-
PDF
- Split View
-
Views
-
Cite
Cite
Katsuhiko Takabayashi, Fumihiko Ando, Kei Ikeda, Hiroshi Nakajima, Hideki Hanaoka, Takahiro Suzuki, Incidence of opportunistic infections in patients with rheumatoid arthritis treated with different molecular-targeted drugs: A population-based retrospective cohort study, Modern Rheumatology, Volume 33, Issue 6, November 2023, Pages 1078–1086, https://doi.org/10.1093/mr/roac133
- Share Icon Share
ABSTRACT
We compared the incidences of four opportunistic infections (OIs) in patients with rheumatoid arthritis (RA) treated with molecular-targeted drugs from big claims data.
We identified 205,906 patients with RA who were prescribed molecular-targeted drugs in 2010–17 from the National Database of Japan and calculated the incidence of four OIs (Pneumocystis pneumonia, tuberculosis, nontuberculous mycobacterial infection, and herpes zoster).
The total number of Pneumocystis pneumonia, tuberculosis, nontuberculous mycobacterial infection, and herpes zoster patients with biological disease-modifying antirheumatic drugs or tofacitinib treatment history in RA was 765, 1158, 834, and 18,336, respectively. The incidence rates of each OI for all biological disease-modifying antirheumatic drugs were 0.14, 0.14, 0.09, and 2.40 per 100 person-years, respectively, while for tofacitinib they were 0.22, 0.22, 0.07, and 7.00 per 100 person-years. No big difference was observed among biological disease-modifying antirheumatic drugs. All OIs showed higher incidence in those >65 years, but Pneumocystis pneumonia, nontuberculous mycobacterial infection, and herpes zoster showed no difference between those 65–74 years old and those >75 years old. The median of occurrence was the third, seventh, ninth, and thirteenth month after treatment, respectively.
We counted real incidence rates of OIs for the whole nation from big claims data.
Introduction
Treatment of rheumatoid arthritis (RA) with biological disease-modifying antirheumatic drugs (bDMARDs) and Janus kinase inhibitors (JAKi) has brought a substantial improvement in the ability to suppress disease activity and control the natural progression of the disease [1]. Despite the advantages of the introduction of these molecular-targeted drugs for RA patients, there is a concern that they could generate immunological alterations in the context of immunosuppressive therapy [2–4]. In particular, RA patients on molecular-targeted treatment have shown an increased risk of opportunistic infections (OIs), such as Pneumocystis pneumonia (PCP), tuberculosis (TB), nontuberculous mycobacterial infection (NTM), and herpes zoster (HZ).
To date, there have been many reports of OIs related to bDMARDs. A meta-analysis of 70 clinical trials identified an overall increased risk of OIs (odds ratio = 1.79 compared with control patients) in patients treated with bDMARDs [5]. However, most of the data are from limited facilities or short time periods within 1 year, and reports covering the whole country or OIs over the long term are scarce [6, 7]. Considering that many OIs occurred in individuals who had previously been on a biological agent for over 1 year, these cases might not have been detected in many clinical trials with shorter follow-up periods.
In this study, we used a large, nationwide database that included claims data for >120 million people in the entire county over 7 years and described the incidence of these infections among bDMARD and JAKi patients. The National Database of Health Insurance Claims and Specific Health Checkups of Japan (NDB) is a collection of electronic claims data of both inpatient and outpatient for all Japanese citizens (126,706,000 people as of October 2017), except for those on welfare public assistance (2,145,415 people as of March 2017, accounting for 1.7% of the population). Japanese citizens have been universally covered by the universal insurance since 1961 and in 2010, 93.7% of all medical institutions, including 99% of all hospitals, issue insurance claims electronically, which means we could collect digital claims data of almost all Japanese citizens. As a result, the NDB is one of the largest and most comprehensive claims databases worldwide, providing a valuable resource for researchers to analyse real-world practice without sampling [8]. By using this large database with the International Classification of Diseases 10th Revision (ICD-10) codes, we can classify and calculate the number of the patients in the country. In terms of RA, there have already been a few studies using the NDB for basic surveys of the RA population in Japan [9] and for geographic variations of the treatment applied [10, 11]. We also reported the head-to-head comparison of retention rates between molecular-targeted drugs [12] and the seasonal change of initiation of bDMARDs in Japan [13] using the NDB.
The present study aimed to clarify the incidence of OIs in patients with RA treated with molecular-targeted drugs for the whole country in the long-term real-world setting by using NDB data.
Methods
System design
Using NDB data, we compared the incidence of OIs (PCP, TB, NTM, and HZ) in RA patients treated with bDMARDs and JAKi.
Setting
The whole Japanese population is taken as a cohort study.
Data collection
This study was reviewed and approved by the Ministry of Health, Labor, and Welfare (MHLW, approval number 0161). We extracted data related to claims for the prescription of molecular-targeted drugs between April 2010 and March 2017 from the NDB provided by MHLW. We included seven bDMARDs: infliximab (IFX), etanercept (ETN), adalimumab (ADA), tocilizumab (TCZ), abatacept (ABT), certolizumab pegol (CZP), and golimumab (GLM); and one JAKi: tofacitinib (TOF) that were marketed in Japan during the study period. Biosimilar IFX was considered equivalent to IFX. No other biosimilars for RA were on the market during the study period in Japan, nor were baricitinib, sarilumab, peficitinib, or upadacitinib. All ICD-10 codes used to extract claims for these drugs and diseases related to RA were mentioned in our previous paper [12].
Data processing and patient selection
After identification of potentially eligible monthly claims, we grouped claims from the same patient together by identifying patients based on two unique hash values: one derived from the insured’s identification number and the other from name and date of birth [14]. We included patients with at least one definitive diagnosis ICD-10 code for RA. Four exclusion criteria include one for the existence of other diseases and three for outliers. If other diseases such as ulcerative colitis exist as well as RA in the claim, the patient may be treated for ulcerative colitis and not for RA. Thus, we exclude those cases. We also excluded outliers such as simultaneous prescription of three or more different molecular-targeted drugs within 1 month, simultaneous prescription of two or more different molecular-targeted drugs for three or more consecutive months, and alternate prescription of two different molecular-targeted drugs for six or more consecutive months.
