-
PDF
- Split View
-
Views
-
Cite
Cite
Teppei Okumura, Masao Hayashi, I-Non Chiu, Yen-Ting Lin, Ken Osato, Bau-Ching Hsieh, Sheng-Chieh Lin, Angular clustering and host halo properties of [O ii] emitters at z > 1 in the Subaru HSC survey, Publications of the Astronomical Society of Japan, Volume 73, Issue 4, August 2021, Pages 1186–1207, https://doi.org/10.1093/pasj/psab068
- Share Icon Share
Abstract
We study the angular correlation function of star-forming galaxies and properties of their host dark matter halos at z > 1 using the Hyper-Suprime Cam (HSC) Subaru Stragetic Program (SSP) survey. We use [O ii] emitters identified using two narrow-band (NB) filters, NB816 and NB921, in the Deep/UltraDeep layers, which respectively cover large angular areas of 16.3 deg2 and 16.9 deg2. Our sample contains 8302 and 9578 [O ii] emitters at z = 1.19 (NB816) and z = 1.47 (NB921), respectively. We detect a strong clustering signal over a wide angular range, |$0{_{.}^{\circ}} 001$| < θ < 1 °, with bias |$b=1.61^{+0.13}_{-0.11}$| (z = 1.19) and |$b=2.09^{+0.17}_{-0.15}$| (z = 1.47). We also find a clear deviation of the correlation from a simple power-law form. To interpret the measured clustering signal, we adopt a halo occupation distribution (HOD) model that is constructed to explain the spatial distribution of galaxies selected by star formation rate. The observed correlation function and number density are simultaneously explained by the best-fitting HOD model. From the constrained HOD model, the average mass of halos hosting the [O ii] emitters is derived to be |$\log {M_{\rm eff}/(h^{-1}\, {M}_{\odot })}=12.70^{+0.09}_{-0.07}$| and |$12.61^{+0.09}_{-0.05}$| at z = 1.19 and 1.47, respectively, which will become halos with the present-day mass M ∼ 1.5 × 1013 h−1 M⊙. The satellite fraction of the [O ii] emitter sample is found to be fsat ∼ 0.15. All these values are consistent with previous studies of similar samples, but we obtain tighter constraints even in a larger parameter space due to the larger sample size from the HSC. The results obtained for host halos of [O ii] emitters in this paper enable the construction of mock galaxy catalogs and the systematic forecast study of cosmological constraints from upcoming emission line galaxy surveys such as the Subaru Prime Focus Spectrograph survey.
1 Introduction
Observation of the large-scale structure of the Universe via the distribution of galaxies in galaxy redshift surveys provides a powerful tool to reveal the nature of dark matter and dark energy (Peebles 1980; Weinberg et al. 2013). As dark energy started to become a dominant energy component toward the current epoch, the Universe experienced a transition from decelerating to accelerating expansion at redshift around unity. It is thus crucial to investigate the large-scale spatial distribution of galaxies over a broad redshift range from z = 0 to z > 1.
The relation between galaxies and their host dark matter halos has been extensively investigated using the prescription of halo occupation distribution (HOD) modeling (Jing et al. 1998; Seljak 2000; Peacock & Smith 2000; Ma & Fry 2000; Scoccimarro et al. 2001; Berlind & Weinberg 2002; Cooray & Sheth 2002). Galaxies selected by the colors, specific star formation rates (sSFR), and stellar masses have been mainly used as tracers of the large-scale structure in wide-angle galaxy redshift surveys. There are a lot of preceding studies of HOD modeling for such populations, and it is known that a simple model proposed by Zheng et al. (2005) can describe the spatial distribution of these galaxies (e.g., Zehavi et al. 2005; Blake et al. 2008; Zheng et al. 2009; Abbas et al. 2010; Zehavi et al. 2011; White et al. 2011; Wake et al. 2011; Coupon et al. 2012; de la Torre et al. 2013; Reid et al. 2014; Guo et al. 2014; Koda et al. 2016; Ishikawa et al. 2020). Most of the work is, however, limited to redshift less than unity because these types of galaxies become harder to target at higher redshifts (Takada et al. 2014; DESI Collaboration 2016): for early/red-type galaxies one needs a long exposure time to get the 4000 Å break and for color-selected galaxies the accuracy of the photometric redshift becomes worse at such redshifts. On the other hand, statistical properties of dark matter halos have been studied using the HOD of high-z quasars and galaxies, i.e., Lyman-break galaxies or Lyman-α emitters at z > 2, for relatively narrow angular regions (Bullock et al. 2002; Moustakas & Somerville 2002; Hamana et al. 2004; Conroy et al. 2006; Richardson et al. 2012; Kayo & Oguri 2012; Durkalec et al. 2015; Harikane et al. 2016, 2018; Ishikawa et al. 2017).
Due to the observational limit of color/sSFR/stellar mass-selected galaxies at higher redshifts, recently the focus of large galaxy surveys has turned to emission line galaxies (ELGs) that can be targeted at z > 1. Such surveys include the Subaru FastSound survey (Tonegawa et al. 2015; Okada et al. 2016), the extended Baryon Oscillation Spectroscopic Survey (eBOSS: Dawson et al. 2016), the Hobby–Eberly Telescope Dark Energy Experiment (HETDEX: Adams et al. 2011), the Subaru Prime Focus Spectrograph (PFS: Takada et al. 2014), the Dark Energy Spectroscopic Instrument (DESI: DESI Collaboration 2016), the Spectro-Photometer for the History of the Universe, Epoch of Reionization, and Ices Explorer (SPHEREx: Doré et al. 2014), and Euclid (Laureijs et al. 2011). However, the statistical properties of the host halos for ELGs are not as simple as those for galaxies selected by color, sSFR, and stellar mass because unlike them there does not necessarily exist an ELG in the center of a halo at the massive end. Geach et al. (2012) proposed a model of HOD for ELGs taking into account the fact that ELGs selected via star formation rates do not necessarily reside in the centers of massive halos. Then they placed a constraint on the HOD parameters for Hα emitters from the Hi-Z Emission Line Survey (HiZELS: Geach et al. 2008). The HOD of the HiZELS sample has been reanalyzed by Cochrane et al. (2017, 2018). Although simple HOD modeling has been performed for the Hα emitters of the FastSound survey at z ∼ 1.4 in Okumura et al. (2016), the sample was so sparse that the clustering signal has been consistent with the HOD of central galaxies only. Kashino et al. (2017) analyzed the Hα-selected galaxies from the FMOS-COSMOS survey at z ∼ 1.6 and constrained the HOD model, but they still adopted the simple model of Zheng et al. (2005). Detailed HOD modeling has been performed by Hong et al. (2019) for Lyα emitters at z ∼ 2.67 selected from the NOAO Deep Wide-Field Survey.
Since [O ii] emitters (λ = 3726, 3729 Å) are one of the main tracers of the large-scale structure employed in upcoming cosmological surveys, it is of crucial importance to investigate the properties of the halos which host them. So far, however, there have been few observational studies of the HOD modeling for [O ii] emitters. From the HiZELS survey Khostovan et al. (2018) measured the clustering of Hβ + [O iii] as well as [O ii] emitters, but they found no deviation of the correlation function from a power-law form. Rather than using HOD, properties of [O ii] emitters have been investigated using a semi-analytic model of galaxy formation (e.g., Contreras et al. 2013; Gonzalez-Perez et al. 2018, 2020; Favole et al. 2020; Avila et al. 2020). Guo et al. (2019) analyzed the clustering of spectroscopically identified [O ii] emitting galaxies at 0.7 < z < 1.2 from the eBOSS survey. They then investigated the properties of the host halos using the conditional stellar mass function. Recently there have also been attempts to determine the HOD of ELGs by directly identifying emission lines in cosmological hydrodynamical simulations (Hadzhiyska et al. 2021; K. Osato & T. Okumura in preparation). Properties of the luminosity function of [O ii] and other ELGs are also being actively investigated (e.g., Comparat et al. 2016; Saito et al. 2020; Hayashi et al. 2020; Gao & Jing 2021).
In this paper we present detailed HOD modeling for [O ii] emitters identified by narrow-band (NB) filters in the Subaru Hyper-Suprime Cam (HSC) survey. Narrow-band imaging surveys of ELGs allow us to cover a wide and homogeneous field of view, providing a suitable sample to probe the large-scale spatial distribution of emission line galaxies. We, for the first time, constrain the general HOD model proposed by Geach et al. (2012) for populations of [O ii] emitters at z = 1.19 and z = 1.47 in the HSC survey. We then discuss the properties of dark matter halos which host the [O ii] emitters based on the constrained HOD parameters.
The structure of this paper is as follows. Section 2 describes the HSC survey and the [O ii] emitter sample. Section 3 presents the measurements of the angular correlation function and its covariance matrix. Power-law and linearly biased dark matter model fittings are shown in section 4. We also discuss dependencies of the correlation function amplitude on different stellar mass and emission line luminosity thresholds. Detailed HOD modeling is performed for the measured correlation functions in section 5 and conclusions are given in section 6. We compare the angular correlation functions measured from four individual fields in appendix 1. We present the HOD parameter constraints without using information about the measured abundance of [O ii] emitters in appendix 2.
Throughout this paper we assume a flat ΛCDM cosmology with cosmological parameters based on the results of Planck CMB measurements (Planck Collaboration et al. 2016): |$\Omega _m=1-\Omega _\Lambda = 0.307$|, Ωb = 0.0486, h = 0.677, ns = 0.967, and σ8 = 0.816.
2 Data
2.1 Hyper-Suprime Cam Survey
The HSC Subaru Strategic Program (SSP) is an ongoing imaging survey running since 2014 (Aihara et al. 2018a) with a 1.77 deg2 field-of-view imaging camera installed on the Subaru Telescope (Miyazaki et al. 2012, 2018; Furusawa et al. 2018; Komiyama et al. 2018).
In the HSC survey the deep imaging is conducted in five broad-band (BB) filters as well as four narrow-band (NB) filters in the Deep (D) and UltraDeep (UD) layers over 28 deg2 in total. The imaging reduction and catalog construction were carried out by Bosch et al. (2018) and Huang et al. (2018). In 2019, the second public data release (PDR2) of the HSC SSP data was made (Aihara et al. 2019).
2.2 Narrow-band filters and [O ii] emitter sample
We use the data from two NB filters, NB816 and NB921, constructed by Hayashi et al. (2020) based on the D and UD layers of Subaru-SSP from PDR2. These data are significantly updated from the PDR1 version (Aihara et al. 2018b; Hayashi et al. 2018): the coverage of NB816 and NB921 increased to 26 deg2 covered by 13 pointings and 28 deg2 covered by 14 pointings, respectively. We apply bright star masks modified from Coupon et al. (2018) and Aihara et al. (2019) because Hayashi et al. (2020) noticed that the mask size for some bright stars is not large enough to remove false detections around prominent stellar halos. As a result, the total area is reduced to 16.3 deg2 (NB816) and 16.9 deg2 (NB921), as shown in table 1.
. | NB816 (1.178 < z < 1.208) . | NB921 (1.453 < z < 1.489) . | ||||||
---|---|---|---|---|---|---|---|---|
Field . | Area (deg2) . | Ng . | ng (h3 Mpc−3) . | Nsub . | Area (deg2) . | Ng . | ng (h3 Mpc−3) . | Nsub . |
COSMOS | 1.37 | 566 | 4.48 × 10−3 | 9 | 5.80 | 3134 | 4.30 × 10−3 | 41 |
DEEP2-3 | 4.98 | 2485 | 5.40 × 10−3 | 35 | 4.92 | 2107 | 3.41 × 10−3 | 35 |
ELAIS-N1 | 4.81 | 2927 | 6.59 × 10−3 | 34 | 4.81 | 3098 | 5.13 × 10−3 | 34 |
SXDS+XMM-LSS | 5.17 | 2324 | 4.86 × 10−3 | 37 | 1.33 | 1239 | 7.44 × 10−3 | 9 |
Total | 16.33 | 8302 | 5.50 × 10−3 | 115 | 16.86 | 9578 | 4.53 × 10−3 | 119 |
. | NB816 (1.178 < z < 1.208) . | NB921 (1.453 < z < 1.489) . | ||||||
---|---|---|---|---|---|---|---|---|
Field . | Area (deg2) . | Ng . | ng (h3 Mpc−3) . | Nsub . | Area (deg2) . | Ng . | ng (h3 Mpc−3) . | Nsub . |
COSMOS | 1.37 | 566 | 4.48 × 10−3 | 9 | 5.80 | 3134 | 4.30 × 10−3 | 41 |
DEEP2-3 | 4.98 | 2485 | 5.40 × 10−3 | 35 | 4.92 | 2107 | 3.41 × 10−3 | 35 |
ELAIS-N1 | 4.81 | 2927 | 6.59 × 10−3 | 34 | 4.81 | 3098 | 5.13 × 10−3 | 34 |
SXDS+XMM-LSS | 5.17 | 2324 | 4.86 × 10−3 | 37 | 1.33 | 1239 | 7.44 × 10−3 | 9 |
Total | 16.33 | 8302 | 5.50 × 10−3 | 115 | 16.86 | 9578 | 4.53 × 10−3 | 119 |
The area value is after masking with bright object masks, Ng is the number of galaxies used in this paper after making the magnitude and flux cuts, ng is the number densities of the galaxies, and Nsub is the number of sub-regions using jackknife resampling (see subsection 3.2). The last row shows the values for the sum of the four fields.
