-
PDF
- Split View
-
Views
-
Cite
Cite
Anaïs Louzolo, Alexander V Lebedev, Malin Björnsdotter, Kasim Acar, Christine Ahrends, Morten L Kringelbach, Martin Ingvar, Andreas Olsson, Predrag Petrovic, Resistance to Extinction of Evaluative Fear Conditioning in Delusion Proneness, Schizophrenia Bulletin Open, Volume 3, Issue 1, January 2022, sgac033, https://doi.org/10.1093/schizbullopen/sgac033
- Share Icon Share
Abstract
Delusional beliefs consist of strong priors characterized by resistance to change even when evidence supporting another view is overwhelming. Such bias against disconfirmatory evidence (BADE) has been experimentally demonstrated in patients with psychosis as well as in delusion proneness. In this fMRI-study, we tested for similar resistance to change and associated brain processes in extinction of fear learning, involving a well-described mechanism dependent of evidence updating. A social fear conditioning paradigm was used in which four faces had either been coupled to an unconditioned aversive stimulus (CS+) or not (CS−). For two of the faces, instructions had been given about the fear contingencies (iCS+/iCS−) while for two other faces no such instructions had been given (niCS+/niCS−). Interaction analysis suggested that individuals who score high on delusion-proneness (hDP; n = 20) displayed less extinction of evaluative fear compared to those with low delusion proneness (lDP; n = 23; n = 19 in fMRI-analysis) for non-instructed faces (F = 5.469, P = .024). The resistance to extinction was supported by a difference in extinction related activity between the two groups in medial prefrontal cortex and its connectivity with amygdala, as well as in a cortical network supporting fear processing. For instructed faces no extinction was noted, but there was a larger evaluative fear (F = 5.048, P = 0.03) and an increased functional connectivity between lateral orbitofrontal cortex and fear processing regions for hDP than lDP. Our study links previous explored BADE-effects in delusion associated phenotypes to fear extinction, and suggest that effects of instructions on evaluative fear learning are more pronounced in delusion prone subjects.
Introduction
Psychosis involves an altered experience and understanding of the external world and the self. While simple perceptions are noisy and quickly shifting in an acute psychotic episode, more complex beliefs are often overly stable and resistant to change even when most people regard them as completely unlikely. Such beliefs have been labelled as delusions and regarded as a hallmark of psychotic states observed in different psychiatric disorders as well as in the general population.1–3
Several cognitive models of delusions rest on conditioning4,5 or predictive coding mechanisms.6–10 The hierarchical predictive coding model11,12 suggest that internal models of the world (or the self), i.e. priors, are present at all levels of information processing, involving both low-level priors (in lower levels of the hierarchy) and high-level priors (in higher levels of the hierarchy). Exteroceptive and interoceptive information reaching the brain that cannot be explained by low-level priors will be transmitted upwards in the hierarchy as error signals where higher order priors will try to explain them. The subjective experience is based both on the priors and on the external signals reaching the brain. From a predictive coding perspective, it has been suggested that the balance between the priors and the information reaching the brain is altered in psychosis ,6,9,10 including delusions.7,8,13 It has also been suggested that while low level priors are weak and imprecise, high level priors are unusually strong and precise, mirroring unstable perceptual experiences and delusions, respectively, on a behavioral level.10,14
The reduced ability to revisit one’s beliefs in light of new evidence, the so-called bias against disconfirmatory evidence (BADE), is of a particular relevance for understanding delusions and their pathological stability.15 This cognitive bias has reliably been shown in several psychosis-related conditions and traits,15 including schizophrenia patients,16–20 schizotypal individuals21,22 and healthy subjects scoring high on a delusion-proneness trait.23,24 These studies have suggested that such phenotypes are more resistant to changing their beliefs although new evidence implies that the initial logic no longer holds. Few studies have also characterized the brain processes associated with BADE in psychosis related phenotypes, and shown altered involvement of three networks (i.e. visual attention network, default-mode network and cognitive evaluation network).25,26
Extinction learning of threat responses (or “fear extinction”) is a well-established experimental paradigm to study the mechanisms underlying the update of knowledge in light of new available safety information, i.e. the previous CS+ stimuli which signals that no aversive experience will follow in the extinction phase.27–31 Similar to previous BADE-experiments,15 extinction paradigms present the individual with new information in order to study how the meaning of specific stimuli change.27,29,30 The extinction learning is indexed through the attenuation of conditioned threat responses and/or changes in explicit evaluations.29,32 The mechanisms associated with extinction learning have been well characterized in both animal and human models, and suggests that medial prefrontal cortex (mPFC) suppresses amygdala-dependent learned fear response by strengthening a local inhibitory network acting on the central nucleus.27,28,30,31 As mPFC and amygdala are interacting in this process they may be defined as an extinction network. Also hippocampal formation is involved in fear conditioning, especially contextual fear conditioning33,34 as well as in extinction related processes in humans.28
Evaluative fear conditioning refers to a change in liking of a stimulus after it has been paired with an aversive event.35,36 Fear conditioning tasks assessing evaluative ratings are similar to BADE-tasks as both measure an update of an explicit belief (concept of an individual or concept of a story/picture, respectively). Given that an altered belief update is central in delusions and that extinction involves a well-described neural mechanism, the extinction of evaluative fear conditioning is a particularly attractive model to study formation of overly stable beliefs in psychosis-associated states.
