-
PDF
- Split View
-
Views
-
Cite
Cite
Alexandra T Geanacopoulos, Pui Y Lee, Todd W Lyons, Kailey E Brodeur, Rachael K Aresco, Michael Monuteaux, Ron L Kaplan, Andrea T Cruz, Peter A Nigrovic, Lise E Nigrovic, for Pedi Lyme Net, Utility of Cytokine Biomarkers for the Diagnosis of Pediatric Pyogenic Musculoskeletal Infections, Open Forum Infectious Diseases, Volume 12, Issue 4, April 2025, ofaf139, https://doi.org/10.1093/ofid/ofaf139
- Share Icon Share
Abstract
Pyogenic musculoskeletal infections, such as septic arthritis and osteomyelitis, require prompt recognition and treatment but can be challenging to distinguish from Lyme or inflammatory arthritis. Our goal was to identify cytokine biomarkers of musculoskeletal infections.
Using 2 multicenter prospective cohorts of children undergoing emergency department evaluation for musculoskeletal infection, we selected children ≤21 years of age with a musculoskeletal infection (cases) matched by age and sex to children with Lyme and inflammatory arthritis (controls). We performed a 45-cytokine/chemokine panel using the Olink proximity extension assay platform and used receiver operator curve analysis to evaluate the discriminative ability of each cytokine. Using forward stepwise logistic regression, we derived a 3-cytokine panel and compared the accuracy with 5 commonly available plasma biomarkers.
We included 47 children with musculoskeletal infection, 48 with Lyme arthritis, and 49 with inflammatory arthritis. Interleukin-6 had the highest accuracy for musculoskeletal infection (area under the curve [AUC], 0.84; 95% CI, 0.77–0.91). A 3-cytokine biosignature panel (interleukin-6, interleukin-17A, and colony stimulating factor–1) had the highest overall accuracy (AUC, 0.90; 95% CI, 0.84–0.96) and performed better than 5 common plasma biomarkers (white blood cell count, absolute neutrophil count, C-reactive protein, erythrocyte sedimentation rate, and procalcitonin; P < .05 for all comparisons).
Plasma cytokines can distinguish musculoskeletal infections from Lyme or inflammatory arthritis and may assist initial decision-making for children undergoing evaluation for musculoskeletal infection.
Given the limitations of our current diagnostic tools, timely diagnosis of pyogenic musculoskeletal infections (MSKIs), such as septic arthritis and osteomyelitis, in children presenting with joint pain and swelling can be challenging [1–4]. Pyogenic MSKIs have considerable overlap with Lyme arthritis and other infectious and inflammatory musculoskeletal conditions [1, 2, 5]. Clinicians are charged with ensuring timely diagnosis to prevent downstream acute and chronic complications such as bacteremia, sepsis, osteoarthritis, and joint destruction [6–8], while at the same time minimizing invasive procedures such as arthrocentesis for children at low risk for MSKIs [9].
Given the distinctly different immunologic host responses to viral infections, bacterial infections, and inflammatory conditions [10, 11], cytokine expression patterns can assist clinicians in identifying etiology of disease. Prior work has identified a distinct cytokine response in the blood of patients with Lyme disease [12]. Blood cytokines may also distinguish between children with bacterial and viral causes of fever or radiographic pneumonia [12–14]. The Olink proximity extension assay is a proteomics platform that accurately quantifies multiple cytokines with high sensitivity and specificity, while requiring only 1 µL of plasma or serum. A recent study using this same platform demonstrated the ability of cytokine expression to distinguish children with Kawasaki disease from clinical mimics [11]. However, this platform has not been explored for the identification of pyogenic MSKIs.
To this end, we evaluated the ability of plasma cytokines to distinguish children with pyogenic MSKI from those with Lyme disease and other types of inflammatory arthritis. Accordingly, we sought to (1) evaluate the discriminatory power of individual cytokines, (2) develop a pilot multicytokine biosignature panel to identify children with a pyogenic MSKI, and (3) compare its performance with that of commonly used traditional biomarkers.
METHODS
Study Design
We performed a case–control analysis using children undergoing evaluation for potential MSKIs from 2 prospective cohort studies of children ≤21 years of age undergoing evaluation for MSKI. The first cohort enrolled children with monoarthritis who had Lyme disease serology obtained at 1 of 8 Pedi Lyme Net centers located in Lyme disease–endemic areas between 2015 and 2023 (cohort details described previously) [15, 16], referred to as the suspected Lyme arthritis cohort. The second cohort enrolled children undergoing evaluation for suspected MSKI between 2020 and 2023 at 1 of 3 centers: Texas Children's Hospital (Houston, TX, USA), Boston Children's Hospital (Boston, MA, USA), and Seattle Children's Hospital (Seattle, WA, USA), referred to as the suspected pyogenic MSKI cohort. Both study protocols were approved by the institutional review board at each participating institution with permission for data and sample sharing.
Study Population
We obtained informed consent for study participation from caregivers (or the patient for ages 18 to 21 years), with assent as appropriate. Study participation included capture of clinical history and phenotype, collection of research biosamples, and clinical follow-up for 1 month from enrollment. After collection, samples were centrifuged immediately, and serum samples were stored at −80℃ and transferred to the study biobank housed at Boston Children's Hospital (Boston, MA, USA).
