-
PDF
- Split View
-
Views
-
Cite
Cite
Deng-Ke Zhou, Shuang-Nan Zhang, Li-Ming Song, Jin-Lu Qu, Liang Zhang, Xiang Ma, You-Li Tuo, Ming-Yu Ge, Yanan Wang, Shu Zhang, Lian Tao, Determination of QPO properties in the presence of strong broad-band noise: a case study on the data of MAXI J1820+070, Monthly Notices of the Royal Astronomical Society, Volume 515, Issue 2, September 2022, Pages 1914–1926, https://doi.org/10.1093/mnras/stac1789
- Share Icon Share
ABSTRACT
Accurate calculation of the phase lags of quasi-periodic oscillations (QPOs) will provide insight into their origin. In this paper, we investigate the phase lag correction method that has been applied to calculate the intrinsic phase lags of the QPOs in MAXI J1820+070. We find that the traditional additive model between broad-band noise (BBN) and QPOs in the time domain is rejected, but the convolution model is accepted. By introducing a convolution mechanism in the time domain, the Fourier cross-spectrum analysis shows that the phase lags between QPOs components in different energy bands will have a simple linear relationship with the phase lags between the total signals, so that the intrinsic phase lags of the QPOs can be obtained by linear correction. The power density spectrum (PDS) thus requires a multiplicative model to interpret the data. We briefly discuss a physical scenario for interpreting the convolution. In this scenario, the corona acts as a low-pass filter, Green’s function containing the noise is convolved with the QPOs to form the low-frequency part of the PDS, while the high-frequency part requires an additive component. We use a multiplicative PDS model to fit the data observed by the Insight-Hard X-ray Modulation Telescope (HXMT). The overall fitting results are similar compared to the traditional additive PDS model. Neither the width nor the centroid frequency of the QPOs obtained from each of the two PDS models was significantly different, except for the rms of the QPOs. Our work thus provides a new perspective on the coupling of noise and QPOs.
1 INTRODUCTION
Decades of research on black hole binaries (BHBs) show that their X-ray emission is variable on different time-scales, including the low-frequency (mHz to 30 Hz) quasi-periodic oscillations (QPOs) and the broad-band noise (BBN; Psaltis, Belloni & van der Klis 1999; Ingram, Done & Fragile 2009; Ingram & Done 2011; Motta 2016). The study of the timing signals can effectively diagnose the geometric characteristics of the disc and the corona near the black hole (Belloni & Hasinger 1990; Belloni, Psaltis & van der Klis 2002; Ingram 2016; Ingram & Motta 2019). The disc and the corona near the black hole continuously radiate X-ray photons outward due to various radiation mechanisms (thermal radiation, Compton radiation, and so on). Photons with different energy arrive at the observer at different times because they may come from different radiation regions (Lin et al. 2000; Rapisarda et al. 2016), or undergo different scattering processes (Cui 1999; Poutanen 2001), or have very complex mechanisms that cause delays (Morgan, Remillard & Greiner 1997; Wijnands, Homan & van der Klis 1999; Qu et al. 2010). Therefore, analysing the phase/time lags of photons between different energy bands helps us better understand the geometric or radiometric characteristics of X-ray BHBs. A common analysis method is based on Fourier cross-spectrum, which measures the frequency-dependent phase lag spectrum (FDPLS) between the signals in two different energy bands (van der Klis et al. 1987). This method allows to study the phase lags of two signals as a function of Fourier frequencies. Thus the phase lags between different components of timing signals, which usually originate from different physical processes (Narayan & Yi 1995; Done, Gierliński & Kubota 2007; Ingram & Done 2011), can be studied separately. For example, Zhang et al. (2020) conducted a systematic study on the phase lag of the type-C QPO and found that the phase lag behaviour of the subharmonic of the QPO is very similar to that of the QPO fundamental component but the second harmonic of the QPO shows a quit different phase lag behaviour. Uttley et al. (2011) investigated the phase lag of the BBN components of GX 339 and found that the large lags can be explained by viscous propagation of mass accretion fluctuations in the disc.
The traditional way to obtain the phase lag of the QPO components is to assume that the other components contribute weakly to the lag in the QPO frequency range, and then directly treat the values in the QPO frequency range as the phase lag of the QPO components (e.g. Morgan et al. 1997; Wijnands et al. 1999; Kara et al. 2019; Zhang et al. 2020). However, the coexistence of various components makes it difficult to calculate any of the individual component. In particular, when the BBN is sufficiently strong in the QPO frequency range, there is no reason to ignore the effect of the BBN on the measured QPO phase lag. Despite attempts by some authors to ameliorate this dilemma by fitting different components of the cross-spectrum (e.g. Qu et al. 2004), there is no broad consensus on how to obtain the intrinsic phase lag of the QPO in the presence of strong BBN. Therefore, it is difficult to determine the intrinsic properties (including phase lag) of the QPO in the presence of strong BBN.
In a recent work (Ma et al. 2021, hereafter Ma21) the authors attempt to correct for the original phase lags, which gives a clear physical picture using the corrected phase lags. Ma21 investigated the behaviour of the QPO phase lags in MAXI J1820+070 using Insight-Hard X-ray Modulation Telescope (HXMT) observations and proposed a method to obtain the intrinsic phase lag of the QPO. In their analysis of the phase lags, they find that by subtracting the phase lags below the QPO frequency range they can obtain consistent QPO phase lags as functions of photon energy for all observations and can explain the lag behaviour through the precession of a compact jet above the black hole. On the data they used, the power density spectrum (PDS) shows that the BBN components are too strong compared to the QPO component to ignore the contribution to the phase lags in the QPO frequency range (see panel c of fig. 1 in Ma21). If they do not correct the phase lags for the QPO, the phase lags obtained from the original FDPLS will be affected by the BBN and thus are not intrinsic phase lags of the QPO. Although Ma21 applied this method to obtain consistent results of the phase lags, the rationale for doing so was not explained in detail, so the plausibility of this correction method needs to be tested. On the data they analysed, some of the observations obtained phase lags with little difference before and after the correction, but some of the phase lags changed significantly (even the sign is totally reversed) before and after the correction. Therefore, we believe it is necessary to investigate under what conditions the correction is effective and how the QPO component is related to the BBN component. The motivation of this paper is to explore the mechanism behind the correction method used by Ma21 and to investigate the QPO properties in the presence of strong BBN in conjunction with the results obtained by Ma21.
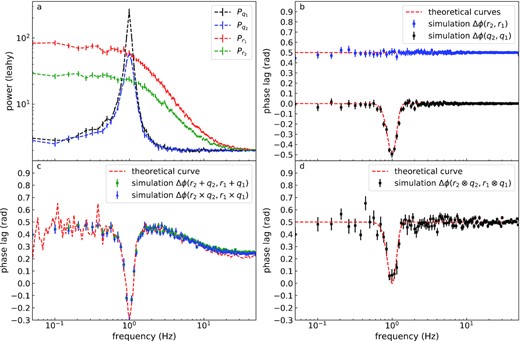
Simulation results of the FDPLS and PDS. Panel (a): PDS of signals r1(t), r2(t), q1(t), and q2(t). Panel (b): simulated FDPLS (blue dots) between r1(t) and r2(t) and simulated FDPLS (black dots) between q1(t) and q2(t). Panel (c): simulated FDPLS (green dots) between r2(t) + q2(t) and r1(t) + q1(t) and simulated FDPLS (blue dots) between r2(t) × q2(t) and r1(t) × r2(t). Panel (d): simulated FDPLS between r2(t) ⊗ q2(t) and r1(t) ⊗ q1(t). The red curves in panels (b), (c), and (d) are theoretically calculated curves. Error bars correspond to 1σ confidence intervals.
Since we want to obtain the properties of a certain component (in our case, the QPO), and what we observe is some kind of superposition of all components, we have to face the problem of how these components contribute to the total signal. In this paper, when we refer to the term signal, we are referring to the light curve or the underlying time series. The total signal is defined as the time series that we directly observe and the subsignals are the subcomponents such as QPO and BBN that make up the total signal. Traditionally, it is believed that the BBN and the QPO are additive in the time domain and that they are incoherent at any frequency, which is why the PDS is fitted by the sum of several Lorentzian functions. Ingram & van der Klis (2013) proposed a possible relationship between QPO and BBN, where the QPO component and the BBN component are multiplied in the time domain. In this case, a convolution model is required for the fitting of the PDS in the frequency domain. Another way in which the QPO component and the BBN component are combined into a total signal in the time domain is convolution, which is usually caused by the response of the QPO signal in the region where the BBN component is generated (a model similar to this mechanism can be found in Cabanac et al. 2010). The calculation of the FDPLS involves the Fourier orthogonal decomposition of the signal, so it can be expected that if the subsignals form the total signal in different ways, then the relationship between the FDPLS of the total signal and the FDPLS of the subsignals must be different.
This paper is structured as follows. Section 2 analyses the relationships between the FDPLS and the PDS of total signals and subsignals. An algorithm to generate two signals satisfied specific PDS and FDPLS simultaneously is also proposed. Besides, one possible way of coupling the QPO component and the BBN component in the time domain is discussed. In Section 3, based on the results of Ma21’s analysis of MAXI J1820+070 on phase lags, we argue that the QPO component and the BBN component constitute the total signal by convolution in the time domain. Using the data of MAXI J1820+070, we fit the PDS in different energy bands using the multiplicative PDS model and the traditional additive PDS model, and compare their differences. In addition, we also performed some simulations to rule out the possibility that the total signal appears to be the sum of the subsignals in the time domain. Section 4 discusses and summarizes the whole paper.
2 THEORY AND SIMULATION
2.1 Phase lag relationship
2.2 PDS relationship
The PDS properties for convolved signals can be generalized to multiplicative signals simply based on the symmetry of the Fourier transform, i.e. the PDS of the multiplicative signal is the convolution of the PDS of the subsignals.
2.3 An algorithm for simultaneously simulating signals with specified PDS and FDPLS
In order to verify the correctness of the above theoretical analysis and to facilitate the analysis below, some simulations need to be done. An algorithm is thus needed to generate two signals with specified PDS and specified FDPLS simultaneously. The algorithm steps are as follows.
Use Timmer & Koenig (1995, hereafter TK95) algorithm to generate two signals s(t) and s′(t) that satisfy the specified PDS. Because the phase given to the signal by TK95 algorithm is random, the phase lag between these two signals is now on average zero. Denote their Fourier transforms as S(f), S′(f), respectively.