. | NB816 (1.178 < z < 1.208) . | NB921 (1.453 < z < 1.489) . | ||||||
---|---|---|---|---|---|---|---|---|
Field . | Area (deg2) . | Ng . | ng (h3 Mpc−3) . | Nsub . | Area (deg2) . | Ng . | ng (h3 Mpc−3) . | Nsub . |
COSMOS | 1.37 | 566 | 4.48 × 10−3 | 9 | 5.80 | 3134 | 4.30 × 10−3 | 41 |
DEEP2-3 | 4.98 | 2485 | 5.40 × 10−3 | 35 | 4.92 | 2107 | 3.41 × 10−3 | 35 |
ELAIS-N1 | 4.81 | 2927 | 6.59 × 10−3 | 34 | 4.81 | 3098 | 5.13 × 10−3 | 34 |
SXDS+XMM-LSS | 5.17 | 2324 | 4.86 × 10−3 | 37 | 1.33 | 1239 | 7.44 × 10−3 | 9 |
Total | 16.33 | 8302 | 5.50 × 10−3 | 115 | 16.86 | 9578 | 4.53 × 10−3 | 119 |
. | NB816 (1.178 < z < 1.208) . | NB921 (1.453 < z < 1.489) . | ||||||
---|---|---|---|---|---|---|---|---|
Field . | Area (deg2) . | Ng . | ng (h3 Mpc−3) . | Nsub . | Area (deg2) . | Ng . | ng (h3 Mpc−3) . | Nsub . |
COSMOS | 1.37 | 566 | 4.48 × 10−3 | 9 | 5.80 | 3134 | 4.30 × 10−3 | 41 |
DEEP2-3 | 4.98 | 2485 | 5.40 × 10−3 | 35 | 4.92 | 2107 | 3.41 × 10−3 | 35 |
ELAIS-N1 | 4.81 | 2927 | 6.59 × 10−3 | 34 | 4.81 | 3098 | 5.13 × 10−3 | 34 |
SXDS+XMM-LSS | 5.17 | 2324 | 4.86 × 10−3 | 37 | 1.33 | 1239 | 7.44 × 10−3 | 9 |
Total | 16.33 | 8302 | 5.50 × 10−3 | 115 | 16.86 | 9578 | 4.53 × 10−3 | 119 |
The area value is after masking with bright object masks, Ng is the number of galaxies used in this paper after making the magnitude and flux cuts, ng is the number densities of the galaxies, and Nsub is the number of sub-regions using jackknife resampling (see subsection 3.2). The last row shows the values for the sum of the four fields.
The survey area in the D layer, where the NB data are available, consists of four separate fields: E-COSMOS, DEEP2-3, ELAIS-N1, and XMM-LSS. Furthermore, each of the E-COSMOS and XMM-LSS fields encompasses the UD layer covered by a single pointing of HSC, named UD-COSMOS and SXDS, respectively. Since UD-COSMOS and E-COSMOS are jointly processed, we call the combination the COSMOS field.
Complete descriptions of the [O ii] emitter sample used in this study are provided in Hayashi et al. (2018, 2020). While we briefly summarize our sample in the following, we refer the reader to these papers for more details.
The ELGs are selected using the NB data together with data from the five BB filters. [O ii] emitters are observed by NB816 and NB921 filters in two narrow redshift ranges, 1.178 < z < 1.208 and 1.453 < z < 1.489, respectively. To identify the [O ii] emitters, we use spectroscopic redshifts if available and photometric redshifts otherwise. If a galaxy has a spectroscopic redshift outside the above ranges, we remove it as a contaminant. When the photometric redshift is used, we have taken into account its uncertainty for the identification of [O ii] emitters. For galaxies not identified with spectroscopic or photometric redshifts, we use color–color diagrams to distinguish [O ii] emitters from other possibilities. Galaxies selected as NB [O ii] emitters are expected to be star-forming galaxies (Ly et al. 2012; Hayashi et al. 2013). Thus, our sample is limited by star formation rate (SFR). While our [O ii] emission lines are affected by dust extinction, the majority of typical star-forming galaxies are properly selected because the cosmic SFR density in our catalogs is consistent with previous studies (Hayashi et al. 2020).
We make magnitude and flux cuts on our data: the model magnitude1 m ≤ 23.5 and the estimated line flux ≥3 × 10−17 erg s−1 cm−2. We refer the reader to Hayashi et al. (2020) for details of the line flux estimation. The limiting magnitude of the NB filters is much deeper than 23.5 [mag] so that fainter [O ii] emitters have been included in our sample, and accordingly [O ii] emitters which have a line flux of (1–2) × 10−17 erg s−1 cm−2 have been selected. However, we have made such conservative magnitude and flux cuts to assure the completeness of the sample. With these ranges, the number density indeed increases monotonically with decreasing flux or increasing magnitude (see Hayashi et al. 2018, 2020) and the mean completeness of our [O ii] sample becomes 0.974 for NB816 and 0.956 for NB921. The numbers of [O ii] emitters are then reduced to 8302 and 9578 for the NB816 and NB921 data, respectively. See table 1 for the sample numbers for each field. The line flux cut corresponds to observed line luminosity thresholds of |$L \ge 2.56\times 10^{41} \, \rm {[erg\, s^{-1}]}$| (z = 1.19) and |$L \ge 4.30 \times 10^{41} \, \rm {[erg \, s^{-1}]}$| (z = 1.47). The median luminosity with the 2.5th–97.5th percentiles is |$\bar{L} = 4.49^{+12.67}_{-1.82} \times 10^{41} \, \rm {[erg \, s^{-1}]}$| at z = 1.19 and |$\bar{L} = 7.88^{+16.95}_{-3.35} \times 10^{41} \, \rm {[erg \, s^{-1}]}$| at z = 1.47. Stellar masses for the [O ii] emitters are estimated by spectral energy distribution fit with five HSC BB data in Hayashi et al. (2020). The median stellar mass of our [O ii] emitter sample with the 2.5th–97.5th percentiles is |$\bar{M}_* = 8.58^{+132.54}_{-7.13}\times 10^{9}\, {M}_{\odot }$| at z = 1.19 and |$\bar{M}_* = 1.72^{+12.40}_{-1.41}\times 10^{10}\, {M}_{\odot }$| at z = 1.47.
Not all the galaxies in the catalog are real [O ii] emitters; some are fake lines due to noise and some others are real emission lines but not [O ii] emitters. The contamination rate is investigated by applying photo-z and color selections to galaxies confirmed with spectroscopic redshifts. The fraction of such non-[O ii] emitters has been significantly reduced in the current sample compared to that in the PDR1 catalog because (i) the method of selecting emission line galaxies has been improved from using the cmodel magnitude to the fixed aperture magnitude (Hayashi et al. 2020), and (ii) the pipeline used for the data processing, hscPipe, has been upgraded in PDR2 (Bosch et al. 2018; Aihara et al. 2019). The analysis by Hayashi et al. (2020) estimated the fraction of non-[O ii] emitters in our sample at less than |$10\%$| for most cases and ∼20% at most, by applying photometric redshift and color selections to galaxies confirmed with spectroscopic redshifts. This contaminant fraction can be further reduced by a machine-learning-based technique for photometric redshift estimates (see, e.g., Hsieh & Yee 2014). However, since the current contaminant fraction is low enough for our analysis, we do not include this process. The angular distribution of the [O ii] emitters is shown in figure 1. Table 1 summarizes the properties of the data.
![Angular distribution of [O ii] emitters at z = 1.19 (top) and z = 1.47 (bottom) with line flux greater than 3 × 10−17 erg s−1 cm−2 and magnitude brighter than 23.5 mag in the NB816 and NB921 filters, respectively, for the four individual fields. The large empty areas where no galaxy is found are mostly the regions masked for bright objects. See figure 4 of Hayashi et al. (2020) for details of the mask information. (Color online)](https://oup.silverchair-cdn.com/oup/backfile/Content_public/Journal/pasj/73/4/10.1093_pasj_psab068/2/m_psab068fig1.jpeg?Expires=1749450935&Signature=u-CvxLom1Gg52U~itMM9~v~8Y6jyGRh2L0g3BhqYEB3Gp0VcySWZqVeus8k6Mb1pAqmm6ZQbQKHPsgdO0f5shsRXDBDpjGv5My2OQQcEe7MYQOAI9GEyLM9JOyRhB2sA-CrUaOXDEy2WEfJMZmMnYe09ImIhIYjcjXP5b0xv-lpuVieonXQbNE4ju40Gw2vmzHUvMmGxL1ShPyqdJ1Oh8THITlaWI30GIIYgmdhVOqDyDRZZHAItXY7N7cdWCeqbVlAAJwGq29CeEwRy3g3ipEyTE9PlxG6e9gaexZ-DU5WUQfsKklbsGasU3b3zu3HLXXnFP9eRISrBlIxSHbq75g__&Key-Pair-Id=APKAIE5G5CRDK6RD3PGA)
Angular distribution of [O ii] emitters at z = 1.19 (top) and z = 1.47 (bottom) with line flux greater than 3 × 10−17 erg s−1 cm−2 and magnitude brighter than 23.5 mag in the NB816 and NB921 filters, respectively, for the four individual fields. The large empty areas where no galaxy is found are mostly the regions masked for bright objects. See figure 4 of Hayashi et al. (2020) for details of the mask information. (Color online)
2.3 Random catalog
HSC-SSP PDR2 provides us with a catalog of random points across the survey area as one of the value-added products (see subsection 5.4 of Aihara et al. 2019). The number density of the random points is 100 arcmin−2. We apply the same basic flags as those for the emission line galaxies except for the flags related to source detection (i.e., merge_peak, sdssshape_flag, cmodel, and psfflux) to select the random points (see, sub-subsection 2.1.1 of Hayashi et al. 2020). We also apply the same bright star masks used for the selection of the emission line galaxies to the random points (see Hayashi et al. 2020 for the details of the masks; sub-subsection 6.6.2 of Aihara et al. 2019; Coupon et al. 2018). Consequently, the random points used in this study are distributed over regions identical to where the emission line galaxies are surveyed.
3 Angular correlation function
3.1 Correlation function estimation
We first measure the angular correlation function of [O ii] emitters at z = 1.19 and z = 1.47 from the four fields COSMOS, DEEP2-3, ELAIS-N1, and SXDS + XMM-LSS. We then combine the four correlation functions at each redshift for the statistical analysis.
The measured correlation function combined over the four fields, |$\hat{w}$|, is shown as the red points in the upper and lower panels of figure 2 for z = 1.19 and z = 1.47, respectively. The correlation function becomes negative at scales θ ∼ 1° (r ∼ 50 h−1 Mpc), due to the integral constraint |$w_\Omega$|. For the model parameter fitting below, this effect is properly taken into account. In this figure we show various lines which are the predictions based on the power-law model and the linearly biased dark matter model. We discuss these in detail in section 4. The measurements of the angular correlation functions for individual fields are presented for a consistency check in appendix 1.
![Angular correlation functions of [O ii] emitters measured from all four fields at z = 1.19 (top) and z = 1.47 (bottom). Note that the vertical axis mixes logarithmic and linear scalings. The red points are measurements without the integral constraint correction, $\hat{w}$. The error bars are estimated from jackknife resampling. The blue solid curve is the best-fitting model of the power-law model, $(1-f_{\rm fake})^2[w(\theta ;A_w,\beta )-w_\Omega (A_w,\beta )]$, with (Aw, β, ffake) = (1.10, 0.534, 0.140) for z = 1.19 and (1.17,0.538,0.139) for z = 1.47. The data enclosed by the two blue vertical lines are used for this analysis. The red solid curve is the best-fitting model of the linearly biased dark matter model, $(1-f_{\rm fake})^2[b^2w_{\rm m}(\theta )-w_\Omega (b)[$, with (b, ffake) = (1.60, 0.140) for z = 1.19 and (2.08,0.140) for z = 1.47 when the data enclosed by the two red vertical lines are used. The red dashed curve is the same model but without subtracting the integral constraint denoted by IC in the legend, (1 − ffake)2b2wm(θ). The non-linear dark matter correlation function, wm(θ), is shown by the red dashed curve as a reference. (Color online)](https://oup.silverchair-cdn.com/oup/backfile/Content_public/Journal/pasj/73/4/10.1093_pasj_psab068/2/m_psab068fig2.jpeg?Expires=1749450935&Signature=Dc1FWdxerFK~vAd4JEkeR792Pr8COs3Ijn4VD2gbnPVj6M5Mp4iHFYMNb1QXuwwHvMhIqBwjFFi1eIxGpcv2~DSdNAnRev8SwCwYjZ4SUM8FLivpuxbChR-w2DfWCAfw04a~zPj-38cZErCjq-nacv8qHDe7DHJ8jmHqY-totsyKruP95b~hiR8Q0uXamBYAv~B2pjKEvfxG1WGkBTLj~hXijQHHRdrf8h0ClLZeeDyIl8jNN7WUoDGuQ8cuCGpkihifoPGIaesRq~mGU~bdjDgNWmm~XpW2kTSk0B1nzV87ycNKjf-GPQoEuz4Bw9122cDGQYP7fgh4n9BQiZfRiQ__&Key-Pair-Id=APKAIE5G5CRDK6RD3PGA)
Angular correlation functions of [O ii] emitters measured from all four fields at z = 1.19 (top) and z = 1.47 (bottom). Note that the vertical axis mixes logarithmic and linear scalings. The red points are measurements without the integral constraint correction, |$\hat{w}$|. The error bars are estimated from jackknife resampling. The blue solid curve is the best-fitting model of the power-law model, |$(1-f_{\rm fake})^2[w(\theta ;A_w,\beta )-w_\Omega (A_w,\beta )]$|, with (Aw, β, ffake) = (1.10, 0.534, 0.140) for z = 1.19 and (1.17,0.538,0.139) for z = 1.47. The data enclosed by the two blue vertical lines are used for this analysis. The red solid curve is the best-fitting model of the linearly biased dark matter model, |$(1-f_{\rm fake})^2[b^2w_{\rm m}(\theta )-w_\Omega (b)[$|, with (b, ffake) = (1.60, 0.140) for z = 1.19 and (2.08,0.140) for z = 1.47 when the data enclosed by the two red vertical lines are used. The red dashed curve is the same model but without subtracting the integral constraint denoted by IC in the legend, (1 − ffake)2b2wm(θ). The non-linear dark matter correlation function, wm(θ), is shown by the red dashed curve as a reference. (Color online)
3.2 Covariance matrix
4 Results
4.1 Setup
In this section we consider two simple models for the angular correlation function, the power-law and linearly biased dark matter models in subsections 4.2 and 4.3, to model the clustering amplitude of [O ii] emitters. The HOD modeling is performed in section 5.