Data Collection
Study team members were trained in data abstraction and utilized a comprehensive study manual of operations. The following data were collected using a standardized data collection tool in Research Electronic Data Capture (REDCap), hosted by Harvard University: demographics (patient age, sex, parent-reported race and ethnicity, enrolling center), clinical history as reported by the treating clinician (fever, joint pain, duration of symptoms), and examination (joint swelling). The study team reviewed medical records to collect the following results: laboratory (white blood cell count [WBC], absolute neutrophil count [ANC], erythrocyte sedimentation rate [ESR], C-reactive protein [CRP]), microbiology (blood and synovial fluid cultures), and radiologic imaging (magnetic resonance imaging [MRI] or bone scan). We performed procalcitonin (PCT) testing using available biosamples in a single research laboratory (Boston Children's Hospital).
Nested Case–Control
For this substudy, we selected all cases with a pyogenic MSKI enrolled in either study cohort with an available research biosample (n = 48). We combined children with pyomyositis, osteomyelitis, and septic arthritis given the overlap in clinical presentations and that all can mimic Lyme and inflammatory arthritis. Selecting from children enrolled in the Lyme arthritis prospective cohort, we then age- and sex-matched 1 control with Lyme arthritis (n = 48) and 1 with other inflammatory arthritis (n = 48).
Outcome Measures
We defined a pyogenic MSKI as 1 or more of the following diagnoses: septic arthritis, pyomyositis, or osteomyelitis [16]. Septic arthritis was defined by 1 of the following: (1) pathogenic bacterial growth on synovial fluid culture and/or (2) pathogenic bacterial growth on blood culture with concurrent synovial fluid pleocytosis (>50 000 WBC/high-power field) [16]. Pyomyositis and osteomyelitis were defined radiographically either with MRI or bone scan. All other children were classified as having a nonpyogenic MSKI including Lyme arthritis or other inflammatory arthritis. We defined Lyme arthritis with a positive standard 2-tier Lyme disease serology (a first-tier enzyme immunoassay followed by a second-tier immunoblot).
Olink Multicytokine Analysis
Using research serum samples, we performed a multicytokine assay using the Target-48 panel from Olink (Thermo Fisher, Waltham, MA, USA) to accurately quantify levels of 45 different proinflammatory mediators with high sensitivity and specificity [11]. The platform utilizes antibody-based cytokine detection coupled with signal amplification of a complementary nucleic acid sequence conjugated to each antibody pair. This technology is ideal for biomarker discovery and exhibits advantages compared with conventional methods due to (1) superior sensitivity of detecting analytes in the subpicogram per mL range, (2) minimal sample requirement (1 µL), and (3) simultaneous analysis of multiple cytokines with high specificity [17, 18].
Primary Analysis
For all analyses, we compared children with a pyogenic MSKI with those with a nonpyogenic MSKI. We chose to combine children with Lyme arthritis and other inflammatory arthritis in the primary analysis with the rationale that these are the children who need to be distinguished from those with pyogenic MSKI. We performed Spearman's rank correlation tests to assess correlation among the 11 cytokines in the research Olink panel that were statistically different on Wilcoxon rank-sum testing. Descriptive statistics, median and IQR, were used to describe the 45 cytokines by category (pyogenic vs nonpyogenic MSKI). Differences between the 2 groups were analyzed using Wilcoxon rank-sum testing, using a Bonferroni-adjusted P value of .001, adjusting for performance of 45 different tests.
For each cytokine that was statistically significant on Wilcoxon rank-sum testing, we constructed a receiver operating characteristics (ROC) curve to ascertain the accuracy in distinguishing between pyogenic and nonpyogenic MSKI. We evaluated the area under the curve (AUC) with 95% confidence intervals. We used the Liu method to select the optimal cut-point values by maximizing the product of sensitivity and specificity [19]. Binomial 95% CIs were calculated for sensitivity and specificity at the optimal cut-points. We used the DeLong method [20] to compare the AUCs of each cytokine.
To derive a pilot multicytokine biosignature panel, we performed a forward selection logistic model with MSKI status as the dependent variable, evaluating each of the cytokines found to be significant on Wilcoxon rank-sum testing. Forward selection is a modeling technique that adds variables individually in order of significance until no additional variables improve the model, and it was chosen due to its minimial susceptibility to variable collinearity [21]. Cytokines were incorporated as continuous values into the model. We then derived the sensitivity and specificity of this multicytokine panel to distinguish pyogenic from nonpyogenic MSKI diagnoses at different output probabilities of 0%, 5%, and 10%, followed by intervals of 10% to 100%.
Supplemental Analyses
To compare the utility of cytokines identified by our analysis with usual blood biomarkers, we also constructed ROC curves for WBC, PCT, ESR, CRP, and ANC to distinguish pyogenic from nonpyogenic MSKIs in the study cohort. We then compared the accuracy of the novel multicytokine biosignature panel with these biomarkers. Based on our prior studies, we believed that children with pyogenic MSKIs and Lyme arthritis would have the greatest clinical overlap [1, 2, 16]. To address this concern, we excluded children with other types of inflammatory arthritis and then compared the pyogenic MSKI cases with controls with Lyme arthritis and determined the diagnostic accuracy of the multicytokine panel to identify children with a pyogenic MSKI.