- Given the FDPLS ϕ(f), then calculate CCF(f) according to the following equation:where i is the imaginary unit.(14)$$\begin{eqnarray*} {\rm CCF}(f)=\left\lbrace \begin{array}{@{}l@{\quad }l@{}}|S(f)||S^{\prime }(f)|\lbrace \cos [\phi (f)]+\mathrm{ i}\sin [\phi (f)]\rbrace &\rm{f\ne 0},\\ |S(f)||S^{\prime }(f)|& \text{f=0}, \end{array}\right. \end{eqnarray*}$$
- The complex array CCF(f) obtained in step (2) is divided by the complex conjugate of S(f) to obtain a new complex array. Then performing inverse Fourier transform to it to obtain the signal s″(t). Expressed in mathematical notation, it is(15)$$\begin{eqnarray*} s^{\prime \prime }(t)=\mathscr {F}^{-1} \left[\frac{{\rm CCF}(f)}{S^{*}(f)} \right]. \end{eqnarray*}$$
The underlying PDS of s″(t) is the same as the PDS of s(t), but the FDPLS between s″(t) and s(t) will satisfy the given FDPLS. In summary, s(t) and s″(t) satisfy both the given PDS and the given FDPLS. In this paper, all PDS and FDPLS are extracted using the X-ray astronomy python package stingray(version 0.3; Huppenkothen et al. 2019), and all PDS and FDPLS fitting are done by xspec (version 12.11.1; Arnaud 1996) or lmfit (version 1.0.2; Newville et al. 2014).
2.4 Simulation
Timing properties of r1(t), r2(t), q1(t), and q2(t) (see Section 2.4 for their definition).
Signals . | Bin size (s) . | νc . | ω . | Mean rate (counts s−1) . | Exposure (s) . | Fractional rms . | PDS type . | FDPLS type . |
---|---|---|---|---|---|---|---|---|
r1(t) | 0.01 | 0 | 3 | 2000 | 2000 | 30 per cent | BBN | Constant |
r2(t) | 0.01 | 0 | 4 | 2000 | 2000 | 20 per cent | BBN | |
q1(t) | 0.01 | 1 | 0.1 | 2000 | 2000 | 15 per cent | QPO | Dip |
q2(t) | 0.01 | 1 | 0.2 | 2000 | 2000 | 10 per cent | QPO |
Signals . | Bin size (s) . | νc . | ω . | Mean rate (counts s−1) . | Exposure (s) . | Fractional rms . | PDS type . | FDPLS type . |
---|---|---|---|---|---|---|---|---|
r1(t) | 0.01 | 0 | 3 | 2000 | 2000 | 30 per cent | BBN | Constant |
r2(t) | 0.01 | 0 | 4 | 2000 | 2000 | 20 per cent | BBN | |
q1(t) | 0.01 | 1 | 0.1 | 2000 | 2000 | 15 per cent | QPO | Dip |
q2(t) | 0.01 | 1 | 0.2 | 2000 | 2000 | 10 per cent | QPO |
Timing properties of r1(t), r2(t), q1(t), and q2(t) (see Section 2.4 for their definition).
Signals . | Bin size (s) . | νc . | ω . | Mean rate (counts s−1) . | Exposure (s) . | Fractional rms . | PDS type . | FDPLS type . |
---|---|---|---|---|---|---|---|---|
r1(t) | 0.01 | 0 | 3 | 2000 | 2000 | 30 per cent | BBN | Constant |
r2(t) | 0.01 | 0 | 4 | 2000 | 2000 | 20 per cent | BBN | |
q1(t) | 0.01 | 1 | 0.1 | 2000 | 2000 | 15 per cent | QPO | Dip |
q2(t) | 0.01 | 1 | 0.2 | 2000 | 2000 | 10 per cent | QPO |
Signals . | Bin size (s) . | νc . | ω . | Mean rate (counts s−1) . | Exposure (s) . | Fractional rms . | PDS type . | FDPLS type . |
---|---|---|---|---|---|---|---|---|
r1(t) | 0.01 | 0 | 3 | 2000 | 2000 | 30 per cent | BBN | Constant |
r2(t) | 0.01 | 0 | 4 | 2000 | 2000 | 20 per cent | BBN | |
q1(t) | 0.01 | 1 | 0.1 | 2000 | 2000 | 15 per cent | QPO | Dip |
q2(t) | 0.01 | 1 | 0.2 | 2000 | 2000 | 10 per cent | QPO |
The results of the simulated PDS and the FDPLS are shown in panels (a) and (b) of Fig. 1, respectively. When the total signal is assumed to be additive or multiplicative signal, the FDPLS between the total signals is shown in panel (c) of Fig. 1. When the total signal is assumed to be the convolved signal, the FDPLS between the total signals is shown in panel (d) of Fig. 1. As stated in the theoretical analysis section, the FDPLS of the multiplicative signal is very close to the FDPLS of the additive signal (see the green data points and the blue data points in panel c of Fig. 1). Because of the symmetry of the Fourier transform to convolution and multiplication, the PDS section will only compare the differences between convolved and additive signals. In panels (b), (c), and (d) of Fig. 1, the data points are obtained by simulation and the red dashed lines are obtained from our theoretical calculation (the theoretical curve drawn in panel c of Fig. 1 is for the additive signal, and we did not draw the theoretical curve for the multiplicative signal because of the analytical difficulties). The theoretical curve shown in panel (c) of Fig. 1 is calculated by using the value of the simulated data (i.e. amplitude and initial phase), and it appears to fluctuate around the data points, which is due to the randomness deliberately introduced by the simulation algorithm (see TK95 for detail). The difference between panels (c) and (d) of Fig. 1 is mainly due to the different dependence of the FDPLS on the different kinds of signals (additive, multiplicative, and convolved signals) on each subsignal. The FDPLS between the convolved signals depends only on the FDPLS between subsignals, independent of the other properties of the subsignals. This is not the case for the additive and multiplicative signals. So it can be seen from panel (c) that the FDPLS depends on the relative power of subsignals, while in panel (d) the FDPLS does not depend on the shape of the PDS of the subsignals. In conclusion, the simulation results of the FDPLS are consistent with the theoretical analysis. The results of the PDS simulation are shown in Fig. 2.
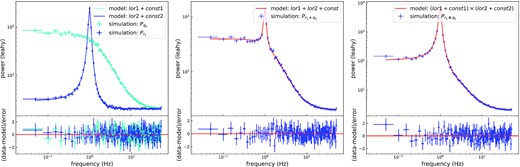
PDS of simulation results. Left-hand panel: the cyan and blue data points are the PDS of the signals r1(t) and q1(t), respectively. The solid lines are the best fit using Lorentzian model (considering the contribution of Poisson noise requires adding a constant to the Lorentzian model). Middle panel: the blue points are the PDS of the sum of the signals r1(t) and q1(t). The red solid line is the best fit using two summed Lorentzian functions. Right-hand panel: the blue points are the PDS of the convolution of the signals r1(t) and q1(t). The red solid line is the best fit using two multiplicative Lorentzian functions (considering the contribution of Poisson noise requires adding a constant to each Lorentzian model). Error bars correspond to 1σ confidence intervals.
The PDS of r1(t) and q1(t) is shown in the left-hand panel of Fig. 2, and the PDS of the additive and convolved signals is shown in the middle and right-hand panels of Fig. 2, respectively. We can see that the PDS of the additive signal is the sum of the PDS of the subsignals, while the PDS of the convolved signal is the multiplication of the PDS of the subsignals. The solid lines running through the data points in the PDS are the best fit using the additive and multiplicative Lorentzian models for the additive signal and the convolved signal, respectively. Overall, the simulation results are in good agreement with those of the theoretical analysis.
2.5 A possible mechanism for introducing a convolution mechanism in the time domain
Furthermore, it is worth noting that the above result is valid only when R << R0 and the assumptions about the white noise and QPO fluctuations are satisfied. The total observed luminosity is the integral of the differential luminosity over the entire corona after considering the emissivity (Ingram & Done 2011), but the form is very complicated. None the less, it is still worthwhile to start with a simple model to explain the data. For this reason, when fitting the PDS of the real data with the multiplicative PDS model in Section 3, only a form similar to equation (20) will be considered.
3 THE FDPLS AND PDS OF MAXI J1820+070
MAXI J1820+070 was discovered by the Monitor of All-sky X-ray Image (MAXI) during the outburst on 2018 March 11 (Kawamuro et al. 2018). It was confirmed to be a BHB (Torres et al. 2019). Insight-HXMT is an X-ray astronomy satellite launched by China on June 15, 2017, and it has obtained a wealth of data (Zhang et al. 2020). Insight-HXMT carried out observations 3 d after its discovery and obtained rich data with total exposure time of over 2000 ks. Ma21 have carried out a detailed temporal analysis of these data and, in particular, detailed calculations of the phase lags in different energy bands. Fig. 3 shows three typical observations from top to bottom, with a clear dip-like feature appearing near the QPO frequency range (the averaged value of this frequency range shown by the cyan dots denote the original QPO phase lag). The phase lags of low-frequency BBN component are marked with red dots, denoted as background phase lag. The intrinsic phase lag of the QPO is obtained by subtracting the average of the background phase lag from the original QPO phase lag. After the correction, the absolute value of phase lag of the QPO becomes larger as the energy increases in all three observations. For the sake of clarity, the detailed correction steps used in Ma21 are resummarized as follows.
Calculate the FDPLS and identify the centroid frequency f0 and the FWHM ω of the QPO according to the PDS.
The original phase lag of the QPO is defined as the average of the phase lags in the frequency range f0 ± ω/2.
The background phase lag is defined as the average of the phase lags below the QPO frequency range.
The intrinsic phase lag of the QPO is then defined as the original phase lag minus the background phase lag.
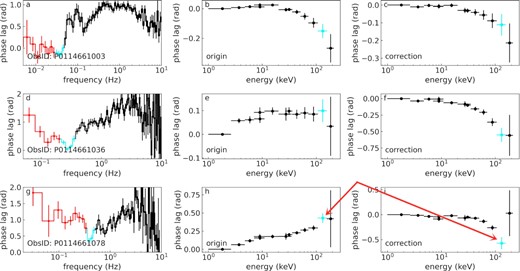
QPO phase lag correction of three typical Insight-HXMT observations (reproduced from the data in Ma21). Each row represents the results of one observation. The FDPLS between 1–2.6 and 100–150 keV energy bands is shown in the left-hand panels. Middle panels are the original QPO energy-dependent phase lags. Right-hand panels are the intrinsic QPO energy-dependent phase lags after correction. The intrinsic QPO phase lags are obtained by subtracting the average of the phase lag of the BBN component (marked by the red dots in the left-hand panels) from the original QPO phase lag (the averaged value marked by the cyan dots in left-hand panels). The red arrows indicate the high-energy band data that we will model in Fig. 6. Error bars correspond to 1σ confidence intervals.
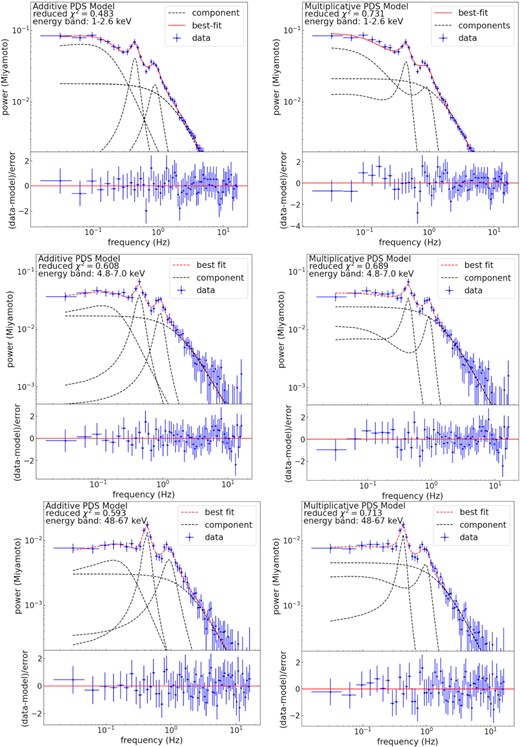
Several examples of the best fit of the observed PDS data (ObsID: P0114661078) in different energy bands with additive or multiplicative PDS models. The PDS model used in the left-hand panels is the additive PDS model (i.e. equation 21). The PDS model used in the right-hand panels is the multiplicative PDS model (i.e. equation 22). The contribution of Poisson noise in all PDS has been subtracted. Error bars correspond to 1σ confidence intervals.