We identified 205,906 patients with RA who were prescribed at least one of the eight molecular-targeted drugs (IFX, ETN, ADA, TCZ, ABT, CZP, GLM, and TOF) between April 2010 and March 2017 from the NDB covering 98.3% (or 126,706,000 people) of the Japanese population [12]. To define naïve cases, we set a 1-year wash-out period before molecular-targeted agent treatment (MTAT).
Then, we counted the occurrence of four OIs—PCP, TB, NTM, and HZ—by detecting the drug treatment specific for each infection in the claims data and calculated the incidence of each. Each OI was identified here by the specific drug treatment in addition to the ICD-10 code of diagnosis in the claims data. PCP was treated with more than six sulfamethoxazole-trimethoprim (ST) tablets a day or pentamidine isethionate (PM) 200 mg for >3 days in a month. TB was treated with a combination of at least two drugs from the list: rifampicin (RFP), ethambutol (EB), isoniazid (INH), streptomycin (SM), and pyrazinamide (PZA). For NTM, clarithromycin (CAM) was added as well as the same antitubercular drug combination. As for HZ, one of the following treatments was required: acyclovir at >3000 mg a day, valaciclovir at >2000 mg a day, famciclovir at >1000 mg a day, or injectable vidarabine. Amenamevir was not commercialized until 2017 in Japan (Table 1).
OI . | Drug . | Patients . | |||||
---|---|---|---|---|---|---|---|
PCP | ST | PM | |||||
+ | 767 | ||||||
+ | 185 | ||||||
TB | RFP | EB | INH | SM | PZA | ||
+ | + | 602 | |||||
+ | + | + | + | 527 | |||
+ | + | + | 518 | ||||
+ | + | 338 | |||||
+ | + | 59 | |||||
+ | + | + | 41 | ||||
+ | + | + | 41 | ||||
+ | + | + | + | 31 | |||
+ | + | + | + | + | 29 | ||
+ | + | + | 27 | ||||
+ | + | + | 24 | ||||
+ | + | + | + | 16 | |||
+ | + | + | 14 | ||||
+ | + | 14 | |||||
+ | + | + | 13 | ||||
+ | + | 12 | |||||
+ | + | 10 | |||||
NTM | RFP | EB | INH | SM | PZA | CAM | |
+ | + | + | 656 | ||||
+ | + | 245 | |||||
+ | + | 133 | |||||
+ | + | 39 | |||||
+ | + | + | 34 | ||||
+ | + | 31 | |||||
+ | + | + | + | 26 | |||
+ | + | + | + | 23 | |||
+ | + | + | 13 | ||||
HZ | VACV | FCV | ACV | VIDA | |||
+ | 15,160 | ||||||
+ | 8731 | ||||||
+ | 1401 | ||||||
+ | 415 |
OI . | Drug . | Patients . | |||||
---|---|---|---|---|---|---|---|
PCP | ST | PM | |||||
+ | 767 | ||||||
+ | 185 | ||||||
TB | RFP | EB | INH | SM | PZA | ||
+ | + | 602 | |||||
+ | + | + | + | 527 | |||
+ | + | + | 518 | ||||
+ | + | 338 | |||||
+ | + | 59 | |||||
+ | + | + | 41 | ||||
+ | + | + | 41 | ||||
+ | + | + | + | 31 | |||
+ | + | + | + | + | 29 | ||
+ | + | + | 27 | ||||
+ | + | + | 24 | ||||
+ | + | + | + | 16 | |||
+ | + | + | 14 | ||||
+ | + | 14 | |||||
+ | + | + | 13 | ||||
+ | + | 12 | |||||
+ | + | 10 | |||||
NTM | RFP | EB | INH | SM | PZA | CAM | |
+ | + | + | 656 | ||||
+ | + | 245 | |||||
+ | + | 133 | |||||
+ | + | 39 | |||||
+ | + | + | 34 | ||||
+ | + | 31 | |||||
+ | + | + | + | 26 | |||
+ | + | + | + | 23 | |||
+ | + | + | 13 | ||||
HZ | VACV | FCV | ACV | VIDA | |||
+ | 15,160 | ||||||
+ | 8731 | ||||||
+ | 1401 | ||||||
+ | 415 |
The running number of the patients for each drug combination is listed. <10 cases are omitted. For PCP and HZ, patients treated with more than the defined dosage of each drug are selected.
OI . | Drug . | Patients . | |||||
---|---|---|---|---|---|---|---|
PCP | ST | PM | |||||
+ | 767 | ||||||
+ | 185 | ||||||
TB | RFP | EB | INH | SM | PZA | ||
+ | + | 602 | |||||
+ | + | + | + | 527 | |||
+ | + | + | 518 | ||||
+ | + | 338 | |||||
+ | + | 59 | |||||
+ | + | + | 41 | ||||
+ | + | + | 41 | ||||
+ | + | + | + | 31 | |||
+ | + | + | + | + | 29 | ||
+ | + | + | 27 | ||||
+ | + | + | 24 | ||||
+ | + | + | + | 16 | |||
+ | + | + | 14 | ||||
+ | + | 14 | |||||
+ | + | + | 13 | ||||
+ | + | 12 | |||||
+ | + | 10 | |||||
NTM | RFP | EB | INH | SM | PZA | CAM | |
+ | + | + | 656 | ||||
+ | + | 245 | |||||
+ | + | 133 | |||||
+ | + | 39 | |||||
+ | + | + | 34 | ||||
+ | + | 31 | |||||
+ | + | + | + | 26 | |||
+ | + | + | + | 23 | |||
+ | + | + | 13 | ||||
HZ | VACV | FCV | ACV | VIDA | |||
+ | 15,160 | ||||||
+ | 8731 | ||||||
+ | 1401 | ||||||
+ | 415 |
OI . | Drug . | Patients . | |||||
---|---|---|---|---|---|---|---|
PCP | ST | PM | |||||
+ | 767 | ||||||
+ | 185 | ||||||
TB | RFP | EB | INH | SM | PZA | ||
+ | + | 602 | |||||
+ | + | + | + | 527 | |||
+ | + | + | 518 | ||||
+ | + | 338 | |||||
+ | + | 59 | |||||
+ | + | + | 41 | ||||
+ | + | + | 41 | ||||
+ | + | + | + | 31 | |||
+ | + | + | + | + | 29 | ||
+ | + | + | 27 | ||||
+ | + | + | 24 | ||||
+ | + | + | + | 16 | |||
+ | + | + | 14 | ||||
+ | + | 14 | |||||
+ | + | + | 13 | ||||
+ | + | 12 | |||||
+ | + | 10 | |||||
NTM | RFP | EB | INH | SM | PZA | CAM | |
+ | + | + | 656 | ||||
+ | + | 245 | |||||
+ | + | 133 | |||||
+ | + | 39 | |||||
+ | + | + | 34 | ||||
+ | + | 31 | |||||
+ | + | + | + | 26 | |||
+ | + | + | + | 23 | |||
+ | + | + | 13 | ||||
HZ | VACV | FCV | ACV | VIDA | |||
+ | 15,160 | ||||||
+ | 8731 | ||||||
+ | 1401 | ||||||
+ | 415 |
The running number of the patients for each drug combination is listed. <10 cases are omitted. For PCP and HZ, patients treated with more than the defined dosage of each drug are selected.