All statistical analyses were performed in R, version 4.3.2 (R Foundation for Statistical Computing, Vienna, Austria).
RESULTS
We enrolled 144 patients for this study: 122 from the suspected Lyme arthritis cohort and 22 from the suspected pyogenic MSKI cohort. Overall, 47 (33%) children had a pyogenic MSKI, 48 (33%) had Lyme arthritis, and 49 (34%) had other inflammatory arthritis. Males represented 60% of the cohort (n = 87). The majority of patients (n = 127; 88%) had not received antibiotics before emergency department evaluation and did not have a history of fever (n = 33; 60%). Of the 133 (94%) with joint involvement on exam, the knee was the most commonly affected (n = 111; 78%), followed by the hip (n = 6; 4%).
Of the 144 study patients, Olink data were available for 143 (99%). One child was excluded due to insufficient research sample quantity for analysis. Of the 45 cytokines/chemokines evaluated, 11 differed between cases and controls after adjustment for multiple comparisons (Figure 1; Supplementary Figure 1). The correlation between the 11 identified cytokines is shown in Supplementary Figure 2. The remaining 34 cytokines did not differ between those children with a pyogenic MSKI and those with other types of arthritis (Supplementary Table 1).
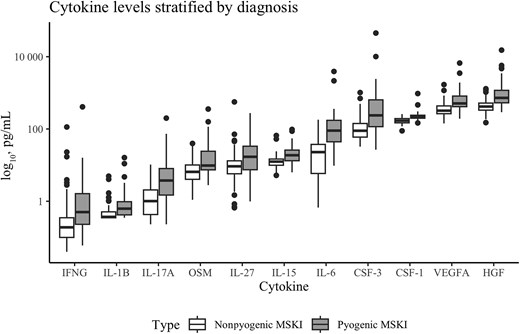
Boxplot showing the median (IQR) levels (pg/mL) for the 11 cytokines that differed between children with and without a pyogenic musculoskeletal infection. Y-axis is logarithmic. The median appears higher in children with pyogenic musculoskeletal infection for all cytokines as compared with children without pyogenic musculoskeletal infection. Abbreviations: CSF-1, colony-stimulating factor-1; CSF-3, colony-stimulating factor-3; HGF, hepatocyte growth factor; IFNG, interferon-gamma; IL-1B, interleukin-1B; IL-6, interleukin-6; IL-15, interleukin-15; IL-17A, interleukin-17A; IL-27, interleukin-27; IQR, interquartile range; MSKI, musculoskeletal infection; OSM, oncostatin M; VEGFA, vascular endothelial growth factor A.
Interleukin-6 (IL-6) had the highest overall accuracy (AUC, 0.84; 95% CI, 0.77–0.91), although the confidence intervals for the AUCs for the other selected cytokines overlapped considerably with that of IL-6. Next, we selected the opimal cut-point for each of the 11 selected cytokines and calculated the sensitivity and specificity (Table 1).
Test Characteristics for Each Individual Cytokine Demonstrating the Area Under the Curve (95% CI), Optimal Cut-point, and Sensitivity and Specificity at the Optimal Cut-point
Cytokine . | AUC (95% CI) . | Optimal Cut-point, pg/mL . | Sensitivity, % . | Specificity, % . | Pa . |
---|---|---|---|---|---|
IL-6 | 0.84 (0.77–0.91) | 38.8 | 80.4 (71.3–84.6) | 76.3 (68.7–82.5) | Ref |
CSF-1 | 0.83 (0.76–0.90) | 198.3 | 76.1 (68.4–82.3) | 81.4 (74.2–86.9) | .85 |
HGF | 0.82 (0.75–0.89) | 525.3 | 76.1 (68.5–82.3) | 77.3 (70.0–83.4) | .58 |
VEGFA | 0.78 (0.70–0.87) | 401.3 | 80.4 (72.7–85.7) | 69.0 (61.0–76.0) | .21 |
IL-17A | 0.76 (0.67–0.85) | 2.6 | 63.0 (54.8–70.5) | 81.4 (74.2–86.9) | .11 |
IL-15 | 0.77 (0.68–0.86) | 15.9 | 69.6 (1.6–76.5) | 81.4 (74.2–86.9) | .17 |
CSF-3 | 0.77 (0.68–0.85) | 149.5 | 63.0 (54.8–70.5) | 79.4 (72.0–85.2) | .10 |
OSM | 0.74 (0.65–0.82) | 6.9 | 80.4 (73.1–86.0) | 54.6 (46.4–62.5) | .03 |
IL-1β | 0.74 (0.65–0.83) | 0.6 | 60.9 (51.4–67.3) | 82.5 (75.4–87.9) | .06 |
IFNγ | 0.72 (0.64–0.82) | 0.4 | 60.9 (52.7–68.5) | 80.4 (73.1–86.1) | .04 |
IL-27 | 0.68 (0.58–0.78) | 12.4 | 63.0 (54.8–70.5) | 74.2 (66.5–80.7) | .01 |
Cytokine . | AUC (95% CI) . | Optimal Cut-point, pg/mL . | Sensitivity, % . | Specificity, % . | Pa . |
---|---|---|---|---|---|