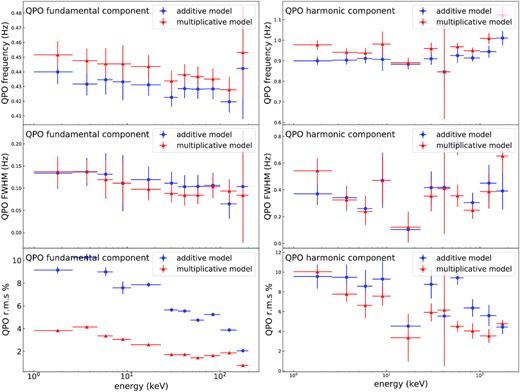
The parameters of the QPO (fundamental and harmonic components) as functions of photon energy that are obtained with the traditional additive and multiplicative PDS model, respectively. Error bars correspond to 1σ confidence intervals.
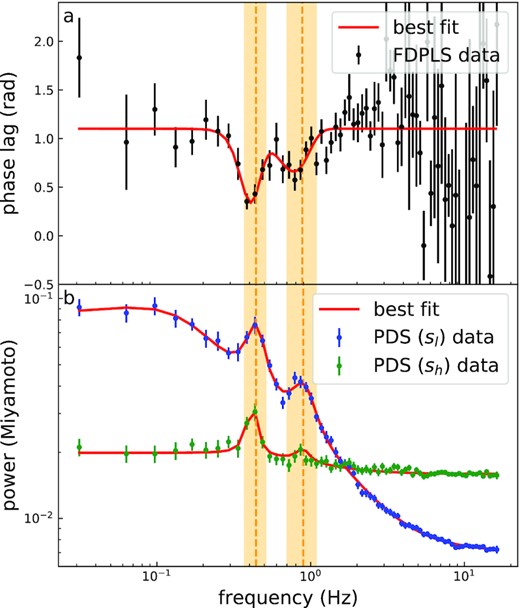
Modelling results for the FDPLS between signals sl and sh and their respective PDS (see Section 3 for the definitions of sl and sh). In panels (a) and (b), the solid lines are the best fit using the models proposed in Section 3 and the best-fitting parameters are listed in Table 2. The yellow bands running through the two panels are the QPO FWHM frequency ranges (including fundamental and harmonic frequencies). Error bars correspond to 1σ confidence intervals.
Such a correction actually implies two assumptions. The first assumption is that the phase lags of the BBN component at the QPO frequency range are the same as the phase lags below the QPO frequency range, at least their averaged values must be approximately equal. The second assumption is that the total phase lags (i.e. the observed original phase lags) at the QPO frequency range are equal to the sum of the BBN component phase lags and the QPO intrinsic phase lags. The correction of the phase lags is valid only when these two assumptions are satisfied simultaneously. The first assumption can be considered to be approximately satisfied. This is because the FDPLS obtained from MAXI J1820+070 shows that the phase lag does not vary significantly with frequency below the QPO frequency range. Thus it is reasonable to assume that at the QPO frequency range, the phase lags of the BBN component are approximately equal to the phase lags below the QPO frequency range. As to whether the second assumption can be satisfied, we need to first make an assumption about how the BBN component and the QPO component synthesize the observed signal. From the discussion of Section 2 we know that if the observed signal is considered to be the sum of the BBN component and the QPO component (which is the default assumption in most of the literatures), the second condition cannot be satisfied. The second condition can be satisfied only when the observed signal is considered as a convolution of the BBN component and the QPO component.
. | QPO frequency (Hz) . | QPO FWHM (Hz) . | ||
---|---|---|---|---|
Energy band (keV) | Additive PDS model | Multiplicative PDS model | Additive PDS model | Multiplicative PDS model |
1.0–2.6 | 0.44 ± 0.01 | 0.45 ± 0.01 | 0.13 ± 0.04 | 0.14 ± 0.03 |
2.6–4.8 | 0.43 ± 0.01 | 0.45 ± 0.01 | 0.14 ± 0.03 | 0.14 ± 0.03 |
4.8–7.0 | 0.43 ± 0.01 | 0.45 ± 0.01 | 0.13 ± 0.05 | 0.12 ± 0.04 |
7.0–11.0 | 0.43 ± 0.01 | 0.45 ± 0.01 | 0.11 ± 0.06 | 0.11 ± 0.05 |
11.0–23.0 | 0.43 ± 0.01 | 0.44 ± 0.01 | 0.12 ± 0.03 | 0.10 ± 0.03 |
25.0–35.0 | 0.42 ± 0.01 | 0.43 ± 0.01 | 0.11 ± 0.03 | 0.09 ± 0.02 |
35.0–48.0 | 0.43 ± 0.01 | 0.44 ± 0.01 | 0.10 ± 0.03 | 0.08 ± 0.02 |
48.0–67.0 | 0.43 ± 0.01 | 0.44 ± 0.01 | 0.10 ± 0.03 | 0.08 ± 0.02 |
67.0–100.0 | 0.43 ± 0.01 | 0.44 ± 0.01 | 0.11 ± 0.03 | 0.10 ± 0.02 |
100.0–150.0 | 0.42 ± 0.01 | 0.43 ± 0.01 | 0.06 ± 0.03 | 0.09 ± 0.03 |
150.0–200.0 | 0.44 ± 0.03 | 0.45 ± 0.03 | 0.10 ± 0.13 | 0.08 ± 0.11 |
QPO rms per centa | Reduced χ2 | |||
Energy band (keV) | Additive PDS model | Multiplicative PDS model | Additive PDS model | Multiplicative PDS model |
1.0–2.6 | 9.14 ± 0.31 | 3.83 ± 0.10 | 0.48 | 0.71 |
2.6–4.8 | 10.26 ± 0.31 | 4.15 ± 0.07 | 0.74 | 0.88 |
4.8–7.0 | 8.99 ± 0.40 | 3.36 ± 0.09 | 0.61 | 0.69 |
7.0–11.0 | 7.59 ± 0.56 | 3.06 ± 0.13 | 0.66 | 0.66 |
11.0–23.0 | 7.86 ± 0.20 | 2.59 ± 0.05 | 0.48 | 0.57 |
25.0–35.0 | 5.65 ± 0.11 | 1.72 ± 0.02 | 1.02 | 1.55 |
35.0–48.0 | 5.54 ± 0.13 | 1.72 ± 0.03 | 0.89 | 1.14 |
48.0–67.0 | 4.75 ± 0.09 | 1.44 ± 0.02 | 0.59 | 0.71 |
67.0–100.0 | 5.24 ± 0.13 | 1.64 ± 0.02 | 0.45 | 0.58 |
100.0–150.0 | 3.88 ± 0.14 | 1.88 ± 0.04 | 0.43 | 0.44 |
150.0–200.0 | 2.05 ± 0.12 | 0.76 ± 0.06 | 0.44 | 0.41 |
. | QPO frequency (Hz) . | QPO FWHM (Hz) . | ||
---|---|---|---|---|
Energy band (keV) | Additive PDS model | Multiplicative PDS model | Additive PDS model | Multiplicative PDS model |
1.0–2.6 | 0.44 ± 0.01 | 0.45 ± 0.01 | 0.13 ± 0.04 | 0.14 ± 0.03 |
2.6–4.8 | 0.43 ± 0.01 | 0.45 ± 0.01 | 0.14 ± 0.03 | 0.14 ± 0.03 |
4.8–7.0 | 0.43 ± 0.01 | 0.45 ± 0.01 | 0.13 ± 0.05 | 0.12 ± 0.04 |
7.0–11.0 | 0.43 ± 0.01 | 0.45 ± 0.01 | 0.11 ± 0.06 | 0.11 ± 0.05 |
11.0–23.0 | 0.43 ± 0.01 | 0.44 ± 0.01 | 0.12 ± 0.03 | 0.10 ± 0.03 |
25.0–35.0 | 0.42 ± 0.01 | 0.43 ± 0.01 | 0.11 ± 0.03 | 0.09 ± 0.02 |
35.0–48.0 | 0.43 ± 0.01 | 0.44 ± 0.01 | 0.10 ± 0.03 | 0.08 ± 0.02 |
48.0–67.0 | 0.43 ± 0.01 | 0.44 ± 0.01 | 0.10 ± 0.03 | 0.08 ± 0.02 |
67.0–100.0 | 0.43 ± 0.01 | 0.44 ± 0.01 | 0.11 ± 0.03 | 0.10 ± 0.02 |
100.0–150.0 | 0.42 ± 0.01 | 0.43 ± 0.01 | 0.06 ± 0.03 | 0.09 ± 0.03 |
150.0–200.0 | 0.44 ± 0.03 | 0.45 ± 0.03 | 0.10 ± 0.13 | 0.08 ± 0.11 |
QPO rms per centa | Reduced χ2 | |||
Energy band (keV) | Additive PDS model | Multiplicative PDS model | Additive PDS model | Multiplicative PDS model |
1.0–2.6 | 9.14 ± 0.31 | 3.83 ± 0.10 | 0.48 | 0.71 |
2.6–4.8 | 10.26 ± 0.31 | 4.15 ± 0.07 | 0.74 | 0.88 |
4.8–7.0 | 8.99 ± 0.40 | 3.36 ± 0.09 | 0.61 | 0.69 |
7.0–11.0 | 7.59 ± 0.56 | 3.06 ± 0.13 | 0.66 | 0.66 |
11.0–23.0 | 7.86 ± 0.20 | 2.59 ± 0.05 | 0.48 | 0.57 |
25.0–35.0 | 5.65 ± 0.11 | 1.72 ± 0.02 | 1.02 | 1.55 |
35.0–48.0 | 5.54 ± 0.13 | 1.72 ± 0.03 | 0.89 | 1.14 |
48.0–67.0 | 4.75 ± 0.09 | 1.44 ± 0.02 | 0.59 | 0.71 |
67.0–100.0 | 5.24 ± 0.13 | 1.64 ± 0.02 | 0.45 | 0.58 |
100.0–150.0 | 3.88 ± 0.14 | 1.88 ± 0.04 | 0.43 | 0.44 |
150.0–200.0 | 2.05 ± 0.12 | 0.76 ± 0.06 | 0.44 | 0.41 |
aNo background correction is applied to the rms because we are only interested in the difference between the results of fitting using the additive PDS model and the multiplicative PDS model, and the correction factors are the same for both models.
