-
PDF
- Split View
-
Views
-
Cite
Cite
Hui Wu, Yaxi Liu, Yunwu Han, Bingdong Liu, Shengyun Chen, Zhiye Ye, Jianbo Li, Liwei Xie, Xiaoli Wu, Integrated Analysis of Gut Microbiome, Inflammation, and Neuroimaging Features Supports the Role of Microbiome–Gut–Brain Crosstalk in Schizophrenia, Schizophrenia Bulletin Open, Volume 5, Issue 1, January 2024, sgae026, https://doi.org/10.1093/schizbullopen/sgae026
- Share Icon Share
Abstract
Gut microbiota has been implicated in the pathogenesis of schizophrenia (SZ) and relevant changes in the brain, but the underlying mechanism remains elusive. This study aims to investigate the microbiota–gut–brain crosstalk centered on peripheral inflammation in SZ patients.
We recruited a cohort of 182 SZ patients and 120 healthy controls (HC). Multi-omics data, including fecal 16S rRNA, cytokine data, and neuroimaging data, were collected and synthesized for analysis. Multi-omics correlations and mediation analyses were utilized to determine the associations of gut microbiome with inflammatory cytokines and neuroimaging characteristics. Additionally, machine learning models for effective SZ diagnosis were separately generated based on gut microbial and neuroimaging data.
Gut microbial dysbiosis, characterized by a decrease in butyrate-producing bacteria and an increase in proinflammatory bacteria, has been identified in SZ patients. These key microbial taxa were associated with increased inflammatory cytokines, potentially through mediating lipid metabolic pathways such as steroid biosynthesis and linoleic acid metabolism. Further analysis revealed altered microbial genera to be correlated with disrupted gray matter volume and regional homogeneity in SZ patients. Importantly, certain inflammatory cytokines mediated the relationship between the SZ-enriched genus Succinivibrio and aberrant activity of anterior cingulate cortex and left inferior temporal gyrus in the SZ group. Moreover, the classification model based on gut microbial data showed comparable efficacy to the model based on brain functional signatures in SZ diagnosis.
This study presents evidence for the dysregulated microbiota–gut–brain axis in SZ and emphasizes the central role of peripheral inflammation.
Introduction
Schizophrenia (SZ) is the most disabling psychiatric disorder with a lifetime prevalence of about 1%,1 threatening public health for a long time. Several hypotheses, such as neurodevelopmental hypothesis,2 neurotransmitter hypothesis.3 and immunoinflammatory hypothesis,4 have been proposed for the pathogenesis of SZ. However, none of these hypotheses has fully elucidated the intricate nature of SZ.
In recent years, researches on the “microbiome–gut–brain axis” have attracted increasing attention, shedding light on the pathogenesis of SZ.5 On the one hand, gut microbiota actively participates in food digestion, converting dietary nutrients into neuroactive metabolites such as short-chain fatty acids (SCFA), which could then regulate the secretion of neurotransmitters (eg, DA, 5-HT, Glu, and GABA) after being absorbed into the blood.6 On the other hand, the gut microbiota assumes a pivotal role in establishing and sustaining immune function. Microbial dysbiosis has been linked to elevated proinflammatory cytokines,7 potentially fostering a chronic inflammatory state intricately tied to the neurodevelopment and onset of SZ.
Emerging evidence indicates gut microbial alterations in SZ patients, including changes in microbial diversity8,9 and shifts in the composition of certain strains.10,11 A review and meta-analysis,12 synthesizing evidence on bacterial taxa commonly linked to mental disorders, including SZ, showed that these disorders are characterized by a decrease in anti-inflammatory butyrate-producing bacteria and an increase in proinflammatory genera. Similar findings were further confirmed in other systematic reviews,13,14 providing additional evidence for the potential contribution of microbiota-derived inflammation in the pathophysiology of SZ. Significantly, inflammation serves as a conduit facilitating crosstalk between the brain and the gut microbiota. For example, elevated proinflammatory cytokines have been detected in both the blood and cerebrospinal fluid of patients with SZ, correlating with impaired cognitive functions.15,16 Additionally, findings of microglial activation and reduced brain volume in neuroimaging studies further substantiate the involvement of subtle neuroinflammatory processes in SZ.17 Nevertheless, the relationship of gut microbiota and microbiota-derived inflammation with brain structure and functional activity remains incompletely understood.
In this study, we conducted a multi-omics analysis to explore the crosstalk between the gut microbiome and the brain centered on peripheral inflammation in SZ patients. Specifically, we initially identified the gut microbiota and neuroimaging features in SZ patients. Then, we examined the correlations of these gut microbiota with brain structure and function, as well as the mediating role of inflammatory markers. Finally, we compared the efficacy of machine learning models based on gut microbiota data and models based on brain imaging data in SZ diagnosis. We hypothesize that a comprehensive analysis of gut microbiota, inflammatory cytokines, and brain imaging features would not only clarify their interrelationships but also unveil the pathophysiological of SZ.