To examine the brain mechanisms underlying a resistance to change of beliefs, we used a well-established fear extinction paradigm, measuring both autonomous fear responses and evaluations as indices of knowledge update, after a classical fear conditioning procedure (non-instructed fear conditioning). On a behavioral level we hypothesized that a similar resistance to change of beliefs in light of new evidence is present for extinction in psychosis-related phenotypes as previously described in BADE.15 Thus, we suggest that a lower degree of extinctions should be present in these groups after non-instructed fear conditioning. We further hypothesized an attenuated activation of an extinction network including mPFC and its interaction with amygdala in psychosis-related phenotypes. Finally, we hypothesized that regions associated with higher order priors such as lateral orbitofrontal cortex (lOFC) should show a differential activation. lOFC may have a specific role in representing higher order priors, as suggested from studies on placebo analgesia,37,38 emotional placebo39 and cognitive reappraisal.40–42
In the present study, we compared delusion prone subjects and controls. Delusion proneness is a personality trait reflecting subclinical delusional ideation tendencies in healthy subjects.43–46 Cognitive, thought- and perceptual mechanisms underlying delusion-proneness are considered to be similar to those underlying delusional ideation in psychosis-spectrum disorders.14,47,48 At the same time, confounding effects related to pharmacological treatment, chronic effect of disorder and comorbidities are smaller than in schizophrenia patients.
Our hypotheses are supported by previous research showing that patients with schizophrenia have a lower extinction recall in terms of autonomic measurements and associated brain activations.49,50 Here, we furthered this finding by focusing on explicit beliefs measured in the form of likability ratings.32,51 We also studied extinction learning instead of extinction recall (that has previously been assessed in schizophrenia49,50) since the learning phase of extinction, but not the recall phase, involves integration of novel evidence with previous priors in a similar way as in BADE-studies.15 Thus, the novelty of this study as compared to previous fMRI-studies on extinction in delusion associated phenotypes49,50 is that it is focused on extinction learning (and not extinction recall), involves explicit ratings (better mirroring beliefs than autonomic measurements) and is performed on healthy subjects with high and low delusion proneness trait rather than psychosis patients (associated with less confounds). The novelty in relation to previous fMRI-studies on the BADE effect is that the present study targets a well-described extinction mechanism.
We also studied the extinction phase following instructed fear conditioning. i.e. fear learning where subjects had received information about the contingencies before the fear conditioning—a form of learning which is highly resistant to extinction.52,53 We hypothesized that instructed fear learning would be more expressed in psychosis related states in line with previous findings14,47 and be associated with involvement of lOfc as in previous studies of delusion proneness14,54 and schizophrenia47 involving suggestions on how to experience an external stimulus.
Methods
Subjects
Participants were recruited through social media and filled in online versions of the included questionnaires (see further Supplementary material). It was stressed twice that they had to be healthy and without any psychiatric history or medication. Upon submission of their contact details and after giving their consent, participants received a link to the questionnaires and an automatically generated unique ID-code that they used when filling in the questionnaires.
We used Peters et al. Delusions Inventory (PDI)45 to assess the level of delusion proneness in 925 screened healthy individuals. PDI is a self-rating questionnaire focusing on delusion associated thoughts and experiences that are existing on a continuum within the general population. We used the 21 item version of PDI that is considered to be a valid instrument to measure delusional ideation in the healthy subjects.45 For each PDI item that is endorsed, three dimensions are rated by the participant on a 5-point Likert scale in order to assess the level of conviction, distress, and preoccupation related to the given item (i.e. conviction, distress, and preoccupation scores, respectively). We recruited subjects with a PDI ≥10 (denoted as subjects with a high delusion proneness; hDP) and subjects with a PDI ≤ 6 and ≥2 (denoted as subjects with a low delusion proneness; lDP). The groups were balanced for ADHD- and autism spectrum disorder traits (see Supplementary material).