. | QPO frequency (Hz) . | QPO FWHM (Hz) . | ||
---|---|---|---|---|
Energy band (keV) | Additive PDS model | Multiplicative PDS model | Additive PDS model | Multiplicative PDS model |
1.0–2.6 | 0.44 ± 0.01 | 0.45 ± 0.01 | 0.13 ± 0.04 | 0.14 ± 0.03 |
2.6–4.8 | 0.43 ± 0.01 | 0.45 ± 0.01 | 0.14 ± 0.03 | 0.14 ± 0.03 |
4.8–7.0 | 0.43 ± 0.01 | 0.45 ± 0.01 | 0.13 ± 0.05 | 0.12 ± 0.04 |
7.0–11.0 | 0.43 ± 0.01 | 0.45 ± 0.01 | 0.11 ± 0.06 | 0.11 ± 0.05 |
11.0–23.0 | 0.43 ± 0.01 | 0.44 ± 0.01 | 0.12 ± 0.03 | 0.10 ± 0.03 |
25.0–35.0 | 0.42 ± 0.01 | 0.43 ± 0.01 | 0.11 ± 0.03 | 0.09 ± 0.02 |
35.0–48.0 | 0.43 ± 0.01 | 0.44 ± 0.01 | 0.10 ± 0.03 | 0.08 ± 0.02 |
48.0–67.0 | 0.43 ± 0.01 | 0.44 ± 0.01 | 0.10 ± 0.03 | 0.08 ± 0.02 |
67.0–100.0 | 0.43 ± 0.01 | 0.44 ± 0.01 | 0.11 ± 0.03 | 0.10 ± 0.02 |
100.0–150.0 | 0.42 ± 0.01 | 0.43 ± 0.01 | 0.06 ± 0.03 | 0.09 ± 0.03 |
150.0–200.0 | 0.44 ± 0.03 | 0.45 ± 0.03 | 0.10 ± 0.13 | 0.08 ± 0.11 |
QPO rms per centa | Reduced χ2 | |||
Energy band (keV) | Additive PDS model | Multiplicative PDS model | Additive PDS model | Multiplicative PDS model |
1.0–2.6 | 9.14 ± 0.31 | 3.83 ± 0.10 | 0.48 | 0.71 |
2.6–4.8 | 10.26 ± 0.31 | 4.15 ± 0.07 | 0.74 | 0.88 |
4.8–7.0 | 8.99 ± 0.40 | 3.36 ± 0.09 | 0.61 | 0.69 |
7.0–11.0 | 7.59 ± 0.56 | 3.06 ± 0.13 | 0.66 | 0.66 |
11.0–23.0 | 7.86 ± 0.20 | 2.59 ± 0.05 | 0.48 | 0.57 |
25.0–35.0 | 5.65 ± 0.11 | 1.72 ± 0.02 | 1.02 | 1.55 |
35.0–48.0 | 5.54 ± 0.13 | 1.72 ± 0.03 | 0.89 | 1.14 |
48.0–67.0 | 4.75 ± 0.09 | 1.44 ± 0.02 | 0.59 | 0.71 |
67.0–100.0 | 5.24 ± 0.13 | 1.64 ± 0.02 | 0.45 | 0.58 |
100.0–150.0 | 3.88 ± 0.14 | 1.88 ± 0.04 | 0.43 | 0.44 |
150.0–200.0 | 2.05 ± 0.12 | 0.76 ± 0.06 | 0.44 | 0.41 |
. | QPO frequency (Hz) . | QPO FWHM (Hz) . | ||
---|---|---|---|---|
Energy band (keV) | Additive PDS model | Multiplicative PDS model | Additive PDS model | Multiplicative PDS model |
1.0–2.6 | 0.44 ± 0.01 | 0.45 ± 0.01 | 0.13 ± 0.04 | 0.14 ± 0.03 |
2.6–4.8 | 0.43 ± 0.01 | 0.45 ± 0.01 | 0.14 ± 0.03 | 0.14 ± 0.03 |
4.8–7.0 | 0.43 ± 0.01 | 0.45 ± 0.01 | 0.13 ± 0.05 | 0.12 ± 0.04 |
7.0–11.0 | 0.43 ± 0.01 | 0.45 ± 0.01 | 0.11 ± 0.06 | 0.11 ± 0.05 |
11.0–23.0 | 0.43 ± 0.01 | 0.44 ± 0.01 | 0.12 ± 0.03 | 0.10 ± 0.03 |
25.0–35.0 | 0.42 ± 0.01 | 0.43 ± 0.01 | 0.11 ± 0.03 | 0.09 ± 0.02 |
35.0–48.0 | 0.43 ± 0.01 | 0.44 ± 0.01 | 0.10 ± 0.03 | 0.08 ± 0.02 |
48.0–67.0 | 0.43 ± 0.01 | 0.44 ± 0.01 | 0.10 ± 0.03 | 0.08 ± 0.02 |
67.0–100.0 | 0.43 ± 0.01 | 0.44 ± 0.01 | 0.11 ± 0.03 | 0.10 ± 0.02 |
100.0–150.0 | 0.42 ± 0.01 | 0.43 ± 0.01 | 0.06 ± 0.03 | 0.09 ± 0.03 |
150.0–200.0 | 0.44 ± 0.03 | 0.45 ± 0.03 | 0.10 ± 0.13 | 0.08 ± 0.11 |
QPO rms per centa | Reduced χ2 | |||
Energy band (keV) | Additive PDS model | Multiplicative PDS model | Additive PDS model | Multiplicative PDS model |
1.0–2.6 | 9.14 ± 0.31 | 3.83 ± 0.10 | 0.48 | 0.71 |
2.6–4.8 | 10.26 ± 0.31 | 4.15 ± 0.07 | 0.74 | 0.88 |
4.8–7.0 | 8.99 ± 0.40 | 3.36 ± 0.09 | 0.61 | 0.69 |
7.0–11.0 | 7.59 ± 0.56 | 3.06 ± 0.13 | 0.66 | 0.66 |
11.0–23.0 | 7.86 ± 0.20 | 2.59 ± 0.05 | 0.48 | 0.57 |
25.0–35.0 | 5.65 ± 0.11 | 1.72 ± 0.02 | 1.02 | 1.55 |
35.0–48.0 | 5.54 ± 0.13 | 1.72 ± 0.03 | 0.89 | 1.14 |
48.0–67.0 | 4.75 ± 0.09 | 1.44 ± 0.02 | 0.59 | 0.71 |
67.0–100.0 | 5.24 ± 0.13 | 1.64 ± 0.02 | 0.45 | 0.58 |
100.0–150.0 | 3.88 ± 0.14 | 1.88 ± 0.04 | 0.43 | 0.44 |
150.0–200.0 | 2.05 ± 0.12 | 0.76 ± 0.06 | 0.44 | 0.41 |
aNo background correction is applied to the rms because we are only interested in the difference between the results of fitting using the additive PDS model and the multiplicative PDS model, and the correction factors are the same for both models.
. | QPO frequency (Hz) . | QPO FWHM (Hz) . | ||
---|---|---|---|---|
Energy band (keV) | Additive PDS model | Multiplicative PDS model | Additive PDS model | Multiplicative PDS model |
1.0–2.6 | 0.90 ± 0.02 | 0.98 ± 0.02 | 0.37 ± 0.08 | 0.54 ± 0.09 |
2.6–4.8 | 0.90 ± 0.02 | 0.94 ± 0.02 | 0.34 ± 0.09 | 0.33 ± 0.08 |
4.8–7.0 | 0.91 ± 0.02 | 0.94 ± 0.02 | 0.26 ± 0.09 | 0.24 ± 0.10 |
7.0–11.0 | 0.91 ± 0.06 | 0.98 ± 0.06 | 0.47 ± 0.21 | 0.47 ± 0.18 |
11.0–23.0 | 0.88 ± 0.02 | 0.89 ± 0.02 | 0.10 ± 0.10 | 0.12 ± 0.12 |
25.0–35.0 | 0.91 ± 0.03 | 0.96 ± 0.03 | 0.42 ± 0.12 | 0.35 ± 0.11 |
35.0–48.0 | 0.85 ± 0.09 | 0.85 ± 0.23 | 0.42 ± 0.34 | 0.41 ± 0.34 |
48.0–67.0 | 0.93 ± 0.04 | 0.97 ± 0.02 | 0.75 ± 0.09 | 0.36 ± 0.08 |
67.0–100.0 | 0.91 ± 0.02 | 0.95 ± 0.02 | 0.31 ± 0.07 | 0.25 ± 0.06 |
100.0–150.0 | 0.94 ± 0.03 | 1.01 ± 0.03 | 0.45 ± 0.14 | 0.39 ± 0.13 |
150.0–200.0 | 1.01 ± 0.04 | 1.12 ± 0.04 | 0.39 ± 0.14 | 0.65 ± 0.12 |
QPO rms per cent | ||||
Energy band (keV) | Additive PDS model | Multiplicative PDS model | ||
1.0–2.6 | 9.55 ± 1.22 | 10.04 ± 0.58 | ||
2.6–4.8 | 9.47 ± 1.28 | 7.76 ± 0.79 | ||
4.8–7.0 | 8.57 ± 1.65 | 6.63 ± 1.30 | ||
7.0–11.0 | 9.29 ± 1.89 | 7.57 ± 0.98 | ||
11.0–23.0 | 4.53 ± 1.19 | 3.35 ± 2.41 | ||
25.0–35.0 | 8.76 ± 1.44 | 5.93 ± 0.92 | ||
35.0–48.0 | 5.55 ± 2.10 | 6.16 ± 5.68 | ||
48.0–67.0 | 9.42 ± 0.69 | 4.51 ± 0.55 | ||
67.0–100.0 | 6.37 ± 0.88 | 4.04 ± 0.77 | ||
100.0–150.0 | 5.59 ± 1.07 | 3.54 ± 0.71 | ||
150.0–200.0 | 4.43 ± 0.71 | 4.77 ± 0.35 |
. | QPO frequency (Hz) . | QPO FWHM (Hz) . | ||
---|---|---|---|---|
Energy band (keV) | Additive PDS model | Multiplicative PDS model | Additive PDS model | Multiplicative PDS model |
1.0–2.6 | 0.90 ± 0.02 | 0.98 ± 0.02 | 0.37 ± 0.08 | 0.54 ± 0.09 |
2.6–4.8 | 0.90 ± 0.02 | 0.94 ± 0.02 | 0.34 ± 0.09 | 0.33 ± 0.08 |
4.8–7.0 | 0.91 ± 0.02 | 0.94 ± 0.02 | 0.26 ± 0.09 | 0.24 ± 0.10 |
7.0–11.0 | 0.91 ± 0.06 | 0.98 ± 0.06 | 0.47 ± 0.21 | 0.47 ± 0.18 |