IL-6 | 0.84 (0.77–0.91) | 38.8 | 80.4 (71.3–84.6) | 76.3 (68.7–82.5) | Ref |
CSF-1 | 0.83 (0.76–0.90) | 198.3 | 76.1 (68.4–82.3) | 81.4 (74.2–86.9) | .85 |
HGF | 0.82 (0.75–0.89) | 525.3 | 76.1 (68.5–82.3) | 77.3 (70.0–83.4) | .58 |
VEGFA | 0.78 (0.70–0.87) | 401.3 | 80.4 (72.7–85.7) | 69.0 (61.0–76.0) | .21 |
IL-17A | 0.76 (0.67–0.85) | 2.6 | 63.0 (54.8–70.5) | 81.4 (74.2–86.9) | .11 |
IL-15 | 0.77 (0.68–0.86) | 15.9 | 69.6 (1.6–76.5) | 81.4 (74.2–86.9) | .17 |
CSF-3 | 0.77 (0.68–0.85) | 149.5 | 63.0 (54.8–70.5) | 79.4 (72.0–85.2) | .10 |
OSM | 0.74 (0.65–0.82) | 6.9 | 80.4 (73.1–86.0) | 54.6 (46.4–62.5) | .03 |
IL-1β | 0.74 (0.65–0.83) | 0.6 | 60.9 (51.4–67.3) | 82.5 (75.4–87.9) | .06 |
IFNγ | 0.72 (0.64–0.82) | 0.4 | 60.9 (52.7–68.5) | 80.4 (73.1–86.1) | .04 |
IL-27 | 0.68 (0.58–0.78) | 12.4 | 63.0 (54.8–70.5) | 74.2 (66.5–80.7) | .01 |
Abbreviations: AUC, area under the curve; CSF-1, colony-stimulating factor-1; CSF-3, colony-stimulating factor-3; HGF, hepatocyte growth factor; IFNγ, interferon-γ; IL-1B, interleukin-1B; IL-6, interleukin-6; IL-15, interleukin-15; IL-17A, interleukin-17A; IL-27, interleukin-27; OSM, oncostatin M; VEGFA, vascular endothelial growth factor A.
aCalculated in comparison with IL-6.
Test Characteristics for Each Individual Cytokine Demonstrating the Area Under the Curve (95% CI), Optimal Cut-point, and Sensitivity and Specificity at the Optimal Cut-point
Cytokine . | AUC (95% CI) . | Optimal Cut-point, pg/mL . | Sensitivity, % . | Specificity, % . | Pa . |
---|---|---|---|---|---|
IL-6 | 0.84 (0.77–0.91) | 38.8 | 80.4 (71.3–84.6) | 76.3 (68.7–82.5) | Ref |
CSF-1 | 0.83 (0.76–0.90) | 198.3 | 76.1 (68.4–82.3) | 81.4 (74.2–86.9) | .85 |
HGF | 0.82 (0.75–0.89) | 525.3 | 76.1 (68.5–82.3) | 77.3 (70.0–83.4) | .58 |
VEGFA | 0.78 (0.70–0.87) | 401.3 | 80.4 (72.7–85.7) | 69.0 (61.0–76.0) | .21 |
IL-17A | 0.76 (0.67–0.85) | 2.6 | 63.0 (54.8–70.5) | 81.4 (74.2–86.9) | .11 |
IL-15 | 0.77 (0.68–0.86) | 15.9 | 69.6 (1.6–76.5) | 81.4 (74.2–86.9) | .17 |
CSF-3 | 0.77 (0.68–0.85) | 149.5 | 63.0 (54.8–70.5) | 79.4 (72.0–85.2) | .10 |
OSM | 0.74 (0.65–0.82) | 6.9 | 80.4 (73.1–86.0) | 54.6 (46.4–62.5) | .03 |
IL-1β | 0.74 (0.65–0.83) | 0.6 | 60.9 (51.4–67.3) | 82.5 (75.4–87.9) | .06 |
IFNγ | 0.72 (0.64–0.82) | 0.4 | 60.9 (52.7–68.5) | 80.4 (73.1–86.1) | .04 |
IL-27 | 0.68 (0.58–0.78) | 12.4 | 63.0 (54.8–70.5) | 74.2 (66.5–80.7) | .01 |
Cytokine . | AUC (95% CI) . | Optimal Cut-point, pg/mL . | Sensitivity, % . | Specificity, % . | Pa . |
---|---|---|---|---|---|
IL-6 | 0.84 (0.77–0.91) | 38.8 | 80.4 (71.3–84.6) | 76.3 (68.7–82.5) | Ref |
CSF-1 | 0.83 (0.76–0.90) | 198.3 | 76.1 (68.4–82.3) | 81.4 (74.2–86.9) | .85 |
HGF | 0.82 (0.75–0.89) | 525.3 | 76.1 (68.5–82.3) | 77.3 (70.0–83.4) | .58 |
VEGFA | 0.78 (0.70–0.87) | 401.3 | 80.4 (72.7–85.7) | 69.0 (61.0–76.0) | .21 |
IL-17A | 0.76 (0.67–0.85) | 2.6 | 63.0 (54.8–70.5) | 81.4 (74.2–86.9) | .11 |
IL-15 | 0.77 (0.68–0.86) | 15.9 | 69.6 (1.6–76.5) | 81.4 (74.2–86.9) | .17 |
CSF-3 | 0.77 (0.68–0.85) | 149.5 | 63.0 (54.8–70.5) | 79.4 (72.0–85.2) | .10 |
OSM | 0.74 (0.65–0.82) | 6.9 | 80.4 (73.1–86.0) | 54.6 (46.4–62.5) | .03 |
IL-1β | 0.74 (0.65–0.83) | 0.6 | 60.9 (51.4–67.3) | 82.5 (75.4–87.9) | .06 |
IFNγ | 0.72 (0.64–0.82) | 0.4 | 60.9 (52.7–68.5) | 80.4 (73.1–86.1) | .04 |
IL-27 | 0.68 (0.58–0.78) | 12.4 | 63.0 (54.8–70.5) | 74.2 (66.5–80.7) | .01 |
Abbreviations: AUC, area under the curve; CSF-1, colony-stimulating factor-1; CSF-3, colony-stimulating factor-3; HGF, hepatocyte growth factor; IFNγ, interferon-γ; IL-1B, interleukin-1B; IL-6, interleukin-6; IL-15, interleukin-15; IL-17A, interleukin-17A; IL-27, interleukin-27; OSM, oncostatin M; VEGFA, vascular endothelial growth factor A.