Then, from those cases we chose the ones in which OI occurred after bDMARD or TOF treatment started in 7 years. Next, we selected the naïve cases that have >1-year non-treatment period of molecular-targeted drug from April 2010, calculated their total observation period, and identified the onset of OI to estimate the incidence rate (IR) for each molecular-targeted drug. In addition, we excluded the cases where an OI occurred later than 4 months after the cessation of molecular-targeted treatment, since one prescription can cover 3 months at maximum. Furthermore, to exclude the cases in which infection had already existed when an agent was initiated, we ruled out the cases when prescription for OI was found in previous 1 month for acute and short-term treatment diseases such as PCP and HZ. For chronic diseases like TB and NTM, however, we decided 3 months for non-treatment period of OI. Because if the patient was infected and already treated, the last prescription might be 3 months before. After these selections, we counted the patients who qualified for each definition of OI shown to calculate the IRs. These steps are summarized in Table 2.
. | PCP . | TB . | NTM . | HZ . | Total patients . |
---|---|---|---|---|---|
(1) RA patients with both MTAT and OI in 7 years | 893 | 1347 | 904 | 24,406 | 205,906 |
(2) RA patients with OI which occurred after MTAT in 7 years | 765 | 1158 | 834 | 18,336 | 205,906 |
(3) Naïve patients among (2) cases | 406 | 538 | 437 | 7892 | 121,288 |
(4) Among naïve patients OI occurred during MTAT | 303–311 | 353 | 323 | 5067 | 121,288 |
(5) Among (4) cases eliminated previous OI just before MTAT started | 302 | 300 | 199 | 5051 | 121,288 |
. | PCP . | TB . | NTM . | HZ . | Total patients . |
---|---|---|---|---|---|
(1) RA patients with both MTAT and OI in 7 years | 893 | 1347 | 904 | 24,406 | 205,906 |
(2) RA patients with OI which occurred after MTAT in 7 years | 765 | 1158 | 834 | 18,336 | 205,906 |
(3) Naïve patients among (2) cases | 406 | 538 | 437 | 7892 | 121,288 |
(4) Among naïve patients OI occurred during MTAT | 303–311 | 353 | 323 | 5067 | 121,288 |
(5) Among (4) cases eliminated previous OI just before MTAT started | 302 | 300 | 199 | 5051 | 121,288 |
This table displays the number of patients in each selection step. The first line shows RA patients with MTAT and OI. The second line shows the selected cases where OI occurred after MTAT. In the third line, we selected the cases with a >1-year interval in the beginning as a wash-out period, adapted to naïve cases. The fourth step is the selection of those where OI occurred during MTAT. Finally, in the fifth step, we eliminated the cases where OI occurred just before MTAT. According to the code of NDB, we cannot open the figures <10. Thus, we had to express 303–311 in (4) PCP in order to mask the specific figure of <10 cases that were eliminated in (5) PCP from (4) PCP.
. | PCP . | TB . | NTM . | HZ . | Total patients . |
---|---|---|---|---|---|
(1) RA patients with both MTAT and OI in 7 years | 893 | 1347 | 904 | 24,406 | 205,906 |
(2) RA patients with OI which occurred after MTAT in 7 years | 765 | 1158 | 834 | 18,336 | 205,906 |
(3) Naïve patients among (2) cases | 406 | 538 | 437 | 7892 | 121,288 |
(4) Among naïve patients OI occurred during MTAT | 303–311 | 353 | 323 | 5067 | 121,288 |
(5) Among (4) cases eliminated previous OI just before MTAT started | 302 | 300 | 199 | 5051 | 121,288 |
. | PCP . | TB . | NTM . | HZ . | Total patients . |
---|---|---|---|---|---|
(1) RA patients with both MTAT and OI in 7 years | 893 | 1347 | 904 | 24,406 | 205,906 |
(2) RA patients with OI which occurred after MTAT in 7 years | 765 | 1158 | 834 | 18,336 | 205,906 |
(3) Naïve patients among (2) cases | 406 | 538 | 437 | 7892 | 121,288 |
(4) Among naïve patients OI occurred during MTAT | 303–311 | 353 | 323 | 5067 | 121,288 |
(5) Among (4) cases eliminated previous OI just before MTAT started | 302 | 300 | 199 | 5051 | 121,288 |
This table displays the number of patients in each selection step. The first line shows RA patients with MTAT and OI. The second line shows the selected cases where OI occurred after MTAT. In the third line, we selected the cases with a >1-year interval in the beginning as a wash-out period, adapted to naïve cases. The fourth step is the selection of those where OI occurred during MTAT. Finally, in the fifth step, we eliminated the cases where OI occurred just before MTAT. According to the code of NDB, we cannot open the figures <10. Thus, we had to express 303–311 in (4) PCP in order to mask the specific figure of <10 cases that were eliminated in (5) PCP from (4) PCP.