11.0–23.0 | 0.88 ± 0.02 | 0.89 ± 0.02 | 0.10 ± 0.10 | 0.12 ± 0.12 |
25.0–35.0 | 0.91 ± 0.03 | 0.96 ± 0.03 | 0.42 ± 0.12 | 0.35 ± 0.11 |
35.0–48.0 | 0.85 ± 0.09 | 0.85 ± 0.23 | 0.42 ± 0.34 | 0.41 ± 0.34 |
48.0–67.0 | 0.93 ± 0.04 | 0.97 ± 0.02 | 0.75 ± 0.09 | 0.36 ± 0.08 |
67.0–100.0 | 0.91 ± 0.02 | 0.95 ± 0.02 | 0.31 ± 0.07 | 0.25 ± 0.06 |
100.0–150.0 | 0.94 ± 0.03 | 1.01 ± 0.03 | 0.45 ± 0.14 | 0.39 ± 0.13 |
150.0–200.0 | 1.01 ± 0.04 | 1.12 ± 0.04 | 0.39 ± 0.14 | 0.65 ± 0.12 |
QPO rms per cent | ||||
Energy band (keV) | Additive PDS model | Multiplicative PDS model | ||
1.0–2.6 | 9.55 ± 1.22 | 10.04 ± 0.58 | ||
2.6–4.8 | 9.47 ± 1.28 | 7.76 ± 0.79 | ||
4.8–7.0 | 8.57 ± 1.65 | 6.63 ± 1.30 | ||
7.0–11.0 | 9.29 ± 1.89 | 7.57 ± 0.98 | ||
11.0–23.0 | 4.53 ± 1.19 | 3.35 ± 2.41 | ||
25.0–35.0 | 8.76 ± 1.44 | 5.93 ± 0.92 | ||
35.0–48.0 | 5.55 ± 2.10 | 6.16 ± 5.68 | ||
48.0–67.0 | 9.42 ± 0.69 | 4.51 ± 0.55 | ||
67.0–100.0 | 6.37 ± 0.88 | 4.04 ± 0.77 | ||
100.0–150.0 | 5.59 ± 1.07 | 3.54 ± 0.71 | ||
150.0–200.0 | 4.43 ± 0.71 | 4.77 ± 0.35 |
. | QPO frequency (Hz) . | QPO FWHM (Hz) . | ||
---|---|---|---|---|
Energy band (keV) | Additive PDS model | Multiplicative PDS model | Additive PDS model | Multiplicative PDS model |
1.0–2.6 | 0.90 ± 0.02 | 0.98 ± 0.02 | 0.37 ± 0.08 | 0.54 ± 0.09 |
2.6–4.8 | 0.90 ± 0.02 | 0.94 ± 0.02 | 0.34 ± 0.09 | 0.33 ± 0.08 |
4.8–7.0 | 0.91 ± 0.02 | 0.94 ± 0.02 | 0.26 ± 0.09 | 0.24 ± 0.10 |
7.0–11.0 | 0.91 ± 0.06 | 0.98 ± 0.06 | 0.47 ± 0.21 | 0.47 ± 0.18 |
11.0–23.0 | 0.88 ± 0.02 | 0.89 ± 0.02 | 0.10 ± 0.10 | 0.12 ± 0.12 |
25.0–35.0 | 0.91 ± 0.03 | 0.96 ± 0.03 | 0.42 ± 0.12 | 0.35 ± 0.11 |
35.0–48.0 | 0.85 ± 0.09 | 0.85 ± 0.23 | 0.42 ± 0.34 | 0.41 ± 0.34 |
48.0–67.0 | 0.93 ± 0.04 | 0.97 ± 0.02 | 0.75 ± 0.09 | 0.36 ± 0.08 |
67.0–100.0 | 0.91 ± 0.02 | 0.95 ± 0.02 | 0.31 ± 0.07 | 0.25 ± 0.06 |
100.0–150.0 | 0.94 ± 0.03 | 1.01 ± 0.03 | 0.45 ± 0.14 | 0.39 ± 0.13 |
150.0–200.0 | 1.01 ± 0.04 | 1.12 ± 0.04 | 0.39 ± 0.14 | 0.65 ± 0.12 |
QPO rms per cent | ||||
Energy band (keV) | Additive PDS model | Multiplicative PDS model | ||
1.0–2.6 | 9.55 ± 1.22 | 10.04 ± 0.58 | ||
2.6–4.8 | 9.47 ± 1.28 | 7.76 ± 0.79 | ||
4.8–7.0 | 8.57 ± 1.65 | 6.63 ± 1.30 | ||
7.0–11.0 | 9.29 ± 1.89 | 7.57 ± 0.98 | ||
11.0–23.0 | 4.53 ± 1.19 | 3.35 ± 2.41 | ||
25.0–35.0 | 8.76 ± 1.44 | 5.93 ± 0.92 | ||
35.0–48.0 | 5.55 ± 2.10 | 6.16 ± 5.68 | ||
48.0–67.0 | 9.42 ± 0.69 | 4.51 ± 0.55 | ||
67.0–100.0 | 6.37 ± 0.88 | 4.04 ± 0.77 | ||
100.0–150.0 | 5.59 ± 1.07 | 3.54 ± 0.71 | ||
150.0–200.0 | 4.43 ± 0.71 | 4.77 ± 0.35 |
. | QPO frequency (Hz) . | QPO FWHM (Hz) . | ||
---|---|---|---|---|
Energy band (keV) | Additive PDS model | Multiplicative PDS model | Additive PDS model | Multiplicative PDS model |
1.0–2.6 | 0.90 ± 0.02 | 0.98 ± 0.02 | 0.37 ± 0.08 | 0.54 ± 0.09 |
2.6–4.8 | 0.90 ± 0.02 | 0.94 ± 0.02 | 0.34 ± 0.09 | 0.33 ± 0.08 |
4.8–7.0 | 0.91 ± 0.02 | 0.94 ± 0.02 | 0.26 ± 0.09 | 0.24 ± 0.10 |
7.0–11.0 | 0.91 ± 0.06 | 0.98 ± 0.06 | 0.47 ± 0.21 | 0.47 ± 0.18 |
11.0–23.0 | 0.88 ± 0.02 | 0.89 ± 0.02 | 0.10 ± 0.10 | 0.12 ± 0.12 |
25.0–35.0 | 0.91 ± 0.03 | 0.96 ± 0.03 | 0.42 ± 0.12 | 0.35 ± 0.11 |
35.0–48.0 | 0.85 ± 0.09 | 0.85 ± 0.23 | 0.42 ± 0.34 | 0.41 ± 0.34 |
48.0–67.0 | 0.93 ± 0.04 | 0.97 ± 0.02 | 0.75 ± 0.09 | 0.36 ± 0.08 |
67.0–100.0 | 0.91 ± 0.02 | 0.95 ± 0.02 | 0.31 ± 0.07 | 0.25 ± 0.06 |
100.0–150.0 | 0.94 ± 0.03 | 1.01 ± 0.03 | 0.45 ± 0.14 | 0.39 ± 0.13 |
150.0–200.0 | 1.01 ± 0.04 | 1.12 ± 0.04 | 0.39 ± 0.14 | 0.65 ± 0.12 |
QPO rms per cent | ||||
Energy band (keV) | Additive PDS model | Multiplicative PDS model | ||
1.0–2.6 | 9.55 ± 1.22 | 10.04 ± 0.58 | ||
2.6–4.8 | 9.47 ± 1.28 | 7.76 ± 0.79 | ||
4.8–7.0 | 8.57 ± 1.65 | 6.63 ± 1.30 | ||
7.0–11.0 | 9.29 ± 1.89 | 7.57 ± 0.98 | ||
11.0–23.0 | 4.53 ± 1.19 | 3.35 ± 2.41 | ||
25.0–35.0 | 8.76 ± 1.44 | 5.93 ± 0.92 | ||
35.0–48.0 | 5.55 ± 2.10 | 6.16 ± 5.68 | ||
48.0–67.0 | 9.42 ± 0.69 | 4.51 ± 0.55 | ||
67.0–100.0 | 6.37 ± 0.88 | 4.04 ± 0.77 | ||
100.0–150.0 | 5.59 ± 1.07 | 3.54 ± 0.71 | ||
150.0–200.0 | 4.43 ± 0.71 | 4.77 ± 0.35 |
. | BBN1 FWHM (Hz) . | BBN2 FWHM (Hz) . | ||
---|---|---|---|---|
Energy band (keV) | Additive PDS model | Multiplicative PDS model | Additive PDS model | Multiplicative PDS model |
1.0–2.6 | 0.48 ± 0.08 | 0.27 ± 0.04 | 3.17 ± 0.51 | 3.01 ± 0.35 |
2.6–4.8 | 0.62 ± 0.19 | 0.28 ± 0.04 | 3.89 ± 0.76 | 3.02 ± 0.44 |
4.8–7.0 | 0.87 ± 0.47 | 0.30 ± 0.06 | 3.70 ± 1.61 | 2.54 ± 0.61 |
7.0–11.0 | 0.54 ± 0.24 | 0.32 ± 0.09 | 10.27 ± 9.14 | 5.56 ± 3.56 |
11.0–23.0 | 0.92 ± 1.92 | 0.42 ± 0.38 | 2.56 ± 1.59 | 2.25 ± 0.44 |
25.0–35.0 | 0.69 ± 0.35 | 0.38 ± 0.07 | 4.70 ± 1.89 | 3.26 ± 0.95 |
35.0–48.0 | 0.58 ± 0.80 | ⋅⋅⋅ | 6.24 ± 15.27 | 2.06 ± 2.09 |
48.0–67.0 | 0.65 ± 0.20 | 0.41 ± 0.05 | 10.83 ± 2.89 | 2.77 ± 0.52 |
67.0–100.0 | 0.69 ± 0.54 | 0.43 ± 0.09 | 2.74 ± 0.82 | 2.44 ± 0.35 |
100.0–150.0 | 0.71 ± 0.45 | 0.50 ± 0.08 | 3.76 ± 1.71 | 3.10 ± 0.87 |
150.0–200.0 | 1.86 ± 0.18 | 0.61 ± 0.08 | 18.88 ± 12.81 | 6.55 ± 2.43 |
BBN1 rms per cent | BBN2 rms per cent | |||
Energy band (keV) | Additive PDS model | Multiplicative PDS model | Additive PDS model | Multiplicative PDS model |
1.0–2.6 | 16.32 ± 1.30 | ⋅⋅⋅ | 20.12 ± 1.18 | 22.07 ± 0.67 |
2.6–4.8 | 14.36 ± 2.21 | ⋅⋅⋅ | 20.56 ± 1.19 | 23.23 ± 0.73 |
4.8–7.0 | 14.40 ± 5.28 | ⋅⋅⋅ | 18.60 ± 3.27 | 22.31 ± 1.29 |
7.0–11.0 | 11.55 ± 2.11 | ⋅⋅⋅ | 15.69 ± 5.61 | 15.50 ± 2.27 |
11.0–23.0 | 21.02 ± 0.75 | ⋅⋅⋅ | 19.22 ± 7.50 | 20.85 ± 1.03 |
25.0–35.0 | 9.87 ± 2.65 | ⋅⋅⋅ | 14.66 ± 1.34 | 16.88 ± 0.92 |
35.0–48.0 | 11.34 ± 1.83 | ⋅⋅⋅ | 7.87 ± 3.83 | 11.38 ± 4.97 |
48.0–67.0 | 8.01 ± 1.10 | ⋅⋅⋅ | 10.53 ± 0.93 | 13.04 ± 0.59 |