Materials and Methods
Study Design and Participants
All the participants were recruited from the same local geographic areas in Kashi, Xinjiang, China, between December 2020 and October 2021. In total, 302 Chinese Uyghur participants were included in this study, including 182 SZ patients, and 120 healthy controls (HC). The SZ patients were recruited from the Psychiatry Department of the First People’s Hospital of Kashi Prefecture, and the detailed enrollment process is shown in Supplementary figure S1. Simultaneously, healthy controls (HC) matching the basic demographic information were recruited from the physical examination center and local communities through advertising. The sample size assessment is presented in Supplementary Method 1.
Inclusion criteria for SZ patients: (1) met the diagnostic criteria for “schizophrenia” according to the International Classification of Diseases the tenth edition (ICD-10); (2) aged 16–60 years; (3) right handedness; (4) had a period of 6 or more years of education; (5) family members or legal guardians of patients fully understood the study contents and had given written, informed consent.
Inclusion criteria for HC: (1) no personal or family history of mental disorder refer to the Mini-International Neuropsychiatric Interview (M.I.N.I); (2) age 16–60 years; (3) right handedness; (4) had a period of 6 or more years of education; (5) family members or legal guardians of patients fully understood the study contents and had given informed consent.
Exclusion criteria: (1) history of other mental disorder, including anxiety, depression, bipolar disorder, mental retardation, eating disorders, etc.; (2) alcohol or substance addiction; (3) have taken any antibiotics, probiotics, or prebiotics within 1 month before sampling; (4) metabolic related diseases such as hypertension, diabetes, chronic hepatitis, hypothyroidism, or hyperthyroidism; (5) had a history of allergy, hormone therapy, or received immune agent treatment in the past 3 months; (6) had gastrointestinal surgery, gastroscopy, colonoscopy, or gastrointestinal barium meal examination in the past 1 year; (7) experienced major life events or changes in living environment and diet in the past 1 month; (8) significant brain lesions, including cerebral infarction, hemorrhage, or intracranial masses; (9) any MRI contraindications, such as metallic implants and claustrophobia; (10) pregnant or lactating women.
Psychological and Clinical Assessments
The SZ diagnosis was made by an associate chief physician using the M.I.N.I. procedure according to the ICD-10 diagnostic criteria. The M.I.N.I. is a short structured diagnostic interview for DSM-IV and ICD-10 psychiatric disorders, with good reliability, validity, and researcher consistency.18 The severity of symptoms in SZ patients was assessed using the Positive and Negative Syndrome Scale (PANSS), a widely used tool for this purpose. PANSS comprises 30 items categorized into 3 symptom dimensions: positive syndrome (7 items), negative syndrome (7 items), and general psychopathology (16 items).19,20 The Montreal cognitive assessment (MoCA) was adopted to measure the cognitive performance in all participants. The MoCA is a cognitive screening test, which provided scores for 7 cognitive domains: visuospatial/constructional abilities, naming, language, attention, abstraction, delayed memory, and orientation.21
Additionally, a self-made questionnaire was used to obtain general data including age, gender, ethnicity, years of education, illness duration, previous treatment with antipsychotics (type, dose, and duration), and lifestyle (smoking, drinking history, eating habits). The weight, height, waist and hip circumferences, and blood pressure of all subjects were tested and collected by the same nurse. Subjects were weighed in a fasting state in light clothing with footwear removed.
Samples Collection
Blood samples were collected from all 182 recruited SZ patients. Blood samples were drawn from fasting individuals by venipuncture between 08:00 am and 10:00 am and stored at −80°C after centrifugating. Fecal samples were collected from 112 SZ patients and 72 matched HC subjects. Subjects were asked to deposit stool into a sterile plastic container and hand it over to a clinical assistant within half an hour after collection. The stool sample was then aliquoted using scoop into 5 sterile freezing tubes and immediately stored at −80°C prior to further processing.
Measurement of Inflammatory Cytokines
Serum interleukin-1β (IL-1β), interleukin-2 (IL-2), interleukin-6 (IL-6), interleukin-17 (IL-17), tumor necrosis factor- α (TNF- α), lipopolysaccharide (LPS), adiponectin (ADP), C-reactive protein (CRP), and leptin levels were measured by using enzyme-linked immunosorbent assay kits from ABclonal Technology Co., Ltd. All experimental procedures were performed following corresponding specifications.
16S rRNA Amplicon Sequencing and Analysis
We extracted bacterial DNA from fecal samples using the E.Z.N.A. Stool DNA Kit (D4015, Omega, Inc., USA) and obtained sequencing library. Subsequently, the library was sequenced on Illumina HiSeq2500 platform, and the amplified sequences were annotated and analyzed against the GreenGene database. Detailed information on library construction and sequencing are provided in Supplementary Method 2. For all taxonomic data submitted in the subsequent analysis, low abundant taxa (mean relative abundance < 0.1%; mean ratio < 50% within each group) were recognized as interfering elements and decontaminated.