As discussed in subsection 2.2, not all the lines detected as [O ii] emitters are actually [O ii] lines, but some are other emission lines or just noise. The fraction of fake [O ii] lines is denoted as ffake. Then, the observed correlation function has contributions not only from the auto-correlation of [O ii] emitters but also from the auto-correlation of fake lines and their cross-correlation, which are suppressed by factors of |$f_{\rm fake}^2$| and 2ffake(1 − ffake), respectively; see, e.g., van den Bosch et al. (2013) and Okumura et al. (2015, 2017) for detailed theoretical schemes for the tracer decomposition. The contamination fraction is estimated to be around ffake ∼ 0.14 by applying photometric redshift and color selections to galaxies confirmed with spectroscopic redshifts (Hayashi et al. 2020). Thus, even though the non-[O ii] emitters have a non-negligible auto-correlation, the (intrinsically small) clustering amplitude is suppressed by 0.142 ∼ 0.02. We can therefore safely assume that the observed correlation is dominated by the auto-correlation of [O ii] emitters (for a similar treatment, see, e.g., Okumura et al. 2016; Kashino et al. 2017).4 The amplitude of the correlation function is thus simply scaled by a factor of (1 − ffake)2. We treat ffake as a free parameter with a prior of ffake = 0.140 ± 0.060 to cover the uncertainties described in subsection 2.2 within the 1 σ confidence level. We chose this uncertainty to be very conservative and to avoid inducing biased constraints on model parameters by imposing strong (and possibly incorrect) priors. The imposed prior is coincidentally equivalent to that adopted in Kashino et al. (2017).
In order to perform a maximum likelihood analysis we use the Markov chain Monte Carlo (MCMC) sampler emcee (Foreman-Mackey et al. 2013).
4.2 Power-law constraints
Figure 3 shows the joint constraints on the power-law parameters and the fake line fraction, (Aw, β, ffake), with blue and orange contours for z = 1.19 and z = 1.47, respectively.6 The parameter set with the minimum value of χ2 for z = 1.19 is (Aw, β, ffake) = (1.10, 0.534, 0.140), while that for z = 1.47 is (Aw, β, ffake) = (1.17, 0.538, 0.139). With these sets of the best-fitting parameters we derive the integral constraints as |$w_\Omega = 0.110$| and |$w_\Omega = 0.114$|, for lower and higher redshifts, respectively. The best-fitting power-law model prediction for the measured correlation function, |$(1-f_{\rm fake})^2(A_w\theta ^{-\beta }-w_\Omega )$|, is shown by the blue line in figure 2. The constrained parameters are shown in table 2.
![Constraints on the power-law model parameters (Aw, β) and the fake line fraction parameter ffake from the angular clustering of [O ii] emitters for z = 1.19 (blue) and z = 1.47 (orange). The contours show the $68\%$ and $95\%$ confidence levels. The diagonal panel shows the posterior probability distribution of each parameter. A Gaussian prior is adopted for the fake line fraction, ffake = 0.140 ± 0.060, which is shown as the black curve compared with the one-dimensional posterior. The posterior of ffake for z = 1.47 (orange) is almost entirely behind that for z = 1.19. (Color online)](https://oup.silverchair-cdn.com/oup/backfile/Content_public/Journal/pasj/73/4/10.1093_pasj_psab068/2/m_psab068fig3.jpeg?Expires=1749450935&Signature=RHm-TiqY2a9~N8onIyoLKDJuNZ6F3F1QNbB6kDNPJbo4riOy7bVFSnVMYmMkZFm3Jz59-DQQl4UWMzCBooO-LMZqn9UmnZcTSHcShcgtvqHMVldl9ipTBp3akQ4YoUUOYRj8o-7lrse0ITendT43VhdaXxY6EtcYjNgjC1h9FRq-zQdFViN-aPg5Li9ADU2l39ltdL5Nj82O57HSmP6UmYiBrs~c2O2MGQLAnOHT4kGdvgDdzyHaj1cvvxUx233l~eiQmW1d0LOOADQas0~ctLSp~WFdYz6S2uG7e~IYZtMM~LYcRhs6WjOBtFl8bcoZBixNDHBMCMPw2m~~a0vO7Q__&Key-Pair-Id=APKAIE5G5CRDK6RD3PGA)
Constraints on the power-law model parameters (Aw, β) and the fake line fraction parameter ffake from the angular clustering of [O ii] emitters for z = 1.19 (blue) and z = 1.47 (orange). The contours show the |$68\%$| and |$95\%$| confidence levels. The diagonal panel shows the posterior probability distribution of each parameter. A Gaussian prior is adopted for the fake line fraction, ffake = 0.140 ± 0.060, which is shown as the black curve compared with the one-dimensional posterior. The posterior of ffake for z = 1.47 (orange) is almost entirely behind that for z = 1.19. (Color online)
Parameter constraints on single power-law and linearly biased dark matter models.*
. | . | NB816 . | NB921 . | ||
---|---|---|---|---|---|
. | Prior . | Best fit . | Posterior PDF . | Best fit . | Posterior PDF . |
Power-law parameter | |||||
Aw (at 1′) | None | 1.10 | |$1.12^{ + 0.21} _{- 0.16}$| | 1.17 | |$1.20^{ + 0.20}_{ - 0.17}$| |
β | None | 0.534 | |$0.533 ^{+ 0.057}_{ - 0.055}$| | 0.538 | |$0.537^{+ 0.044}_{ - 0.043}$| |
ffake | 0.140 ± 0.060 | 0.140 | |$0.149^{ + 0.059}_{ - 0.059}$| | 0.139 | |$0.149^{ + 0.060}_{ - 0.059}$| |
r0(h−1 Mpc) | — | 4.08 | |$4.12^{ + 0.50}_{ - 0.41}$| | 4.55 | |$4.61^{+ 0.51}_{- 0.45}$| |
Linearly biased DM parameter | |||||
b | None | 1.60 | |$1.61 ^{+ 0.13}_{ - 0.11}$| | 2.08 | |$2.09^{ + 0.17}_{ - 0.15}$| |
ffake | 0.140 ± 0.060 | 0.140 | |$0.145^{ + 0.059}_{ - 0.059}$| | 0.140 | |$0.145^{ + 0.060}_{ - 0.059}$| |
. | . | NB816 . | NB921 . | ||
---|---|---|---|---|---|
. | Prior . | Best fit . | Posterior PDF . | Best fit . | Posterior PDF . |
Power-law parameter | |||||
Aw (at 1′) | None | 1.10 | |$1.12^{ + 0.21} _{- 0.16}$| | 1.17 | |$1.20^{ + 0.20}_{ - 0.17}$| |
β | None | 0.534 | |$0.533 ^{+ 0.057}_{ - 0.055}$| | 0.538 | |$0.537^{+ 0.044}_{ - 0.043}$| |
ffake | 0.140 ± 0.060 | 0.140 | |$0.149^{ + 0.059}_{ - 0.059}$| | 0.139 | |$0.149^{ + 0.060}_{ - 0.059}$| |
r0(h−1 Mpc) | — | 4.08 | |$4.12^{ + 0.50}_{ - 0.41}$| | 4.55 | |$4.61^{+ 0.51}_{- 0.45}$| |
Linearly biased DM parameter | |||||
b | None | 1.60 | |$1.61 ^{+ 0.13}_{ - 0.11}$| | 2.08 | |$2.09^{ + 0.17}_{ - 0.15}$| |
ffake | 0.140 ± 0.060 | 0.140 | |$0.145^{ + 0.059}_{ - 0.059}$| | 0.140 | |$0.145^{ + 0.060}_{ - 0.059}$| |
The values in the “Prior” column quote the mean and standard deviation of the Gaussian priors. The “Best fit” column shows the parameter set which gives the minimum value of χ2. In the “Posterior PDF” column the central value is a median and the error means the 16th–84th percentiles after the other parameters are marginalized over.
Parameter constraints on single power-law and linearly biased dark matter models.*
. | . | NB816 . | NB921 . | ||
---|---|---|---|---|---|
. | Prior . | Best fit . | Posterior PDF . | Best fit . | Posterior PDF . |
Power-law parameter | |||||
Aw (at 1′) | None | 1.10 | |$1.12^{ + 0.21} _{- 0.16}$| | 1.17 | |$1.20^{ + 0.20}_{ - 0.17}$| |
β | None | 0.534 | |$0.533 ^{+ 0.057}_{ - 0.055}$| | 0.538 | |$0.537^{+ 0.044}_{ - 0.043}$| |
ffake | 0.140 ± 0.060 | 0.140 | |$0.149^{ + 0.059}_{ - 0.059}$| | 0.139 | |$0.149^{ + 0.060}_{ - 0.059}$| |
r0(h−1 Mpc) | — | 4.08 | |$4.12^{ + 0.50}_{ - 0.41}$| | 4.55 | |$4.61^{+ 0.51}_{- 0.45}$| |
Linearly biased DM parameter | |||||
b | None | 1.60 | |$1.61 ^{+ 0.13}_{ - 0.11}$| | 2.08 | |$2.09^{ + 0.17}_{ - 0.15}$| |
ffake | 0.140 ± 0.060 | 0.140 | |$0.145^{ + 0.059}_{ - 0.059}$| | 0.140 | |$0.145^{ + 0.060}_{ - 0.059}$| |
. | . | NB816 . | NB921 . | ||
---|---|---|---|---|---|
. | Prior . | Best fit . | Posterior PDF . | Best fit . | Posterior PDF . |
Power-law parameter | |||||
Aw (at 1′) | None | 1.10 | |$1.12^{ + 0.21} _{- 0.16}$| | 1.17 | |$1.20^{ + 0.20}_{ - 0.17}$| |
β | None | 0.534 | |$0.533 ^{+ 0.057}_{ - 0.055}$| | 0.538 | |$0.537^{+ 0.044}_{ - 0.043}$| |
ffake | 0.140 ± 0.060 | 0.140 | |$0.149^{ + 0.059}_{ - 0.059}$| | 0.139 | |$0.149^{ + 0.060}_{ - 0.059}$| |
r0(h−1 Mpc) | — | 4.08 | |$4.12^{ + 0.50}_{ - 0.41}$| | 4.55 | |$4.61^{+ 0.51}_{- 0.45}$| |
Linearly biased DM parameter | |||||
b | None | 1.60 | |$1.61 ^{+ 0.13}_{ - 0.11}$| | 2.08 | |$2.09^{ + 0.17}_{ - 0.15}$| |
ffake | 0.140 ± 0.060 | 0.140 | |$0.145^{ + 0.059}_{ - 0.059}$| | 0.140 | |$0.145^{ + 0.060}_{ - 0.059}$| |
The values in the “Prior” column quote the mean and standard deviation of the Gaussian priors. The “Best fit” column shows the parameter set which gives the minimum value of χ2. In the “Posterior PDF” column the central value is a median and the error means the 16th–84th percentiles after the other parameters are marginalized over.
4.3 Linearly biased dark matter model
The joint constraints on (b, ffake) are shown in figure 4. We find the best-fitting value of the bias parameter, |$b= 1.61 ^{+ 0.13}_{ - 0.11}$| and |$b= 2.09^{ + 0.17}_{ - 0.15}$|, respectively for the lower- and higher-redshift measurements. These bias values can be compared to the effective bias beff to be determined by HOD modeling [equation (27)]. The integral constraint is calculated by these best-fitting parameter sets for the correlation in the combined field as |$w_\Omega = 0.0270$| and |$w_\Omega = 0.0277$| for z = 1.19 and 1.47, respectively.
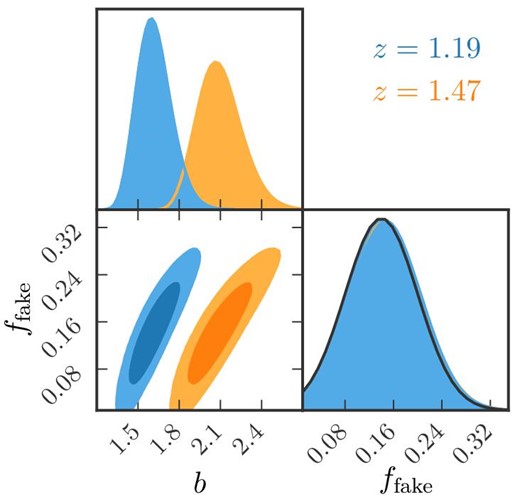
Constraints on the linear galaxy bias b of the non-linear dark matter model and the fake line fraction parameter ffake for z = 1.19 (blue) and z = 1.47 (orange). The contours show the |$68\%$| and |$95\%$| confidence levels. The diagonal panels show the posterior probability distribution of each parameter. A Gaussian prior is adopted for the fake line fraction, ffake = 0.140 ± 0.060, which is shown as the black curve compared with the one-dimensional posterior. The posterior of ffake for z = 1.47 (orange) is almost entirely behind that for z = 1.19. (Color online)
The best-fitting linearly biased dark matter model, |$(1-f_{\rm fake})^2[b^2w_{\rm m}(\theta )-w_\Omega ]$|, is shown as the red solid curve in figure 2. To see the contribution of the integral constraint, we show the same model without the |$w_\Omega$| term, (1 − ffake)2b2wm(θ), as the red dashed curve. As expected, the model correlation function is positive over all the scales studied here. As a reference, the dark matter correlation function, wm, is shown by the black dashed curve in figure 2.
In the next subsection we further analyze the [O ii] emitter samples with different luminosity and stellar mass thresholds using the linearly biased DM model.
4.4 Line luminosity and stellar mass dependencies of [O ii]-emitter clustering
It is interesting to see how the amplitude of a measured correlation function depends on the properties of the [O ii] emitters. Here we consider two basic properties, the luminosity of the [O ii] emission line and the stellar mass of the host galaxies, and split our sample into subsamples based on these thresholds.
Figure 5 shows the angular correlation functions measured with different line luminosity cuts. For clarity, we add the integral constraint correction so that all the correlation functions become positive at all scales probed. For each correlation function, we perform a model fitting using the linearly biased dark matter model considered in subsection 4.3. The best-fitting model for each measurement, (1 − ffake)2b2wm(θ), is presented by the dashed curve. As discussed in subsection 4.3, this simple model fails to explain the measured correlation function at small scales. To see the dependence of the clustering amplitude on the luminosity, we constrain the parameter |$\tilde{b}$| in equation (19) as a function of the minimum line luminosity Lmin. The result is shown in the left panel of figure 6. For each redshift, we find that the bias b increases with increasing line luminosity, by ∼10%. If the fake line fraction, ffake, changed significantly with the luminosity, this luminosity dependence would be modulated.
