-
PDF
- Split View
-
Views
-
Cite
Cite
Stephan Maurer, Ferdinand Rauch, Economic geography aspects of the Panama Canal, Oxford Economic Papers, Volume 75, Issue 1, January 2023, Pages 142–162, https://doi.org/10.1093/oep/gpac009
- Share Icon Share
Abstract
This paper studies how the opening of the Panama Canal in 1914 changed counties’ market potential and influenced the economic geography of the USA. We compute shipment effective distances with and without the canal from each US county to each other US county and to international ports and compute the resulting change in market potential. The main elasticity would imply that a 1% increase in market potential led to a total increase of population by around 2.3% in 1940. We compute similar elasticities for wages, land values, and immigration from out of state. Tradable (manufacturing) industries react stronger than non-tradable (services) industries.
1. Introduction
The effect of changes in trading opportunities on the spatial equilibrium is an old question in the economic literature. It is of practical importance to policy makers that consider investing in transportation infrastructure. Typically, this question is addressed in case studies that consider the effects of railroads, highways, ports, and other changes in transport infrastructure. Here, we use the opening of the Panama Canal in 1914 and see how it influenced the economic geography of the USA in following decades. The opening of the canal was one of the largest changes to international shipment distances, leading to sizable changes of market potential for many US counties, while at the same time giving much variation of the degree of this change within the USA. The opening took place at a time when international trade overwhelmingly happened by ship, which makes this change in distances a more precise measure for trade than it would be in later decades.
Contributions of this project include the following four: First, we build a dataset of international and domestic market potential for US counties for around 1900 that may be useful for other studies. We also measure the change in market potential induced by the Panama Canal for each US county. Second, we show that there is a strong positive causal effect of market potential change on population growth throughout the 20th century. Our main magnitude implies that an increase of market potential of 1% increases population by 2.3%. This is an elasticity estimate that may be of use elsewhere. We also provide related numbers for manufacturing wages, agricultural land prices, and immigration from out of state, and show that all these react positively to increased market potential. Third, we show that this effect is present both for tradable and non-tradable industries overall, with tradable workers reacting stronger. Employment in agriculture, on the other hand, does not react at all. Finally, we use economic theory to provide a cost–benefit analysis of the canal that suggests that the canal increased US welfare and seems to pass a cost–benefit test easily.
The basis of our dataset is an existing 20-year frequency county panel for the USA from 1880 to 2000 (Michaels et al., 2012). This dataset also allows us to consider total population growth and employment growth in agriculture, manufacturing, and services separately. We add the year 1910 to this dataset. We combine these data with US domestic transport costs in 1890 (Donaldson and Hornbeck, 2016) and data on the location and population of major international ports at the turn of the centuries (Pascali, 2017). We compute shipment effective distances with and without the canal from each US county to each other US county and to each international port. We then use a gravity-type framework to compute market potential measures that are distance weighted population measures for every county.1
A first set of results describes the impact of the canal. Using our preferred set of parameters, the canal decreases the distance between about 3% of US county pairs. Nearly all counties have some improvement in international market potential, with much variation of the magnitude of the change throughout the country. On average, US counties experience a 2.9% total market potential improvement.
Looking at results over time, we find that the canal had no effect on population growth of counties in the placebo period before its opening, from 1880 to 1900, and no clear pattern emerges until 1920. A significant positive effect only arises afterwards, which is consistent with the full opening of the canal in 1920. The sample is large enough that we can consider heterogeneous effects. When we non-parametrically decompose the effect by initial density deciles, we find that there is a stable relationship between improved market potential and population growth, with no large difference for small or large counties. We also find that the effect rises with treatment intensity. Our results are robust to various ways of calculating market potential changes.
This paper relates to a large literature on the relationship between trade and growth (Frankel and Romer, 1999; Redding and Venables, 2004; Ortega and Peri, 2014; Pascali, 2017; Donaldson, 2018; Bakker et al., 2020; Ellingsen, 2020; Heblich et al., 2020). Our setting is particularly close to Feyrer (2009), who studies the impact of the closure of the Suez Canal following the Six Day War, and to Hugot and Umana Dajud (2016), who examine the effects of the Panama and Suez canals for international trade. Our paper differs in that we consider effects within a country rather than across countries and that we study a permanent change over a longer time horizon. In this sense, our paper also relates to the literature on location fundamentals and cities’ long-term development (Davis and Weinstein, 2002; Bleakley and Lin, 2012; Bosker and Buringh, 2017; Hanlon, 2017; Michaels and Rauch, 2018). Umana Dajud (2017) studies the impact of the Panama Canal in Canadian municipalities. Apart from the obvious difference of studying a different country, our paper differs in four substantial ways from his. First, while Umana Dajud (2017) defines treatment based on least-cost paths to the key ports of Vancouver and Montreal, we explicitly compute and quantify market potential changes for every county. Second, we consider changes in the industrial structure and migratory responses as additional outcomes. Third, the two papers have different geographical coverage. Umana Dajud (2017) uses municipalities with 10,000 inhabitants or more, while we employ a geographically larger panel of nearly all mainland US counties, thus also including rural regions. Finally, while the focus in Umana Dajud (2017) is on Canadian inter-coastal traffic, our market potential calculations also include international destinations.
Related are also papers that examine the role of trading opportunities in determining city growth. Redding and Sturm (2008) study the effect of the Iron Curtain on the development of towns in West Germany. What we add to their findings is a more precise measurement of market potential changes in our setting. A paper by Donaldson and Hornbeck (2016) on the effects of railroads is closely connected in data, econometric setup, and research question. In a broader sense, our paper is also connected to several other papers that have looked at the growth implications of infrastructure measures that improve trading opportunities. In this literature, railroads have received particular attention,2 but so have roads and highways (Banerjee et al., 2012; Duranton and Turner, 2012; Faber, 2014; Baum-Snow et al., 2019; Jedwab and Storeygard, 2020; Herzog, 2021), air links (Campante and Yanagizawa-Drott, 2018), and ports (Ducruet et al., 2020). Our findings and the magnitudes of the effects we report may contribute to a recent theoretical literature on the spatial effects of trade (e.g., Fajgelbaum and Redding, 2014; Cosar and Fajgelbaum, 2016; Fajgelbaum and Gaubert, 2018; Potlogea, 2018; Bakker 2019). Finally, we also contribute to a literature on the economic effects of the Panama Canal (Huebner, 1915; Hutchinson and Ungo, 2004; Maurer and Yu, 2008; Maurer and Yu, 2010; Umana Dajud, 2017) by adding our own measures and estimates.
The next section will give a brief overview of the history of the Panama Canal and present some facts on its usage today. Section 3 describes the dataset we assemble for this project. Section 4 presents the main regression results. Section 5 presents a few robustness checks on the main results. Section 6 uses the results in combination with a theoretical model to produce an estimate of the welfare contribution to the USA by the canal and uses it in a cost–benefit analysis. Section 7 concludes.