Out of the screened individuals, 23 subjects displaying low levels of delusion proneness (lDP; PDI mean = 3.78, SD = 1.38) were compared with 20 subjects displaying high level of delusion proneness (hDP; PDI mean = 12.85, SD = 1.84) in the behavioral part of the extinction phase of the experiment. A total of 19 lDP subjects (PDI mean = 3.89, SD = 1.41) and 20 hDP subjects (PDI mean = 12.85, SD = 1.84) completed the fMRI part of the extinction phase successfully. For more detailed information on subject inclusion see Supplementary material.
All participants in the experimental part of the study gave once again their informed consent before the experiment, and were paid for their participation. The study was approved by the regional ethical board of Stockholm (www.epn.se).
Experimental Design and Procedures
All subjects went through a combined instructed and non-instructed fear conditioning session while brain activity was measured with fMRI. The fear learning paradigm started with an instruction phase that was followed by a fear acquisition phase, and ended with an extinction phase. The conditioned stimuli (CS) consisted of four Caucasian male faces selected from a picture set used in Johansson et al. 201355 displaying a neutral facial expression (2 CS+ and 2 CS−) and randomized between participants. The UCS consisted of a mildly aversive electric stimulation (see further below).
In the instruction phase two of the faces (instructed CS+ and CS−; iCS+/iCS−) were coupled with information about their contingencies with the UCS (including a fabricated short description about their personality and the risk of being associated with a “shock”). The text included information that one of the faces would be associated with a shock (instructed CS+/iCS+) and the other would never be associated with a shock (instructed CS−/iCS−). The two other CS faces (non-instructed CS+ and CS−; niCS+/niCS−) contained no information about their contingencies with the UCS. All faces were shown twice during the instruction phase followed by a rating procedure.
In the fear acquisition phase that was performed during fMRI scanning the subjects underwent a delayed fear conditioning paradigm in which the unconditioned stimulus (UCS) consisted of a mildly aversive electric stimulation. Prior to the start of the experiment a pair of Ag/AgCl electrodes (27 × 36 mm) was attached to participants’ left forearm with electrode gel and used to deliver electrical stimulation. Before lying down in the scanner, participants went through a standard work-up procedure, during which stimulation intensity was gradually increased until participants judged it as unpleasant, but not intolerably painful. Stimulus delivery was controlled by a monopolar DC-pulse electric stimulation (STM200; Biopac Systems Inc. (http://www.biopac.com). Each electrical stimulation lasted for 200 ms, co-terminating the presentation of the reinforced CS+ stimuli. The experiment was presented using Presentation (www.neurobs.com, version 9.13) and was displayed on a screen inside the scanner. Participants controlled the computer cursor through the use of a trackball device. Each CS was displayed 12 times for 5 s, and the jittered inter-trial interval was 11.5 ± 2 s in the acquisition phase. The CS+ was coupled with UCS with a 50% contingency in the acquisition phase. Thus, after acquisition, the total fear learning of instructed stimuli was a combination of top-down and bottom-up level learning while fear learning of non-instructed stimuli was predominantly bottom-up learning, i.e. classical fear conditioning. See Supplementary material for details on methods and hypotheses of the behavioral part of the study. Further information about the instruction phases is given in Louzolo et al. 2019.54
After the fear learning phases the subjects underwent an extinction phase that was also performed during fMRI scanning. As in the fear acquisition phase each CS was displayed 12 times for 5 s, and the jittered inter-trial interval was 11.5 ± 2 s but no stimulus was coupled with the UCS. No information was given that the UCS would not be present. In the present study we focused on extinction phase, while the fear condition phase is presented elsewhere.54
Behavioral Analyses
Since our focus was on explicit learning we used evaluative fear ratings51 as our main outcome. On several occasions throughout the experiment (before instructions, during instructions, before acquisition, before and after extinction) participants had to rate how friendly each CS face was experienced, using a visual analogue scale (VAS) with “the least sympathetic person you can imagine” stated on the left anchor, and “the most sympathetic person you can imagine” on the right anchor (originally in Swedish). The X-axis coordinates of the scale were converted into numbers, from −100 (left anchor) to +100 (right anchor) and used as the rating scores. The first rating of each CS was referred to as the baseline rating (T0) and used to normalize the subsequent ratings for a given CS. The normalized scores were computed for each CS, by subtracting the first ratings from the following ratings. In order to estimate learning in the different phases in our paradigm we calculated the difference between CS− rating and CS+ rating, for each CS pair (instructed and non-instructed). This difference score is referred to as “affective learning index” and represents the main behavioral outcome value in the study as we were interested in explicit learning. Instructions were presented twice (followed by ratings: T1′ and T1) in order to increase explicit learning. Out of these two ratings we used the one following the second instruction presentation (T1) in subsequent analyses as it represented the total effect of the instruction manipulation. This resulted in three affective learning indices: (1) T1—after instruction learning, (2) T2—after acquisition, and (3) T3—after extinction. In order to assess extinction effect of the affective learning we were especially interested in comparing T2 with T3.