Claims data are anonymised in the database and information on patient age is provided in classes of 5-year intervals according to the NDB privacy code. In addition, <10 patients cannot be analysed, according to the same code.
Main outcome measures
The main outcome measures were the IRs of four OIs in the RA patients treated with molecular-targeted drugs. The IR of OI for each molecular-targeted drug was counted with naïve cases only when we compared the rates between agents to avoid the influence of predecessor agent. We also classified patients into three age groups: <65, between 65 and 74, and >75 years, and compared each IR. Furthermore, we calculated the median month of onset of OIs after molecular-targeted drug treatment was initiated.
Statistical analysis
Data processing was performed using the Big Data Module developed by NTT Data Mathematical Systems Inc. (Tokyo, Japan). All statistical analyses were performed using JMP®14 (SAS Institute Inc., Cary, NC, USA). IRs of hospitalized infection with 95% confidence intervals (CIs) were calculated by dividing the number of cases by the number of corresponding biologic-exposed 100 person-years (PYs) for each treatment group. The cumulative incidence of hospitalized infection during the 72-month follow-up period for each treatment group was computed from life tables using the Kaplan–Meier approach.
Results
In the 7 years of observation, the incidence of PCP, TB, NTM, and HZ in RA patients after treatment with molecular-targeted drugs was 765, 1158, 834, and 18,336, respectively (Table 2). These figures equate to 109, 165, 119, and 2619, respectively, in 1 year in the whole country. For the calculation of IRs, 121,228 naïve patients with 2,613,399, 2,612,541, 2,611,185, and 2,521,360 years of follow-up, respectively, were compared in this study. The IR of four OIs is summarized in Table 3. The IRs (95% CI) of PCP, TB, NTM, and HZ for all bDMARDs were 0.14 (0.12–0.15), 0.14 (0.12–0.15), 0.09 (0.08–0.11), and 2.40 (2.31–2.44) per 100 PYs, respectively, while for TOF they were 0.22 (0.07–0.63), 0.22 (0.07–0.64), 0.07 (0.01–0.41), and 7.00 (5.70–8.59) per 100 PYs, respectively.
. | . | bDMARDs . | JAKi . | . | |||||||
---|---|---|---|---|---|---|---|---|---|---|---|
. | . | ABT . | ADA . | CZP . | ETN . | GLM . | IFX . | TCZ . | . | TOF . | All . |
PCP | Patients | 17,647 | 14,248 | 4797 | 36,887 | 15,197 | 12,000 | 19,314 | 120,090 | 1182 | 121,272 |
Events | 40 | 44 | 12 | 84 | 30 | 47 | 42 | 299 | <10 | 300–308 | |
IRs (/100PY) | 0.14 | 0.18 | 0.20 | 0.11 | 0.12 | 0.21 | 0.12 | 0.14 | 0.22 | 0.14 | |
95% CI | 0.1–0.18 | 0.13–0.24 | 0.12–0.36 | 0.09–0.14 | 0.09–0.17 | 0.16–0.28 | 0.09–0.16 | 0.12–0.15 | 0.07–0.63 | 0.12–0.16 | |
TB | Patients | 17,622 | 14,241 | 4791 | 36,869 | 15,196 | 11,997 | 19,311 | 120,027 | 1181 | 121,208 |
Events | 36 | 41 | 16 | 82 | 36 | 44 | 42 | 297 | <10 | 298–306 | |
IRs (/100PY) | 0.12 | 0.17 | 0.27 | 0.11 | 0.15 | 0.19 | 0.12 | 0.14 | 0.22 | 0.14 | |
95% CI | 0.09–0.17 | 0.12–0.23 | 0.17–0.44 | 0.09–0.14 | 0.11–0.2 | 0.14–0.26 | 0.09–0.16 | 0.12–0.15 | 0.07–0.64 | 0.12–0.15 | |
NTM | Patients | 17,566 | 14,243 | 4794 | 36,867 | 15,193 | 11,999 | 19,309 | 119,971 | 1180 | 121,151 |
Events | 56 | 20 | <10 | 50 | 17 | 14 | 38 | 196–204 | <10 | 197–205 | |
IRs (/100PY) | 0.19 | 0.08 | 0.05 | 0.07 | 0.07 | 0.06 | 0.11 | 0.09 | 0.07 | 0.09 | |
95% CI | 0.15–0.25 | 0.05–0.13 | 0.02–0.15 | 0.05–0.09 | 0.04–0.11 | 0.04–0.1 | 0.08–0.15 | 0.08–0.11 | 0.01–0.41 | 0.08–0.11 | |
HZ | Patients | 17,609 | 14,227 | 4793 | 36,845 | 15,177 | 11,982 | 19,287 | 119,920 | 1180 | 121,100 |