2. The Panama Canal
The idea to connect the Atlantic and the Pacific to facilitate trade is old. A priest by the name of Francisco López de Gómara drew an optimistic plan to dig a canal in the area for the King of Castile already in 1552. A glance at a world map shows that the obvious place to dig is in the area of today’s Nicaragua, Costa Rica, or Panama, where the oceans are separated only by a small strip of land. The current canal in Panama is in fact close to the shortest possible passage. Nicaragua was frequently considered as a viable alternative, offering a longer distance but with lower heights to cross. Alexander von Humboldt wrote a study on a canal project in this area in 1811 and likely discussed it with US president Jefferson, another early proponent of this idea.3
An old Spanish trading route existed in the area of today’s canal from possibly the 16th century. This heavily used path was developed by private entrepreneurs into a railway line connecting the oceans that opened in 1855. This railway line consisted of 76 km of track and connected with ships at either end. This railway line benefitted from the gold rush in California, and helped transport people to California, and gold the other way. In the 19th century, it had the heaviest volume of freight of any railroad in the world. At some point, its parent company was the highest priced stock listed on the New York Stock exchange. Yet despite its great success, the railway line had severe shortcomings, essentially excluding bulky or heavy goods trade. It was not a useful substitute for a proper canal and the idea to dig remained a consideration for the US government and others. President Ulysses S. Grant remarked in 1881 ‘To Europeans the benefits of and advantages of the proposed canal are great, to Americans they are incalculable’ (cited in McCullough and Herrmann, 1977, p. 26).
Yet despite the great importance of the canal to the USA, it was a Frenchman who pioneered this project. After playing the central role in the construction of the Suez Canal, French diplomat Ferdinand de Lesseps founded the ‘Panama Canal Company’, obtained the rights to dig from the Colombian government (at the time Panama was part of Colombia), raised private funds, and started digging. Construction started in 1882. This project relied primarily on workers from the West Indies as well as French engineers, but also sourced moderate amounts of supplies and workers from the USA. The company underestimated the difficulties that a combination of yellow fever, malaria, tropical climate, and remoteness presented. It also may have made an error in insisting on building a canal at sea level. The company went bankrupt in 1889. About 20,000 workers, mainly from the West Indies, died while working for the French company, primarily from malaria and yellow fever. Many French families lost money following the bankruptcy.
The project was abandoned, until President Theodore Roosevelt made it a priority and revived it. He declared in his first message to congress in 1901: ‘No single great material work [..] is of such consequence to the American people’. The US government bought the remains of the French company and continued construction from 1904. The USA encouraged a revolution in Panama and prevented the Colombian government from interfering. This created the country of Panama and secured control over the canal for the US government in its first decades. The canal was completed and opened in 1914. However, strikes, landslides, and the effects of World War I prevented the full opening for civilian traffic until July 1920 (Maurer and Yu, 2010). Table 1 gives an overview over the cargo that passed through the canal. It illustrates that there was sizable cargo transit immediately after the canal’s opening, but also how the amount of cargo increased considerably after 1920: Between 1915 and 1925, the annual cargo passing through the canal quintupled.
Fiscal year . | Number of vessels . | Tons of cargo (millions) . |
---|---|---|
1915 | 1,075 | 4.9 |
1916 | 758 | 3.1 |
1917 | 1,803 | 7.1 |
1918 | 2,069 | 7.5 |
1919 | 2,024 | 6.9 |
1920 | 2,478 | 9.4 |
1921 | 2,892 | 11.6 |
1922 | 2,736 | 10.9 |
1923 | 3,967 | 19.6 |
1924 | 5,230 | 27.0 |
1925 | 4,673 | 24.0 |
1926 | 5,197 | 26.0 |
1927 | 5,475 | 27.7 |
1928 | 6,456 | 29.6 |
1929 | 6,413 | 30.7 |
Fiscal year . | Number of vessels . | Tons of cargo (millions) . |
---|---|---|
1915 | 1,075 | 4.9 |
1916 | 758 | 3.1 |
1917 | 1,803 | 7.1 |
1918 | 2,069 | 7.5 |
1919 | 2,024 | 6.9 |
1920 | 2,478 | 9.4 |
1921 | 2,892 | 11.6 |
1922 | 2,736 | 10.9 |
1923 | 3,967 | 19.6 |
1924 | 5,230 | 27.0 |
1925 | 4,673 | 24.0 |
1926 | 5,197 | 26.0 |
1927 | 5,475 | 27.7 |
1928 | 6,456 | 29.6 |
1929 | 6,413 | 30.7 |
Note: The fiscal year ends on June 30 of the respective calendar year.
Source: Department of Commerce (1930, p. 442).
Fiscal year . | Number of vessels . | Tons of cargo (millions) . |
---|---|---|
1915 | 1,075 | 4.9 |
1916 | 758 | 3.1 |
1917 | 1,803 | 7.1 |
1918 | 2,069 | 7.5 |
1919 | 2,024 | 6.9 |
1920 | 2,478 | 9.4 |
1921 | 2,892 | 11.6 |
1922 | 2,736 | 10.9 |
1923 | 3,967 | 19.6 |
1924 | 5,230 | 27.0 |
1925 | 4,673 | 24.0 |
1926 | 5,197 | 26.0 |
1927 | 5,475 | 27.7 |
1928 | 6,456 | 29.6 |
1929 | 6,413 | 30.7 |
Fiscal year . | Number of vessels . | Tons of cargo (millions) . |
---|---|---|
1915 | 1,075 | 4.9 |
1916 | 758 | 3.1 |
1917 | 1,803 | 7.1 |
1918 | 2,069 | 7.5 |
1919 | 2,024 | 6.9 |
1920 | 2,478 | 9.4 |
1921 | 2,892 | 11.6 |
1922 | 2,736 | 10.9 |
1923 | 3,967 | 19.6 |
1924 | 5,230 | 27.0 |
1925 | 4,673 | 24.0 |
1926 | 5,197 | 26.0 |
1927 | 5,475 | 27.7 |
1928 | 6,456 | 29.6 |
1929 | 6,413 | 30.7 |
Note: The fiscal year ends on June 30 of the respective calendar year.
Source: Department of Commerce (1930, p. 442).