For our primary analysis we used mixed linear models to analyze the effect of extinction on the main behavioral outcome variable, i.e. the affective learning index. We analyzed the results for instructed extinction and non-instructed extinction separately (as they had separate hypotheses) and performed a time (T2 vs. T3) × group (hDP vs. lDP) general linear model on repeated measures for each of them. We also performed pair-sampled t-tests within the groups. Analyses were conducted using the software R 3.2.3 (R core team 2015) using packages lme456 and lmerTest.57
Skin conductance response (SCR) was measured to assess fear learning in terms of the autonomic threat response as a complement to the ratings. The SCR measurement and analysis is further described in the Supplementary material.
Functional Imaging
Participants were scanned in a 3T MR General Electric scanner with a 32-channel head coil. Data pre-processing and analyses were performed using a default strategy in the SPM12 software package (Statistical Parametric Mapping, http://www.fil.ion.ucl.ac.uk/spm).
We performed 1st level analyses of extinction effects for instructed stimuli [iCS+ vs. iCS−] and non-instructed stimuli [niCS+ vs. niCS−] for each subject. We then analyzed the main effect of extinction for instructed [iCS+ vs. iCS−] and non-instructed [niCS+ vs. niCS−] stimuli in each group (hDP and lDP) as well as the differences between hDP and lDP in 2nd level analyses. Given our specific a priori hypothesis, we used small-volume correction (SVC) for comparisons within the studied masks in our regions of interest (ROIs) followed by an exploratory whole brain analysis. We also used the average activity of an extended fear-ROI in specific analyses where we expected a general effect on fear processing. We examined effective connectivity using a psychophysiological interaction (PPI) analysis in SPM.58 For the exploratory analysis of dynamic functional connectivity patterns, we used the Leading Eigenvector Dynamics Analysis (LEiDA59). See Supplementary material for further details on hypotheses and methods of the fMRI part of the study. Detailed fMRI analyses for the acquisition phase are presented elsewhere.54
Results
Fear Learning (Acquisition Phase)
A significant and robust explicit fear learning was observed in lDP and hDP for both the instructed and non-instructed stimuli after the acquisition phase using the affective learning index (Supplementary tables 1 and 2). SCR showed a general effect of conditioning and no group differences (Supplementary table 3) in the acquisition phase. The acquisition results of the present study are presented in detail elsewhere.54
Extinction of Non-Instructed Stimuli
Affective Ratings
For lDP the mean affective learning index for non-instructed stimuli was 63.00 (SD = 62.16) before extinction and 51.65 (SD = 56.43) after extinction. For hDP the mean affective learning index for non-instructed stimuli was 89.45 (SD = 81.52) before extinction and 99.35 (SD = 77.16;) after extinction. Thus, when comparing the affective learning index for non-instructed stimuli, before vs. after extinction lDP decreased in evaluative ratings (mean = −11.35; SD = 33.38) while hDP increased in the same ratings (mean = 9.90; SD = 24.81).
In line with our hypothesis, an interaction analysis showed that the extinction effect of learned evaluative fear for non-instructed stimuli was significantly larger for lDP compared to hDP (F = 5.469, P = 0.024), while no significant main effect of group or extinction was present (group: F = 3.204, P = .081, extinction: F = 0.025, P > .05) (figure 1). An explorative analysis of the interaction effect showed a trend towards the expected extinction effect for the lDP (t = 1.63, df = 22, P = .059), while the hDP tended to show an opposite effect, i.e. increased affective learning index after extinction (t = −1.78, df = 19, P = .09).
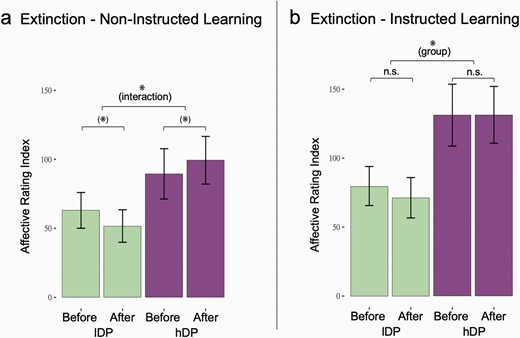
Effects of extinction on affective learning index in lDP and hDP. (a) There was a significant interaction in the extinction effect measured with affective learning index between the groups consisting of expected extinction in lDP and a opposite effect in hDP (F = 5.469, P = .024). (b) While no extinction effects were observed in any of the groups for the instructed stimuli, hDP showed a general higher affective learning index than lDP (F = 5.048, P = 0.03). * = Significant effect. (*) = thershold signifcant effect.