aCalculated in comparison with IL-6.
Using a forward selection process, we selected the following 3 cytokines for inclusion in a multiplex diagnostic panel for pyogenic MSKI: IL-6 (adjusted odds ratio [aOR], 1.01; 95% CI, 1.00–1.03), interleukin-17A (IL-17A; aOR, 1.35; 95% CI, 1.09–1.67), and colony-stimulating factor 1 (CSF-1; OR, 1.03; 95% CI, 1.01–1.05). This 3-cytokine panel accurately distinguishes pyogenic from nonpyogenic MSKI (AUC, 0.90; 95% CI, 0.84–0.96) (Figure 2). This panel demonstrated better accuracy than IL-17A alone but did not improve on IL-6 alone or CSF-1 alone.
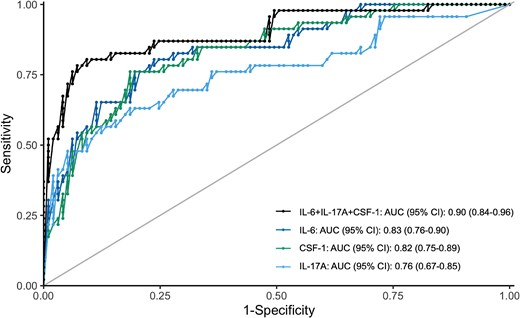
Receiver operating characteristics curves demonstrating the performance of the 3-cytokine panel (IL-6 + CSF-1 + IL-17A) compared with IL-6 alone, CSF-1 alone, and IL-17A alone. IL-6 + CSF-1 + IL-17A: AUC, 0.90; 95% CI, 0.84–0.96. IL-6: AUC, 0.83; 95% CI, 0.76–0.90. CSF-1: AUC, 0.82; 95% CI, 0.75–0.89. IL-17A: AUC, 0.76; 95% CI, 0.67–0.85. Abbreviations: AUC, area under the curve; CSF-1, colony-stimulating factor 1; IL-6, interleukin 6; IL-17A, interleukin 17A.
Using the 3-cytokine panel, increasing predictive probability decreased sensitivity but increased specificity (Table 2). For a predictive probability of pyogenic MSKI ≥5% based on the regression model, the 1 misclassified child with a pyogenic MSKI was a 13-year-old girl who presented with 3 days of fever, limp, and foot pain, found to have metatarsal osteomyelitis on MRI. For a predicted probability of ≥10%, the model additionally misclassified a 14-year-old boy with fever and elbow swelling with a positive elbow synovial fluid culture for Staphylococcus aureus. For a predicted probability of ≥20%, 4 additional children were misclassified: a 15-year-old afebrile boy with ankle pain and limp found to have pyomyositis and metatarsal osteomyelitis by MRI, a 16-year-old afebrile girl with knee pain found to have postoperative Staphylococcus epidermidis septic arthritis, a 12-year-old afebrile boy with foot pain with osteomyelitis by MRI, and a 14-year-old febrile boy with knee pain found to have Staphylococcus aureus septic arthritis.