Downstream bioinformatic analyses were performed using the “EasyMicroPlot” package based on the R platform.22 Shannon and Simpson indices were used to assess the α-diversity. Principal coordinates analysis (PCoA) based on Bray–Curtis distance was used to assess the β-diversity. Linear discriminant analysis (LDA) effect sizes (LEfSe) were used to detect differential abundance taxa, with thresholds set at LDA scores >2.0 and P < .05 (Wilcoxon rank sum test). The redundancy analysis (RDA) and correlation analysis were used to determine the relationship between the differential bacteria and clinical and imaging indicators. To probe the microbial function, PICRUSt2 was utilized to explore differences of the KEGG pathway between groups.23
Imaging Acquisition and Analysis
The structural MRI data were obtained from 143 SZ patients and 107 matched HC subjects, and the functional MRI data were obtained from 119 SZ patients and 106 matched HC subjects. The structural data were analyzed using Voxel-Based Morphometry (VBM) to calculate Gray Matter Volume (GMV); the functional data were analyzed using the Regional Homogeneity (ReHo) to analyze the brain spontaneous functional activity. The MRI data acquisition parameters and the preprocessing steps are presented in Supplementary Method 3.
We applied a whole-brain 2-sample t-test, controlling for age, sex, education, and total intracranial volume (ICV), to determine the differences in GMV/ReHo between the 2 groups. The voxel-wise threshold was set at P < .001 and corrected by a cluster-level familywise error (FWE) correction. For reporting purposes, the masks of significant cluster were defined using the automated anatomical labeling (AAL) atlas. Then, the brain regions with significant difference were saved as masks, and the mean GMV/ReHo values within each significant cluster were extracted for all subjects.
Machine Learning Prediction Model
Utilizing data from the gut microbiota (genus level) and brain imaging (ReHo index), distinct classification models were constructed to assess the inherent capacity of discriminating between the SZ and HC cohorts. The entire dataset was partitioned into training and validation subsets, with the validation set constituting 20% of the overall dataset. The training set was employed for model training, while the validation set was utilized to gauge the model’s generalizability. To maximize data utilization and enhance model stability, a 5-fold cross-validation strategy was adopted, coupled with the application of 5 distinct classifier algorithms: logistic regression classifier, linear discriminant analysis classifier (LDA), random forest classifier (RFC), support vector machine classifier (SVM), and multi-layer perceptron classifier (MLPC). The construction and evaluation of the models were conducted using Python 3.9.13 in a Jupyter environment, with the aid of the sklearn, pandas, and numpy libraries.
Statistical Analyses
Statistical analyses for all demographic and clinical data were performed using IBM SPSS Statistics for Windows Version 26.0 (IBM Corp., Armonk, NY), with the statistical significance threshold set to P < .05. The continuous variables were summarized by means ± SDs and analyzed by independent t-test or analysis of variance (ANOVA). The categorical variables were summarized using frequencies and analyzed by chi-square test. Correlation analyses were conducted using Spearman correlation coefficient, and false discovery rate was used to correct multiple testing. The mediation analysis was conducted by employing a model 4 of the PROCESS software (http://www.processmacro.org) implanted in SPSS, controlling for age, gender, and duration of illness.
Results
Demographic and Clinical Characteristics
Figure 1 illustrates the overview of the multi-omics study design. The demographic and clinical characteristics of all participants are summarized in Supplementary table S1. No significant differences were observed between the SZ and HC groups in terms of age, gender, BMI, and smoking or drinking status (P > .05), but the SZ group showed a significantly lower education level than the HC group (P < .05). In addition, SZ exhibited significant cognitive deficits across several domains compared to HC (P < .05).

Overview of the multi-omics study design. (A) Enrollment of SZ and HC subjects. (B) Characterization of the gut microbiota was examined by 16S ribosomal RNA sequencing. (C) Characterization of brain structure and function was examined by fMRI analysis. (D) The link between the gut microbiota and the brain was explored, and machine learning models were constructed.
Gut Microbial Diversity and Composition in SZ Patients
There was no statistical difference in the α-diversity between the SZ and HC groups (figure 2A), but the β-diversity indicated a significant separation between the 2 groups (figure 2B). At the phylum level, the top four dominant phyla were consistent across the 2 groups, ie, the Firmicutes, Bacteroidetes, Actinobacteria, and Proteobacteria. Among these, Firmicutes and Bacteroidetes constitute over 75% of the entire microbial community (figure 2C). Relative to HCs, the SZ group exhibits higher relative abundances of Bacteroidetes, Proteobacteria, and Verrucomicrobia, while lower relative abundance of Firmicutes as well as Firmicutes/Bacteroidetes (F/B) ratio (figure 2D). To identify the potential microbial biomarkers between the 2 groups, we performed Lesfe analysis at the genus level. As illustrated in figure 2E, we identified 12 key microbial genera, of which 10 exhibited significantly lower relative abundances and 2 showed significantly higher relative abundance in the SZ group. Specifically, SZ patients exhibited a significant decrease in the relative abundance of many butyrate-producing bacteria (eg, Faecalibacterium, Blautia, Alistipes, Gemmiger, and Butyricicoccus), while a significant increase in the relative abundance of genera such as Proteus and Succinivibrio.