SCR
An interaction was observed for niCS+ between lDP and hDP (time × group interaction effect on SCR (t(df) = −2.12(383), P = .03)) in that a habituation was observed for lDP but not for hDP. No other differences between the groups were observed. See Supplementary material for detailed results of SCR-effects and exploratory analyses that suggested a relation between extinction related SCR and the subjectively rated extinction effects.
fMRI Results
Group Difference in Medial PFC and its Functional Connectivity
Contrast analysis of our fMRI-data mirrored the behavioral findings in that the lDP group showed a larger activation in mPFC during the extinction phase for niCS+ vs. niCS− as compared to the hDP group (figure 2a; Cluster-wise-pfwe < 0.05; MNI coordinates of the peak: −3, 54, 18; Z = 3.42).
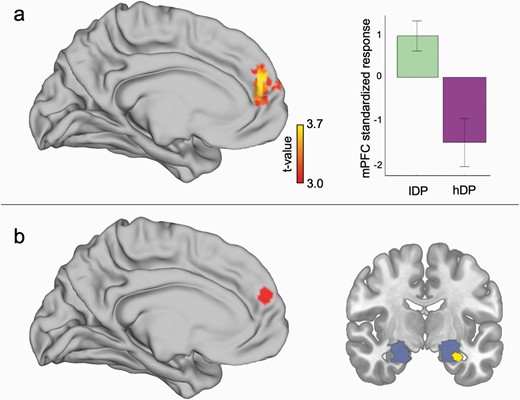
Group difference in brain activations related to extinction effects. (a) There was a significant interaction between the groups during extinction phase in medial prefrontal cortex (mPFC) in that the activation associated with extinction learning was larger for lDP than for hDP. (b) The PPI-analysis suggested that there was a significant difference in the connectivity between mPFC and amygdala between lDP than in hDP. Red area in (b) represents the seed region in mPFC. Blue area represents amygdala ROI. Yellow color indicates the significant connectivity result.
We further probed whether the underlying connectivity between mPFC and anterior medial temporal lobe differed between the groups in a PPI-analysis, as this is a central route of modulation in fear extinction.27,28,30,31 The seed volume of interest (VOI) was defined as mPFC MNI coordinates from the main interaction contrast (see above), and a bilateral amygdala-hippocampal mask, in which SVC was applied, was derived from neurosynth (http://neurosynth.org) using the search term “fear” (see details in Supplementary materials). Our analysis suggested that there was a between-group difference in the PPI-effects, in that there was a stronger negative connectivity between mPFC and anterior medial temporal lobe in lDP as compared to hDP group (XYZ = −30 −6 −24; Z = 3.50; pfwe = 0.042) (figure 2b).
Group Difference in Fear-Related Activity
There was no difference between the groups (t = 0.45; P = .66) in fear related activation for non-instructed stimuli (niCS+ vs. niCS−) when analyzing the extended fear-ROI. However, the t-values for fear related activity (niCS+ vs. niCS−) in the same extended fear-ROI as above were differently related to the level of extinction of evaluative affective ratings in the two groups. We observed an interaction between level of extinction and group (figure 3; F(1,35) = 6.57; P = .015). When we studied each group separately we observed that lDP showed a significant negative relation between behaviorally measured extinction level and fear related (niCS+ vs. niCS−) activation (r = −0.56; P = .01), while hDP did not show any significant relation (r = 0.18; P = 0.45). To better localize the interaction effects, we performed a post-hoc test showing a contribution from caudal anterior cingulate cortex (cACC) (XYZ = −9 15 42; Z = 3.67; pfwe = 0.030; XYZ = 15 −6 63; Z=3.66; pfwe = 0.032) and amygdala/hippocampus on a threshold level (XYZ = −27 −12 −12; Z=3.44; pfwe = 0.054 and XYZ = 30 0 −21; Z = 3.29; pfwe = 0.081) for the same interaction contrast. Ratings of delusion proneness (PDI and its subcomponents) had no effect on extinction related activations.
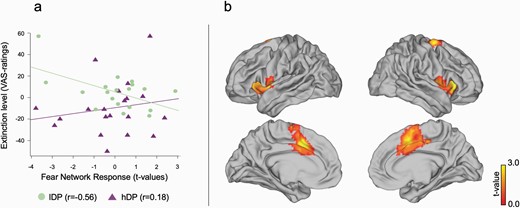
The relation between extinction and fear processing. (a) The degree of extinction in affective learning index (VAS-rating before extinction vs. after extinction) correlated negatively with the t-values of the extended fear network ROI for niCS+ vs. niCS− specifically for lDP as compared to hDP. (b) A post-hoc test suggested that this effect was especially driven by interactions in cACC but contributions were seen throughout the “fear network.”.