![Angular correlation functions of [O ii] emitters at z = 1.19 (top) and z = 1.47 (bottom) with different peak luminosity cuts. The unit of L in the legend is [erg s−1]. For visualization purposes, the integral constraint is taken into account, and thus the vertical axis represents $\hat{w}+(1-f_{\rm fake})^2w_\Omega$. The amplitudes of the correlation functions shown by the blue, green, yellow, brown, and purple points are multiplied by 2n, where n = 1, 2, …, 5, respectively. The dashed curves are the best-fitting models of the linearly biased dark matter model, (1 − ffake)2b2wm(θ). (Color online)](https://oup.silverchair-cdn.com/oup/backfile/Content_public/Journal/pasj/73/4/10.1093_pasj_psab068/2/m_psab068fig5.jpeg?Expires=1749450935&Signature=kiW33HramsR9XAPDTuKnKUt-0RHLfKevjh8~yaO2CgdDCYgfbTKPutjaUxbDVuijnY5suOhICDOuTljG2udH7mMI9hSpJHdY~8W~ArNTbmU1hLfV4dO5ixnzVMh7EexTo~0Sptc7TGwJjeZXMuMUC~EvBAy9D4btg1Df3s0Qc0ya2V0wpfP1SHAmBMBDBZxJO5XtLJLGkcy35eizGK8HRA6RtrnWvITZCvfG2ZUSX9Bq1gmKxPzWDarG7~3P2kcPgckn9rJ8yj-T6JO8ZC6j7KgUOu5cbsV7UP2F320tCmAYRmXKJXzxkkkhporI44qMAqAilP6x5XJwBmwLfJib4Q__&Key-Pair-Id=APKAIE5G5CRDK6RD3PGA)
Angular correlation functions of [O ii] emitters at z = 1.19 (top) and z = 1.47 (bottom) with different peak luminosity cuts. The unit of L in the legend is [erg s−1]. For visualization purposes, the integral constraint is taken into account, and thus the vertical axis represents |$\hat{w}+(1-f_{\rm fake})^2w_\Omega$|. The amplitudes of the correlation functions shown by the blue, green, yellow, brown, and purple points are multiplied by 2n, where n = 1, 2, …, 5, respectively. The dashed curves are the best-fitting models of the linearly biased dark matter model, (1 − ffake)2b2wm(θ). (Color online)
![Constraints on the measured clustering amplitude of [O ii] emitters, $\tilde{b}=(1-f_{\rm fake})b$, as a function of the minimum peak luminosity Lmin (left) and stellar mass M*, min (right). The blue and orange points are the results at z = 1.19 and z = 1.47, respectively. (Color online)](https://oup.silverchair-cdn.com/oup/backfile/Content_public/Journal/pasj/73/4/10.1093_pasj_psab068/2/m_psab068fig6.jpeg?Expires=1749450935&Signature=hTMqvHNsZ8uRH4WIh5bkTJv2PiIdNglCe8TbGJpVs6mPrFv1CUFHca4AxF~BSWyKv3hJLAGB68bY7OM68Nzg2PQ0i5KQnamMhH8I75vwxULUGgFu-Cf1W8qwvihIwgqmINh~SupNSethwbUNpwd9VMJvac7aT5K5azpC0OD0WJvLQxznBcckVU712qiLqw~587M0qUye3eDD-Bb6Oo2m9tVfWtDTF5ZT7hMfER-woltzjSkDp0h5Ag7LHR5sngd7PBb6G5PSIybgQEJLuXUNdSUzus6KzQhnQVoisyecUw~KjXZNss44zh~IENAJBP-c4ZukX5aI1K7nwnY8w1L0MA__&Key-Pair-Id=APKAIE5G5CRDK6RD3PGA)
Constraints on the measured clustering amplitude of [O ii] emitters, |$\tilde{b}=(1-f_{\rm fake})b$|, as a function of the minimum peak luminosity Lmin (left) and stellar mass M*, min (right). The blue and orange points are the results at z = 1.19 and z = 1.47, respectively. (Color online)
We repeat the same analysis by splitting our [O ii] emitter sample into subsamples with stellar mass cuts M*, min. We measure the angular correlation function of [O ii] emitters with stellar mass M* ≥ M*, min. Since the fitting result for w(θ) is similar to that with the luminosity cuts in figure 5, we do not plot the figure. We constrain the measured clustering amplitude |$\tilde{b}$| as a function of M*, min, as shown in the right panel of figure 6. A trend similar to the line luminosity limited samples is found: [O ii] emitters with higher stellar masses cluster more strongly than those with lower masses. Almost no mass dependence is seen in the clustering at z = 1.47. As discussed in subsection 3.6 of Hayashi et al. (2018), multi-band data at longer wavelengths, such as near-infrared data, would be required to accurately estimate the stellar masses at z ∼ 1.5. Thus, our result for the stellar mass dependence of the clustering at z = 1.47 needs to be interpreted with caution. Overall, however, the dependence of the clustering of [O ii] emitters on stellar masses is not as strong as on line luminosities, which is qualitatively consistent with the result of Khostovan et al. (2018).
5 Dark matter–[O ii] emitter connections
Halo occupation distribution modeling is an empirical and parametric way to describe the connection between galaxies and their host halos through modeling the abundance and clustering of galaxies (Jing et al. 1998; Peacock & Smith 2000; Seljak 2000; Ma & Fry 2000; Scoccimarro et al. 2001; Cooray & Sheth 2002; Berlind & Weinberg 2002; Zheng et al. 2005; van den Bosch et al. 2013; Hikage et al. 2013; Okumura et al. 2015). In this section we use HOD modeling to investigate physical properties of halos which host [O ii] emitters.
5.1 Formalism of halo model
5.2 Halo occupation distribution model for [O ii] emitters
We fix the least important parameter, δlog M, to δlog M = 1. Thus, the number of free parameters in the Geach HOD model together with the fake line fraction is eight: |$\Theta = (M_{\rm c}, M_{\rm min}, \sigma _{\log {M}}, \alpha , F_c^A, F_c^B, F_{\rm s}, f_{\rm fake})$|. The parameter α is known to have a value around unity. We thus apply a Gaussian prior on α as α = 1.00 ± 0.20. As with the analysis in section 4, we further impose a Gaussian prior on the fake line fraction parameter as ffake = 0.140 ± 0.060. Uniform priors are applied for the other six parameters. Table 3 summarizes the priors on all eight parameters. We use the Python package HALOMOD (Murray et al. 2013) to calculate the HOD model prediction for the angular correlation function.
. | . | NB816 . | NB921 . | ||
---|---|---|---|---|---|
. | . | Best fit . | Posterior PDF . | Best fit . | Posterior PDF . |
Parameter | Prior | ||||
log Mc/(h−1 M⊙) | None | 11.75 | |$12.04^{+0.57}_{-0.32}$| | 11.93 | |$11.91^{+ 0.19} _{- 0.18}$| |
log Mmin/(h−1 M⊙) | None | 12.46 | |$12.61^{+0.32}_{-0.43}$| | 12.47 | |$12.57^{+0.29}_{-0.35}$| |
σlog M | [0,1] | 0.06 | |$0.40^{+0.28}_{-0.31}$| | 0.13 | |$0.17^{+ 0.15}_{- 0.10}$| |
α | 1.00 ± 0.20 | 1.06 | |$1.03^{+0.12}_{-0.13}$| | 1.23 | |$1.12^{+ 0.13}_{- 0.12}$| |
|$F_c^A$| | [0, 0.5] | 0.13 | |$0.26^{+0.16}_{-0.12}$| | 0.14 | |$0.26^{+ 0.15}_{- 0.13}$| |
|$F_c^B$| | [0,1] | 0.95 | |$0.37^{+0.39}_{-0.28}$| | 0.90 | |$0.53^{+ 0.32}_{- 0.34}$| |
Fs | [0, 1] | 0.98 | |$0.54^{+0.31}_{-0.34}$| | 0.73 | |$0.55^{+ 0.31}_{- 0.33}$| |
ffake | 0.140 ± 0.060 | 0.172 | |$0.140^{+0.048}_{-0.053}$| | 0.128 | |$0.104^{+ 0.043}_{- 0.041}$| |
χ2/ν (ν = 7) | 2.17 | 0.856 | |||
Inferred quantity | Measurement | ||||
log ng/(h−1 Mpc)−3 | −2.259 ± 0.068 (NB816) | −2.313 | |$-2.316^{+0.071}_{-0.073}$| | −2.401 | |$-2.381^{+0.074}_{-0.073}$| |
−2.344 ± 0.070 (NB921) | |||||
fsat | — | 0.308 | |$0.159^{+0.120}_{-0.047}$| | 0.242 | |$0.159^{ + 0.109}_{ - 0.049}$| |
beff | — | 1.797 | |$1.700^{+0.084}_{-0.111}$| | 2.029 | |$1.981^{+0.072}_{-0.068}$| |
log Meff/(h−1 M⊙) | — | 12.831 | |$12.703^{+0.093}_{-0.071}$| | 12.699 | |$12.609^{+0.085}_{-0.051}$| |
. | . | NB816 . | NB921 . | ||
---|---|---|---|---|---|
. | . | Best fit . | Posterior PDF . | Best fit . | Posterior PDF . |
Parameter | Prior | ||||
log Mc/(h−1 M⊙) | None | 11.75 | |$12.04^{+0.57}_{-0.32}$| | 11.93 | |$11.91^{+ 0.19} _{- 0.18}$| |
log Mmin/(h−1 M⊙) | None | 12.46 | |$12.61^{+0.32}_{-0.43}$| | 12.47 | |$12.57^{+0.29}_{-0.35}$| |
σlog M | [0,1] | 0.06 | |$0.40^{+0.28}_{-0.31}$| | 0.13 | |$0.17^{+ 0.15}_{- 0.10}$| |
α | 1.00 ± 0.20 | 1.06 | |$1.03^{+0.12}_{-0.13}$| | 1.23 | |$1.12^{+ 0.13}_{- 0.12}$| |
|$F_c^A$| | [0, 0.5] | 0.13 | |$0.26^{+0.16}_{-0.12}$| | 0.14 | |$0.26^{+ 0.15}_{- 0.13}$| |
|$F_c^B$| | [0,1] | 0.95 | |$0.37^{+0.39}_{-0.28}$| | 0.90 | |$0.53^{+ 0.32}_{- 0.34}$| |
Fs | [0, 1] | 0.98 | |$0.54^{+0.31}_{-0.34}$| | 0.73 | |$0.55^{+ 0.31}_{- 0.33}$| |
ffake | 0.140 ± 0.060 | 0.172 | |$0.140^{+0.048}_{-0.053}$| | 0.128 | |$0.104^{+ 0.043}_{- 0.041}$| |
χ2/ν (ν = 7) | 2.17 | 0.856 | |||
Inferred quantity | Measurement | ||||
log ng/(h−1 Mpc)−3 | −2.259 ± 0.068 (NB816) | −2.313 | |$-2.316^{+0.071}_{-0.073}$| | −2.401 | |$-2.381^{+0.074}_{-0.073}$| |
−2.344 ± 0.070 (NB921) | |||||
fsat | — | 0.308 | |$0.159^{+0.120}_{-0.047}$| | 0.242 | |$0.159^{ + 0.109}_{ - 0.049}$| |
beff | — | 1.797 | |$1.700^{+0.084}_{-0.111}$| | 2.029 | |$1.981^{+0.072}_{-0.068}$| |
log Meff/(h−1 M⊙) | — | 12.831 | |$12.703^{+0.093}_{-0.071}$| | 12.699 | |$12.609^{+0.085}_{-0.051}$| |
In the “Prior” column the ranges specified in brackets are for uniform priors while for the others we quote the mean and standard deviation of the Gaussian priors. The “Best fit” column shows the parameter set which gives the minimum value of χ2. In the “Posterior PDF” column the central value is a median and the error means the 16th–84th percentiles after the other parameters are marginalized over. The measured number density log ng includes non-[O ii] emitters, and thus its best-fitting values differ from the measure ones by log (1 − ffake) ∼ −0.06.