In this paper, we compare a world with the canal to one without the canal. When do we expect to see a difference between the two? There could have been some small effect of distance to the Panama Canal on trade from the time of the railway in 1850. The years of construction of the Canal from 1904 to 1914 drew some resources and people from the USA to Panama and may account for some small effect. Similarly, there might be anticipatory effects due to the canal’s imminent opening. The canal was then opened partially in 1914, but the full opening did not take place until 1920. We would thus expect 1920 to mark the greatest change to transport costs we observe and we expect to see big effects after this year. The canal continues to become more important throughout the 20th century as international trade increases, as shipping technology improves, shipping volumes increase, and as the destination markets grow, first Europe after the wars, followed by the rise of Asian countries. For these reasons, we expect a continued effect after 1914 and 1920. The effects we report for 1940 are less influenced by these other factors and isolate the gains through the change in distances. Effects for the second half of the 20th century add additional treatments through technology and the growth of destinations and are therefore harder to interpret. For this reason, we typically focus on results up to the year 1940, but we show results for later years in the Online Appendix (Maurer and Rauch, 2022).
3. Data
Our dataset aims to construct effective distances and destination market sizes as they were before the opening of the Canal, around 1900. We do not rely on information on either destination markets or domestic transport costs in the USA after the opening, since both are potentially endogenous to the new transport cost matrix. This implies that our measurement of the market potential induced by the canal is more precise in the earlier decades of the 20th century than in the later ones. For this reason, we usually focus on the time period until 1940. However, in the Online Appendix, we also report estimates over a longer time period.
The Panama Canal facilitates commerce between US coasts, but also between US and international ports. Our measure of market potential change includes both effects. To calculate how the Canal changed international market potential, we draw on a dataset on major ports in the 19th century assembled by Pascali (2017). For every country, this dataset identifies the primary ports in 1850 and assigns them the country’s respective population and GDP data for 1900. We then calculate seaborne least-cost paths and distances from every coastal county in the USA to every major international port, using a 20× 20 km grid of the world and ArcGIS’s ‘Cost Distance’ tool. We calculate these distances under two scenarios: Once with the Panama Canal being closed or not existent and once with it being open.4
For every mainland US county, we then calculate the effective distance from this county’s centroid to every international port. This effective distance consists of two components: The distance from the US coast to the international port calculated before and the effective distance from the respective county to the coast. For the latter, we draw on a 1890 cost matrix provided by Donaldson and Hornbeck (2016). This matrix takes into account railroads, wagon roads, and canals with different cost parameters and therefore gives a precise picture of the effective distance between counties in that period. We restrict the paths from US counties to international ports to go through coastal counties that have a port. For this, we use the list of official ports of entry into the USA as per 1910. According to the Department of Commerce and Labor (1912), there were 109 such ports (not all of them situated on the ocean coast), which we match to our coastal counties where possible.
One of the crucial parameters of this exercise is to define the relative cost of shipment of 1 km inland against 1 km by sea, parametrized as α. This parameter is such that α = 1 would imply that a kilometre of trade over sea costs the same as a kilometre inland, while implies that trading over sea is half the cost of trading over land. Data on freight rates between Cardiff and Port Royal in Pascali (2017) suggest that transporting one ton over a straight-line mile over the ocean cost around 0.15 cents in 1890, or a bit more than 0.09 cents per km. Ocean transport was thus much cheaper than land-based transportation modes. Donaldson and Hornbeck (2016), for example, assume a cost of 0.63 cent per ton-mile for railroad transportation within the USA and even higher values for wagon transportation. We calculate the average Donaldson–Hornbeck cost for a straight-line mile, which is roughly 1.7 cents per ton-mile in 1890. Comparing this with the ocean transportation costs from above suggests an α of around . Even this might be on the conservative end, as Maurer and Yu (2008) estimate a variable cost for a ton-mile over the ocean of only 0.035 cents in 1890 dollars, with implied αs of when using railroads as the comparison and nearly when using the average Donaldson–Hornbeck values. Given these estimates, we use a value of in our preferred specification as a plausible, yet conservative estimate for the cost advantage of ocean-based transportation. We show robustness to including different costs in the effective distance calculation and to using different cost parametrizations.
θ is the elasticity of market potential with respect to effective distance and is the second key parameter. The unit of measurement of is in terms of population per cost-distance. Irrespective of parameter θ, this variable will increase by one if one person with the same GDP per capita as in the USA is added at a cost-distance of one dollar. In line with the tradition of the market potential literature (Harris, 1954), we set parameter θ to –1.
We use the ratio, which gives us a percentage change in market potential and delivers summary statistics that are straightforward to interpret. A second advantage of using a ratio here is that it does not change with the arbitrary unit of distance measurement. Finally, when we later take the log of this variable, it automatically creates differences in log market potential due to the canal.
We then merge this with county-level data on population and employment in three broad industry categories (agriculture, services, and manufacturing) compiled by Michaels et al. (2012). These data are based on the US census and are available at 20-year intervals from 1880 to 2000. They span the whole mainland USA, excluding only North Dakota, Oklahoma, South Dakota, and Wyoming, which had not obtained statehood by 1880. To further study the evolution of population just prior to the canal, we also merge in population data for 1910 from IPUMS (Ruggles et al., 2019). In addition, we also add data on manufacturing wages and land values in 1900 and 1940 from Haines and ICPSR (2010). Land values are measured as the average value of farmland and buildings per acre, manufacturing wages as the ratio of a county’s total manufacturing wage sum and total manufacturing employment. Finally, to shed lights on the potential reallocation of populations across space, we also include data on migration. Unfortunately, the historical US census does not allow us to identify all migrants. However, based on birthplace information, we can at least identify all the people that live in a state different from the one they were born in. Drawing on the full count census records available from IPUMS (Ruggles et al., 2019) for 1900 and 1940, we therefore create the share of a county’s population that was born in a different state. This at least allows us to analyse long-distance migration. We merge the ICPSR and IPUMS data with our main dataset using standard state and county codes, which might lead to some minor measurement error in the resulting outcome variables, as the Michaels et al.’s (2012) counties are created in a consistent way over time and sometimes represent combinations of counties. In addition, we also collect basic geographic control variables such as the longitude and latitude of a county’s centroid.
Table 2 provides summary statistics for these main variables of interest, computed using our preferred parameters.6 As this table shows, the Panama Canal increased market potential by 2.9% for the average US county. Population numbers for the average county reflect population growth of the USA over this period.