A complementary full brain analysis identified interaction effects in the extrastriatal cortex (Supplementary figure 1) in that the hDP group exhibited a pattern of failed suppression of the visual cortex (observed in the lDP) when extinguishing aversive reactions to conditioned stimuli. No other region survived a full brain correction.
Extinction of Instructed Stimuli
Affective Ratings
For lDP the mean affective learning index for instructed stimuli was 79.74 (SD = 67.93) before extinction and 71.26 (SD = 70.26) after extinction. For hDP the mean affective learning index for instructed stimuli was 131.15 (SD = 100.35) before extinction and 131.40 (SD = 92.07) after extinction. When comparing the affective learning index for instructed stimuli, before vs. after extinction lDP decreased in evaluative ratings (mean= −8.48; SD = 39.01) while hDP increased marginally in the same ratings (mean = 0.25; SD = 23.42).
The general linear model analyses found no significant main effect of extinction (F = 0.676, P > .05) in line with previous research on instructed fear learning,52,53 and no interaction between the groups (F = 0.761, P > 0.05)—although the was a difference between the means of the groups. However, there was a significant effect of group (F = 5.048, P = .03) in that the hDP had a larger affective learning index than lDP (figure 1).
SCR
The groups did not significantly differ in the main time-by-CS type effects (full interaction) for the instructed stimuli. See supplementary material for detailed results of SCR-effects.
fMRI Results
In line with the behavioral results, no significant main effect or groups difference was observed for extinction [(iCS+ vs. iCS−)hDP and (iCS+ vs. iCS−)lDP]. However, our PPI-analysis showed a higher functional connectivity in the contrast (iCS+ vs. iCS−) for the hDP group than the lPD group between the left lOFC and cACC (XYZ = −9 12 33; Z = 3.76; pfwe = 0.042) as well as insula (XYZ = −30 15 6; Z = 3.77; pfwe = 0.041), both part of the previously functionally defined fear network (figure 4).
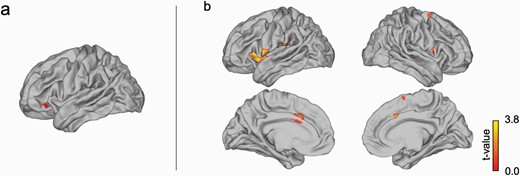
For the instructed fear stimuli, (a) left lOFC showed a stronger functional connectivity in the contrast [(iCS+ vs. iCS−) with (b) caudal ACC and insula as a part of the functionally defined “fear network” for the hDP group than the lPD group.
LEiDA Analysis
We used Leading Eigenvector Dynamics Analysis (LEiDA)59,60; see also Supplementary figures 2 and 3) to estimate dynamic functional connectivity via clusters of phase coherence. Figure 5a shows the resulting phase coherence (PC)-states for the clustering solution of 10 states (k = 10). We then explored relationships between individual switching rate, i.e. the degree to which a participant either switches flexibly between states or tends to remain longer in one state, and delusion proneness. Normalized scores of the three PDI sub-components preoccupation, distress and conviction were used in this analysis as these were normally distributed over both groups. We calculated the normalized scores of the three PDI sub-components by dividing the total points for each subcomponent rating by the number of endorsed items (number of “yes” answers in the PDI”). This analysis showed a positive linear relationships between switching rates and normalized preoccupation scores (in solution of k = 10 shown in figure 5: r = 0.368, p = 0.021—significance correction for multiple comparisons61). No significant group difference was observed between hDP and lDP.
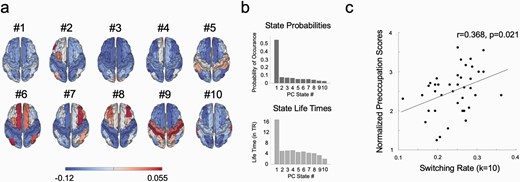
Leading eigenvector analysis (LEiDA) results for clustering solution k = 10. (a) Surface plots of the resulting 10 phase coherence (PC)-states in the Desikan-Killiany 80 (DK80) anatomical parcellation. (b) Average probabilities of occurrence and life times for all 10 states across the time series. Using the LEiDA-method on fMRI-data, a global state (PC-state #1) is always found as most prominent state both in terms of probability of occurrence and life time. (c) The rate at which individuals switch between the 10 states is significantly positively correlated with normalized preoccupation scores.
Given our hypothesis of a relation between higher order emotional priors and lOFC, we analyzed the 10 states within the solution of k = 10 specifically for networks including this region. In that solution (k = 10), but also present in all other different clustering solutions, a left-lateralized network focused around the lOFC emerged (PC-state #2 in the solution for 10 states, see figure 5a and 6a). When comparing probabilities of occurrence of this state between the lDP and hDP groups, we found a significant group difference (permutation tests with 1000 permutations; for probabilities of PC-state #2 in solution for k = 10; t = 0.443, P =.033). Here, the probability of occurrence for this state was larger in the lDP group compared to the hDP group. The group difference in the lOFC state was present across all clustering solutions tested where k > 9 (Supplementary figure 2). The probability of occurrence for this state was not significantly related to the normalized PDI sub-components.