. | . | NB816 . | NB921 . | ||
---|---|---|---|---|---|
. | . | Best fit . | Posterior PDF . | Best fit . | Posterior PDF . |
Parameter | Prior | ||||
log Mc/(h−1 M⊙) | None | 11.75 | |$12.04^{+0.57}_{-0.32}$| | 11.93 | |$11.91^{+ 0.19} _{- 0.18}$| |
log Mmin/(h−1 M⊙) | None | 12.46 | |$12.61^{+0.32}_{-0.43}$| | 12.47 | |$12.57^{+0.29}_{-0.35}$| |
σlog M | [0,1] | 0.06 | |$0.40^{+0.28}_{-0.31}$| | 0.13 | |$0.17^{+ 0.15}_{- 0.10}$| |
α | 1.00 ± 0.20 | 1.06 | |$1.03^{+0.12}_{-0.13}$| | 1.23 | |$1.12^{+ 0.13}_{- 0.12}$| |
|$F_c^A$| | [0, 0.5] | 0.13 | |$0.26^{+0.16}_{-0.12}$| | 0.14 | |$0.26^{+ 0.15}_{- 0.13}$| |
|$F_c^B$| | [0,1] | 0.95 | |$0.37^{+0.39}_{-0.28}$| | 0.90 | |$0.53^{+ 0.32}_{- 0.34}$| |
Fs | [0, 1] | 0.98 | |$0.54^{+0.31}_{-0.34}$| | 0.73 | |$0.55^{+ 0.31}_{- 0.33}$| |
ffake | 0.140 ± 0.060 | 0.172 | |$0.140^{+0.048}_{-0.053}$| | 0.128 | |$0.104^{+ 0.043}_{- 0.041}$| |
χ2/ν (ν = 7) | 2.17 | 0.856 | |||
Inferred quantity | Measurement | ||||
log ng/(h−1 Mpc)−3 | −2.259 ± 0.068 (NB816) | −2.313 | |$-2.316^{+0.071}_{-0.073}$| | −2.401 | |$-2.381^{+0.074}_{-0.073}$| |
−2.344 ± 0.070 (NB921) | |||||
fsat | — | 0.308 | |$0.159^{+0.120}_{-0.047}$| | 0.242 | |$0.159^{ + 0.109}_{ - 0.049}$| |
beff | — | 1.797 | |$1.700^{+0.084}_{-0.111}$| | 2.029 | |$1.981^{+0.072}_{-0.068}$| |
log Meff/(h−1 M⊙) | — | 12.831 | |$12.703^{+0.093}_{-0.071}$| | 12.699 | |$12.609^{+0.085}_{-0.051}$| |
. | . | NB816 . | NB921 . | ||
---|---|---|---|---|---|
. | . | Best fit . | Posterior PDF . | Best fit . | Posterior PDF . |
Parameter | Prior | ||||
log Mc/(h−1 M⊙) | None | 11.75 | |$12.04^{+0.57}_{-0.32}$| | 11.93 | |$11.91^{+ 0.19} _{- 0.18}$| |
log Mmin/(h−1 M⊙) | None | 12.46 | |$12.61^{+0.32}_{-0.43}$| | 12.47 | |$12.57^{+0.29}_{-0.35}$| |
σlog M | [0,1] | 0.06 | |$0.40^{+0.28}_{-0.31}$| | 0.13 | |$0.17^{+ 0.15}_{- 0.10}$| |
α | 1.00 ± 0.20 | 1.06 | |$1.03^{+0.12}_{-0.13}$| | 1.23 | |$1.12^{+ 0.13}_{- 0.12}$| |
|$F_c^A$| | [0, 0.5] | 0.13 | |$0.26^{+0.16}_{-0.12}$| | 0.14 | |$0.26^{+ 0.15}_{- 0.13}$| |
|$F_c^B$| | [0,1] | 0.95 | |$0.37^{+0.39}_{-0.28}$| | 0.90 | |$0.53^{+ 0.32}_{- 0.34}$| |
Fs | [0, 1] | 0.98 | |$0.54^{+0.31}_{-0.34}$| | 0.73 | |$0.55^{+ 0.31}_{- 0.33}$| |
ffake | 0.140 ± 0.060 | 0.172 | |$0.140^{+0.048}_{-0.053}$| | 0.128 | |$0.104^{+ 0.043}_{- 0.041}$| |
χ2/ν (ν = 7) | 2.17 | 0.856 | |||
Inferred quantity | Measurement | ||||
log ng/(h−1 Mpc)−3 | −2.259 ± 0.068 (NB816) | −2.313 | |$-2.316^{+0.071}_{-0.073}$| | −2.401 | |$-2.381^{+0.074}_{-0.073}$| |
−2.344 ± 0.070 (NB921) | |||||
fsat | — | 0.308 | |$0.159^{+0.120}_{-0.047}$| | 0.242 | |$0.159^{ + 0.109}_{ - 0.049}$| |
beff | — | 1.797 | |$1.700^{+0.084}_{-0.111}$| | 2.029 | |$1.981^{+0.072}_{-0.068}$| |
log Meff/(h−1 M⊙) | — | 12.831 | |$12.703^{+0.093}_{-0.071}$| | 12.699 | |$12.609^{+0.085}_{-0.051}$| |
In the “Prior” column the ranges specified in brackets are for uniform priors while for the others we quote the mean and standard deviation of the Gaussian priors. The “Best fit” column shows the parameter set which gives the minimum value of χ2. In the “Posterior PDF” column the central value is a median and the error means the 16th–84th percentiles after the other parameters are marginalized over. The measured number density log ng includes non-[O ii] emitters, and thus its best-fitting values differ from the measure ones by log (1 − ffake) ∼ −0.06.
5.3 HOD parameter constraints
The resulting constraints on the HOD parameters of the Geach model are shown in figure 7. This and the following two figures are the main results of this paper. The eight-dimensional posterior distributions are visualized in two-dimensional contours with the other six parameters marginalized over. The top row of each column shows the one-dimensional posterior. The constraints are summarized in table 3. The normalization parameters, |$F_c^{A,B}$| and Fs, are poorly constrained, which is expected and consistent with Geach et al. (2012) and Hong et al. (2019). Overall, constraints from the z = 1.47 sample are tighter than those from the z = 1.19 one. In particular, the marginalized distribution for σlog M for the z = 1.19 sample is much wider and even becomes bimodal. Interestingly, this bimodality was seen by Hong et al. (2019) who analyzed the clustering of Lyα emitters using the Geach HOD model. Although there are many free parameters with few priors, we obtain meaningful constraints due to our large sample. Particularly strong constrains are obtained for the two mass parameters, Mc and Mmin, as seen in table 3, because Mc can be determined by the observed number density, ng, and Mmin primarily degenerates with Mc. We do not see a strong evolution of HOD from z = 1.47 to 1.19, which is consistent with our ongoing work based on cosmological hydrodynamical simulations (K. Osato & T. Okumura in preparation).
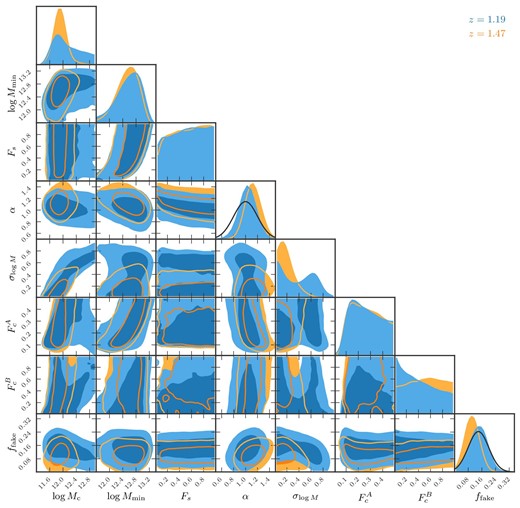
Constraints on parameters of the Geach HOD model, |$(\log {M_{\rm c}}, \log {M_{\rm min}}, \alpha , \sigma _{\log {M}}, F_{\rm s}, F_c^A,F_c^B)$| and ffake, for z = 1.19 (blue) and z = 1.47 (orange). Two-dimensional contours show the |$68\%$| and |$95\%$| confidence levels after the other six parameters are marginalized over. The diagonal panels show the posterior probability distribution of each parameter. Gaussian priors are assumed for α and ffake, as depicted by the black sold curves in the one-dimensional posterior panels. (Color online)
The posterior distribution of the HOD is shown in figure 8. The dark and light shaded regions show the 68% and 95% confidence intervals for the total HOD, respectively, and the red solid curve is the median. The black solid curve is the HOD with a set of parameters which give the minimum χ2 value in the eight-dimensional parameter space. The HOD with the minimum χ2 looks somewhat different from the median of the HOD, particularly the central HOD. This is a similar trend to that found by Hong et al. (2019). They found that two independent algorithms give very different best-fitting HOD parameters (see Model#1 and Model#2 of their figure 7). This small but non-negligible discrepancy largely comes from the fact that the normalization parameters, especially |$F_c^B$|, and the scatter of the mass, σlog M, are poorly constrained. Even though the HODs are different, the predicted angular correlation functions with these HOD parameter sets become very similar.
![HOD with the best-fitting parameters of the Geach model at z = 1.19 (top) and z = 1.47 (bottom). The blue and yellow lines show the average numbers of centrals from the Gaussian and error function terms [see equation (30)], while the green line shows that of satellites. The red solid line is the sum of the blue, yellow, and green lines, the average number of all the [O ii] emitters. The dark and light red shaded regions indicate the $68\%$ and $95\%$ confidence intervals. The red dashed curve is the same as the red solid curve but for the best-fitting HOD of Zheng et al. (2005). For comparison, the black curve shows the HOD of the Geach model which gives the minimum χ2 value. (Color online)](https://oup.silverchair-cdn.com/oup/backfile/Content_public/Journal/pasj/73/4/10.1093_pasj_psab068/2/m_psab068fig8.jpeg?Expires=1749450935&Signature=jMSdMx5jdPNY7p9vUQqKJMc63RnHqv4cP-8PDkhNKFspDxm1xmRRwAWwkNHNShdC9nOTkJh56JGXBabX3~pE8xlUnlamcqxKHzGHOt4aotVXTogQP8E8fjM7jcnvoEgX3r~WSny-ZipmvnU6ympTN5OnfXJaQPVk-o7YWdQKD9yqK2lVgG9Qlw5nphnC7fPnv~jcH6d0X4MZ0ECCfvQmQOKBjTmpvlecLA8K5JI4EtgNVS9H2PmJj92wu9OQZJ1p4vT8N~T7MqRdTZzkK3ZQDBTAp14n3B8QnfflNbDtgQ-pU797DN05CuaTa7SCqxmv9SsNEugnRUIY-zi97wQGRA__&Key-Pair-Id=APKAIE5G5CRDK6RD3PGA)
HOD with the best-fitting parameters of the Geach model at z = 1.19 (top) and z = 1.47 (bottom). The blue and yellow lines show the average numbers of centrals from the Gaussian and error function terms [see equation (30)], while the green line shows that of satellites. The red solid line is the sum of the blue, yellow, and green lines, the average number of all the [O ii] emitters. The dark and light red shaded regions indicate the |$68\%$| and |$95\%$| confidence intervals. The red dashed curve is the same as the red solid curve but for the best-fitting HOD of Zheng et al. (2005). For comparison, the black curve shows the HOD of the Geach model which gives the minimum χ2 value. (Color online)
The best-fitting Geach HOD model prediction, |$(1-f_{\rm fake})^2[w(\theta ;\Theta )-w_\Omega ]$|, is shown as the red solid curve in figure 9. The contributions from the one- and two-halo components are shown as the blue and green curves, respectively. Obviously, agreement of the measured correlation function with the HOD model prediction is much more remarkable than that with the power-law or linearly biased dark matter model, compared to figure 2.
![Angular correlation functions of [O ii] emitters at z = 1.19 (top) and z = 1.47 (bottom). Note that the vertical axis mixes logarithmic and linear scalings. The red points are the measurement, $\hat{w}$, the same as those in figure 2. The blue and green solid curves are the one- and two-halo terms with the best-fitting Geach HOD model, respectively, and the red solid curve is their sum. For comparison, we also show the best-fitting Zheng HOD model as the red dashed curve. The data enclosed by the two red vertical lines are used for this HOD analysis. (Color online)](https://oup.silverchair-cdn.com/oup/backfile/Content_public/Journal/pasj/73/4/10.1093_pasj_psab068/2/m_psab068fig9.jpeg?Expires=1749450935&Signature=U3S8mpDlxkjegwxIhn5KyM1IGkGhsuBtd-k0SY8hLGGJYYx5UvpzPO9llIRRMZo2o-AjMww6expa08ksTqTVymFITXQVolt8FJlqPq-ZgbDjNn0Q0hFOv3bvjjRxBOK2U2AUoJ2f4c7WT0sev6UAxvibY2KUzJ8NuleaUe3IL1QrqSFvOJIhG2-LzfIKbCu1GH30fz9iU11txoJrD4~6QEwLj6ozX2PeKGDxPvrQOVwOXEa2ayscWc3zonJvglR8BrClEtxiM~7ZS~BIhw1L-Q4P4R8ZXezbWCwBCFMSx0SPBtz5QVYciDBHHZ4xl8U5UolYkZDxWU9CxDkZgl7Bew__&Key-Pair-Id=APKAIE5G5CRDK6RD3PGA)
Angular correlation functions of [O ii] emitters at z = 1.19 (top) and z = 1.47 (bottom). Note that the vertical axis mixes logarithmic and linear scalings. The red points are the measurement, |$\hat{w}$|, the same as those in figure 2. The blue and green solid curves are the one- and two-halo terms with the best-fitting Geach HOD model, respectively, and the red solid curve is their sum. For comparison, we also show the best-fitting Zheng HOD model as the red dashed curve. The data enclosed by the two red vertical lines are used for this HOD analysis. (Color online)
5.4 Derived physical parameters for host halos
Using the constrained HOD parameters, one can infer the parameters which characterize the properties of [O ii] emitters and their host dark matter halos, such as the galaxy number density, effective halo bias and mass, and satellite galaxy fraction, through equations (21), (27), (28), and (29), respectively. The posterior distribution for these parameters is shown in figure 10 and summarized in table 3. The number density is constrained following the imposed prior. Note again that the constrained number density is different from the observed number density by a factor of (1 − ffake), |$n_{\rm g}^{\rm th} = (1-f_{\rm fake})n_{\rm g}^{\rm obs}$|. The effective bias parameter is determined at z = 1.19 and 1.47 as |$b_{\rm eff}=1.701^{+0.083}_{-0.110}$| and |$b_{\rm eff}=1.981^{+0.072}_{-0.068}$|, respectively. They are fully consistent with the linear bias parameter from much simpler analysis in subsection 4.3: |$b=1.61^{+0.13}_{-0.11}$| and |$b=2.09^{+0.17}_{-0.15}$|.