Sensitivity and Specificity at Different Cut-points for Three-Cytokine Panel (IL-6 + IL-17A + CSF-1)
Predicted Probability, % . | True Positive n = 46 . | True-Positive Rate Sensitivity (95% CI), % . | 1 - False-Positive Rate Specificity (95% CI), % . |
---|---|---|---|
≥0 | 46 | 100 (90–100) | 0 (0–4) |
≥5 | 45 | 98 (87–100) | 30 (21–40) |
≥10 | 44 | 96 (84–99) | 51 (40–61) |
≥20 | 40 | 87 (73–95) | 72 (62–81) |
≥30 | 37 | 80 (66–90) | 86 (77–92) |
≥40 | 34 | 74 (59–85) | 94 (87–98) |
≥50 | 31 | 67 (52–80) | 96 (89–99) |
≥60 | 27 | 59 (43–73) | 96 (90–98) |
≥70 | 24 | 52 (37–67) | 97 (91–99) |
≥80 | 23 | 50 (36–64) | 99 (94–100) |
≥90 | 18 | 39 (26–56) | 99 (94–100) |
≥100 | 0 | 0 (0–10) | 100 (96–100) |
Predicted Probability, % . | True Positive n = 46 . | True-Positive Rate Sensitivity (95% CI), % . | 1 - False-Positive Rate Specificity (95% CI), % . |
---|---|---|---|
≥0 | 46 | 100 (90–100) | 0 (0–4) |
≥5 | 45 | 98 (87–100) | 30 (21–40) |
≥10 | 44 | 96 (84–99) | 51 (40–61) |
≥20 | 40 | 87 (73–95) | 72 (62–81) |
≥30 | 37 | 80 (66–90) | 86 (77–92) |
≥40 | 34 | 74 (59–85) | 94 (87–98) |
≥50 | 31 | 67 (52–80) | 96 (89–99) |
≥60 | 27 | 59 (43–73) | 96 (90–98) |
≥70 | 24 | 52 (37–67) | 97 (91–99) |
≥80 | 23 | 50 (36–64) | 99 (94–100) |
≥90 | 18 | 39 (26–56) | 99 (94–100) |
≥100 | 0 | 0 (0–10) | 100 (96–100) |
Abbreviations: CSF-1, colony-stimulating factor 1; IL-6, interleukin; IL-17A, interleukin 17A.
Sensitivity and Specificity at Different Cut-points for Three-Cytokine Panel (IL-6 + IL-17A + CSF-1)
Predicted Probability, % . | True Positive n = 46 . | True-Positive Rate Sensitivity (95% CI), % . | 1 - False-Positive Rate Specificity (95% CI), % . |
---|---|---|---|
≥0 | 46 | 100 (90–100) | 0 (0–4) |
≥5 | 45 | 98 (87–100) | 30 (21–40) |
≥10 | 44 | 96 (84–99) | 51 (40–61) |
≥20 | 40 | 87 (73–95) | 72 (62–81) |
≥30 | 37 | 80 (66–90) | 86 (77–92) |
≥40 | 34 | 74 (59–85) | 94 (87–98) |
≥50 | 31 | 67 (52–80) | 96 (89–99) |
≥60 | 27 | 59 (43–73) | 96 (90–98) |
≥70 | 24 | 52 (37–67) | 97 (91–99) |
≥80 | 23 | 50 (36–64) | 99 (94–100) |
≥90 | 18 | 39 (26–56) | 99 (94–100) |
≥100 | 0 | 0 (0–10) | 100 (96–100) |
Predicted Probability, % . | True Positive n = 46 . | True-Positive Rate Sensitivity (95% CI), % . | 1 - False-Positive Rate Specificity (95% CI), % . |
---|---|---|---|
≥0 | 46 | 100 (90–100) | 0 (0–4) |
≥5 | 45 | 98 (87–100) | 30 (21–40) |
≥10 | 44 | 96 (84–99) | 51 (40–61) |
≥20 | 40 | 87 (73–95) | 72 (62–81) |
≥30 | 37 | 80 (66–90) | 86 (77–92) |
≥40 | 34 | 74 (59–85) | 94 (87–98) |
≥50 | 31 | 67 (52–80) | 96 (89–99) |
≥60 | 27 | 59 (43–73) | 96 (90–98) |
≥70 | 24 | 52 (37–67) | 97 (91–99) |
≥80 | 23 | 50 (36–64) | 99 (94–100) |
≥90 | 18 | 39 (26–56) | 99 (94–100) |
≥100 | 0 | 0 (0–10) | 100 (96–100) |
Abbreviations: CSF-1, colony-stimulating factor 1; IL-6, interleukin; IL-17A, interleukin 17A.
Next, we examined the accuracy of our novel cytokine panel and each of the 3 cytokines alone compared with the routinely available blood biomarkers. Clinically obtained WBC, CRP, and ESR were available for >75% of the cohort and ANC for >50%, as well as research PCT testing for >50%. The 3-cytokine panel performed better than each of the 5 evaluated traditional biomarkers (P < .05 for all) (Table 3). IL-6 and CSF-1 alone each performed better than WBC, ESR, and CRP (P < .05 for all), but not better than PCT or ESR. IL-17A alone did not perform better than any of these routinely available serum biomarkers.
Biomarker Accuracy for Each Traditional Biomarkers as Compared With the Novel Three-Cytokine Biosignature Panel
Test . | AUC (95% CI) . | P Valuea . |
---|---|---|
Biosignature panel | … | … |
IL-6 + IL-17A + CSF-1 | 0.90 (0.84–0.96) | Ref |
Traditional biomarker | … | … |
Absolute neutrophil count (n = 122) | 0.76 (0.66–0.86) | .02 |
C-reactive protein (n = 129) | 0.73 (0.64–0.82) | .004 |
Procalcitonin (n = 74) | 0.72 (0.61–0.84) | .01 |
White blood cell count (n = 130) | 0.71 (0.61–0.81) | .002 |
Erthrocyte sedimentation rate (n = 126) | 0.67 (0.57–0.77) | <.001 |
Test . | AUC (95% CI) . | P Valuea . |
---|---|---|
Biosignature panel | … | … |
IL-6 + IL-17A + CSF-1 | 0.90 (0.84–0.96) | Ref |
Traditional biomarker | … | … |
Absolute neutrophil count (n = 122) | 0.76 (0.66–0.86) | .02 |
C-reactive protein (n = 129) | 0.73 (0.64–0.82) | .004 |
Procalcitonin (n = 74) | 0.72 (0.61–0.84) | .01 |
White blood cell count (n = 130) | 0.71 (0.61–0.81) | .002 |
Erthrocyte sedimentation rate (n = 126) | 0.67 (0.57–0.77) | <.001 |
Abbreviations: AUC, area under the curve; CSF-1, colony-stimulating factor 1; IL-6, interleukin; IL-17A, interleukin 17A.