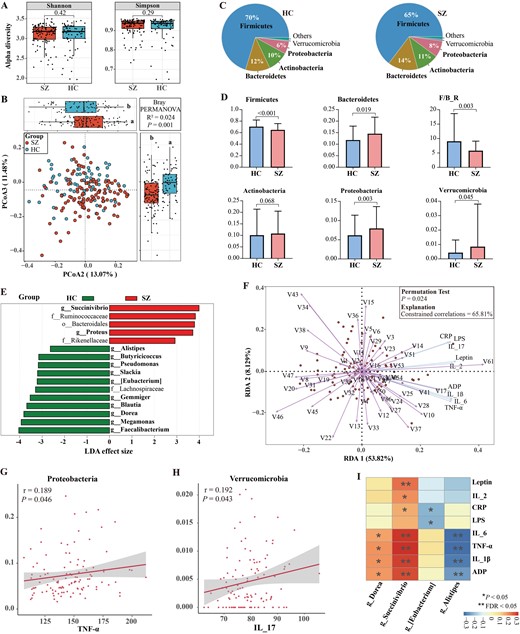
Characterization of gut microbial structure in SZ patients and its relationships with inflammatory cytokines. (A) Box plots showed no significant differences in α-diversity indices (Shannon and Simpson) between the SZ and HC groups. (B) The PCoA of β-diversity based on bacterial distribution at the genus level by Bray–Curtis algorithm showed significant differences in gut taxonomic composition between the SZ and HC groups. (C and D) Comparison of the overall composition and relative abundance of the bacterial community between the 2 groups at the phylum level. (E) 12 key differential microbial genera were confirmed between the 2 groups. Wilcoxon rank sum test, LDA scores >2.0 and P < .05. (F) RDA showed core bacteria at the genus level were strongly associated with inflammatory cytokines. V1-V86 represent the taxonomy ID of genus-level bacteria. (G and H) Spearman correlation analysis of the key microbial taxa at phylum and inflammatory cytokines. (I) Spearman correlation analysis of the key microbial taxa and inflammatory cytokines. The intensity of color represents correlation coefficient, red and blue represent negative and positive correlations, respectively. False discovery rate (FDR) was applied to correct multiple testing.
To comprehensively explore the relationship between the gut microbiota and inflammatory cytokines, we conducted redundancy analysis (RDA), treating the matrix of gut microbial data at genus level as explanatory variables and the matrix of inflammation cytokines as response variables. As depicted in figure 2F, the RDA indicates that the gut microbial data explains 65.81% of the variability in inflammatory cytokines (permutation number = 999, P = .024). In addition, Spearman correlation analysis showed that the phyla Proteobacteria and Verrucomicrobia were positively correlated with TNF-α and IL-17 (r = 0.189, P = .046; r = 0.192, P = .043), respectively (figure 2G–H). Moreover, the butyrate-producing genus Alistipes displayed negative correlations with several proinflammatory cytokines. In contrast, the genus Succinivibrio exhibited positive correlations with multiple proinflammatory cytokines, including IL-1β, IL-2, IL-6, TNF-α, and CRP (P < .05) (figure 2I), indicating that Succinivibrio may represent a potential proinflammatory group.
Characterization of Gut Microbial Function in SZ Patients
Given the possibility of functional redundancy within bacteria,24 we performed KEGG-based PICRUSt analysis for microbial function prediction. As shown in figure 3A, the distinctions between the 2 groups were attributed to a total of 32 differential KEGG pathways. Among them, 22 pathways were significantly up-regulated in the SZ group, while 10 pathways showed a significant down-regulation in the SZ group.
Characterization of gut microbial function in SZ patients. (A) Heatmap of PICRUSt analysis showed significantly different KEGG pathways between the SZ and HC groups. Wilcoxon rank sum test, P < .05. (B) Sankey chart showed interrelationships between the key microbial genus, KEGG pathways, and inflammatory cytokines. Connections colored with red, and blue represent positive and negative correlations, respectively, and were estimated by Spearman correlation coefficient (P < .05). (C) Co-occurrence network of gut bacteria at genus-level, inflammatory cytokines, and KEGG pathway. All bacterial nodes were colored at the phylum level (isolated nodes were excluded), inflammation cytokines, and KEGG pathways were stained uniformly, and edges were estimated by Spearman correlation coefficient (P < .05). Edges colored with red, and blue represent positive and negative correlations, respectively. Signature bacteria were marked with yellow 5-pointed star. The heatmap showed the importance of each node in the network calculated by 3 algorithms, and the transition from red to blue represent the gradual decrease of the node’s importance. V3 Faecalibacterium, V4 Proteus, V10 Succinivibrio, V12 Dorea, V14 Gemmiger, V16 Blautia, V22 [Eubacterium], V23 Megamonas, V36 Pseudomonas, V43 Alistipes, V53 Slackia, V54 Butyricicoccus.