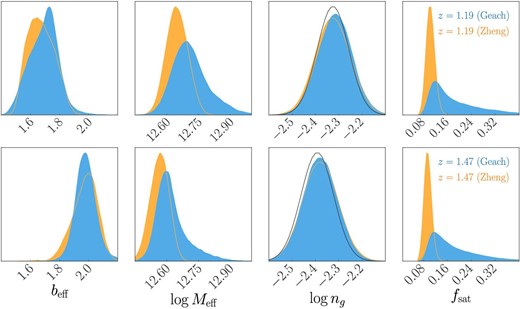
Posterior distribution for the parameters derived from the best-fitting parameters for the Geach (blue) and Zheng (orange) HOD models. From left to right, the posteriors for the effective bias, effective halo mass, galaxy number density, and satellite fraction are shown. The upper and lower panels show the results for z = 1.19 and z = 1.47, respectively. The number density presented here is different from the observed number density shown in table 1 by a factor of (1 − ffake), |$(1-f_{\rm fake})n_{\rm g}^{\rm obs}$| (see table 3 for the constrained values for ffake). The Gaussian priors assumed for log ng are depicted by the black solid curves, where the best-fitting value of ffake is adopted to multiply by (1 − ffake). The posterior of log ng for z = 1.47 (orange) is almost entirely behind the one for z = 1.19. (Color online)
The effective masses of halos hosting [O ii] emitters are derived to be |$\log {M_{\rm eff}/(h^{-1}\, {M}_{\odot })}=12.703^{+0.091}_{-0.069}$| and |$12.609^{+0.085}_{-0.051}$| at z = 1.19 and 1.47, respectively. Figure 11 shows the constraints on Meff as a function of redshift obtained from our HSC [O ii] emitters, together with the previous studies at 0 < z < 4 which were already presented in Kashino et al. (2017). The constraints include the studies of photo-z galaxies from the CFHT Legacy Survey at z ∼ 0.3, 0.5, and 0.7 (Coupon et al. 2012), the VIMOS-VLT Deep Survey (VVDS) at z ∼ 0.55 and 1 (Abbas et al. 2010), the NEWFIRM Medium Band Survey at z ∼ 1.1 and 1.5 (Wake et al. 2011), Hα emitters from the FMOS-COSMOS Survey at z ∼ 1.6 (Kashino et al. 2017) and from the HiZELS survey at z ∼ 2.2 (Geach et al. 2012), and VUDS at z ∼ 2.5 and 3.5 (Durkalec et al. 2015). These samples have number densities and stellar masses similar to our [O ii] emitter samples. Here we also plot the average mass assembly history of halos with different present-day masses, as derived by Behroozi, Wechsler, and Conroy (2013) based on an N-body simulation. According to this prediction, our measurements of Meff at two redshifts are well explained by the mass assembly history with M(z = 0) = 1.5 × 1013 h−1 M⊙, as depicted by the blue solid curve. The effective halo mass of [O ii] emitters at z = 1.47 is slightly higher than the blue curve, which reflects the fact that [O ii] emitters of our z = 1.47 sample are intrinsically brighter than those of the lower-z sample (see subsection 2.2). Given the accurate constraints on HOD, one can in principle infer the mass of the host halo for an individual emission line galaxy (Oguri & Lin 2015). It is, however, beyond the scope of this paper and will be investigated in future work.
![Average mass of halos hosting ELGs as a function of redshift. The blue stars are the results from our analysis of [O ii] emitters at z = 1.19 and z = 1.47. The other points are the results of the previous HOD analysis by Abbas et al. (2010), Wake et al. (2011), Geach et al. (2012), Coupon et al. (2012), Durkalec et al. (2015), and Kashino et al. (2017) (see the legend). The solid curves show a prediction of mass accretion histories for halos with M(z = 0) = 1015, 1014, 1013, and 1012 $M_{\odot} $ from top to bottom (Behroozi et al. 2013). The blue curve represents the one with M(z = 0) = 1.5 × 1013 [h−1 $M_{\odot} $]. (Color online)](https://oup.silverchair-cdn.com/oup/backfile/Content_public/Journal/pasj/73/4/10.1093_pasj_psab068/2/m_psab068fig11.jpeg?Expires=1749450935&Signature=ytb2J-N7fw1H-VUPYxsIEAxviFPnQ0quEKwgRSuPiDzusv~YsWT1VDgta5RzUmv0nBjP3m-UBjbbAVCJRqEx2NYaEbyjE5Shkp4HTIsdjOk5w9BF473vrmLHgo-xcTaOY83OvtmwOueGasNUIK1S6XVSl~LSILAKZVuG--WgaB8nh-jNXOEAVKRNCxVNVdGLokrZSjV0rs-SaEBT71ErmkgV04sqDG7lV7V9lhbwRfhzYhtFPxTYkbYcBLs-MLv2ihkbN9hkP77IIQskDObYlD0TMfd2Ng5c2B26ls3zWCKPd5ntjs6Qq553c-WOiJY0R1raSttXG~wGJrvNKtoKKQ__&Key-Pair-Id=APKAIE5G5CRDK6RD3PGA)
Average mass of halos hosting ELGs as a function of redshift. The blue stars are the results from our analysis of [O ii] emitters at z = 1.19 and z = 1.47. The other points are the results of the previous HOD analysis by Abbas et al. (2010), Wake et al. (2011), Geach et al. (2012), Coupon et al. (2012), Durkalec et al. (2015), and Kashino et al. (2017) (see the legend). The solid curves show a prediction of mass accretion histories for halos with M(z = 0) = 1015, 1014, 1013, and 1012 |$M_{\odot} $| from top to bottom (Behroozi et al. 2013). The blue curve represents the one with M(z = 0) = 1.5 × 1013 [h−1 |$M_{\odot} $|]. (Color online)
For our [O ii] emitter sample, the satellite galaxy fraction is constrained as |$f_{\rm sat}=0.158^{+0.114}_{-0.047}$| and |$0.159^{+0.109}_{-0.049}$| at z = 1.19 and 1.47, respectively. The preceding studies revealed that the satellite galaxy fraction of a given population would depend on the redshift, number density, and stellar mass (Coupon et al. 2012; Guo et al. 2014, 2019). At least over the redshift we studied here, the satellite fraction of [O ii] emitters in our sample does not significantly evolve with redshift. The analysis of Favole et al. (2016) constrained the satellite fraction of ELGs with the host halo mass M ∼ 1012 h−1 M⊙ as fsat = 0.225 ± 0.025. Avila et al. (2020) constrained the value of fsat for ELGs from the eBOSS survey, and the best-fitting value of their baseline model is fsat = 0.22, consistent with our measurements. However, their constraints are significantly model dependent, and varying the baseline model changes the best-fitting value over 0.18 ≤ fsat ≤ 0.70. Moreover, the selection of ELGs in the eBOSS survey is quite different from ours based on the NB. We thus cannot make a quantitative comparison with their result. Guo et al. (2019) further studied the relation among the satellite fraction, stellar mass, and halo mass for ELGs from the eBOSS survey. They showed that the host halo mass of ELGs with fsat ∼ 0.15 is log M/(h−1 M⊙) ∼ 12.6 and a strong function of the stellar mass. It provides good agreement with our clustering measurements of [O ii] emitters from the HSC survey.
5.5 Comparison with a simpler HOD
While the Geach HOD model is general and flexible, as a trade-off the HOD parameters are constrained more poorly and more degenerate with each other. Furthermore, it is not clear whether different halo occupation functions provide the same physical interpretation for a measured correlation function. A similar attempt has been made by studying one of the simplest HOD models (Zehavi et al. 2005) in Hong et al. (2019); see their appendix B. Here we investigate whether the predicted correlation function, halo occupation function, and host halo parameters of [O ii] emitters can be different between different HOD models.
The resulting constraints on the HOD parameters of Zheng’s model are shown in figure 12 and summarized in table 4. Overall, the constraints on the Zheng HOD model are tighter than those on the Geach model due to the smaller number of parameters. The posterior distribution of the HOD N(M) is shown as the red dashed curve in figure 8. Interestingly, the two models predict very similar HODs, particularly the central ones, for the observed angular correlation function of the [O ii] emitters. The discrepancy seen at M > 1013 h−1 M⊙ is reasonable because such massive halos are rare as hosts of [O ii] emitters; since the varied range of Fs in the Geach model is 0 ≤ Fs ≤ 1, the larger number of 〈Nsat〉 is allowed in the model (see the factor of 1/2 in the Zheng model). Furthermore, since the Zheng model has 〈Ncen〉 = 1 at the high-mass end, it would make sense for the Zheng model to have the lower number of 〈Nsat〉 when the total number density is fixed. Nevertheless, the two N(M) are consistent with each other within 2 σ. The best-fitting correlation function is shown as the red dashed curve in the upper and lower panels of figure 9 for z = 1.19 and z = 1.47, respectively. As expected, the overall shape of the correlation function is very similar to that of the Geach model depicted by the red solid curve. However, one can see that the Geach model shows a better fit to the measured w(θ) at the smallest scales, which would imply that the ELGs in our sample prefer the HOD model which allows massive halos to have no central ELG.
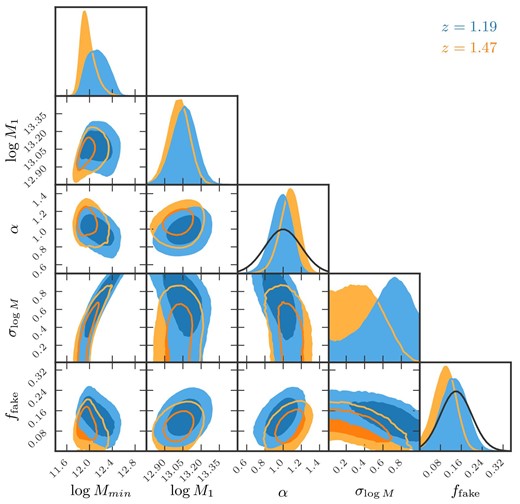
Constraints on HOD parameters of Zheng’s model, (log Mc, log Mmin, α, σlog M) and ffake, for z = 1.19 (blue) and z = 1.47 (orange). The contours show the |$68\%$| and |$95\%$| confidence levels. The diagonal panels show the posterior probability distribution of each parameter. Gaussian priors are assumed for α and ffake, as depicted by the black curves in the panels of the one-dimensional posterior distributions. (Color online)
. | . | NB816 . | NB921 . | ||
---|---|---|---|---|---|
. | . | Best fit . | Posterior PDF . | Best fit . | Posterior PDF . |
Parameter | Prior | ||||
log Mmin/(h−1 M⊙) | None | 12.16 | |$12.14^{+0.19}_{-0.18}$| | 11.88 | |$11.95^{+0.15}_{-0.10}$| |
log M1/(h−1 M⊙) | None | 13.070 | |$13.062^{+0.084}_{-0.090}$| | 13.000 | |$13.017^{+0.075}_{-0.078}$| |
σlog M | [0,1] | 0.68 | |$0.65^{+0.21}_{-0.32}$| | 0.18 | |$0.34^{+ 0.25}_{- 0.23}$| |
α | 1.00 ± 0.20 | 0.99 | |$0.98^{+0.11}_{-0.12}$| | 1.11 | |$1.07^{+ 0.10}_{- 0.11}$| |
ffake | 0.140 ± 0.060 | 0.123 | |$0.133^{+0.048}_{-0.048}$| | 0.115 | |$0.098^{+ 0.041}_{- 0.043}$| |
χ2/ν (ν = 10) | 1.86 | 1.42 | |||
Inferred quantity | Measurement | ||||
log ng/(h−1 Mpc)−3 | −2.259 ± 0.068 (NB816) | −2.327 | |$-2.325^{+0.075}_{-0.073}$| | −2.369 | |$-2.379^{+ 0.075}_{- 0.074}$| |
−2.344 ± 0.070 (NB921) | |||||
fsat | — | 0.100 | |$0.102^{+0.013}_{-0.012}$| | 0.098 | |$0.094^{ + 0.012}_{ - 0.011}$| |
beff | — | 1.659 | |$1.668^{+0.102}_{-0.089}$| | 2.034 | |$1.983^{+0.077}_{-0.095}$| |
log Meff/(h−1 M⊙) | — | 12.645 | |$12.647^{+0.052}_{-0.050}$| | 12.591 | |$12.567^{+0.043}_{-0.046}$| |
. | . | NB816 . | NB921 . | ||
---|---|---|---|---|---|
. | . | Best fit . | Posterior PDF . | Best fit . | Posterior PDF . |
Parameter | Prior | ||||
log Mmin/(h−1 M⊙) | None | 12.16 | |$12.14^{+0.19}_{-0.18}$| | 11.88 | |$11.95^{+0.15}_{-0.10}$| |
log M1/(h−1 M⊙) | None | 13.070 | |$13.062^{+0.084}_{-0.090}$| | 13.000 | |$13.017^{+0.075}_{-0.078}$| |
σlog M | [0,1] | 0.68 | |$0.65^{+0.21}_{-0.32}$| | 0.18 | |$0.34^{+ 0.25}_{- 0.23}$| |
α | 1.00 ± 0.20 | 0.99 | |$0.98^{+0.11}_{-0.12}$| | 1.11 | |$1.07^{+ 0.10}_{- 0.11}$| |
ffake | 0.140 ± 0.060 | 0.123 | |$0.133^{+0.048}_{-0.048}$| | 0.115 | |$0.098^{+ 0.041}_{- 0.043}$| |
χ2/ν (ν = 10) | 1.86 | 1.42 | |||
Inferred quantity | Measurement | ||||
log ng/(h−1 Mpc)−3 | −2.259 ± 0.068 (NB816) | −2.327 | |$-2.325^{+0.075}_{-0.073}$| | −2.369 | |$-2.379^{+ 0.075}_{- 0.074}$| |
−2.344 ± 0.070 (NB921) | |||||
fsat | — | 0.100 | |$0.102^{+0.013}_{-0.012}$| | 0.098 | |$0.094^{ + 0.012}_{ - 0.011}$| |
beff | — | 1.659 | |$1.668^{+0.102}_{-0.089}$| | 2.034 | |$1.983^{+0.077}_{-0.095}$| |
log Meff/(h−1 M⊙) | — | 12.645 | |$12.647^{+0.052}_{-0.050}$| | 12.591 | |$12.567^{+0.043}_{-0.046}$| |
In the “Prior” column the ranges specified in brackets are for uniform priors while for the others we quote the mean and standard deviation of the Gaussian priors. The “Best fit” column shows the parameter set which gives the minimum value of χ2. In the “Posterior PDF” column the central value is a median and the error means the 16th–84th percentiles after other parameters are marginalized over. The measured number density log ng includes non-[O ii] emitters, and thus its best-fitting values differ from the measure ones by log (1 − ffake) ∼ −0.06.