aComparison with performance of the cytokine biosignature panel.
Biomarker Accuracy for Each Traditional Biomarkers as Compared With the Novel Three-Cytokine Biosignature Panel
Test . | AUC (95% CI) . | P Valuea . |
---|---|---|
Biosignature panel | … | … |
IL-6 + IL-17A + CSF-1 | 0.90 (0.84–0.96) | Ref |
Traditional biomarker | … | … |
Absolute neutrophil count (n = 122) | 0.76 (0.66–0.86) | .02 |
C-reactive protein (n = 129) | 0.73 (0.64–0.82) | .004 |
Procalcitonin (n = 74) | 0.72 (0.61–0.84) | .01 |
White blood cell count (n = 130) | 0.71 (0.61–0.81) | .002 |
Erthrocyte sedimentation rate (n = 126) | 0.67 (0.57–0.77) | <.001 |
Test . | AUC (95% CI) . | P Valuea . |
---|---|---|
Biosignature panel | … | … |
IL-6 + IL-17A + CSF-1 | 0.90 (0.84–0.96) | Ref |
Traditional biomarker | … | … |
Absolute neutrophil count (n = 122) | 0.76 (0.66–0.86) | .02 |
C-reactive protein (n = 129) | 0.73 (0.64–0.82) | .004 |
Procalcitonin (n = 74) | 0.72 (0.61–0.84) | .01 |
White blood cell count (n = 130) | 0.71 (0.61–0.81) | .002 |
Erthrocyte sedimentation rate (n = 126) | 0.67 (0.57–0.77) | <.001 |
Abbreviations: AUC, area under the curve; CSF-1, colony-stimulating factor 1; IL-6, interleukin; IL-17A, interleukin 17A.
aComparison with performance of the cytokine biosignature panel.
Restricting our cohort to children with either pyogenic MSKI or Lyme arthritis, the same 11 cytokines differed significantly between pyogenic MSKI and Lyme arthritis. Using a forward selection model, we identified the following 3-cytokine panel for pyogenic MSKI from Lyme arthritis: IL-17A, IL-6, and IL-1β (Supplementary Table 2).
DISCUSSION
In this study, we assessed the performance of novel cytokine biomarkers to distinguish children with pyogenic MSKIs from those with Lyme or inflammatory arthritis. We found that IL-6 alone and a novel 3-cytokine panel incorporating IL-6, IL-17A, and CSF-1 demonstrated high diagnostic accuracy for pyogenic MSKIs. The 3-cytokine panel had higher accuracy than current clinically available diagnostic biomarkers (eg, WBC, ANC, CRP, ESR, and PCT).
Our current diagnostic tools to distinguish pyogenic MSKI from other etiologies of joint pain and swelling in the emergency department have limitatons [1, 5]. A previous septic arthritis clinical prediction rule found that children with a swollen knee are at low risk for septic arthritis if they have both an ANC <10 000 cells/mm3 and an ESR <40 mm/h [2, 3, 22]. A second clinical prediction rule for pyogenic MSKI among children with monoarthritis identified that children with a PCT of 0.50 ng/mL, a CRP of ≥0.6 mg/dL, and a negative Lyme test were not low risk for an MSKI [16]. While sensitive, the lower specificities may lead to overtesting of low-risk children [2, 3, 16, 22]. Prior work evaluating the diagnostic accuracy of traditional blood biomarkers (WBC, CRP, PCT, ANC, and ESR) to identify children with MSKI in Lyme disease–endemic areas found that no single biomarker had sufficient accuracy to be used in isolation [22]. Given these well-recognized limitations in our current diagnostics, additional tools for use in the emergency department setting are needed.
Differential cytokine expression has shown promise for distinguishing bacterial from nonbacterial disease in other pediatric disease states [12, 13, 23, 24]. In a case–control study of children with sickle cell disease and fever, IL-6 was higher in children with serious bacterial infection (eg, bacteremia, pneumonia, and urinary tract infection) than those with viral illness or no infection [12]. In a second study, IL-6 differentiated bacterial from nonbacterial (ie, respiratory syncytial virus and Mycoplasma pneumoniae) pneumonia with high sensitivity (100%) and specificity (99%) [13]. A systematic review and meta-analysis evaluating the utility of IL-6 as a diagnostic marker for bacterial infections in childen found a sensitivity of 73% (95% CI, 61%–82%) and specificity of 76% (95% CI, 61%–87%) [23].