. | . | Mean . | Standard deviation . | N . |
---|---|---|---|---|
MP, no canal | 254,882 | 57,824 | 2,425 | |
MP, canal | 262,681 | 61,798 | 2,425 | |
MPCtot | Total MP change as ratio | 1.029 | 0.027 | 2,425 |
pop1880 | 1880 population | 20,598 | 43,188 | 2,425 |
pop1900 | 1900 population | 31,073 | 80,524 | 2,425 |
pop1910 | 1910 population | 35,341 | 101,171 | 2,425 |
pop1920 | 1920 population | 42,093 | 122,008 | 2,425 |
pop1940 | 1940 population | 52,672 | 166,068 | 2,425 |
pop1960 | 1960 population | 71,932 | 245,521 | 2,425 |
pop1980 | 1980 population | 90,641 | 301,078 | 2,425 |
pop2000 | 2000 population | 113,039 | 378,513 | 2,425 |
. | . | Mean . | Standard deviation . | N . |
---|---|---|---|---|
MP, no canal | 254,882 | 57,824 | 2,425 | |
MP, canal | 262,681 | 61,798 | 2,425 | |
MPCtot | Total MP change as ratio | 1.029 | 0.027 | 2,425 |
pop1880 | 1880 population | 20,598 | 43,188 | 2,425 |
pop1900 | 1900 population | 31,073 | 80,524 | 2,425 |
pop1910 | 1910 population | 35,341 | 101,171 | 2,425 |
pop1920 | 1920 population | 42,093 | 122,008 | 2,425 |
pop1940 | 1940 population | 52,672 | 166,068 | 2,425 |
pop1960 | 1960 population | 71,932 | 245,521 | 2,425 |
pop1980 | 1980 population | 90,641 | 301,078 | 2,425 |
pop2000 | 2000 population | 113,039 | 378,513 | 2,425 |
Note: In this table, the abbreviation MP stands for market potential and MPC indicates the ratio of market potential with the canal to market potential without.
Source: Author calculation.
. | . | Mean . | Standard deviation . | N . |
---|---|---|---|---|
MP, no canal | 254,882 | 57,824 | 2,425 | |
MP, canal | 262,681 | 61,798 | 2,425 | |
MPCtot | Total MP change as ratio | 1.029 | 0.027 | 2,425 |
pop1880 | 1880 population | 20,598 | 43,188 | 2,425 |
pop1900 | 1900 population | 31,073 | 80,524 | 2,425 |
pop1910 | 1910 population | 35,341 | 101,171 | 2,425 |
pop1920 | 1920 population | 42,093 | 122,008 | 2,425 |
pop1940 | 1940 population | 52,672 | 166,068 | 2,425 |
pop1960 | 1960 population | 71,932 | 245,521 | 2,425 |
pop1980 | 1980 population | 90,641 | 301,078 | 2,425 |
pop2000 | 2000 population | 113,039 | 378,513 | 2,425 |
. | . | Mean . | Standard deviation . | N . |
---|---|---|---|---|
MP, no canal | 254,882 | 57,824 | 2,425 | |
MP, canal | 262,681 | 61,798 | 2,425 | |
MPCtot | Total MP change as ratio | 1.029 | 0.027 | 2,425 |
pop1880 | 1880 population | 20,598 | 43,188 | 2,425 |
pop1900 | 1900 population | 31,073 | 80,524 | 2,425 |
pop1910 | 1910 population | 35,341 | 101,171 | 2,425 |
pop1920 | 1920 population | 42,093 | 122,008 | 2,425 |
pop1940 | 1940 population | 52,672 | 166,068 | 2,425 |
pop1960 | 1960 population | 71,932 | 245,521 | 2,425 |
pop1980 | 1980 population | 90,641 | 301,078 | 2,425 |
pop2000 | 2000 population | 113,039 | 378,513 | 2,425 |
Note: In this table, the abbreviation MP stands for market potential and MPC indicates the ratio of market potential with the canal to market potential without.
Source: Author calculation.
This has the advantage of omitting the potentially ambiguous years 1910 and 1920 and only comparing a clear pre-canal year with a clear post-canal year. Throughout the analysis, we cluster standard errors at the level of a grid of five by five degrees following Bester et al. (2011) to account for spatial correlation in all our regressions. Figure 1 shows the market potential gain due to the Panama Canal. The map interpolates information from county centroids and darker pixels show areas that benefit more. We condition in this maps on the same set of control variables we use in the regression, such as controls for latitude and longitude, coastal counties, and initial population. In the robustness checks and the Online Appendix, we also report results for specifications where we include state × year effects, time-varying effects of initial market potential levels or of distance to the coast, and other geographic controls. Figure 2 shows the other main variable in this paper, population growth from 1900 to 1940. The scale is in terms of 30 bins of equal size. Darker areas in this figure experienced more population growth.
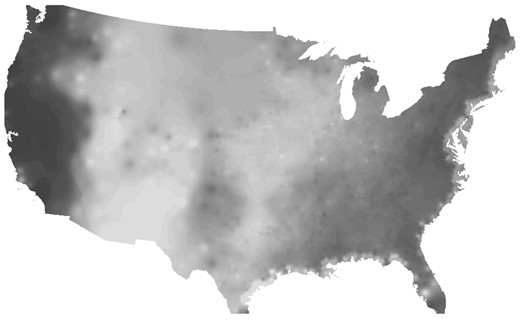
Market potential change impact of the Panama Canal, conditional on linear latitude, longitude, 1880 log population, and an indicator for coastal counties. Interpolation from county centroids. The scale is 30 equally spaced shades of gray. Darker pixels indicate areas that benefit more from the Panama Canal.
Source: Author calculation.
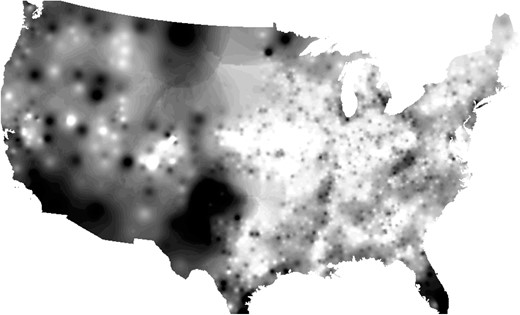
Population growth between 1900 and 1940. Interpolation from county centroids. The scale is in terms of 30 equally spaced shades of gray. Darker pixels indicate areas that experienced more population growth.
Source: Author calculation.
Market potential expressed in units of distance weighted population is a proxy for actual trade flows, which we do not observe directly. Yet, it is a useful proxy: Policy makers that evaluate the consequences of new infrastructure programs such as new railway lines or highways typically can more easily measure the implied market potential changes in units similar to ours than the implied actual trade flow changes. In our discussion of welfare effects below, we show how policy makers can translate the effect into welfare estimations.
4. Results
We start by showing a scatterplot of population growth between 1940 and 1900 and the log market potential change due to the opening of the Panama Canal in Fig. 3. As discussed above, construction of the canal started in 1904, a first opening took place in 1914, and the full opening in 1920. We therefore use 1900 as a clear pre-canal year. 1940 is an end year that gives the canal two decades since its full opening to establish its main effect. As can be seen, there is a strong positive correlation. This is also confirmed by the fitted bivariate regression line, whose parameter estimates are shown in Column 1 of Table 3. The regression coefficient is positive and strongly statistically significant, indicating that counties with improved market potential due to the Panama Canal indeed experience higher population growth. Increasing total market potential by 1% would increase the population in 1940 by 4.1% In Column 2, we additionally control for longitude, latitude, log population in 1880, and an indicator for being a coastal county, which decreases the estimate slightly. In Column 3, we move to our preferred specification, based on the full 60-year panel. We estimate a statistically significant coefficient of around 2.3, implying a long-run elasticity of population with respect to market potential above one.