To further investigate whether the key microbial genera impact peripheral inflammation through these differential KEGG pathways, we conducted multi-omics correlation and mediation analyses, as well as co-occurrence network on “gut microbiota, KEGG pathways, and inflammation cytokines.” As illustrated in figure 3B, key microbial genera were significantly associated with 4 KEGG pathways, of which 2 pathways were linked to lipid metabolism, ie, the steroid biosynthesis and the linoleic acid metabolism. Meanwhile, these 4 KEGG pathways were in turn significantly associated with inflammatory cytokines. The results regarding the mediating effects of the four KEGG pathways are shown in Supplementary table S2. The co-occurrence network of gut bacteria, inflammatory cytokines, and KEGG pathway are shown in figure 3C, in which the gut microbiota form extensive and complex associations with both inflammatory cytokines and KEGG pathways, and the key microbial genera such as Faecalibacterium, Blautia, and Proteus, are in the crossover region of the network, suggesting that these bacteria play an important role in the co-occurrence network.
Brain Structural and Functional Changes in SZ Patients
Compared with HCs, the SZ patients exhibited significantly lower GMV in the right hippocampus (HIP), bilateral insula (INS), rectus gyrus (REC), middle cingulate gyrus (DCG), and thalamus (THA) (P < .001, FWE-corrected) (figure 4A and B, Supplementary table S3). In terms of brain function, SZ patients demonstrated lower ReHo in the anterior cingulate gyrus (ACG), postcentral gyrus (PoCG), right precuneus (PCUN), and right inferior occipital gyrus (IOG) compared to the HC group. In contrast, they exhibited higher ReHo in the bilateral caudate nucleus (CAU), right orbitofrontal gyrus (OFG), right superior frontal gyrus (SFG), left inferior temporal gyrus (ITG), and right middle temporal gyrus (MTG) as compared with the HC group (P < .001, FWE-corrected) (figure 4C and D, Supplementary table S4).
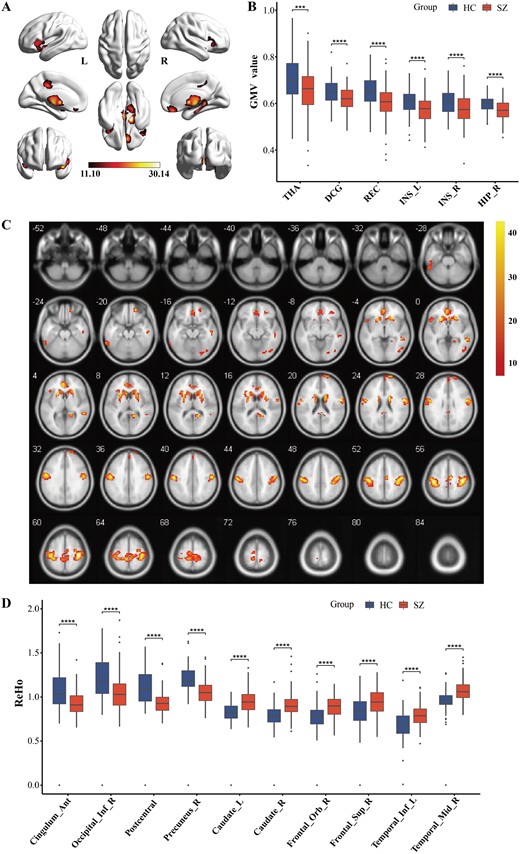
Brain structural and functional changes in SZ patients. (A and B) The whole-brain VBM analysis and post-hoc intergroup comparisons (P < .001, FEW corrected). (C and D) The whole-brain ReHo analysis and post-hoc intergroup comparisons (P < .001, FEW corrected). *P < .05, **P < .01, ***P < .001, ****P < .0001. L, left; R, right; THA, thalamus; DCG, middle cingulate gyrus; REC, gyrus rectus; INS, insula; HIP, hippocampus; caudate, caudate nucleus; frontal_Orb, orbital frontal gyrus; frontal_Sup, superior frontal gyrus; temporal_Inf, inferior temporal gyrus; temporal_Mid, middle temporal gyrus; cingulum_Ant, anterior cingulate gyrus; occipital_Inf, inferior occipital gyrus.
Microbiota–Gut–Brain Crosstalk Centered on Peripheral Inflammation
To gain a better understanding of the microbiota–gut–brain crosstalk, we investigated the relationships between the key microbial genera and changes in brain structure and function (figure 5A). Specifically, the SZ-enriched genus (ie, Proteus) was negatively correlated with brain GMV alterations, while SZ-depleted genera (ie, Butyricicoccus, Blautia, [Eubacterium], Slackia) were positively correlated with brain GMV alteration. In terms of altered brain function, the SZ-enriched genera (ie, Proteus and Succinivibrio) showed significant positive correlations with the enhanced brain ReHo in SZ, while significant negative correlation with the diminished ReHo in SZ. Conversely, the SZ-depleted genera (ie, Blautia, Faecalibacterium, Butyricicoccus, Alistipes, Gemmiger, Dorea, Pseudomonas, and [Eubacterium]) yielded opposite results. Taken together, these findings suggest that changes in brain structure and function may be driven by inflammation derived from the key microbial genera.