Our work highlights how differential cytokine expression, with particular emphasis on IL-6, IL-17A, and CSF-1, may be useful in the initial diagnosis of pyogenic MSKI. Prior work has demonstrated upregulation of these cytokines in various inflammatory and infectious states [25–27]. While the 3-cytokine panel had a similar AUC as IL-6 or IL-17A alone, the panel performed better than each of the traditional biomarkers. Using a predicted probability of ≥5%, 29 of 97 (29.9%) patients without pyogenic MSKI would have been classified as low risk, potentially obviating the need for imaging and arthrocentesis. In these patients, the 3-cytokine panel could identify children at low risk for a pyogenic MSKI who could safely avoid arthrocentesis or additional cross-sectional imaging. However, the predicted probability of ≥5% was limited by low specificity and a high false-positive rate. Therefore, use of the cytokine panel alone would have resulted in children without pyogenic MSKI being classified as high risk and undergoing unnecessary additional diagnostics including arthrocentesis or MRI. Our findings need additional replication and validation in a larger cohort of children with a suspected pyogenic MSKI. In addition, we must recognize that, in its current form, processing of samples through the Olink platform takes up to 24 hours. Future assay development to operationalize this technology to provide results at the time of clinical decision-making will be important.
At a predicted probability of ≥10%, the 3-cytokine panel misclassified 1 child with osteomyelitis of the metatarsal bone and 1 child with septic arthritis of the elbow. Prior work has demonstrated that traditional inflammatory markers have reduced sensitivity for the detection of pyogenic MSKI in smaller bones, particularly those in the foot [28]. Similarly, prior work has highlighted that septic arthritis of the elbow can be challenging to diagnose due to a paucity of systemic signs and symptoms [29]. While there may be a strong local cytokine response to a bacterial infection of the foot or elbow, circulating levels of cytokines in the blood may be lower, thereby limiting discriminative ability of plasma biomarkers. Future studies focused on children with concern for pyogenic MSKIs of the knee or hip may better delineate the diagnostic accuracy of cytokines.
Our study has several limitations. First, our sample size was small, and the findings should be considered exploratory. However, we did include children enrolled at multiple participating centers, improving generalizability. Second, each of the centers in the suspected Lyme arthritis parent cohort was located in a Lyme-endemic region. Although we included 2 additional centers not located in Lyme disease–endemic areas, more studies are needed to determine model accuracy in non-Lyme-endemic or community settings. Third, we were unable to determine the final diagnoses for all of the children with other inflammatory arthritis. However, in our subgroup analysis after excluding these children, the same 11 cytokines identified children with pyogenic MSKIs. Last, our findings have not yet been independently validated. Further study is needed before clinical application of our findings.
In our multicenter study of children undergoing evaluation for suspected MSKI, we demonstrate how serum cytokines may assist clinicians evaluating children with joint pain and swelling to distinguish MSKI from Lyme or inflammatory arthritis. Specifically, we identified a novel 3-cytokine panel including IL-6, IL17-A, and CSF-1 for MSKI, although further validation and optimization of sensitivity and specificity are needed. Ultimately, a multivariate clinical prediction rule that incorporates cytokine data, traditional blood biomarkers, history, and physical examination may more accurately identify children with pyogenic MSKI at the time of initial presentation.
Supplementary Data
Supplementary materials are available at Open Forum Infectious Diseases online. Consisting of data provided by the authors to benefit the reader, the posted materials are not copyedited and are the sole responsibility of the authors, so questions or comments should be addressed to the corresponding author.
Acknowledgments
Author contributions. A. T. Geanacopoulos and L. E. Nigrovic obtained funding, designed the study, performed the data analysis, drafted the initial manuscript, and critically reviewed and revised the manuscript. P. Y. Lee obtained funding, designed the study, assisted in data acquisition and data analysis, and critically reviewed and revised the manuscript. T. W. Lyons contributed to study design, oversaw data analysis, performed data acquisition, and critically reviewed and revised the manuscript. K. E. Brodeur and R. K. Aresco performed data acquisition and critically reviewed and revised the manuscript. M. C. Monuteaux contributed to study design, assisted in the data analysis, and critically reviewed and revised the manuscript. R. L. Kaplan and A. T. Cruz supervised patient enrollment, contributed to study design, and critically reviewed and revised the manuscript. P. A. Nigrovic obtained funding, contributed to study design, and critically reviewed and revised the manuscript.
Financial support. This work was supported by the Agency for Healthcare Research and Quality (T32HS000063) to A.T.G., the Joint Biology Consortium (National Institutes of Health/National Institute of Arthritis and Musculoskeletal and Skin Diseases 5P30 AR070253) to A.T.G., P.A.N., and L.E.N. including a JBC Microgrant to A.T.G., the Peabody Foundation to P.Y.L., P.A.N., and L.E.N., the Global Lyme Alliance to L.E.N., the Boston Children's Hospital Medical Staff Organization Faculty Innovated Research Award to T.W.L., the American Academy of Pediatrics Ken Graff Young Investigator Award to T.W.L., and the Boston Children's Hospital Institutional Centers for Clinical and Translational Research Population Health Pilot Grant to T.W.L.
References
Author notes
Potential conflicts of interest. The authors have no conflicts of interest relevant to this article to disclose.
Comments