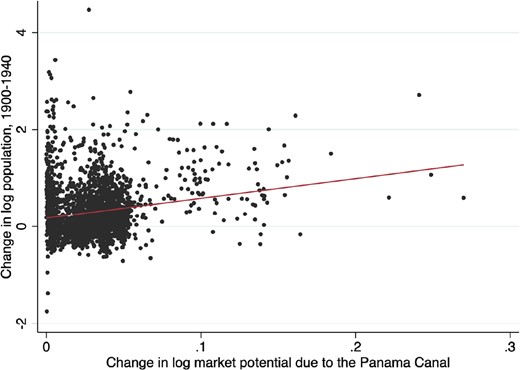
Bivariate correlation between population growth between 1940 and 1900 and the market potential change induced by the opening of the canal.
Source: Author calculation.
The main explanatory variable is log market potential change due to the Panama Canal, in Column 3 interacted with a dummy for the year 1940
. | (1) . | (2) . | (3) . | (4) . |
---|---|---|---|---|
. | Δ lnpop 1940–1900 . | Lnpop . | ||
4.070*** | 3.631*** | 2.303** | ||
(1.313) | (1.175) | (1.058) | ||
0.496 | ||||
(1.932) | ||||
−1.438* | ||||
(0.829) | ||||
0.503 | ||||
(0.612) | ||||
2.193*** | ||||
(0.568) | ||||
Observations | 2,425 | 2,425 | 12,125 | 12,125 |
Specification | Long difference | Long difference | Panel 1880–1940 | Panel 1880–1940 |
Controls | No | Yes | Yes | Yes |
Clusters | 47 | 47 | 47 | 47 |
. | (1) . | (2) . | (3) . | (4) . |
---|---|---|---|---|
. | Δ lnpop 1940–1900 . | Lnpop . | ||
4.070*** | 3.631*** | 2.303** | ||
(1.313) | (1.175) | (1.058) | ||
0.496 | ||||
(1.932) | ||||
−1.438* | ||||
(0.829) | ||||
0.503 | ||||
(0.612) | ||||
2.193*** | ||||
(0.568) | ||||
Observations | 2,425 | 2,425 | 12,125 | 12,125 |
Specification | Long difference | Long difference | Panel 1880–1940 | Panel 1880–1940 |
Controls | No | Yes | Yes | Yes |
Clusters | 47 | 47 | 47 | 47 |
Notes: In Column 4, the omitted reference year is 1920. Control variables are longitude, latitude, log population in 1880, and an indicator for coastal counties. Panel regressions include year- and county-fixed effects and interact the control variables with year dummies. Robust standard errors are clustered using a chessboard grid of 5 × 5 degrees.
p < 0.01,
p < 0.05,
p < 0.1.
Source: Author calculation.
The main explanatory variable is log market potential change due to the Panama Canal, in Column 3 interacted with a dummy for the year 1940
. | (1) . | (2) . | (3) . | (4) . |
---|---|---|---|---|
. | Δ lnpop 1940–1900 . | Lnpop . | ||
4.070*** | 3.631*** | 2.303** | ||
(1.313) | (1.175) | (1.058) | ||
0.496 | ||||
(1.932) | ||||
−1.438* | ||||
(0.829) | ||||
0.503 | ||||
(0.612) | ||||
2.193*** | ||||
(0.568) | ||||
Observations | 2,425 | 2,425 | 12,125 | 12,125 |
Specification | Long difference | Long difference | Panel 1880–1940 | Panel 1880–1940 |
Controls | No | Yes | Yes | Yes |
Clusters | 47 | 47 | 47 | 47 |
. | (1) . | (2) . | (3) . | (4) . |
---|---|---|---|---|
. | Δ lnpop 1940–1900 . | Lnpop . | ||
4.070*** | 3.631*** | 2.303** | ||
(1.313) | (1.175) | (1.058) | ||
0.496 | ||||
(1.932) | ||||
−1.438* | ||||
(0.829) | ||||
0.503 | ||||
(0.612) | ||||
2.193*** | ||||
(0.568) | ||||
Observations | 2,425 | 2,425 | 12,125 | 12,125 |
Specification | Long difference | Long difference | Panel 1880–1940 | Panel 1880–1940 |
Controls | No | Yes | Yes | Yes |
Clusters | 47 | 47 | 47 | 47 |
Notes: In Column 4, the omitted reference year is 1920. Control variables are longitude, latitude, log population in 1880, and an indicator for coastal counties. Panel regressions include year- and county-fixed effects and interact the control variables with year dummies. Robust standard errors are clustered using a chessboard grid of 5 × 5 degrees.
p < 0.01,
p < 0.05,
p < 0.1.
Source: Author calculation.
Our estimate is essentially a difference-in-differences estimator with a continuous treatment, given by the change in log market potential due to the canal. Given our panel, we can estimate ‘leads’ of this treatment to analyse whether population already changed systematically with future market potential change before the Canal was opened. This is done in Column 4, where instead of one Post Opening dummy, we interact the log market potential change with dummies for 1880, 1900, 1910, and 1940, the omitted reference category being 1920. We find that the market potential change due to the canal did not have an effect on population in 1880 and if anything a slight negative effect in 1900. This is reassuring, as both periods are essentially a ‘placebo check’: The canal was not open in this period and US construction had not yet started, so we would not expect to find an effect of canal-induced market potential change on population growth over those years. The effect continues to fluctuate in the years 1910 and 1920, when the canal was being constructed, and it is only in 1940, 20 years after the canal’s definitive opening, that we find a strong and positive effect of the change. In line with a causal interpretation of our results, market potential change thus only affects population in the period that experienced the impact of the finished canal.
Several recent contributions show that the effect of market potential on subsequent city growth can vary with initial city size. For example, Baum-Snow et al. (2019) find that the benefits of highway expansion in China accrued mostly to more important cities. Jedwab and Storeygard (2020), on the other hand, find that road upgrading in Africa has benefitted smaller and remote cities more. A natural question thus is whether the effects of the Panama Canal also varied with initial county population. To analyse this, we divide the counties into deciles according to their 1880 population. We then perform difference regressions for 1900–1940 on interactions of (log) total market potential changes with these deciles, controlling for the direct population effects. The resulting coefficients by initial decile are shown in Fig. 4. We do not find much evidence for heterogeneity along this dimension. Coefficients do not appear very different along the initial population distribution, the one exception being a somewhat lower effect for the initially most populated counties.