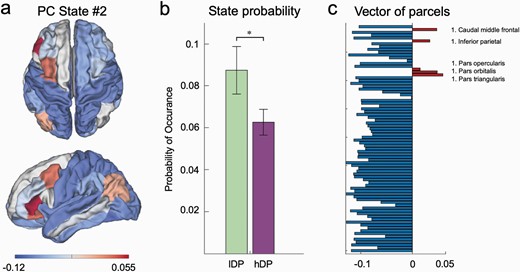
Characteristics of PC State #2 from LEiDA-results for k = 10. (a) Surface plot for the lOFC-network (PC State #2) in DK80 anatomical parcellation. (b) The probability of occurrence of PC State #2 is significantly larger in the lDP group compared to the hDP group. (c) Vector of phase coherence values of all 80 parcels included in the DK80 parcellation.
Discussion
Patients with psychosis and psychosis-related phenotypes often show a strong resistance to change of beliefs, which represents a core aspect of delusional ideation. Here, we show a significant interaction suggesting resistance to change of evaluative fear ratings in delusion-prone individuals (i.e. hDP) after extinction learning. Extinction learning is a well-described process in which the adjustment of an associative value relies on integration of novel evidence,27,28,30,31 thus mimicking bias against disconfirmatory evidence (BADE) models.15,17 Moreover, we show a plausible underlying neuronal mechanism, involving a differential response in the previously described extinction network including mPFC and its interaction with the amygdala-hippocampal complex.27,28,30,31 Also, specifically the control group displaying low delusion proneness (i.e. lDP) showed a decreased activation in functionally defined fear network and amygdala-hippocampal complex related to the degree of extinction. In contrast, hDP individuals did not exhibit such a relationship, confirming altered fear extinction in this group. Finally, we show that the hDP group rates explicitly learned fear (i.e. instructed fear learning) significantly higher than the lDP group and shows a stronger prefrontal functional connectivity with the fear network in the extinction phase of instructed fear learning. Our proof-of-concept study suggests a novel way to understand formations of overly strong beliefs in psychosis-related phenotypes.
Abnormally strong stability of formed beliefs is a core feature of delusions observed in psychosis-related conditions.1–3 One reason is that such individuals often show a bias against disconfirmatory evidence captured elegantly in the BADE-experiments.15,17 The BADE-effect has been described in schizophrenia patients,16–20 but also in individuals with at-risk-mental-states for psychosis,62 in subjects with schizotypy21,22 and in healthy subjects with high delusion proneness.23,24 Here, we took a novel approach by using the extinction paradigm to examine the mechanisms for knowledge update underlying belief formation, indexed by evaluative ratings, in psychosis-associated states. Our main behavioral outcome was affective learning index based on evaluative ratings of the face stimuli, as they are arguably closer to explicit beliefs than autonomic responses. Notably, SCR showed a clear effect of conditioning. Moreover, a significant differential effect of time was shown for niCS+ in SCR between the groups, as only the lDP group exhibited a significant smaller effect of time. Thus, also the automimic response suggested a lower extinction in hDP as compared to lDP for the non-instructed condition.
The differential activity between lDP and hDP individuals in mPFC during extinction-learning mirrors the behavioral difference between the groups in evaluative ratings. While it is clear that mPFC has a pivotal role in fear extinction,27,28,30,31 different sub-regions have been suggested to be mainly involved in this regulatory process. A recent meta-analysis28 suggests the involvement of a similar part of mPFC in extinction learning as where our interaction was observed. This region is more caudal than ventromedial PFC that has been suggested to be involved in extinction recall28 but more rostral than cACC involved in the main effect of fear. The finding is in line with the suggestions that cACC is processing the appraisal and expression of fear, while more ventral ACC and mPFC is involved in emotional regulation.63,64 Our findings of a differential connectivity between mPFC and amygdala-hippocampal complex between the groups also support this view. In sum, we argue that our fMRI result suggests a stronger extinction process in lDP group than in the hDP group mirroring the behavioral results.
It should also be noted that similar regions of mPFC and neighboring ACC have been implicated in psychotic disorders. For example, these regions show a progressive loss of grey matter in high risk subjects that convert to psychosis vs. those who do not65 and are related to positive symptom in schizophrenia.66 Moreover, similar areas have been shown to have a different response pattern in patients with schizophrenia as compared to controls during self-referential processing.67 Thus, it is possible that our results, suggesting a lower activation of mPFC during extinction in hDP as compared to lDP, are related to a general dysfunction of this region in psychosis spectrum states.