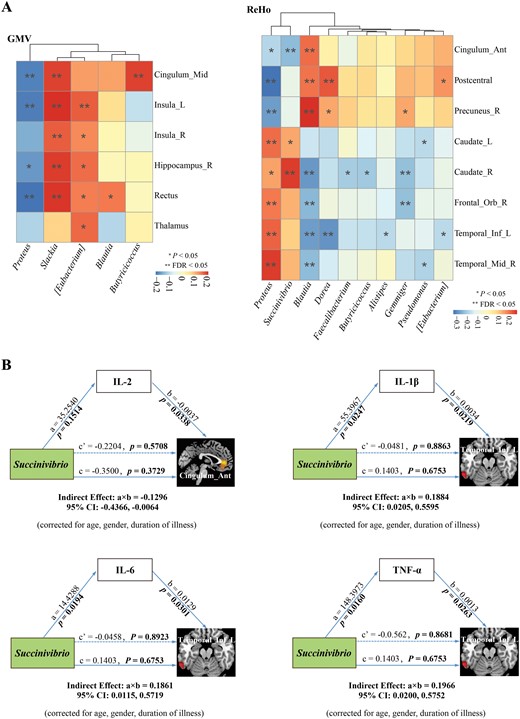
Microbiota–gut–brain crosstalk centered on peripheral inflammation. (A) Spearman correlation analysis of the key microbial genera and changes in brain structure and function. The intensity of color represents correlation coefficient, red and blue represent negative and positive correlations, respectively. False discovery rate (FDR) was applied to correct multiple testing. (B) Mediation analysis testing whether inflammatory cytokines mediate the relationships between key microbial genera and changes in brain structure and function.
To further determine whether the relationships between key microbial genera and neuroimaging features were mediated by inflammatory cytokines, we applied mediation analysis in SZ and revealed 4 mediation linkages. As shown in figure 5B, the genus Succinivibrio may reduce ReHo in the ACG by elevating the level of IL-2 (a × b = −0.1296; 95% CI: −0.4366, −0.0064). In addition, the genus Succinivibrio may enhance ReHo in the left ITG by elevating the levels of IL-1β (a × b = 0.1884; 95% CI: 0.0205, 0.5595), IL-6 (a × b = 0.1861; 95% CI: 0.0115, 0.5719), and TNF-α (a × b = 0.1966; 95% CI: 0.0200, 0.5752), respectively. Hence, certain inflammatory cytokines mediated the effect of higher abundance of Succinivibrio on aberrant activity in ACG and left ITG.
Diagnostic Models Based on Microbial Profiles and Neuroimaging Features
We constructed machine learning models based on genus-level gut microbiota features to explore their efficacy in distinguishing between the SZ and HC groups. As depicted in figure 6A, the machine learning models exhibited excellent performance, with AUC values ranging from 88.7% to 90.9%. Similarly, we separately constructed machine learning models based on brain GMV and ReHo features. The results showed that the AUC values for models based on brain ReHo features ranged from 90.1% to 93.5% (figure 6B), while those based on brain GMV features were approximately 75.2%–80.8% (Supplementary figure S2). To assess the generalization ability of the models, we also evaluated the classification efficacy of the 3 feature models on the validation set data. As shown in Supplementary table S5, the classification model based on gut microbiota features performed comparably to those based on brain ReHo features, with both exhibiting high AUC values, accuracy, specificity, sensitivity, and F1 scores.
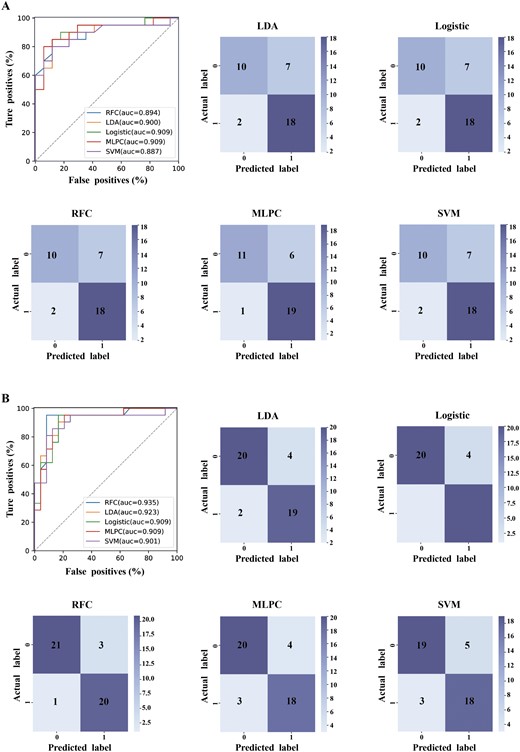
Diagnostic models based on microbial profiles and neuroimaging features. (A) Machine learning models based on gut microbial features at genus-level. (B) Machine learning models based on brain ReHo features. LDA, linear discriminant analysis classifier; RFC, random forest classifier; SVM, support vector machine classifier; MLPC, multi-layer perceptron classifier.