67.0–100.0 | 6.19 ± 4.02 | ⋅⋅⋅ | 13.18 ± 1.96 | 14.56 ± 0.56 |
100.0–150.0 | 5.33 ± 2.21 | ⋅⋅⋅ | 9.17 ± 1.30 | 10.51 ± 0.66 |
150.0–200.0 | 10.49 ± 0.56 | ⋅⋅⋅ | 11.46 ± 4.92 | 9.34 ± 0.77 |
. | BBN1 FWHM (Hz) . | BBN2 FWHM (Hz) . | ||
---|---|---|---|---|
Energy band (keV) | Additive PDS model | Multiplicative PDS model | Additive PDS model | Multiplicative PDS model |
1.0–2.6 | 0.48 ± 0.08 | 0.27 ± 0.04 | 3.17 ± 0.51 | 3.01 ± 0.35 |
2.6–4.8 | 0.62 ± 0.19 | 0.28 ± 0.04 | 3.89 ± 0.76 | 3.02 ± 0.44 |
4.8–7.0 | 0.87 ± 0.47 | 0.30 ± 0.06 | 3.70 ± 1.61 | 2.54 ± 0.61 |
7.0–11.0 | 0.54 ± 0.24 | 0.32 ± 0.09 | 10.27 ± 9.14 | 5.56 ± 3.56 |
11.0–23.0 | 0.92 ± 1.92 | 0.42 ± 0.38 | 2.56 ± 1.59 | 2.25 ± 0.44 |
25.0–35.0 | 0.69 ± 0.35 | 0.38 ± 0.07 | 4.70 ± 1.89 | 3.26 ± 0.95 |
35.0–48.0 | 0.58 ± 0.80 | ⋅⋅⋅ | 6.24 ± 15.27 | 2.06 ± 2.09 |
48.0–67.0 | 0.65 ± 0.20 | 0.41 ± 0.05 | 10.83 ± 2.89 | 2.77 ± 0.52 |
67.0–100.0 | 0.69 ± 0.54 | 0.43 ± 0.09 | 2.74 ± 0.82 | 2.44 ± 0.35 |
100.0–150.0 | 0.71 ± 0.45 | 0.50 ± 0.08 | 3.76 ± 1.71 | 3.10 ± 0.87 |
150.0–200.0 | 1.86 ± 0.18 | 0.61 ± 0.08 | 18.88 ± 12.81 | 6.55 ± 2.43 |
BBN1 rms per cent | BBN2 rms per cent | |||
Energy band (keV) | Additive PDS model | Multiplicative PDS model | Additive PDS model | Multiplicative PDS model |
1.0–2.6 | 16.32 ± 1.30 | ⋅⋅⋅ | 20.12 ± 1.18 | 22.07 ± 0.67 |
2.6–4.8 | 14.36 ± 2.21 | ⋅⋅⋅ | 20.56 ± 1.19 | 23.23 ± 0.73 |
4.8–7.0 | 14.40 ± 5.28 | ⋅⋅⋅ | 18.60 ± 3.27 | 22.31 ± 1.29 |
7.0–11.0 | 11.55 ± 2.11 | ⋅⋅⋅ | 15.69 ± 5.61 | 15.50 ± 2.27 |
11.0–23.0 | 21.02 ± 0.75 | ⋅⋅⋅ | 19.22 ± 7.50 | 20.85 ± 1.03 |
25.0–35.0 | 9.87 ± 2.65 | ⋅⋅⋅ | 14.66 ± 1.34 | 16.88 ± 0.92 |
35.0–48.0 | 11.34 ± 1.83 | ⋅⋅⋅ | 7.87 ± 3.83 | 11.38 ± 4.97 |
48.0–67.0 | 8.01 ± 1.10 | ⋅⋅⋅ | 10.53 ± 0.93 | 13.04 ± 0.59 |
67.0–100.0 | 6.19 ± 4.02 | ⋅⋅⋅ | 13.18 ± 1.96 | 14.56 ± 0.56 |
100.0–150.0 | 5.33 ± 2.21 | ⋅⋅⋅ | 9.17 ± 1.30 | 10.51 ± 0.66 |
150.0–200.0 | 10.49 ± 0.56 | ⋅⋅⋅ | 11.46 ± 4.92 | 9.34 ± 0.77 |
. | BBN1 FWHM (Hz) . | BBN2 FWHM (Hz) . | ||
---|---|---|---|---|
Energy band (keV) | Additive PDS model | Multiplicative PDS model | Additive PDS model | Multiplicative PDS model |
1.0–2.6 | 0.48 ± 0.08 | 0.27 ± 0.04 | 3.17 ± 0.51 | 3.01 ± 0.35 |
2.6–4.8 | 0.62 ± 0.19 | 0.28 ± 0.04 | 3.89 ± 0.76 | 3.02 ± 0.44 |
4.8–7.0 | 0.87 ± 0.47 | 0.30 ± 0.06 | 3.70 ± 1.61 | 2.54 ± 0.61 |
7.0–11.0 | 0.54 ± 0.24 | 0.32 ± 0.09 | 10.27 ± 9.14 | 5.56 ± 3.56 |
11.0–23.0 | 0.92 ± 1.92 | 0.42 ± 0.38 | 2.56 ± 1.59 | 2.25 ± 0.44 |
25.0–35.0 | 0.69 ± 0.35 | 0.38 ± 0.07 | 4.70 ± 1.89 | 3.26 ± 0.95 |
35.0–48.0 | 0.58 ± 0.80 | ⋅⋅⋅ | 6.24 ± 15.27 | 2.06 ± 2.09 |
48.0–67.0 | 0.65 ± 0.20 | 0.41 ± 0.05 | 10.83 ± 2.89 | 2.77 ± 0.52 |
67.0–100.0 | 0.69 ± 0.54 | 0.43 ± 0.09 | 2.74 ± 0.82 | 2.44 ± 0.35 |
100.0–150.0 | 0.71 ± 0.45 | 0.50 ± 0.08 | 3.76 ± 1.71 | 3.10 ± 0.87 |
150.0–200.0 | 1.86 ± 0.18 | 0.61 ± 0.08 | 18.88 ± 12.81 | 6.55 ± 2.43 |
BBN1 rms per cent | BBN2 rms per cent | |||
Energy band (keV) | Additive PDS model | Multiplicative PDS model | Additive PDS model | Multiplicative PDS model |
1.0–2.6 | 16.32 ± 1.30 | ⋅⋅⋅ | 20.12 ± 1.18 | 22.07 ± 0.67 |
2.6–4.8 | 14.36 ± 2.21 | ⋅⋅⋅ | 20.56 ± 1.19 | 23.23 ± 0.73 |
4.8–7.0 | 14.40 ± 5.28 | ⋅⋅⋅ | 18.60 ± 3.27 | 22.31 ± 1.29 |
7.0–11.0 | 11.55 ± 2.11 | ⋅⋅⋅ | 15.69 ± 5.61 | 15.50 ± 2.27 |
11.0–23.0 | 21.02 ± 0.75 | ⋅⋅⋅ | 19.22 ± 7.50 | 20.85 ± 1.03 |
25.0–35.0 | 9.87 ± 2.65 | ⋅⋅⋅ | 14.66 ± 1.34 | 16.88 ± 0.92 |
35.0–48.0 | 11.34 ± 1.83 | ⋅⋅⋅ | 7.87 ± 3.83 | 11.38 ± 4.97 |
48.0–67.0 | 8.01 ± 1.10 | ⋅⋅⋅ | 10.53 ± 0.93 | 13.04 ± 0.59 |
67.0–100.0 | 6.19 ± 4.02 | ⋅⋅⋅ | 13.18 ± 1.96 | 14.56 ± 0.56 |
100.0–150.0 | 5.33 ± 2.21 | ⋅⋅⋅ | 9.17 ± 1.30 | 10.51 ± 0.66 |
150.0–200.0 | 10.49 ± 0.56 | ⋅⋅⋅ | 11.46 ± 4.92 | 9.34 ± 0.77 |
. | BBN1 FWHM (Hz) . | BBN2 FWHM (Hz) . | ||
---|---|---|---|---|
Energy band (keV) | Additive PDS model | Multiplicative PDS model | Additive PDS model | Multiplicative PDS model |
1.0–2.6 | 0.48 ± 0.08 | 0.27 ± 0.04 | 3.17 ± 0.51 | 3.01 ± 0.35 |
2.6–4.8 | 0.62 ± 0.19 | 0.28 ± 0.04 | 3.89 ± 0.76 | 3.02 ± 0.44 |
4.8–7.0 | 0.87 ± 0.47 | 0.30 ± 0.06 | 3.70 ± 1.61 | 2.54 ± 0.61 |
7.0–11.0 | 0.54 ± 0.24 | 0.32 ± 0.09 | 10.27 ± 9.14 | 5.56 ± 3.56 |
11.0–23.0 | 0.92 ± 1.92 | 0.42 ± 0.38 | 2.56 ± 1.59 | 2.25 ± 0.44 |
25.0–35.0 | 0.69 ± 0.35 | 0.38 ± 0.07 | 4.70 ± 1.89 | 3.26 ± 0.95 |
35.0–48.0 | 0.58 ± 0.80 | ⋅⋅⋅ | 6.24 ± 15.27 | 2.06 ± 2.09 |
48.0–67.0 | 0.65 ± 0.20 | 0.41 ± 0.05 | 10.83 ± 2.89 | 2.77 ± 0.52 |
67.0–100.0 | 0.69 ± 0.54 | 0.43 ± 0.09 | 2.74 ± 0.82 | 2.44 ± 0.35 |
100.0–150.0 | 0.71 ± 0.45 | 0.50 ± 0.08 | 3.76 ± 1.71 | 3.10 ± 0.87 |
150.0–200.0 | 1.86 ± 0.18 | 0.61 ± 0.08 | 18.88 ± 12.81 | 6.55 ± 2.43 |
BBN1 rms per cent | BBN2 rms per cent | |||
Energy band (keV) | Additive PDS model | Multiplicative PDS model | Additive PDS model | Multiplicative PDS model |
1.0–2.6 | 16.32 ± 1.30 | ⋅⋅⋅ | 20.12 ± 1.18 | 22.07 ± 0.67 |
2.6–4.8 | 14.36 ± 2.21 | ⋅⋅⋅ | 20.56 ± 1.19 | 23.23 ± 0.73 |
4.8–7.0 | 14.40 ± 5.28 | ⋅⋅⋅ | 18.60 ± 3.27 | 22.31 ± 1.29 |
7.0–11.0 | 11.55 ± 2.11 | ⋅⋅⋅ | 15.69 ± 5.61 | 15.50 ± 2.27 |
11.0–23.0 | 21.02 ± 0.75 | ⋅⋅⋅ | 19.22 ± 7.50 | 20.85 ± 1.03 |
25.0–35.0 | 9.87 ± 2.65 | ⋅⋅⋅ | 14.66 ± 1.34 | 16.88 ± 0.92 |
35.0–48.0 | 11.34 ± 1.83 | ⋅⋅⋅ | 7.87 ± 3.83 | 11.38 ± 4.97 |
48.0–67.0 | 8.01 ± 1.10 | ⋅⋅⋅ | 10.53 ± 0.93 | 13.04 ± 0.59 |
67.0–100.0 | 6.19 ± 4.02 | ⋅⋅⋅ | 13.18 ± 1.96 | 14.56 ± 0.56 |
100.0–150.0 | 5.33 ± 2.21 | ⋅⋅⋅ | 9.17 ± 1.30 | 10.51 ± 0.66 |
150.0–200.0 | 10.49 ± 0.56 | ⋅⋅⋅ | 11.46 ± 4.92 | 9.34 ± 0.77 |
Timing properties of slq, slnq, shq, and shnq (see Section 3 for their definition).