In contrast to the non-instructed fear conditioning there were no signs of extinction for the instructed fear conditioning in either group, in line with previous studies showing a strong resistance to any type of extinction for this form of learning.52,53 It has been suggested that instructions have a particularly strong effect on fear learning that involves different computational mechanisms than conditioning.68 Notably, there was a difference between the groups in evaluative affective ratings, suggesting a stronger effect of learning in hDP than in lDP for instructed fear in general. This behavioral finding was supported by an increased functional connectivity in hDP vs. lDP between lOFC and cACC as well as right insula for the contrast iCS+ vs. iCS−, similarly as observed in the learning phase of the same experiment.54 These findings are in line with previous suggestions that psychosis-associated phenotypes tend to rely more on higher hierarchical information processing systems such as orbitofrontal cortex, when interpreting visual stimuli14,47 and assigning self-referential meaning to generic stimuli.67
We also performed an exploratory dynamic functional connectivity analysis using LEiDA on the full data set involving both the instructed and non-instructed stimuli, showing a positive correlation between switching rate amongst different networks and the degree of pre-occupation of delusion-like thoughts. The interpretation of this finding must be seen as preliminary, but the difference in switching rate may point towards instability of brain states, which may cause a noisy general information processing related to how preoccupied an individual is with psychosis-associated thoughts. This is in line with recent hypotheses that have suggested schizophrenia to be a disorder of under-coupling in the brain,69 which would result in faster switches between different brain states. Moreover, the analysis further suggested a lesser occurrence of the state involving the left lOFC in hDP compared to lDP subjects. One interpretation would be that lDP update their higher order priors dependent on lOFC more than hDP during extinction phase. This idea is in line with a lower activation of cognitive evaluative network, including inferior frontal cortex and lateral orbitofrontal cortex, when processing disconformity information during evidence integration in both patients with schizophrenia26 and in relation to subclinical delusional ideation.25
Overall, a complex function of the orbitofrontal cortex seems to emerge, in that it shows a stronger engagement during presentation of belief congruent stimuli14,47,54,67 but a lower involvement during presentation of disconformity stimuli25,26 in delusion associated phenotypes as compared to controls. Our present results are compatible with this model in that lOFC shows a stronger functional connectivity with the fear related network specifically in the instructed fear contrast (iCS+ vs. iCS−) that is belief congruent—but a lower involvement in the extinction phase in general (dominated by stimuli that are disconfirmatory to the initial beliefs) as suggested by the LEiDA. Such a function of lOFC would then mirror how higher order priors guide beliefs in individuals with delusional ideations. On a more general level this idea is in line with involvement of lOFC in the placebo effect.37–39,70,71 Namely, in both the placebo effect and delusions the subjective experience is guided by a change of beliefs due to belief congruent priors. Finally, it has been suggested that task-state representations are dependent on OFC in multidimensional decision making,72 which also points towards the idea that OFC has a role in harnessing higher order priors.
There were several limitations to the current study. Our subjects were not tested with classical BADE-paradigms, and thus the relation between BADE and fear extinction cannot be studied. Another limitation is the moderate sample of included subjects. More subjects would have yielded a stronger power and possibly revealed prefrontal networks that may support difference in higher order priors. However, it should be noted that >900 subjects were screened in order to find the delusion prone subjects and the well-matched controls that were included in the analyses. Moreover, the groups were carefully matched in regards to autism and ADHD-traits that closely related to delusion proneness trait.73 Further, future studies should test whether our findings may be translated to patients with clinical delusions. However, research on delusion proneness in healthy subjects is also a strength since it suffers less from problems with comorbidities, effects of chronic illness on the brain and medical treatments. It is also of interest to study since relates to conspiracy ideas and the belief in alternative facts.74–76
In conclusion, our findings of an attenuated extinction learning in delusion prone subjects are in line with results from classical BADE-studies, in that both approaches show a resistance to change of explicit beliefs in psychosis related phenotypes. We extend this knowledge by identifying that resistance to evaluative fear extinction involves a well-characterized extinction network. Thereby, our study links previous explored BADE-effects in delusion-associated phenotypes to an established brain mechanism.
Acknowledgments
The authors have declared that there are no conflicts of interest in relation to the subject of this study.
Funding
This work was supported by grants from Vetenskapsrådet (2014-30186-113005-19 / 2019-01253), ALF Medicin Funding Region Stockholm (20140306/20160039), Karolinska Institutet (2-70/2014-97; KID-funding 2011/2020), Hjärnfonden (FO2016-0083), and Marianne och Marcus Wallenbergs Stiftelse (MMW2014.0065). CA is funded by the Danish National Research Foundation (DNRF117).
References
Author notes
These authors share the first author position.