. | . | NB816 . | NB921 . | ||
---|---|---|---|---|---|
. | . | Best fit . | Posterior PDF . | Best fit . | Posterior PDF . |
Parameter | Prior | ||||
log Mmin/(h−1 M⊙) | None | 12.16 | |$12.14^{+0.19}_{-0.18}$| | 11.88 | |$11.95^{+0.15}_{-0.10}$| |
log M1/(h−1 M⊙) | None | 13.070 | |$13.062^{+0.084}_{-0.090}$| | 13.000 | |$13.017^{+0.075}_{-0.078}$| |
σlog M | [0,1] | 0.68 | |$0.65^{+0.21}_{-0.32}$| | 0.18 | |$0.34^{+ 0.25}_{- 0.23}$| |
α | 1.00 ± 0.20 | 0.99 | |$0.98^{+0.11}_{-0.12}$| | 1.11 | |$1.07^{+ 0.10}_{- 0.11}$| |
ffake | 0.140 ± 0.060 | 0.123 | |$0.133^{+0.048}_{-0.048}$| | 0.115 | |$0.098^{+ 0.041}_{- 0.043}$| |
χ2/ν (ν = 10) | 1.86 | 1.42 | |||
Inferred quantity | Measurement | ||||
log ng/(h−1 Mpc)−3 | −2.259 ± 0.068 (NB816) | −2.327 | |$-2.325^{+0.075}_{-0.073}$| | −2.369 | |$-2.379^{+ 0.075}_{- 0.074}$| |
−2.344 ± 0.070 (NB921) | |||||
fsat | — | 0.100 | |$0.102^{+0.013}_{-0.012}$| | 0.098 | |$0.094^{ + 0.012}_{ - 0.011}$| |
beff | — | 1.659 | |$1.668^{+0.102}_{-0.089}$| | 2.034 | |$1.983^{+0.077}_{-0.095}$| |
log Meff/(h−1 M⊙) | — | 12.645 | |$12.647^{+0.052}_{-0.050}$| | 12.591 | |$12.567^{+0.043}_{-0.046}$| |
. | . | NB816 . | NB921 . | ||
---|---|---|---|---|---|
. | . | Best fit . | Posterior PDF . | Best fit . | Posterior PDF . |
Parameter | Prior | ||||
log Mmin/(h−1 M⊙) | None | 12.16 | |$12.14^{+0.19}_{-0.18}$| | 11.88 | |$11.95^{+0.15}_{-0.10}$| |
log M1/(h−1 M⊙) | None | 13.070 | |$13.062^{+0.084}_{-0.090}$| | 13.000 | |$13.017^{+0.075}_{-0.078}$| |
σlog M | [0,1] | 0.68 | |$0.65^{+0.21}_{-0.32}$| | 0.18 | |$0.34^{+ 0.25}_{- 0.23}$| |
α | 1.00 ± 0.20 | 0.99 | |$0.98^{+0.11}_{-0.12}$| | 1.11 | |$1.07^{+ 0.10}_{- 0.11}$| |
ffake | 0.140 ± 0.060 | 0.123 | |$0.133^{+0.048}_{-0.048}$| | 0.115 | |$0.098^{+ 0.041}_{- 0.043}$| |
χ2/ν (ν = 10) | 1.86 | 1.42 | |||
Inferred quantity | Measurement | ||||
log ng/(h−1 Mpc)−3 | −2.259 ± 0.068 (NB816) | −2.327 | |$-2.325^{+0.075}_{-0.073}$| | −2.369 | |$-2.379^{+ 0.075}_{- 0.074}$| |
−2.344 ± 0.070 (NB921) | |||||
fsat | — | 0.100 | |$0.102^{+0.013}_{-0.012}$| | 0.098 | |$0.094^{ + 0.012}_{ - 0.011}$| |
beff | — | 1.659 | |$1.668^{+0.102}_{-0.089}$| | 2.034 | |$1.983^{+0.077}_{-0.095}$| |
log Meff/(h−1 M⊙) | — | 12.645 | |$12.647^{+0.052}_{-0.050}$| | 12.591 | |$12.567^{+0.043}_{-0.046}$| |
In the “Prior” column the ranges specified in brackets are for uniform priors while for the others we quote the mean and standard deviation of the Gaussian priors. The “Best fit” column shows the parameter set which gives the minimum value of χ2. In the “Posterior PDF” column the central value is a median and the error means the 16th–84th percentiles after other parameters are marginalized over. The measured number density log ng includes non-[O ii] emitters, and thus its best-fitting values differ from the measure ones by log (1 − ffake) ∼ −0.06.
The posterior distribution of the derived physical parameters is shown by the function colored orange in figure 10. Since the number density is primarily constrained by our prior, the two models predict the same values. We find that the effective bias beff and halo mass log Meff determined from the Zheng HOD model are also consistent with those from the Geach model within the statistical uncertainties. On the other hand, the satellite fraction is derived to be |$f_{\rm sat} =0.102^{+0.013}_{-0.012}$| and |$0.093^{+0.012}_{-0.011}$| at z = 1.19 and 1.47, respectively. These values are slightly lower than those determined based on the Geach HOD model, respectively |$0.158^{+0.114}_{-0.047}$| and |$0.159^{+0.109}_{-0.049}$|, though the differences are not significant. This again reflects the fact that the Geach model adopts a more flexible parameterization and the larger amplitude of 〈Nsat〉 is allowed. Hence, the one-dimensional posterior of fsat obtained from the Geach model has a long tail toward the high-fsat end.
Overall, the differences in the halo parameters for [O ii] emitters determined from the Geach and Zheng HOD models are not significant compared to the current statistical uncertainties. However, such small differences need to be carefully treated in future clustering analysis for ELGs from large galaxy surveys such as the Subaru PFS, DESI, and Euclid surveys.
6 Summary
In this paper we have studied the clustering of emission line galaxies at z > 1 and the physical properties of the dark matter halos which host them. For this purpose we used [O ii] emitters detected at z = 1.19 and 1.47 using two narrow-band filters, NB816 and NB921, respectively, in the Subaru HSC survey (Hayashi et al. 2020). We then measured the angular correlation functions of 8302 (z = 1.19) and 9578 (z = 1.47) [O ii] emitters. Using a simple model of the non-linear correlation function with a linear galaxy bias factor, we measured the bias of [O ii] emitters as |$b=1.61^{+0.13}_{-0.11}$| and |$2.09^{+0.17}_{-0.15}$| at z = 1.19 and 1.47, respectively. We also found that the bias monotonically increases with the line luminosity.
We, for the first time, performed a HOD analysis for the measured correlation functions of [O ii] emitters based on a model developed to describe the population of galaxies selected by star-forming rate (Geach et al. 2012). We varied eight parameters simultaneously with only a few priors. Nevertheless, the two HOD parameters related to the host halo mass have been well constrained given the large sample from the HSC survey.
Based on the constrained HOD parameters, we have derived parameters which describe properties of the host halos of [O ii] emitters, such as the effective bias, effective halo mass, and satellite fraction. The effective biases are determined as |$b_{\rm eff}=1.701^{+0.083}_{-0.110}$| and |$1.981^{+0.072}_{-0.068}$| at z = 1.19 and 1.47, respectively. They are consistent with the linear bias values determined based on a simple non-linear dark matter model. The effective halo masses and satellite fractions are derived as log Meff/(h−1 M⊙) ≃ 12.7 (12.6) and fsat ≃ 0.16 (0.16) at z = 1.19 (1.47). They are consistent with the results of Guo et al. (2019), who studied the relation among fsat, Meff, and the stellar mass using the ELG sample from the eBOSS survey. Furthermore, the determined effective halo masses are in good agreement with previous studies of similar tracers of the mass assembly history with M(z = 0) = 1.5 × 1013 h−1 M⊙.
As stated in section 1, ELGs will be a main tracer in large galaxy surveys at high redshifts. In particular, [O ii] emitters will be targeted by many ongoing/future surveys such as the Subaru PFS survey (Takada et al. 2014). The result presented in this paper is useful to construct a mock catalog for cosmological analysis. The physical properties of halos hosting [O ii] emitters revealed in this paper can also be applicable to the Subaru PFS, DESI, and other forthcoming surveys.
In this analysis, both the shape of the angular correlation function and the number density are constrained by the HOD modeling. However, there is a claim that in three-dimensional analysis the HOD would fail to explain the redshift-space clustering and number density simultaneously (Reid et al. 2014; Saito et al. 2016). To investigate this, we need a spectroscopic survey, which can be done with the PFS, DESI, or Euclid surveys.
The narrow-band data of HSC-SSP PDR2 provide emission lines of not only [O ii] but also Hα and [O iii], ranging from z ∼ 0.4 to z ∼ 1.6 (Hayashi et al. 2020). The redshift evolution of physical properties such as the halo mass for ELGs using these multiple emission lines will be studied in future work.
Acknowledgements
TO thanks the members of the Subaru PFS Cosmology Working Group, particularly Masahiro Takada, Eiichiro Komatsu, and Ryu Makiya, for useful correspondences during the regular telecon. TO is grateful to Shogo Ishikawa for discussions. We also thank the anonymous referee for their careful reading and suggestions. TO acknowledges support from the Ministry of Science and Technology of Taiwan under Grant MOST 109-2112-M-001-027- and the Career Development Award, Academia Sinica (AS-CDA-108-M02) for the period 2019–2023. YTL is grateful for support from the Ministry of Science & Technology of Taiwan under grant MOST 109-2112-M-001-005 and a Career Development Award from Academia Sinica (AS-CDA-106-M01). KO is supported by JSPS Overseas Research Fellowships. This work is based on data collected at Subaru Telescope, which is operated by the National Astronomical Observatory of Japan.
The Hyper Suprime-Cam (HSC) collaboration includes the astronomical communities of Japan and Taiwan, and Princeton University. The HSC instrumentation and software were developed by the National Astronomical Observatory of Japan (NAOJ), the Kavli Institute for the Physics and Mathematics of the Universe (Kavli IPMU), the University of Tokyo, the High Energy Accelerator Research Organization (KEK), the Academia Sinica Institute for Astronomy and Astrophysics in Taiwan (ASIAA), and Princeton University. Funding was contributed by the FIRST program from the Japanese Cabinet Office, the Ministry of Education, Culture, Sports, Science and Technology (MEXT), the Japan Society for the Promotion of Science (JSPS), Japan Science and Technology Agency (JST), the Toray Science Foundation, NAOJ, Kavli IPMU, KEK, ASIAA, and Princeton University.
Appendix 1 Correlation functions in individual fields
![Angular correlation functions of [O ii] emitters of each of the four fields measured at z = 1.19 (top) and z = 1.47 (bottom). In each column, the measurement from each of the individual four fields is presented. The black points are the raw measurement without the correction of the integral constraints, $\hat{w}_k$ (1 ≤ k ≤ 4). The red points are after the integral constraint (denoted by IC in the legend) is taken into account with the linearly biased dark matter model, $\hat{w}_k+(1-f_{\rm fake})^2w_{\Omega ,k}$, with the best-fitting parameters obtained for the combined field, (b, ffake) = (1.60, 0.140) (z = 1.19) and (b, ffake) = (2.08, 0.140) (z = 1.47). For reference, the angular correlation function measured for the combined field, $\hat{w}+(1-f_{\rm fake})^2w_\Omega$, is shown as the blue dashed curve. (Color online)](https://oup.silverchair-cdn.com/oup/backfile/Content_public/Journal/pasj/73/4/10.1093_pasj_psab068/2/m_psab068fig13.jpeg?Expires=1749450936&Signature=HX3yxx~0NHigC9uh5J6nkK2yiGzO3g6fgiyc~aJjHcpFrHq74PR8RXkHHuzSJsnj1~~A0nyXDEiI-95AEguLWl4x2mWMAFWqzng5EQmQFi9fCfBI30pVP2MvjltS-NzZu-Y2iGfDk5xJCuRRYbKpujHvwYptzZuPvXdn4AFcltI7~nKn0EEhvcXHE5vSFY8HTbf4IunMM0fMssaN9qvK8Y6icppoVPkr8P627TBh5JQHqyeVNb8DVHoMXbvaOnR7NBjAToe3dphQBO82Zt9fuDDWug2Hp9kPHN34PjnKHKcYg05RdLp~~s2hxFwxhGExIZlJ3UkD~i3R2fzYtVmGng__&Key-Pair-Id=APKAIE5G5CRDK6RD3PGA)
Angular correlation functions of [O ii] emitters of each of the four fields measured at z = 1.19 (top) and z = 1.47 (bottom). In each column, the measurement from each of the individual four fields is presented. The black points are the raw measurement without the correction of the integral constraints, |$\hat{w}_k$| (1 ≤ k ≤ 4). The red points are after the integral constraint (denoted by IC in the legend) is taken into account with the linearly biased dark matter model, |$\hat{w}_k+(1-f_{\rm fake})^2w_{\Omega ,k}$|, with the best-fitting parameters obtained for the combined field, (b, ffake) = (1.60, 0.140) (z = 1.19) and (b, ffake) = (2.08, 0.140) (z = 1.47). For reference, the angular correlation function measured for the combined field, |$\hat{w}+(1-f_{\rm fake})^2w_\Omega$|, is shown as the blue dashed curve. (Color online)
Appendix 2 Constraints on HOD parameters using clustering only
Given a HOD model for a certain galaxy population, the average number density is calculated by equation (21). One therefore needs to simultaneously analyze the observed correlation function and the number density to constrain the HOD model, as performed in subsection 5.3. In this appendix, we present the constraints on the HOD parameters based on the Geach and Zheng models without using the information of the measured number density of [O ii] emitters for readers who are interested in the constraining power coming from the clustering only; see, e.g., Matsuoka et al. (2011) and Martinez-Manso et al. (2015) for similar attempts.
Table 5 shows these results without using the abundance information. The halo mass parameters are strongly constrained by the abundance of [O ii] emitters. The constraints on these parameters are thus not as tight as those presented in tables 3 and 4, as expected: the errors on the Mc and Mmin constraints without the abundance information in the Geach model are ∼1.5 and ∼3 times larger than those with the simultaneous analysis of clustering and abundance, and the errors on the Mmin and M1 constraints without the abundance information in the Zheng model are ∼3 and ∼4 times larger. There are no significant changes in the constraints of the other HOD parameters. Interestingly, even though we do not use the number density to constrain the HOD parameters, the posterior of the number density is consistent with the observed one.