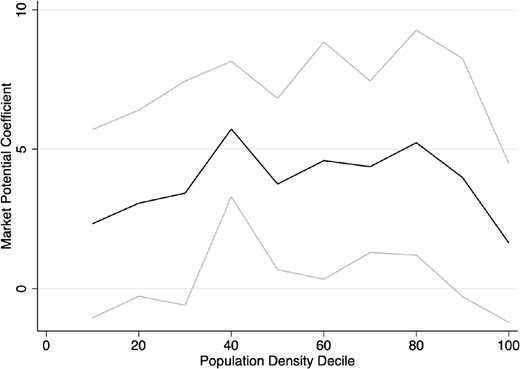
Effect by initial population. The graph shows regression coefficients of 10 indicator variables for 10 initial population deciles interacted with the main treatment effect. The gray lines show 95% confidence intervals.
Source: Author calculation.
A related concern is that the effect may be non-linear in treatment intensity. To analyse this, we run another non-parametric specification, in which we replace the total market potential change variable by 10 decile indicator variables for the intensity of treatment. Coefficients and 95% confidence intervals of this exercise are displayed in Fig. 5. The figure suggests that the treatment effect increases relatively monotonously in treatment intensity, though there is a jump around median values of market access change.
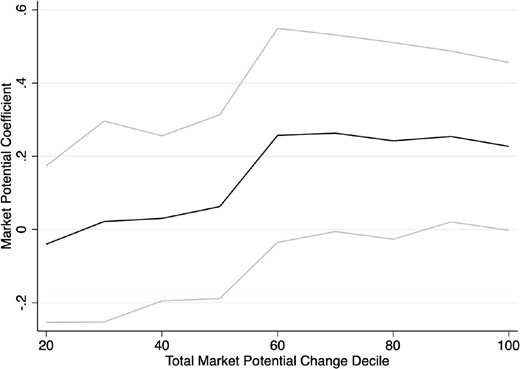
Effect by market potential change. The graph shows regression coefficient of nine indicator variables for nine treatment intensity deciles and the omitted reference category being the lowest decile of market potential change. The gray lines show 95% confidence intervals.
Source: Author calculation.
In Table 4, we analyse how the opening of the Panama Canal affected the sectoral composition of local economies. For this, we use data from Michaels et al. (2012) on employment in three broad economic sectors: agriculture, manufacturing, and services. We focus on the 1900–1940 ‘long difference’ specification akin to Column 2 of Table 3. The only change is that we additionally add control variables for manufacturing and services sectoral shares in 1880, since initial industry shares are likely to influence sectoral developments. Columns 1 and 2 show that both manufacturing and service sector employment reacted strongly to the opening of the canal. Agricultural employment, on the other hand, does not seem to be affected at all.
. | 1940–1900 log difference in employment in . | ||
---|---|---|---|
. | Manufacturing . | Services . | Agriculture . |
8.199*** | 4.621*** | −0.798 | |
(2.088) | (1.352) | (0.997) | |
Observations | 2,383 | 2,417 | 2,423 |
Clusters | 47 | 47 | 47 |
. | 1940–1900 log difference in employment in . | ||
---|---|---|---|
. | Manufacturing . | Services . | Agriculture . |
8.199*** | 4.621*** | −0.798 | |
(2.088) | (1.352) | (0.997) | |
Observations | 2,383 | 2,417 | 2,423 |
Clusters | 47 | 47 | 47 |
Notes: All regressions control for longitude, latitude, log 1880 population, an indicator for coastal counties, and employment shares in manufacturing and services in the 1880. Standard errors, clustered on a 5×5 degree grid, in parentheses. ***p < 0.01, **p < 0.05, *p < 0.1.
Source: Author calculation.
. | 1940–1900 log difference in employment in . | ||
---|---|---|---|
. | Manufacturing . | Services . | Agriculture . |
8.199*** | 4.621*** | −0.798 | |
(2.088) | (1.352) | (0.997) | |
Observations | 2,383 | 2,417 | 2,423 |
Clusters | 47 | 47 | 47 |
. | 1940–1900 log difference in employment in . | ||
---|---|---|---|
. | Manufacturing . | Services . | Agriculture . |
8.199*** | 4.621*** | −0.798 | |
(2.088) | (1.352) | (0.997) | |
Observations | 2,383 | 2,417 | 2,423 |
Clusters | 47 | 47 | 47 |
Notes: All regressions control for longitude, latitude, log 1880 population, an indicator for coastal counties, and employment shares in manufacturing and services in the 1880. Standard errors, clustered on a 5×5 degree grid, in parentheses. ***p < 0.01, **p < 0.05, *p < 0.1.
Source: Author calculation.
A simplification that may have some justification in the earlier parts of the 20th century would be to call manufacturing industries the tradable sector and the service sector the non-tradable sector. This simplification is less justified in later years, when services become increasingly traded. But why would non-tradable jobs react to changes in market potential? One explanation might be that tradable workers cause local demand, which in turn attracts workers in the non-tradable sector. Estimates suggest that one additional tradable job creates 0.8 (Van Dijk, 2015), 1.5 (Moretti, 2010), or 1.6 (Van Dijk, 2017) non-tradable jobs. Given these estimates, we would expect non-tradable employment to react in a way that is similar than employment in the tradable sector. The finding that the tradable and non-tradable sector reacts to market potential changes in a somewhat similar way may be of interest to theories of spatial economies. Frequently such models nest separate CES indices for tradable and non-tradable sectors in a utility function using a Cobb–Douglas function as aggregator. This would imply constant expenditures for each sector and might for many sets of assumptions on production lead indeed to similar reactions for both sectors due to market potential changes. Our results could give some empirical validation to such a modelling choice.
We consider other outcome variables in Table 5. Regressions here are based on a 1940–1900 long difference specification, except that we use different dependent variables here. In Column 1, we use the log difference in manufacturing wages, as calculated from data in Haines and ICPSR (2010). The relationship is positive and significant. We caution against overstating this result, since the wage measure suffers from a few shortcomings. It represents average manufacturing wages, without adjusting for occupation, education, age, or any other control variable. It also shows nominal wage growth, without taking into account real wage growth that could vary due to changes in house prices. In Column 2, we look at growth in agricultural land values and find a positive and significant relationship, consistent with results by Donaldson and Hornbeck (2016). Column 3 uses the change in the share of population born outside of the state on the left-hand side. Again, the relationship is positive and significant, which suggests that immigration from outside the state contributes to the population growth we find.