Discussion
The present study identified significant alterations in the gut microbial composition among SZ patients, characterized by a decrease in anti-inflammatory butyrate-producing bacteria and an increase in proinflammatory pathogenic bacteria. Notably, these key microbial taxa were associated with changes in brain structure and function, with gut microbiota-derived inflammation potentially serving as a significant mediating factor. Moreover, the classification model based on gut microbial data showed comparable efficacy to the model based on brain functional signatures in SZ diagnosis.
Gut microbiota plays an important role in maintaining host immune homeostasis. Substantial research has confirmed the role of beneficial bacteria, such as Faecalibacterium,25Butyricicoccus,26Blautia,27 and Alistipes,28 in fermenting indigestible carbohydrates from dietary fiber into SCFAs, and these SCFAs are pivotal in preserving the integrity of the intestinal barrier and suppressing inflammation.6 Conversely, some pathogenic bacteria, notably Gram-negative members like Proteus29 and Succinivibrio, may increase intestinal permeability and facilitate the entry of LPS into the circulatory system, thereby triggering an inflammatory response. Beyond identifying changes in gut microbial composition, the PICRUSt analysis provided additional insights into the potential functions of these key microbial taxa, and tentatively identified the roles of the steroid biosynthesis and linoleic acid metabolism pathways in regulating immune inflammation. Specifically, butyrate-producing genera (Faecalibacterium, Alistipes, and Gemmiger) could reduce levels of proinflammatory cytokines such as IL-1β, IL-6, and TNF-α by down-regulating steroid biosynthesis and linoleic acid metabolic pathways. In contrast, the SZ-enriched bacteria such as Proteus and Succinivibrio could elevated the proinflammatory cytokines by up-regulating the two lipid metabolism pathways. This is consistent with previous findings that disturbances in lipid metabolism could trigger immune-inflammatory responses by activating macrophages, leading to the release of inflammatory cytokines.30,31 Thus, these findings revealed an increase of proinflammatory bacteria, while a reduction of anti-inflammatory bacteria in SZ, which further supports the hypothesis of immuno-inflammation in the etiology of SZ.
The gut and the brain may interact with each other through various pathways. Specifically, the gut microbiota can interact intimately with the gut immune system, thereby affecting neuroimmune.32 In addition, microbial products and metabolites, such as SCFAs, have been shown to influence the homeostasis and function of neurons by reducing blood-brain barrier permeability and diminishing microglia activation,33,34 and also enhance neuroregeneration by modulating levels of brain neurotrophic factors.35,36 Previous studies have reported a significant positive correlation between decreased relative abundance of genus Actinobacillus and family Veillonellaceae in SZ patients and abnormal enlargement of the right middle frontal gyrus.37 Additionally, a reduced relative abundance of genus Roseburia was negatively correlated with the ReHo index in the left cuneus, right middle temporal gyrus, and right superior temporal gyrus.38 In this study, we observed disrupted GMV and ReHo in SZ, and we also identified clear correlations between key microbial genera and these neuroimaging alterations. Specifically, higher abundance of proinflammatory bacteria and lower abundance of anti-inflammatory bacteria were associated with GMV reduction in SZ patients. Similarly, this imbalance between “proinflammatory bacteria” and “anti-inflammatory bacteria” was also correlated with aberrant functional activity in SZ. Hence, our investigation provides initial evidence supporting the connection between gut microbiota and neuroimaging features, with microbiota-derived inflammation potentially serving as a crucial mediator in this association.
Further mediation analysis revealed that certain proinflammatory cytokines mediated the relationships between Succinivibrio and brain functional activity. Succinivibrio, a genus of succinic acid-producing bacteria, plays a crucial role in host health and nutrition. Its classification falls within the family Succinivibrionaceae of the phylum Proteobacteria. An increase in the relative abundance of Succinivibrio has been previously reported in SZ patients,10 consistent with our findings. As a potential producer of short-chain fatty acids such as acetate, Succinivibrio has also been documented to be positively correlated with the level of anti-inflammatory cytokine such as IL-10,39 suggesting its potential anti-inflammatory properties. Interestingly, our results revealed a significant positive correlation between the relative abundance of Succinivibrio and levels of several proinflammatory cytokines such as IL-1β, IL-6, and TNF-α, indicating a potential role of Succinivibrio in fostering inflammation. One possible explanation for this conflicting result is that different species of the genus Succinivibrio may exert differing effects on host health. Hence, further metagenomic sequencing is warranted to verify the species discrepancies within this genus in subsequent research. Moreover, our investigation also highlights the mediating effect of inflammatory cytokines on the brain, focus mainly on the ACG and the left ITG, indicating a potential heightened sensitivity of the two brain regions to inflammatory cytokines. Given that dysfunction of the ACG and ITG have been identified to be strongly associated with the psychotic symptoms40,41 and cognitive impairments42 in SZ patients, these “microbiome-gut-brain” findings may provide a theoretical basis for future interventions targeting the gut microbiome to improve the clinical prognosis of SZ patients.