Priors and constraints of the HOD parameters for the Geach and Zheng models obtained using the clustering information only.*
. | . | NB816 . | NB921 . |
---|---|---|---|
. | . | Posterior PDF . | Posterior PDF . |
Geach HOD model | |||
Parameter | Prior | ||
log Mc/(h−1 M⊙) | None | |$11.50^{+0.72}_{-0.28}$| | |$11.70^{+ 0.25} _{- 0.20}$| |
log Mmin/(h−1 M⊙) | None | |$11.40^{+1.25}_{-1.00}$| | |$11.89^{+0.76}_{-1.36}$| |
σlog M | [0,1] | |$0.27^{+0.33}_{-0.17}$| | |$0.22^{+ 0.16}_{- 0.13}$| |
α | 1.00 ± 0.20 | |$0.96^{+0.11}_{-0.09}$| | |$1.10^{+ 0.13}_{- 0.13}$| |
|$F_c^A$| | [0, 0.5] | |$0.22^{+0.14}_{-0.07}$| | |$0.17^{+ 0.18}_{- 0.06}$| |
|$F_c^B$| | [0,1] | |$0.56^{+0.32}_{-0.39}$| | |$0.61^{+ 0.27}_{- 0.34}$| |
Fs | [0, 1] | |$0.54^{+0.32}_{-0.30}$| | |$0.52^{+ 0.32}_{- 0.34}$| |
ffake | 0.140 ± 0.060 | |$0.124^{+0.054}_{-0.051}$| | |$0.091^{+ 0.054}_{- 0.046}$| |
Inferred quantity | Measurement | ||
log ng/(h−1 Mpc)−3 | — | |$-1.47^{+0.89}_{-0.80}$| | |$-1.96^{+1.18}_{-0.42}$| |
fsat | — | |$0.58^{+0.33}_{-0.43}$| | |$0.38^{ + 0.54}_{ - 0.23}$| |
beff | — | |$1.64^{+0.12}_{-0.10}$| | |$1.94^{+0.13}_{-0.11}$| |
log Meff/(h−1 M⊙) | — | |$12.70^{+0.10}_{-0.09}$| | |$12.63^{+0.12}_{-0.10}$| |
Zheng HOD model | |||
Parameter | Prior | ||
log Mmin/(h−1 M⊙) | None | |$12.01^{+0.62}_{-0.61}$| | |$11.85^{+ 0.56}_{-0.26}$| |
log M1/(h−1 M⊙) | None | |$12.97^{+0.43}_{-0.64}$| | |$12.91^{+0.36}_{-0.31}$| |
σlog M | [0,1] | |$0.58^{+0.30}_{-0.41}$| | |$0.32^{+0.41}_{-0.22}$| |
α | 1.00 ± 0.20 | |$0.94^{+0.11}_{-0.11}$| | |$1.02^{+0.11}_{-0.11}$| |
ffake | 0.140 ± 0.060 | |$0.123^{+0.060}_{-0.063}$| | |$0.077^{+ 0.055}_{- 0.047}$| |
Inferred quantity | Measurement | ||
log ng/(h−1 Mpc)−3 | — | |$-2.24^{+0.56}_{- 0.44}$| | |$-2.27^{+0.28}_{-0.38}$| |
fsat | — | |$0.113^{+0.100}_{-0.044}$| | |$0.106^{+ 0.047}_{-0.040}$| |
beff | — | |$1.64^{+ 0.15}_{-0.15}$| | |$1.91^{+0.13}_{-0.11}$| |
log Meff/(h−1 M⊙) | — | |$12.633^{+0.102}_{-0.107}$| | |$12.534^{+0.091}_{-0.077}$| |
. | . | NB816 . | NB921 . |
---|---|---|---|
. | . | Posterior PDF . | Posterior PDF . |
Geach HOD model | |||
Parameter | Prior | ||
log Mc/(h−1 M⊙) | None | |$11.50^{+0.72}_{-0.28}$| | |$11.70^{+ 0.25} _{- 0.20}$| |
log Mmin/(h−1 M⊙) | None | |$11.40^{+1.25}_{-1.00}$| | |$11.89^{+0.76}_{-1.36}$| |
σlog M | [0,1] | |$0.27^{+0.33}_{-0.17}$| | |$0.22^{+ 0.16}_{- 0.13}$| |
α | 1.00 ± 0.20 | |$0.96^{+0.11}_{-0.09}$| | |$1.10^{+ 0.13}_{- 0.13}$| |
|$F_c^A$| | [0, 0.5] | |$0.22^{+0.14}_{-0.07}$| | |$0.17^{+ 0.18}_{- 0.06}$| |
|$F_c^B$| | [0,1] | |$0.56^{+0.32}_{-0.39}$| | |$0.61^{+ 0.27}_{- 0.34}$| |
Fs | [0, 1] | |$0.54^{+0.32}_{-0.30}$| | |$0.52^{+ 0.32}_{- 0.34}$| |
ffake | 0.140 ± 0.060 | |$0.124^{+0.054}_{-0.051}$| | |$0.091^{+ 0.054}_{- 0.046}$| |
Inferred quantity | Measurement | ||
log ng/(h−1 Mpc)−3 | — | |$-1.47^{+0.89}_{-0.80}$| | |$-1.96^{+1.18}_{-0.42}$| |
fsat | — | |$0.58^{+0.33}_{-0.43}$| | |$0.38^{ + 0.54}_{ - 0.23}$| |
beff | — | |$1.64^{+0.12}_{-0.10}$| | |$1.94^{+0.13}_{-0.11}$| |
log Meff/(h−1 M⊙) | — | |$12.70^{+0.10}_{-0.09}$| | |$12.63^{+0.12}_{-0.10}$| |
Zheng HOD model | |||
Parameter | Prior | ||
log Mmin/(h−1 M⊙) | None | |$12.01^{+0.62}_{-0.61}$| | |$11.85^{+ 0.56}_{-0.26}$| |
log M1/(h−1 M⊙) | None | |$12.97^{+0.43}_{-0.64}$| | |$12.91^{+0.36}_{-0.31}$| |
σlog M | [0,1] | |$0.58^{+0.30}_{-0.41}$| | |$0.32^{+0.41}_{-0.22}$| |
α | 1.00 ± 0.20 | |$0.94^{+0.11}_{-0.11}$| | |$1.02^{+0.11}_{-0.11}$| |
ffake | 0.140 ± 0.060 | |$0.123^{+0.060}_{-0.063}$| | |$0.077^{+ 0.055}_{- 0.047}$| |
Inferred quantity | Measurement | ||
log ng/(h−1 Mpc)−3 | — | |$-2.24^{+0.56}_{- 0.44}$| | |$-2.27^{+0.28}_{-0.38}$| |
fsat | — | |$0.113^{+0.100}_{-0.044}$| | |$0.106^{+ 0.047}_{-0.040}$| |
beff | — | |$1.64^{+ 0.15}_{-0.15}$| | |$1.91^{+0.13}_{-0.11}$| |
log Meff/(h−1 M⊙) | — | |$12.633^{+0.102}_{-0.107}$| | |$12.534^{+0.091}_{-0.077}$| |
In the “Prior” column the ranges specified in brackets are for uniform priors while for the others we quote the mean and standard deviation of the Gaussian priors. In the “Posterior PDF” column the central value is a median and the error means the 16th–84th percentiles after other parameters are marginalized over.
Priors and constraints of the HOD parameters for the Geach and Zheng models obtained using the clustering information only.*
. | . | NB816 . | NB921 . |
---|---|---|---|
. | . | Posterior PDF . | Posterior PDF . |
Geach HOD model | |||
Parameter | Prior | ||
log Mc/(h−1 M⊙) | None | |$11.50^{+0.72}_{-0.28}$| | |$11.70^{+ 0.25} _{- 0.20}$| |
log Mmin/(h−1 M⊙) | None | |$11.40^{+1.25}_{-1.00}$| | |$11.89^{+0.76}_{-1.36}$| |
σlog M | [0,1] | |$0.27^{+0.33}_{-0.17}$| | |$0.22^{+ 0.16}_{- 0.13}$| |
α | 1.00 ± 0.20 | |$0.96^{+0.11}_{-0.09}$| | |$1.10^{+ 0.13}_{- 0.13}$| |
|$F_c^A$| | [0, 0.5] | |$0.22^{+0.14}_{-0.07}$| | |$0.17^{+ 0.18}_{- 0.06}$| |
|$F_c^B$| | [0,1] | |$0.56^{+0.32}_{-0.39}$| | |$0.61^{+ 0.27}_{- 0.34}$| |
Fs | [0, 1] | |$0.54^{+0.32}_{-0.30}$| | |$0.52^{+ 0.32}_{- 0.34}$| |
ffake | 0.140 ± 0.060 | |$0.124^{+0.054}_{-0.051}$| | |$0.091^{+ 0.054}_{- 0.046}$| |
Inferred quantity | Measurement | ||
log ng/(h−1 Mpc)−3 | — | |$-1.47^{+0.89}_{-0.80}$| | |$-1.96^{+1.18}_{-0.42}$| |
fsat | — | |$0.58^{+0.33}_{-0.43}$| | |$0.38^{ + 0.54}_{ - 0.23}$| |
beff | — | |$1.64^{+0.12}_{-0.10}$| | |$1.94^{+0.13}_{-0.11}$| |
log Meff/(h−1 M⊙) | — | |$12.70^{+0.10}_{-0.09}$| | |$12.63^{+0.12}_{-0.10}$| |
Zheng HOD model | |||
Parameter | Prior | ||
log Mmin/(h−1 M⊙) | None | |$12.01^{+0.62}_{-0.61}$| | |$11.85^{+ 0.56}_{-0.26}$| |
log M1/(h−1 M⊙) | None | |$12.97^{+0.43}_{-0.64}$| | |$12.91^{+0.36}_{-0.31}$| |
σlog M | [0,1] | |$0.58^{+0.30}_{-0.41}$| | |$0.32^{+0.41}_{-0.22}$| |
α | 1.00 ± 0.20 | |$0.94^{+0.11}_{-0.11}$| | |$1.02^{+0.11}_{-0.11}$| |
ffake | 0.140 ± 0.060 | |$0.123^{+0.060}_{-0.063}$| | |$0.077^{+ 0.055}_{- 0.047}$| |
Inferred quantity | Measurement | ||
log ng/(h−1 Mpc)−3 | — | |$-2.24^{+0.56}_{- 0.44}$| | |$-2.27^{+0.28}_{-0.38}$| |
fsat | — | |$0.113^{+0.100}_{-0.044}$| | |$0.106^{+ 0.047}_{-0.040}$| |
beff | — | |$1.64^{+ 0.15}_{-0.15}$| | |$1.91^{+0.13}_{-0.11}$| |
log Meff/(h−1 M⊙) | — | |$12.633^{+0.102}_{-0.107}$| | |$12.534^{+0.091}_{-0.077}$| |
. | . | NB816 . | NB921 . |
---|---|---|---|
. | . | Posterior PDF . | Posterior PDF . |
Geach HOD model | |||
Parameter | Prior | ||
log Mc/(h−1 M⊙) | None | |$11.50^{+0.72}_{-0.28}$| | |$11.70^{+ 0.25} _{- 0.20}$| |
log Mmin/(h−1 M⊙) | None | |$11.40^{+1.25}_{-1.00}$| | |$11.89^{+0.76}_{-1.36}$| |
σlog M | [0,1] | |$0.27^{+0.33}_{-0.17}$| | |$0.22^{+ 0.16}_{- 0.13}$| |
α | 1.00 ± 0.20 | |$0.96^{+0.11}_{-0.09}$| | |$1.10^{+ 0.13}_{- 0.13}$| |
|$F_c^A$| | [0, 0.5] | |$0.22^{+0.14}_{-0.07}$| | |$0.17^{+ 0.18}_{- 0.06}$| |
|$F_c^B$| | [0,1] | |$0.56^{+0.32}_{-0.39}$| | |$0.61^{+ 0.27}_{- 0.34}$| |
Fs | [0, 1] | |$0.54^{+0.32}_{-0.30}$| | |$0.52^{+ 0.32}_{- 0.34}$| |
ffake | 0.140 ± 0.060 | |$0.124^{+0.054}_{-0.051}$| | |$0.091^{+ 0.054}_{- 0.046}$| |
Inferred quantity | Measurement | ||
log ng/(h−1 Mpc)−3 | — | |$-1.47^{+0.89}_{-0.80}$| | |$-1.96^{+1.18}_{-0.42}$| |
fsat | — | |$0.58^{+0.33}_{-0.43}$| | |$0.38^{ + 0.54}_{ - 0.23}$| |
beff | — | |$1.64^{+0.12}_{-0.10}$| | |$1.94^{+0.13}_{-0.11}$| |
log Meff/(h−1 M⊙) | — | |$12.70^{+0.10}_{-0.09}$| | |$12.63^{+0.12}_{-0.10}$| |
Zheng HOD model | |||
Parameter | Prior | ||
log Mmin/(h−1 M⊙) | None | |$12.01^{+0.62}_{-0.61}$| | |$11.85^{+ 0.56}_{-0.26}$| |
log M1/(h−1 M⊙) | None | |$12.97^{+0.43}_{-0.64}$| | |$12.91^{+0.36}_{-0.31}$| |
σlog M | [0,1] | |$0.58^{+0.30}_{-0.41}$| | |$0.32^{+0.41}_{-0.22}$| |
α | 1.00 ± 0.20 | |$0.94^{+0.11}_{-0.11}$| | |$1.02^{+0.11}_{-0.11}$| |
ffake | 0.140 ± 0.060 | |$0.123^{+0.060}_{-0.063}$| | |$0.077^{+ 0.055}_{- 0.047}$| |
Inferred quantity | Measurement | ||
log ng/(h−1 Mpc)−3 | — | |$-2.24^{+0.56}_{- 0.44}$| | |$-2.27^{+0.28}_{-0.38}$| |
fsat | — | |$0.113^{+0.100}_{-0.044}$| | |$0.106^{+ 0.047}_{-0.040}$| |
beff | — | |$1.64^{+ 0.15}_{-0.15}$| | |$1.91^{+0.13}_{-0.11}$| |
log Meff/(h−1 M⊙) | — | |$12.633^{+0.102}_{-0.107}$| | |$12.534^{+0.091}_{-0.077}$| |
In the “Prior” column the ranges specified in brackets are for uniform priors while for the others we quote the mean and standard deviation of the Gaussian priors. In the “Posterior PDF” column the central value is a median and the error means the 16th–84th percentiles after other parameters are marginalized over.
Footnotes
We use a composite model magnitude named cmodel.
See also Peebles (1980).
To show the parameter constraints using the MCMC sampling here and in the rest of this paper we use the Python package pygtc (Bocquet & Carter 2016).