The left-hand side variable uses log changes in manufacturing wages (Column 1), land values (Column 2), and the difference in the share of population born outside of the state (Column 3)
. | Manuf. wage growth . | Land value growth . | Immigrant growth . |
---|---|---|---|
. | (1) . | (2) . | (3) . |
. | 1900–1940 . | 1900–1940 . | 1900–1940 . |
1.962*** | 5.120*** | 3.972*** | |
(0.483) | (1.294) | (0.498) | |
Clusters | 47 | 47 | 47 |
Observations | 1,928 | 2,397 | 2,423 |
. | Manuf. wage growth . | Land value growth . | Immigrant growth . |
---|---|---|---|
. | (1) . | (2) . | (3) . |
. | 1900–1940 . | 1900–1940 . | 1900–1940 . |
1.962*** | 5.120*** | 3.972*** | |
(0.483) | (1.294) | (0.498) | |
Clusters | 47 | 47 | 47 |
Observations | 1,928 | 2,397 | 2,423 |
Notes: The right-hand side variables show the log change of total market potential due to the Panama Canal. Regressions control for longitude, latitude, log population in 1880, and an indicator for coastal counties. Robust standard errors are clustered using a 5 × 5 degree chessboard grid.
p < 0.01,
p < 0.05,
p < 0.1.
Source: Author calculation.
The left-hand side variable uses log changes in manufacturing wages (Column 1), land values (Column 2), and the difference in the share of population born outside of the state (Column 3)
. | Manuf. wage growth . | Land value growth . | Immigrant growth . |
---|---|---|---|
. | (1) . | (2) . | (3) . |
. | 1900–1940 . | 1900–1940 . | 1900–1940 . |
1.962*** | 5.120*** | 3.972*** | |
(0.483) | (1.294) | (0.498) | |
Clusters | 47 | 47 | 47 |
Observations | 1,928 | 2,397 | 2,423 |
. | Manuf. wage growth . | Land value growth . | Immigrant growth . |
---|---|---|---|
. | (1) . | (2) . | (3) . |
. | 1900–1940 . | 1900–1940 . | 1900–1940 . |
1.962*** | 5.120*** | 3.972*** | |
(0.483) | (1.294) | (0.498) | |
Clusters | 47 | 47 | 47 |
Observations | 1,928 | 2,397 | 2,423 |
Notes: The right-hand side variables show the log change of total market potential due to the Panama Canal. Regressions control for longitude, latitude, log population in 1880, and an indicator for coastal counties. Robust standard errors are clustered using a 5 × 5 degree chessboard grid.
p < 0.01,
p < 0.05,
p < 0.1.
Source: Author calculation.
5. Robustness checks
The computation of the market potential variables in this paper relies on several assumptions. In Table 6, we assess the robustness of our key population result by varying a few of these assumptions. We always focus on the panel specification for 1880–1940. For ease of comparison, Panel A repeats our baseline result.
. | (1) . |
---|---|
ln(pop) | |
Panel A: Baseline specification | |
2.303** | |
(1.058) | |
Panel B: Do not weight international populations | |
2.687*** | |
(0.926) | |
Panel C: Weight domestic populations | |
2.258** | |
(1.080) | |
Panel D: State FE | |
−0.342 | |
(1.403) | |
Panel E: State trends | |
2.256** | |
(1.077) | |
Panel F: Additional costs | |
3.126** | |
(1.354) | |
Panel G: Additional costs, Maurer and Yu (2008) parameter values | |
3.261* | |
(1.854) | |
Panel H: Geometric domestic distance matrix | |
1.312** | |
(0.599) |
. | (1) . |
---|---|
ln(pop) | |
Panel A: Baseline specification | |
2.303** | |
(1.058) | |
Panel B: Do not weight international populations | |
2.687*** | |
(0.926) | |
Panel C: Weight domestic populations | |
2.258** | |
(1.080) | |
Panel D: State FE | |
−0.342 | |
(1.403) | |
Panel E: State trends | |
2.256** | |
(1.077) | |
Panel F: Additional costs | |
3.126** | |
(1.354) | |
Panel G: Additional costs, Maurer and Yu (2008) parameter values | |
3.261* | |
(1.854) | |
Panel H: Geometric domestic distance matrix | |
1.312** | |
(0.599) |
Notes: Each regression uses the 1880–1940 panel with 12,125 observations (12,120 in panel D) and 47 clusters. All regressions control for period and county-fixed effects and time-varying effects of longitude, latitude, log population in 1880, and an indicator for coastal counties. Standard errors, clustered on a 5×5 degree grid, in parentheses.
p < 0.01,
p < 0.05,
p < 0.1.
Source: Author calculation.
. | (1) . |
---|---|
ln(pop) | |
Panel A: Baseline specification | |
2.303** | |
(1.058) | |
Panel B: Do not weight international populations | |
2.687*** | |
(0.926) | |
Panel C: Weight domestic populations | |
2.258** | |
(1.080) | |
Panel D: State FE | |
−0.342 | |
(1.403) | |
Panel E: State trends | |
2.256** | |
(1.077) | |
Panel F: Additional costs | |
3.126** | |
(1.354) | |
Panel G: Additional costs, Maurer and Yu (2008) parameter values | |
3.261* | |
(1.854) | |
Panel H: Geometric domestic distance matrix | |
1.312** | |
(0.599) |
. | (1) . |
---|---|
ln(pop) | |
Panel A: Baseline specification | |
2.303** | |
(1.058) | |
Panel B: Do not weight international populations | |
2.687*** | |
(0.926) | |
Panel C: Weight domestic populations | |
2.258** | |
(1.080) | |
Panel D: State FE | |
−0.342 | |
(1.403) | |
Panel E: State trends | |
2.256** | |
(1.077) | |
Panel F: Additional costs | |
3.126** | |
(1.354) | |
Panel G: Additional costs, Maurer and Yu (2008) parameter values | |
3.261* | |
(1.854) | |
Panel H: Geometric domestic distance matrix | |
1.312** | |
(0.599) |
Notes: Each regression uses the 1880–1940 panel with 12,125 observations (12,120 in panel D) and 47 clusters. All regressions control for period and county-fixed effects and time-varying effects of longitude, latitude, log population in 1880, and an indicator for coastal counties. Standard errors, clustered on a 5×5 degree grid, in parentheses.
p < 0.01,
p < 0.05,
p < 0.1.
Source: Author calculation.
In our main calculation of market potential, we weighted international populations by their GDP per capita relative to the USA. The rationale behind this was that mobility across the USA would lead to a spatial equilibrium of equal utility levels, but that this could not be assumed for the whole world. One shortcoming of this was, however, that we lose some international destination countries for which we only have population, but not GDP per capita data. In Panels B and C, we probe the sensitivity of our results to this assumption. In Panel B, we do not use GDP weights for international population, treating population the same across the world as within the USA. The coefficient does not change much in either magnitude or statistical significance. In Panel C instead, we also weight domestic populations by their economic strength. Unfortunately, data on GDP or wages of the whole population are not available from the US census for 1880. Instead, we construct weights by drawing on the earnings score variable available from IPUMS. This variable assigns every occupation its %ile rank in the 1950 earnings distribution. It thus tells us whether counties on average have occupations that pay well or badly. We then weight counties by their average occupational earnings score relative to the national average. While this is doubtless a crude measure, it will capture differences in occupational quality across space, for example, due to the industrial structure. Panel C shows that if we use these domestic weights in addition to the GDP weights for international destinations, the estimates are again virtually unchanged.