With the advancement of sequencing technologies, in-depth analysis of the gut microbial profiles provides rich information for the diagnosis, treatment, and prognosis of diseases.43 As a noninvasive detection method, the gut microbiota has achieved significant milestones in diagnosing conditions such as obesity,44 diabetes,45 and colorectal cancer.46 In recent years, the gut microbiota has gained increasing attention in the field of mental health. A cross-sectional study published in 2018 revealed substantial differences in the gut microbial profiles between SZ patients and healthy controls, and that these microbial features were effective in distinguishing between the two groups, with an AUC value of 83.7%.10 Furthermore, distinctive microbial features were identified between SZ patients in acute and remission phases, demonstrating robust discriminatory potential.47 Nevertheless, the existing evidence supporting the value of gut microbial profiles in the diagnosis and prognosis assessment of SZ is insufficient. Few studies have compared classification models based on gut microbiota features with those based on brain imaging features. In our study, the gut microbiota-based classification model achieved an AUC value of up to 90% in distinguishing SZ patients from HCs. Its classification performance was comparable to models based on brain ReHo features and significantly outperformed models based on brain GMV features, further highlighting the great potential of gut microbiota characteristics in SZ diagnosis. Given the high examination cost, long scanning time, and challenges with patient cooperation associated with fMRI, the cost-effective alternative of gut microbiota sequencing may facilitate the future clinical diagnosis and treatment of SZ.
Conclusion
In our study, we identified significant alterations in the gut microbial composition among SZ patients, characterized by a reduction in anti-inflammatory bacteria and an elevation in proinflammatory bacteria. Notably, these key microbial taxa were associated with changes in brain structure and function, with gut microbiota-derived inflammation potentially serving as a significant mediating factor. Moreover, the classification model based on gut microbial data showed comparable efficacy to the model based on brain functional signatures in SZ diagnosis. Taken together, these findings present evidence for the dysregulated microbiota-gut-brain axis in SZ and emphasize the central role of peripheral inflammation. Thus, a more in-depth understanding of the relationship between gut microbiota and inflammation could provide essential therapeutic targets for SZ patients.
Limitations
There are certain limitations in this study. First, this is a cross-sectional study that precluded causal inferences, thus longitudinal design is needed to determine whether the alterations in the gut microbiome occurred before or after the onset of SZ. Second, this study utilized 16s rRNA sequencing, which was insufficient to elaborate the relationships between gut microbiota and brain function at the strain-level. Future studies should provide deeper insight into these relationships via metagenomics and meta-transcriptomics. Third, although we identified several key bacterial genera that may have the potential to affect brain activity, further fundamental studies are still required for confirmation. Finally, the SZ patients in this study mainly consisted of chronic patients taking medications, thus failing to eliminate the confounders of illness chronicity and prolonged exposure to antipsychotics on microbiota–gut–brain axis. Additionally, we did not collect information on factors (eg, diet) that may influence the gut microbial composition. Future studies will benefit from this consideration.
Acknowledgments
We thank all members of the Departments of Psychiatry at the First People’s Hospital of Kashi Prefecture for conducting this study as well as all the participants for their cooperation. We also appreciated the members from Dr. Liwei Xie laboratory at the Institute of Microbiology of Guangdong Academy of Sciences provided long-term support on study design, discussion, and data analysis. These authors declare no conflict of interest.
Author Contributions
Author contributions included conception and study design (H.W., L.X., and X.W.), carrying out the investigation and data collection (H.W., Y.L., Y.H., S.C., Z.Y., and J.L.), statistical analysis (H.W., Y.L., B.L., and L.X.), interpretation of results (H.W., L.X., and X.W.), drafting the manuscript (H.W.), revising the manuscript critically for important intellectual content (L.X. and X.W.) and approval of final version to be published (All authors).
Funding
This work was support by the Guangzhou Daan Gene Co., Ltd, Horizontal Project (Grant No. 2021-397) and Natural Science Foundation of Guangdong Province (Grant No. 2020A1515011288) to Xiaoli Wu, National Natural Science Foundation of China (Grant No. 81900797, 82072436) and Guangdong Basic and Applied Basic Research Foundation (Grant No. 2020B1515020046) to Liwei Xie.
Ethics Approval and Consent to Participate
The Ethics Committee of the First People’s Hospital of Kashi Prefecture approved this study protocol (no. 2021-05). Informed consent was obtained from all participants.
Data Availability
This study has been registered at the China Clinical Trial Registry under approval no. ChiCTR2300070482. The datasets generated by this study are available from the corresponding authors upon reasonable request.