Events | 737 | 571 | 125 | 1542 | 605 | 533 | 847 | 4960 | 91 | 5051 | |
IRs (/100PY) | 2.6 | 2.4 | 2.2 | 2.2 | 2.6 | 2.4 | 2.5 | 2.4 | 7.0 | 2.4 | |
95% CI | 2.42–2.79 | 2.21–2.6 | 1.83–2.6 | 2.05–2.26 | 2.36–2.77 | 2.24–2.66 | 2.35–2.68 | 2.31–2.44 | 5.7–8.59 | 2.34–2.47 |
. | . | bDMARDs . | JAKi . | . | |||||||
---|---|---|---|---|---|---|---|---|---|---|---|
. | . | ABT . | ADA . | CZP . | ETN . | GLM . | IFX . | TCZ . | . | TOF . | All . |
PCP | Patients | 17,647 | 14,248 | 4797 | 36,887 | 15,197 | 12,000 | 19,314 | 120,090 | 1182 | 121,272 |
Events | 40 | 44 | 12 | 84 | 30 | 47 | 42 | 299 | <10 | 300–308 | |
IRs (/100PY) | 0.14 | 0.18 | 0.20 | 0.11 | 0.12 | 0.21 | 0.12 | 0.14 | 0.22 | 0.14 | |
95% CI | 0.1–0.18 | 0.13–0.24 | 0.12–0.36 | 0.09–0.14 | 0.09–0.17 | 0.16–0.28 | 0.09–0.16 | 0.12–0.15 | 0.07–0.63 | 0.12–0.16 | |
TB | Patients | 17,622 | 14,241 | 4791 | 36,869 | 15,196 | 11,997 | 19,311 | 120,027 | 1181 | 121,208 |
Events | 36 | 41 | 16 | 82 | 36 | 44 | 42 | 297 | <10 | 298–306 | |
IRs (/100PY) | 0.12 | 0.17 | 0.27 | 0.11 | 0.15 | 0.19 | 0.12 | 0.14 | 0.22 | 0.14 | |
95% CI | 0.09–0.17 | 0.12–0.23 | 0.17–0.44 | 0.09–0.14 | 0.11–0.2 | 0.14–0.26 | 0.09–0.16 | 0.12–0.15 | 0.07–0.64 | 0.12–0.15 | |
NTM | Patients | 17,566 | 14,243 | 4794 | 36,867 | 15,193 | 11,999 | 19,309 | 119,971 | 1180 | 121,151 |
Events | 56 | 20 | <10 | 50 | 17 | 14 | 38 | 196–204 | <10 | 197–205 | |
IRs (/100PY) | 0.19 | 0.08 | 0.05 | 0.07 | 0.07 | 0.06 | 0.11 | 0.09 | 0.07 | 0.09 | |
95% CI | 0.15–0.25 | 0.05–0.13 | 0.02–0.15 | 0.05–0.09 | 0.04–0.11 | 0.04–0.1 | 0.08–0.15 | 0.08–0.11 | 0.01–0.41 | 0.08–0.11 | |
HZ | Patients | 17,609 | 14,227 | 4793 | 36,845 | 15,177 | 11,982 | 19,287 | 119,920 | 1180 | 121,100 |
Events | 737 | 571 | 125 | 1542 | 605 | 533 | 847 | 4960 | 91 | 5051 | |
IRs (/100PY) | 2.6 | 2.4 | 2.2 | 2.2 | 2.6 | 2.4 | 2.5 | 2.4 | 7.0 | 2.4 | |
95% CI | 2.42–2.79 | 2.21–2.6 | 1.83–2.6 | 2.05–2.26 | 2.36–2.77 | 2.24–2.66 | 2.35–2.68 | 2.31–2.44 | 5.7–8.59 | 2.34–2.47 |
According to the code of NDB, we cannot open the figures less than 10. Thus, we had to express less than 10 as <10. For the same reason, the total number here cannnot be shown the exact number but expressed as 300–308. If we demonstrate the exact number here, the number of TOF can be calculated, which is less than 10.
. | . | bDMARDs . | JAKi . | . | |||||||
---|---|---|---|---|---|---|---|---|---|---|---|
. | . | ABT . | ADA . | CZP . | ETN . | GLM . | IFX . | TCZ . | . | TOF . | All . |
PCP | Patients | 17,647 | 14,248 | 4797 | 36,887 | 15,197 | 12,000 | 19,314 | 120,090 | 1182 | 121,272 |
Events | 40 | 44 | 12 | 84 | 30 | 47 | 42 | 299 | <10 | 300–308 | |
IRs (/100PY) | 0.14 | 0.18 | 0.20 | 0.11 | 0.12 | 0.21 | 0.12 | 0.14 | 0.22 | 0.14 | |
95% CI | 0.1–0.18 | 0.13–0.24 | 0.12–0.36 | 0.09–0.14 | 0.09–0.17 | 0.16–0.28 | 0.09–0.16 | 0.12–0.15 | 0.07–0.63 | 0.12–0.16 | |
TB | Patients | 17,622 | 14,241 | 4791 | 36,869 | 15,196 | 11,997 | 19,311 | 120,027 | 1181 | 121,208 |
Events | 36 | 41 | 16 | 82 | 36 | 44 | 42 | 297 | <10 | 298–306 | |
IRs (/100PY) | 0.12 | 0.17 | 0.27 | 0.11 | 0.15 | 0.19 | 0.12 | 0.14 | 0.22 | 0.14 | |
95% CI | 0.09–0.17 | 0.12–0.23 | 0.17–0.44 | 0.09–0.14 | 0.11–0.2 | 0.14–0.26 | 0.09–0.16 | 0.12–0.15 | 0.07–0.64 | 0.12–0.15 | |
NTM | Patients | 17,566 | 14,243 | 4794 | 36,867 | 15,193 | 11,999 | 19,309 | 119,971 | 1180 | 121,151 |
Events | 56 | 20 | <10 | 50 | 17 | 14 | 38 | 196–204 | <10 | 197–205 | |
IRs (/100PY) | 0.19 | 0.08 | 0.05 | 0.07 | 0.07 | 0.06 | 0.11 | 0.09 | 0.07 | 0.09 | |
95% CI | 0.15–0.25 | 0.05–0.13 | 0.02–0.15 | 0.05–0.09 | 0.04–0.11 | 0.04–0.1 | 0.08–0.15 | 0.08–0.11 | 0.01–0.41 | 0.08–0.11 | |
