLASSO. We used the ESLR to further explore the characteristics of the ES in the follow-up analyses. In general, patients had higher ESLR than both controls and siblings, whereas siblings had higher ESLR than controls. The ESLR explained more variance (Nagelkerke R2 = 0.21) than the ESMeta-analyses (Nagelkerke R2 = 0.13). In accordance with our previous findings showing an additive effect for environmental factors,22,23 our results indicate that the ESLR shows a dose-response effect: the odds of schizophrenia increase as a function of the ESLR. Eventually, an individual with ESLR in the top 10% of the control distribution was around 5 times more likely to have schizophrenia compared to an individual below that cutoff.
Limitations of Exposome Score
Our analysis was limited to the environmental exposures that were reliably measurable and equally available in both datasets. The ES can be extended to include other environmental exposures (eg, obstetric and pregnancy complications and urban environment). We included winter birth as an exposure in the current analyses as previous studies suggest an association between winter birth and psychosis.14 However, summer birth was also previously associated with deficit schizophrenia and might therefore be evaluated as an exposure as well.24,25 Considering evidence showing that common environmental factors (eg, childhood adversity) are not specific to the psychosis phenotype but instead are more generally related to psychopathology,26,27 the ES would likely (to a degree) be associated with other mental disorders in mixed samples. Therefore, a low discriminant capacity for the ES should be anticipated. Given the nature of observational studies, causality claims should be avoided. Finally, it should be noted that although aggregating exposures leads to an increase in the predictive power and may be particularly beneficial in exploring shared mechanisms, the inherent heterogeneity of a single score may lead to information loss and biological imprecision. Considering the reasons described earlier, we have avoided using the term “risk” and opted for a neutral alternative: ES.
Conclusion
Our findings demonstrate that predictive modeling approaches can be harnessed to evaluate the exposome. In the future, we aim to explore models by including more exposures as well as interaction terms and test the predictive power of the ES in epidemiologically representative general population cohorts.
Funding
The EUGEI project was supported by the grant agreement HEALTH-F2-2010-241909 from the European Community’s Seventh Framework Programme. The authors are grateful to the patients and their families for participating in the project. They also thank all research personnel involved in the GROUP project, in particular J. van Baaren, E. Veermans, G. Driessen, T. Driesen, E. van’t Hag and J. de Nijs. Bart PF Rutten was funded by a VIDI award number 91718336 from the Netherlands Scientific Organisation.
Appendix
GROUP-EUGEI investigators are: Behrooz Z. Alizadeh1, Therese van Amelsvoort2, Richard Bruggeman1, Wiepke Cahn3,4, Lieuwe de Haan5, Jurjen J. Luykx3,6,7, Ruud van Winkel2,8, Bart P.F. Rutten2, Jim van Os2,3,9
University of Groningen, University Medical Center Groningen, University Center for Psychiatry, Rob Giel Research center, Groningen, The Netherlands;
Maastricht University Medical Center, Department of Psychiatry and Neuropsychology, School for Mental Health and Neuroscience, Maastricht, The Netherlands;
Department of Psychiatry, UMC Utrecht Brain Center, University Medical Center Utrecht, Utrecht University, Utrecht, the Netherlands;
Altrecht, General Menthal Health Care, Utrecht, The Netherlands;
Amsterdam UMC, University of Amsterdam, Department of Psychiatry, Amsterdam, The Netherlands;
Department of Translational Neuroscience, UMC Utrecht Brain Center, University Medical Center Utrecht, Utrecht University, Utrecht, The Netherlands;
GGNet Mental Health, Apeldoorn, The Netherlands;
KU Leuven, Department of Neuroscience, Research Group Psychiatry, Leuven, Belgium King’s;
College London, King’s Health Partners, Department of Psychosis Studies, Institute of Psychiatry, London, United Kingdom
References
Author notes
These authors contributed equally to the article.
The Genetic Risk and Outcome of Psychosis (GROUP) investigators in EUGEI are listed in the Appendix.