Signals . | Bin size (s) . | Mean rate (counts s−1) . | Exposure (s) . | Fractional rms . | PDS type . | FDPLS type . |
---|---|---|---|---|---|---|
slq | 0.03125 | 142.5 | 8000 | 27.00 per cent | QPO | Dip |
shq | 0.03125 | 63.5 | 8000 | 9.43 per cent | QPO | |
slnq | 0.03125 | 142.5 | 8000 | 51.00 per cent | Non-QPO | Constant |
shnq | 0.03125 | 63.5 | 8000 | 15.70 per cent | Non-QPO |
Signals . | Bin size (s) . | Mean rate (counts s−1) . | Exposure (s) . | Fractional rms . | PDS type . | FDPLS type . |
---|---|---|---|---|---|---|
slq | 0.03125 | 142.5 | 8000 | 27.00 per cent | QPO | Dip |
shq | 0.03125 | 63.5 | 8000 | 9.43 per cent | QPO | |
slnq | 0.03125 | 142.5 | 8000 | 51.00 per cent | Non-QPO | Constant |
shnq | 0.03125 | 63.5 | 8000 | 15.70 per cent | Non-QPO |
Timing properties of slq, slnq, shq, and shnq (see Section 3 for their definition).
Signals . | Bin size (s) . | Mean rate (counts s−1) . | Exposure (s) . | Fractional rms . | PDS type . | FDPLS type . |
---|---|---|---|---|---|---|
slq | 0.03125 | 142.5 | 8000 | 27.00 per cent | QPO | Dip |
shq | 0.03125 | 63.5 | 8000 | 9.43 per cent | QPO | |
slnq | 0.03125 | 142.5 | 8000 | 51.00 per cent | Non-QPO | Constant |
shnq | 0.03125 | 63.5 | 8000 | 15.70 per cent | Non-QPO |
Signals . | Bin size (s) . | Mean rate (counts s−1) . | Exposure (s) . | Fractional rms . | PDS type . | FDPLS type . |
---|---|---|---|---|---|---|
slq | 0.03125 | 142.5 | 8000 | 27.00 per cent | QPO | Dip |
shq | 0.03125 | 63.5 | 8000 | 9.43 per cent | QPO | |
slnq | 0.03125 | 142.5 | 8000 | 51.00 per cent | Non-QPO | Constant |
shnq | 0.03125 | 63.5 | 8000 | 15.70 per cent | Non-QPO |
sl PDS model . | sh PDS model . | FDPLS model . | |||
---|---|---|---|---|---|
Parameter name . | Value . | Parameter name . | Value . | Parameter name . | Value . |
K1 | 0.007 ± 0.002 | K1 | 0.001 ± 0.000 | A1 | −0.109 ± 0.015 |
fc1 | 0.442 ± 0.008 | fc1 | 0.430 ± 0.010 | μ1 | 0.405 ± 0.011 |
ω1 | 0.119 ± 0.033 | ω1 | 0.090 ± 0.030 | σ1 | 0.062 ± 0.008 |
K2 | 0.009 ± 0.002 | K2 | 0.001 ± 0.000 | A2 | −0.204 ± 0.041 |
fc2 | 0.900 ± 0.020 | fc2 | 0.860 (frozen) | μ2 | 0.776 ± 0.037 |
ω2 | 0.370 ± 0.083 | ω2 | 0.200 (frozen) | σ2 | 0.185 ± 0.024 |
K3 | 0.027 ± 0.004 | K3 | 0.006 (frozen) | c | 1.102 ± 0.040 |
fc3 | 0.000 (frozen) | fc3 | 0.000 (frozen) | ⋅⋅⋅ | ⋅⋅⋅ |
ω3 | 0.477 ± 0.080 | ω3 | 2.061 ± 0.425 | ⋅⋅⋅ | ⋅⋅⋅ |
K4 | 0.040 ± 0.005 | c | 0.016 ± 0.000 | ⋅⋅⋅ | ⋅⋅⋅ |
fc4 | 0.000 (frozen) | ⋅⋅⋅ | ⋅⋅⋅ | ⋅⋅⋅ | ⋅⋅⋅ |
ω4 | 3.175 ± 0.512 | ⋅⋅⋅ | ⋅⋅⋅ | ⋅⋅⋅ | ⋅⋅⋅ |
c | 0.007 ± 0.000 | ⋅⋅⋅ | ⋅⋅⋅ | ⋅⋅⋅ | ⋅⋅⋅ |
sl PDS model . | sh PDS model . | FDPLS model . | |||
---|---|---|---|---|---|
Parameter name . | Value . | Parameter name . | Value . | Parameter name . | Value . |
K1 | 0.007 ± 0.002 | K1 | 0.001 ± 0.000 | A1 | −0.109 ± 0.015 |
fc1 | 0.442 ± 0.008 | fc1 | 0.430 ± 0.010 | μ1 | 0.405 ± 0.011 |
ω1 | 0.119 ± 0.033 | ω1 | 0.090 ± 0.030 | σ1 | 0.062 ± 0.008 |
K2 | 0.009 ± 0.002 | K2 | 0.001 ± 0.000 | A2 | −0.204 ± 0.041 |
fc2 | 0.900 ± 0.020 | fc2 | 0.860 (frozen) | μ2 | 0.776 ± 0.037 |
ω2 | 0.370 ± 0.083 | ω2 | 0.200 (frozen) | σ2 | 0.185 ± 0.024 |
K3 | 0.027 ± 0.004 | K3 | 0.006 (frozen) | c | 1.102 ± 0.040 |
fc3 | 0.000 (frozen) | fc3 | 0.000 (frozen) | ⋅⋅⋅ | ⋅⋅⋅ |
ω3 | 0.477 ± 0.080 | ω3 | 2.061 ± 0.425 | ⋅⋅⋅ | ⋅⋅⋅ |
K4 | 0.040 ± 0.005 | c | 0.016 ± 0.000 | ⋅⋅⋅ | ⋅⋅⋅ |
fc4 | 0.000 (frozen) | ⋅⋅⋅ | ⋅⋅⋅ | ⋅⋅⋅ | ⋅⋅⋅ |
ω4 | 3.175 ± 0.512 | ⋅⋅⋅ | ⋅⋅⋅ | ⋅⋅⋅ | ⋅⋅⋅ |
c | 0.007 ± 0.000 | ⋅⋅⋅ | ⋅⋅⋅ | ⋅⋅⋅ | ⋅⋅⋅ |
sl PDS model . | sh PDS model . | FDPLS model . | |||
---|---|---|---|---|---|
Parameter name . | Value . | Parameter name . | Value . | Parameter name . | Value . |
K1 | 0.007 ± 0.002 | K1 | 0.001 ± 0.000 | A1 | −0.109 ± 0.015 |
fc1 | 0.442 ± 0.008 | fc1 | 0.430 ± 0.010 | μ1 | 0.405 ± 0.011 |
ω1 | 0.119 ± 0.033 | ω1 | 0.090 ± 0.030 | σ1 | 0.062 ± 0.008 |
K2 | 0.009 ± 0.002 | K2 | 0.001 ± 0.000 | A2 | −0.204 ± 0.041 |
fc2 | 0.900 ± 0.020 | fc2 | 0.860 (frozen) | μ2 | 0.776 ± 0.037 |
ω2 | 0.370 ± 0.083 | ω2 | 0.200 (frozen) | σ2 | 0.185 ± 0.024 |
K3 | 0.027 ± 0.004 | K3 | 0.006 (frozen) | c | 1.102 ± 0.040 |
fc3 | 0.000 (frozen) | fc3 | 0.000 (frozen) | ⋅⋅⋅ | ⋅⋅⋅ |
ω3 | 0.477 ± 0.080 | ω3 | 2.061 ± 0.425 | ⋅⋅⋅ | ⋅⋅⋅ |
K4 | 0.040 ± 0.005 | c | 0.016 ± 0.000 | ⋅⋅⋅ | ⋅⋅⋅ |
fc4 | 0.000 (frozen) | ⋅⋅⋅ | ⋅⋅⋅ | ⋅⋅⋅ | ⋅⋅⋅ |
ω4 | 3.175 ± 0.512 | ⋅⋅⋅ | ⋅⋅⋅ | ⋅⋅⋅ | ⋅⋅⋅ |
c | 0.007 ± 0.000 | ⋅⋅⋅ | ⋅⋅⋅ | ⋅⋅⋅ | ⋅⋅⋅ |
sl PDS model . | sh PDS model . | FDPLS model . | |||
---|---|---|---|---|---|
Parameter name . | Value . | Parameter name . | Value . | Parameter name . | Value . |
K1 | 0.007 ± 0.002 | K1 | 0.001 ± 0.000 | A1 | −0.109 ± 0.015 |
fc1 | 0.442 ± 0.008 | fc1 | 0.430 ± 0.010 | μ1 | 0.405 ± 0.011 |
ω1 | 0.119 ± 0.033 | ω1 | 0.090 ± 0.030 | σ1 | 0.062 ± 0.008 |
K2 | 0.009 ± 0.002 | K2 | 0.001 ± 0.000 | A2 | −0.204 ± 0.041 |
fc2 | 0.900 ± 0.020 | fc2 | 0.860 (frozen) | μ2 | 0.776 ± 0.037 |
ω2 | 0.370 ± 0.083 | ω2 | 0.200 (frozen) | σ2 | 0.185 ± 0.024 |
K3 | 0.027 ± 0.004 | K3 | 0.006 (frozen) | c | 1.102 ± 0.040 |
fc3 | 0.000 (frozen) | fc3 | 0.000 (frozen) | ⋅⋅⋅ | ⋅⋅⋅ |
ω3 | 0.477 ± 0.080 | ω3 | 2.061 ± 0.425 | ⋅⋅⋅ | ⋅⋅⋅ |
K4 | 0.040 ± 0.005 | c | 0.016 ± 0.000 | ⋅⋅⋅ | ⋅⋅⋅ |
fc4 | 0.000 (frozen) | ⋅⋅⋅ | ⋅⋅⋅ | ⋅⋅⋅ | ⋅⋅⋅ |
ω4 | 3.175 ± 0.512 | ⋅⋅⋅ | ⋅⋅⋅ | ⋅⋅⋅ | ⋅⋅⋅ |
c | 0.007 ± 0.000 | ⋅⋅⋅ | ⋅⋅⋅ | ⋅⋅⋅ | ⋅⋅⋅ |
We then simulate four subsignals based on the best FDPLS and PDS models obtained above. The PDS of the QPO component is modelled using the sum of the non-zero-centred Lorentzian components and the PDS of the BBN component is modelled using the sum of the zero-centred Lorentzian components. We first simulate four signals using TK95 algorithm, noted as slq, slnq, shq, and shnq, which stand for the QPO component and the BBN component in the reference energy band, and the QPO component and the BBN component in the high-energy band, respectively. After that, we use the algorithm proposed in Section 2.3 to make the FDPLS of slq and shq satisfy the Gaussian components of the best-fitting model and make the FDPLS of slnq and shnq satisfy the constant component of the best-fitting model. The timing properties of these four signals are listed in Table 6. Note that in calculating the FDPLS we split the signal into QPO and non-QPO components, which in effect assumes that the contribution of the additive component of equation (20) to the overall FDPLS can be neglected, i.e. the destruction of this additive component to the additivity of the phase lag between the convolution components can be neglected, as we will explain in detail in the subsequent discussion section. The PDS of slq, slnq, shq, and shnq is shown in top panel of Fig. 7. The FDPLS between slq and shq are two dips, and the FDPLS between slnq and shnq are constant, which are shown in the middle panel of Fig. 7.