HZ | Patients | 17,609 | 14,227 | 4793 | 36,845 | 15,177 | 11,982 | 19,287 | 119,920 | 1180 | 121,100 |
Events | 737 | 571 | 125 | 1542 | 605 | 533 | 847 | 4960 | 91 | 5051 | |
IRs (/100PY) | 2.6 | 2.4 | 2.2 | 2.2 | 2.6 | 2.4 | 2.5 | 2.4 | 7.0 | 2.4 | |
95% CI | 2.42–2.79 | 2.21–2.6 | 1.83–2.6 | 2.05–2.26 | 2.36–2.77 | 2.24–2.66 | 2.35–2.68 | 2.31–2.44 | 5.7–8.59 | 2.34–2.47 |
. | . | bDMARDs . | JAKi . | . | |||||||
---|---|---|---|---|---|---|---|---|---|---|---|
. | . | ABT . | ADA . | CZP . | ETN . | GLM . | IFX . | TCZ . | . | TOF . | All . |
PCP | Patients | 17,647 | 14,248 | 4797 | 36,887 | 15,197 | 12,000 | 19,314 | 120,090 | 1182 | 121,272 |
Events | 40 | 44 | 12 | 84 | 30 | 47 | 42 | 299 | <10 | 300–308 | |
IRs (/100PY) | 0.14 | 0.18 | 0.20 | 0.11 | 0.12 | 0.21 | 0.12 | 0.14 | 0.22 | 0.14 | |
95% CI | 0.1–0.18 | 0.13–0.24 | 0.12–0.36 | 0.09–0.14 | 0.09–0.17 | 0.16–0.28 | 0.09–0.16 | 0.12–0.15 | 0.07–0.63 | 0.12–0.16 | |
TB | Patients | 17,622 | 14,241 | 4791 | 36,869 | 15,196 | 11,997 | 19,311 | 120,027 | 1181 | 121,208 |
Events | 36 | 41 | 16 | 82 | 36 | 44 | 42 | 297 | <10 | 298–306 | |
IRs (/100PY) | 0.12 | 0.17 | 0.27 | 0.11 | 0.15 | 0.19 | 0.12 | 0.14 | 0.22 | 0.14 | |
95% CI | 0.09–0.17 | 0.12–0.23 | 0.17–0.44 | 0.09–0.14 | 0.11–0.2 | 0.14–0.26 | 0.09–0.16 | 0.12–0.15 | 0.07–0.64 | 0.12–0.15 | |
NTM | Patients | 17,566 | 14,243 | 4794 | 36,867 | 15,193 | 11,999 | 19,309 | 119,971 | 1180 | 121,151 |
Events | 56 | 20 | <10 | 50 | 17 | 14 | 38 | 196–204 | <10 | 197–205 | |
IRs (/100PY) | 0.19 | 0.08 | 0.05 | 0.07 | 0.07 | 0.06 | 0.11 | 0.09 | 0.07 | 0.09 | |
95% CI | 0.15–0.25 | 0.05–0.13 | 0.02–0.15 | 0.05–0.09 | 0.04–0.11 | 0.04–0.1 | 0.08–0.15 | 0.08–0.11 | 0.01–0.41 | 0.08–0.11 | |
HZ | Patients | 17,609 | 14,227 | 4793 | 36,845 | 15,177 | 11,982 | 19,287 | 119,920 | 1180 | 121,100 |
Events | 737 | 571 | 125 | 1542 | 605 | 533 | 847 | 4960 | 91 | 5051 | |
IRs (/100PY) | 2.6 | 2.4 | 2.2 | 2.2 | 2.6 | 2.4 | 2.5 | 2.4 | 7.0 | 2.4 | |
95% CI | 2.42–2.79 | 2.21–2.6 | 1.83–2.6 | 2.05–2.26 | 2.36–2.77 | 2.24–2.66 | 2.35–2.68 | 2.31–2.44 | 5.7–8.59 | 2.34–2.47 |
According to the code of NDB, we cannot open the figures less than 10. Thus, we had to express less than 10 as <10. For the same reason, the total number here cannnot be shown the exact number but expressed as 300–308. If we demonstrate the exact number here, the number of TOF can be calculated, which is less than 10.
For PCP, the IRs were almost in the same range of 0.10–0.20/100 PYs in both bDMARD and JAKi users. The IRs of TB in most bDMARD users were in the range of 0.10–0.20/100 PYs, while CZP users showed a higher IR (0.27/100 PYs). As for NTM, in most bDMARD users the IRs were between 0.05 and 0.10 per 100 PYs, while ABT users showed a higher IR (0.19/100 PYs). For HZ, the IRs were 2.00–3.00/100 PYs among bDMARD users, while TOF users showed more than double that IR at 7.00/100 PYs (95% CI = 5.70–8.59) (Figure 1). The cumulative incidences of these OIs are shown in Figure 2.
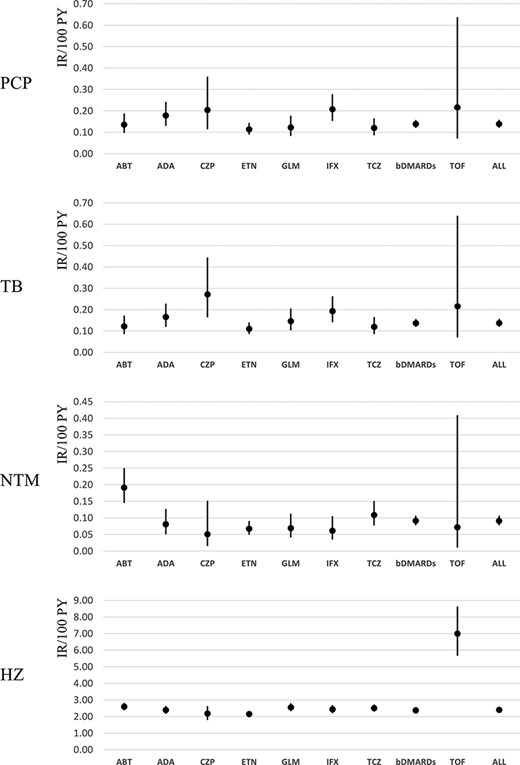
Comparison of IRs for OI among bDMARD and JAKi users. In PCP, CZP shows higher IR; in TB and NTM, ABT shows higher IR; and in HZ, TOF shows higher IR than other drugs.
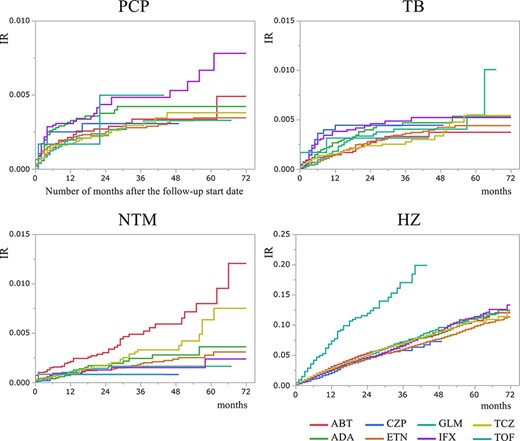
Between three age groups, all OIs showed a higher incidence in people >65 years old compared with those <65 years old. PCP and HZ showed little difference between 65–74 years old and those >75 years old, while for TB there were also some differences between 65–74 years old and >75 years old (Figure 3).