In Panel D, we additionally introduce state- × year-fixed effects. This is a very demanding specification, as the change in market potential due to the canal of course has a strong geographical component and varies much more across states than within.7 As a result, our results become highly insignificant. We interpret this observation to suggest that variation within state is too subtle to be picked up by this specification. This is also borne out by Panel E, which introduces state-specific linear trends rather than fixed effects, and shows point estimates that are very similar to the baseline. Given that state- × year-fixed effects appear to be a too demanding specification to control for potential geographic confounders, we assess robustness to other such confounders in the Online Appendix.
In Panel F, we add additional costs to the market potential calculation. We impose an additional fixed costs for loading goods from domestic modes of transport to ocean ships. Following Fogel (1964) and Donaldson and Hornbeck (2016), we set this fixed cost for transshipment from one mode of transport to another at 50 cent per ton, corresponding to roughly 57 additional kilometres. Secondly, we add a toll cost to all routes via the Panama Canal. According to Huebner (1915), initial canal tolls were such that a ton of cargo cost around 90 cents, corresponding to 80 cents in 1890 dollars. We therefore add a fixed cost of 80 cents (92 km). A final complication is tariffs. Actual tariffs during our period of analysis depended on the country of origin and the type of good imported, which we both don’t observe and which might be endogenous to trade distances. They also were typically assessed on an ad valorem basis, making it difficult to assign a precise value per average traded ton. In our robustness check, we assign a fixed cost of 5$ (573 km). While this is a simplified approach, it captures the basic idea that tariffs made international trade more costly than domestic one. Judging from examples listed by the Treasury Department (1913), we think this parameter is similar to the tariffs levied on the few goods that were assessed on a per-ton basis, and if anything at the upper end.8 Reassuringly, adding these additional costs does not change our qualitative conclusion and if anything leads to a slightly larger point estimate. In Panel G, we perform a similar exercise, but using different cost parameters that are based on the calculations of Maurer and Yu (2008), who estimate (in terms of 1890 dollars) a cost advantage of sea transport of around 50, as well as a fixed transshipment cost of $1.09 (net of toll costs). The results are very similar to Panel F, albeit a bit less precise.
Finally, in Panel H, we use the same way of calculating effective distances as before, but replace the domestic transport cost matrix from Donaldson and Hornbeck (2016) by a simpler matrix based on geometric distances. This is of course a more crude measure of distance and hence of market potential change. The estimate remains positive and statistically significant, but is lower than our baseline estimate, which is consistent with this measure being less precise.
6. Welfare and cost–benefit analyses
To assess the overall welfare impact of the Panama Canal on the US economy requires the use of a general equilibrium model. In the Online Appendix, we apply a model developed by Redding (2016) to compute welfare gains from the canal. This exercise suggests that the canal led to a 0.2% increase in welfare in the year 1940. The cost of the Panama Canal was 352 million nominal USD in 1914. Using conventional inflation indices, this roughly corresponds to 10 billion USD in 2018 USD. The cost of the canal corresponds to 0.88% of US GDP at the time, which in 1913 was around 40,000 million nominal USD (Maddison, 2007). This exercise suggests that the costs of the canal were greater than the gains realized in 1940. However, when aggregating these annual welfare gains over a few years, the benefits of the canal easily outweigh the costs. There are several caveats to this exercise, which we also discuss in the Online Appendix.
7. Conclusion
This paper presents three main contributions. First, it uses exogenous variation in market potential provided by the opening of the Panama Canal to estimate the elasticity of population with respect to market potential. This elasticity is an important parameter for policy makers that try to evaluate the potential benefits of transport infrastructure such as a new railway or highway. We find that a 1% increase in market potential increased population by around 2.3% in the medium to long run. This sizable elasticity can help explain the well-established fact (Rappaport and Sachs, 2003) that the US population is disproportionately located near the coasts. We also show similar results for the growth of manufacturing wages, agricultural land values, and immigration from out of state. Second, we show that this effect seems to be fairly similar for tradable and non-tradable industries.
Finally, we use a general equilibrium framework to provide a cost–benefit analysis for the Panama Canal, one of the largest infrastructure projects in the history of the USA. It suggests that the canal did indeed increase US welfare and that its benefits outweighed its costs.
Supplementary material
Supplementary material is available on the OUP website. These are the data, replication files, and the Online Appendix.
Funding
This work was not supported by funding outside of our universities.
Footnotes
We use distance weighted population measures as proxy for trade flows that we don’t measure directly. Distance-weighted population is typically also a variable that is observable to policy makers who consider to invest in transport infrastructure.
See, for example, Atack et al.(2010), Hornung (2015), Jedwab and Moradi (2016), Berger and Enflo (2017), Bogart et al.(2018), Donaldson (2018), Büchel and Kyburz (2019), and Braun and Franke (2019).
The historic information in this section draws mainly on Cameron (1971), McCullough (1977), and Maurer and Yu (2010).
Given the global scale of our analysis, and our interest in distances, we use a Azimuthal Equidistant Projection of the world, centred around 39.83N 98.58W, the geographic centre of the USA. The maps we use are based on Manson et al.(2018) for the USA, Bjorn Sandvik’s public domain map on world borders (available from http://thematicmapping.org/downloads/world_borders.php), and the Rivers and lake centreline dataset available from Natural Earth (https://www.naturalearthdata.com/downloads/10m-physical-vectors/10m-rivers-lake-centerlines/).
Data on relative GDP per capita come also from Pascali (2017) and are based on the Maddison Project Database (Bolt and Luiten van Zanden, 2014), an updated version of the Maddison (2004) database.
When decomposed, the change for domestic market potential is 1.006 and the change for international market potential is 1.036. In the world without the canal, weighted domestic market potential is 57,619 and international market potential 197,263. Domestic market potential is considerably lower than international one, which is consistent with the USA being still relatively lightly populated country in 1900. It also should be borne in mind that these values measure market potential and thus trading opportunities, not actual trade flows.
In line with this, when we regress log market potential change on a set of state dummies, the resulting R2 is 86%, illustrating how the state-fixed effects remove most of our identifying variation.
For example, the tariff of 1913 stipulated a rate of 50 cent to $1.50 per ton for clay and earth, 2$ for hay, and 7$ for nitrate of saltpetre (Treasury Department, 1913).
Acknowledgements
We thank many colleagues and participants at multiple seminars and conferences for valuable comments and suggestions. We also thank Luigi Pascali for sharing his dataset of major ports.