Age difference of cumulative incidence of OI. In TB, IR increases according to age, while in PCP, NTM, and HZ, there is no difference between Groups II and III. Group I: <65 years old, Group II: between 65 and 75 years old, and Group III: >75 years old.
In terms of the onset of the OI, the median for PCP, TB, NTM, and HZ was 3, 7, 9, and 13 months, respectively, after the initiation of molecular-targeted therapy (Figure 4). There was no big difference among the three age groups in all OIs.
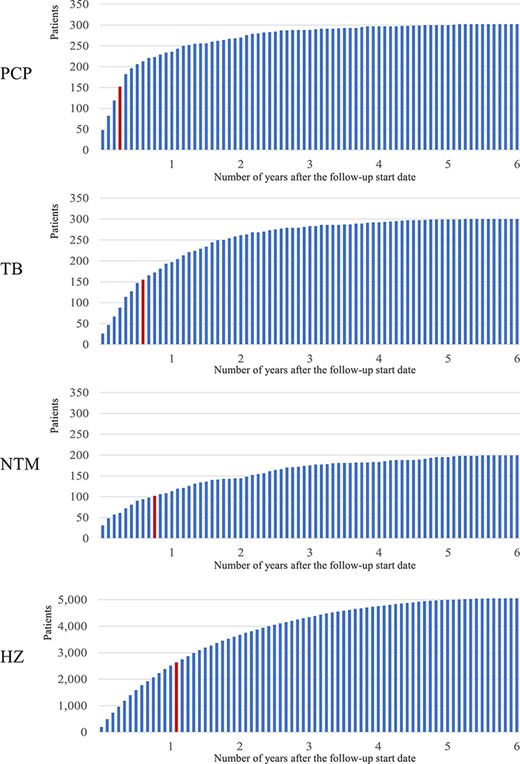
Time of onset of OIs. The frequency of OI every month after MTAT is indicated and the red bar means the month of median (PCP:3 months, TB:7 months, NTM:9 months, HZ:13 months).
Discussion
We conducted a comparative analysis of the incidence of OIs among patients with RA treated with bDMARDs and TOF, using the NDB which covers the whole country. The resultant incidences of PCP, TB, NTM, and HZ were 765, 1158, 834, and 18,336 in 7 years, respectively, which implied 109, 165, 119, and 2619, respectively, per year in the whole of Japan.
The biggest difference in IR among them was seen in HZ: TOF shows a higher HZ IR of 7.00 (95% CI = 5.70–8.59) than that of the bDMARDs as a whole [2.40 (95% CI = 2.31–2.44)], which has also been reported by others [15]. There was no big difference in IRs of OIs between Tumor Necrotizing Factor (TNF) and non-TNF, although there were statistically significant differences between a few TNF. As there was post-marketing surveillance for each agent in Japan, we can compare the data between them [16–21].
For PCP, the IRs were almost in the same range of 0.10–0.20/100 PYs for all bDMARDs and JAKi, almost equal to the reported IR of PCP for bDMARD users (0.10–0.30) in a previous study [22]. On the other hand, there were some differences seen in the IRs of TB and NTM. Although in other bDMARD users the IRs were in the range of 0.10–0.20/100 PYs, only CZP users showed TB IR of 0.27/100 PYs. The IR of NTM was between 0.05 and 0.10 for all bDMARD users except ABT users, who showed a higher IR (0.19/100 PYs). These facts are more clearly represented in the cumulative IR curve, especially for ABT in NTM (Figure 2). However, we have to carefully consider whether these results are due to their pharmaceutical characteristics. Kameda mentioned four cases of TB in 2579 patients treated with CZP in post-marketing surveillance [23], while in our study, there were 12 cases in 4791 patients. Harigai reported only one TB patient and did not refer to NTM in his post-marketing surveillance of 3882 ABT-treated patients [20]. Kameda summarized the incidence of OIs with bDMARDs from papers in Japan which reported that ABT showed a lower incidence than other drugs [21]. Our result might not imply that patients treated with ABT are more likely to become infected with NTM, but ABT would be favourable for cases at risk of NTM, according to the information that ABT is believed to be safer. As a matter of fact, 0.47% of all ABT-treated patients received NTM treatment within 3 months before bDMARD started (those cases were eliminated in our statistics as shown in Table 2), much higher than those of all other drugs (Supplementary Table 1), suggesting that ABT is favoured to be used for NTM patients. These paradoxical data tell us that even drugs that are believed to be safer do not show a big difference in safety, and the risk of OI cannot be avoided. Ichinose compared the hospitalized infections between patients using bDMARDs and showed that ABT had a higher odds ratio than ETN for TB or NTM [6].
When we compare the IRs of OIs internationally, we must consider the original IR of the general population in each region. Japan has more TB patients than other Organization for Economic Cooperation and Development countries, and NTM has emerged in the past two decades [24, 25].
The IR of TB in the general population was reported to be 0.0129 and that of NTM was 0.0147 cases per 100 PYs in 2015 in Japan [26], while in the USA, Wintrop reported the IR of NTM in the general population and in TNF inhibitor users to be only 0.0041 and 0.0740 per 100 PYs, respectively [27]. According to the Korean National Healthcare Claims Database, which is the Korean counterpart of Japan’s NDB, the IR of TB and HZ in RA patients is 0.71 (0.67–0.75) and 2.79 (2.72–2.85), respectively [28]. The IR of TB is higher, while that of HZ is lower than in our study.
In terms of HZ, there is not much difference between bDMARDs, but when compared with other OIs, its incidence is about six or seven times higher than PCP, TB, and NTM, which is a much higher ratio than other post-marketing research [16–21]. For example, Koike reported that the IR of HZ was 0.47/100 PYs in TCZ post-marketing surveillance. One reason to explain this discrepancy is the observation period. Post-marketing research is stopped 1 or 2 years after launch when the cases reach 5000 patients, but the median onset of HZ is 10 months after treatment and can show up even later, after 1 year. Another reason was that we decided the diagnosis not by the doctor’s decision but by the ICD-10 codes in the claims database and anti-HZ drug dosage, which may have overestimated the number of affected patients. However, Curtis reported that the crude incidence of HZ associated with TOF was 3.87/100 PYs in 6967 patients, which is higher than our IR of 2.5 (2.35–2.68) [15]. As the Mongolian population is known to show a higher IR of HZ than that seen in Caucasians, our results seem rational. Hsu reported IRs of 0.08 (0.05–0.13) for PCP, 3.69 (3.44–3.95) for TB, 0.53 (0.44–0.64) for NTM, and 17.9 (17.4–18.5) for HZ from Taiwan’s National Health Insurance Research Database targeted for five rheumatic diseases, totalling 76,966 patients in 13 years [29].
We also should consider the change of IR in the general population in these 7 years. In particular, NTM is emerging in this period in Japan [26] as well as HZ [30]. These facts also reflected in our data. The numbers of patients with NTM and HZ are increasing in 7 years (Supplementary Table 2).
Regarding ages, OIs showed a higher IR in the elderly as many papers previously reported. However, there was little difference between 65–74 years old and those >75 years old, except for TB. Thus far, no big real-world data analysis has been conducted to compare age groups [28, 29]. We measured the median time of onset of PCP, TB, NTM, and HZ to be 3, 7, 9, and 13 months, respectively (Figure 4). There was no difference between the three age groups and drugs. It is important to recognize each onset time for starting prophylaxis. Since the median time of PCP onset was 3 months, prophylaxis should be started together with bDMARDs. This result is similar to that of a study by Kameda which reported that PCP appeared 3–4 months after bDMARDs treatment was initiated [31]. Tanaka mentioned that HZ incidence was the highest 18–24 months after treatment with peficitinib was initiated [32].
As for the limitations of our study, although we can detect almost whole patients in Japan from NDB, we have to recognize the inaccuracy of diagnosis at first. The diagnosis of OI in this study was based on the usage of drugs particular to each OI and not on the definitive diagnosis by doctors or clinical criteria, and as a result, the number of affected patients could have been over- or underestimated. In particular for NTM, there is some possibility that we could not detect all cases because of missing the combination such as kanamycin, amykacin, erythromycin, and fluoroquinolones, or non-treatment cases. Despite this, the IRs we calculated this time were not so different from those reported in previous works [21, 27]. We can pursue and refine these methods hereafter.
Another limitation is that we cannot adjust the baseline data and cannot neglect confounding factors and doctors’ selection bias as we already mentioned. For all OIs, we have to check if the prophylaxis treatment or vaccination was performed or not, as well as the influence of additional drugs such as steroids and methotrexate (MTX). These immunosuppressants may make quite a few influences on the number of patients with OIs. However, as we did not apply steroid- or MTX-only users in this study, we could not compare with or without steroid/MTX. Furthermore, it is not easy to decide each case as concomitant or not because in a long period like 7 years many cases were treated with immunosuppressants not completely but partly together with molecular-targeted drugs. However, it is an important issue to make it clear the difference of with or without immunosuppressant, and we will start a new project to analyse this difference. In addition, JAKi were not commonly prescribed in this observation period. We have to consider that the number of TOF users being much smaller than the bDMARD users could have influenced the results. This will be corrected in the study in the near future.
Conclusions and implications
While there is a big difference between the IRs of HZ in bDMARD and JAKi users, there is little difference in the IRs of PCP, TB, and NTM among TNF, non-TNF, and JAKi users. We should notice that even the molecular-targeted drugs that are believed to be safer than others can also cause OIs. Real-world big data analysis, such as that using the NDB, will give us information from the different aspects of previous reports.
Acknowledgements
We are grateful to MHLW in Japan for providing the NDB data. We would also like to thank Enago (www.enago.jp) for the English language review.
Supplementary data
Supplementary data are available at Modern Rheumatology online.
Conflict of interest
K.T. reports speaker fees from Chugai Pharmaceutical Corporation, Pfizer Japan, Takeda Pharmaceutical Company, Astellas Pharma, Eli Lilly Japan, Ono Pharmaceutical Company, Bristol-Myers Squibb Company, Janssen Pharmaceutical, Mitsubishi Tanabe Pharma Corporation, AYUMI Pharmaceutical Corporation, Nippon Shinyaku Company, and Otsuka Pharmaceutical Company. K.I. reports research grants from Mitsubishi Tanabe Pharma Corporation and speaker fees from AbbVie GK, Eli Lilly Japan, Novartis Japan, Bristol-Myers Squibb Company, and Mitsubishi Tanabe Pharma Corporation. H.N. reports research grants from Chugai Pharmaceutical Corporation, AbbVie GK, Takeda Pharmaceutical Company, Astellas Pharma, Eli Lilly Japan, Asahi Kasei Corporation, Pfizer Japan, UCB Japan Company, Eisai Company, Mitsubishi Tanabe Pharma Corporation, and Bristol-Myers Squibb Company and speaker fee from Chugai Pharmaceutical Corporation, AbbVie GK, Takeda Pharmaceutical Company, Astellas Pharma, Eli Lilly Japan, Asahi Kasei Corporation, Janssen Pharmaceutical, Mitsubishi Tanabe Pharma Corporation, Eisai Company, Bristol-Myers Squibb Company, and Nippon Kayaku Company. H.H. reports a consulting fee from AYUMI Pharmaceutical Corporation.
Funding
This work was supported by the Grants-in-Aid for Scientific Research-KAKENHI from the Ministry of Education, Culture, Sports, Science and Technology (No. 21K12119), 2021.
Data availability
The data that support the findings of this study are available from MHLW in Japan, but restrictions apply to the availability of these data, which were used under licence for the current study, and so are not publicly available. Data are, however, available from MHLW in Japan upon reasonable request and with permission.
Ethical approval
All study procedures were conducted according to the principles of the World Medical Association Declaration of Helsinki.