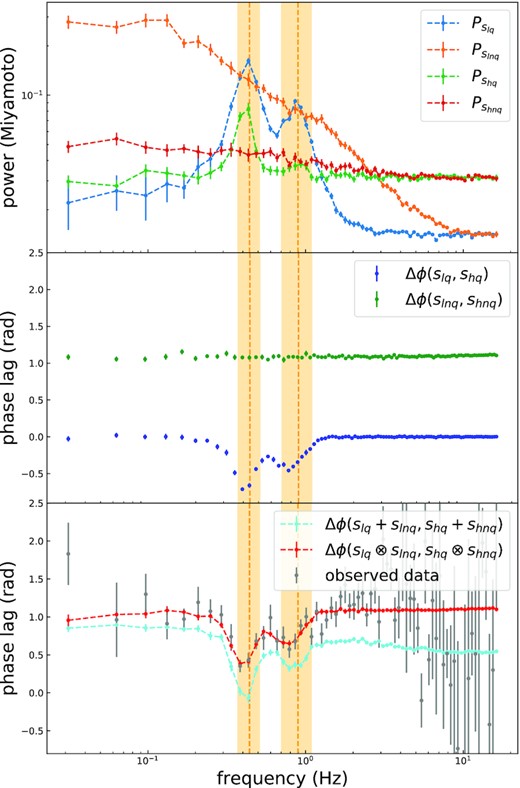
Simulation results based on the data modelling shown in Fig. 6. Upper panel: the simulated PDS of the signals slq, slnq, shq, and shnq. Middle panel: the simulated FDPLS (blue dots) of slq and shq and the simulated FDPLS (green dots) of slnq and shnq. Bottom panel: the calculated FDPLS of the total signal when the total signal is an/a additive/convolved signal (cyan/red data points). The grey dots are the observed FDPLS. The yellow bands running through panels are the QPO FWHM frequency ranges (including fundamental and harmonic frequencies). Error bars correspond to 1σ confidence intervals.
Then, slq and slnq are added/convolved to get the additive/convolved signal. shq and shnq are added/convolved to get the other additive/convolved signal. The FDPLS of the additive/convolved signals is shown by the cyan/red dotted lines in the lower panel of Fig. 7. The grey dots in the figure are the observed data. As can be seen from Fig. 7, the simulated results for the additive signal are very different from those given by the data. But the simulated results for the convolved signal match the data perfectly. This simulation result indicates that the observed data can distinguish between convolved and additive signals for FDPLS.
4 DISCUSSION AND SUMMARY
In this paper, we investigate the mechanism behind the phase lag correction that was successfully applied for the first time by Ma21 for MAXI J1820+070, where the strong BBN and the QPO coexist. After correcting the phase lag of the QPO, the absolute value of QPO phase lag increases monotonically with photon energy in all observations. Ma21 explained the phase lag behaviour of the QPO by employing a compact jet with precession. In this scenario, the high-energy photons come from the part of the jet closer to the black hole, and the precession of the compact jet causes the QPO phenomenon and allows the high-energy photons to reach the observer first, resulting in a soft lag. Because the phase lag behaviour can have a large impact on physical conclusions, it is necessary to investigate the rationality of this correction method. Since we want to obtain the intrinsic properties of the QPO, and what we observe is some kind of superposition of the QPO and the BBN components, we have to face the question of how these components constitute the total signal. We found that the correction method is effective only when the subsignals are synthesized into the total signal by convolution. If the total signal is the convolved signal, the intrinsic phase lags of the QPO can be obtained by subtracting the phase lags of the BBN component from the original phase lags of the total signal, as successfully implemented in Ma21.
If the observed total signals are convolved signals, the corresponding PDS cannot be fitted simply by summing a series of Lorentzian functions (conventions in most of the literatures) but require a multiplicative PDS model. We then try to introduce the convolution mechanism by assuming the propagation of the QPO waves in the corona (may be due to the magnetoacoustic wave propagating within the corona; e.g. Cabanac et al. 2010). The fluctuation propagation in the form of Dirac delta function resulting in Green’s function. Any form of timing fluctuation will be the convolution of that fluctuation and Green’s function (Ingram 2016). We assume that Green’s function is first convolved with the white noise and then convolved with the QPO signal to form the low-frequency part of the observed signal, while the high-frequency part is the result of the convolution of Green’s function with the two white noise components. If Green’s function and the QPO signal are convoluted in the time domain, the total PDS will be the multiplication of their respective PDS according to the convolution theorem. Based on this, we introduce a multiplicative PDS model to fit the observed PDS in a representative Insight-HXMT observation in 11 different energy bands. For comparison, we also fitted the same data using the traditional additive PDS model. Overall, both additive and multiplicative PDS models fit the observed data well, but the individual components have some differences. The two models give little difference in the centroid frequency and in the FWHM of the QPO. For the fundamental frequency component of the QPO, the fraction of rms of the QPO given by the traditional additive PDS model is about 2–3 higher than that of the multiplicative PDS model, but the trend is the same for both results. For the harmonic frequency component of QPO, the fractional rms given by the two models is not significantly different.
For the traditional additive PDS model, the low-frequency zero-centred Lorentzian component can be considered as the variability due to the propagation of the white noise fluctuation in the outer region of the corona (Ingram & Done 2011), the narrow Lorentzian components stand for the fundamental and harmonic components of the QPO, and the high-frequency zero-centred Lorentzian component is responsible for the variability due to the propagation of the white noise fluctuation in the inner region of the corona (Ingram & Done 2011). All these terms are simply added together, which means that there is no coherence between them.
For the multiplicative PDS model, we find that the low-frequency component of the PDS can be fitted by multiplying the fundamental and harmonic components of the QPO with a zero-centred Lorentzian function, in addition to an additional additive component to produce the high-frequency part of the PDS. The additive Lorentzian component plays the same as the role in the additive PDS model. Therefore, for our multiplicative model, the high-frequency part does not need to be convolved to the QPO signal, but is simply added together. This additive component appearing in the PDS model looks to destroy the additivity of the phase lag brought about by the time domain convolution. We ignored the contribution of this additive component in our previous phase lag calculations. We make a simulation to investigate the effect of this additional additive component on the total FDPLS. Because of the dependence of the FDPLS of additive signals on the PDS of the individual components, we need to know the PDS parameters of each component. Specifically, we first obtain the PDS parameters for each component based on the results of the fit of the multiplicative PDS model (shown in the right-hand panels of Fig. 4), and then simulate four signals based on the mean of these parameters in two energy bands: x1 (the convolved signal of energy band 1), y1 (the additional additive signal of energy band 1), x2 (the convolved signal of energy band 2), and y2 (the additional additive signal of energy band 2). The PDS of these four signals is shown in the upper panel of Fig. 8. We then set the FDPLS model of x1 and x2 to |$\phi (f)=-0.5\, {\rm e}^{-\frac{(f-0.4)^2}{0.1^2}}-0.3\, {\rm e}^{-\frac{(f-0.8)^2}{0.15^2}}+1$| and set the FDPLS model of y1 and y2 to 1. Finally, we calculate the FDPLS of x1 and x2 and the FDPLS of x1 + y1 and x2 + y2, respectively. By comparing these two FDPLS, the effect of the additional additive component on the total FDPLS can be known. As can be seen from the lower panel of Fig. 8, the additional additive component has almost no effect on the total FDPLS, except for a slight dilution of the phase lag of the harmonic component. It is therefore reasonable to ignore the effect of the additional additive component on the FDPLS of the convolution components in our previous analysis.
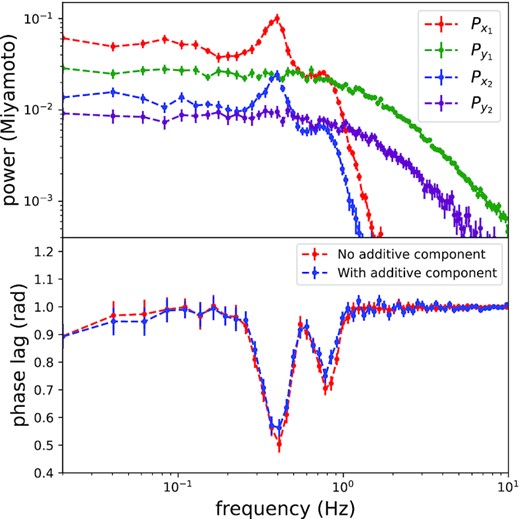
The effect of an additional additive component on the total FDPLS. Upper panel: PDS of simulated signals. Lower panel: FDPLS with or without an additional additive component.
Traditionally, it is mostly assumed that the observed components are additive in the time domain, and it has also been suggested that it might be more reasonable to multiply these components in the time domain based on the fluctuation propagation model (e.g. Ingram & van der Klis 2013). However, neither of these two models can explain the phase lag correction in Ma21. In order to explain the correction of phase lags in Ma21, we propose a convolution model instead of the additive and multiplicative models in the time domain, which is supported by comparison between simulations and data on both PDS and FDPLS. This suggests that the convolution model can explain the behaviour of the phase lag observed in MAXI J1820+070, in which case the phase lag correction method applied in Ma21 is correct.
Finally, it is worth pointing out that our current convolution model still has limitations. For examples, it is not yet possible to explain the energy dependence of the phase lag using the convolution model, and the relationship of individual components to specific physical processes needs further development. However, it is certain that at least part of the time domain signal is filtered by the system before it reaches the observer (e.g. both the accretion disc and corona/jet play the role of low-pass filters to some extent), and these response processes are necessarily accompanied by time domain convolution operations.
ACKNOWLEDGEMENTS
This research has made use of the data from the Insight-HXMT mission, a project funded by China National Space Administration and the Chinese Academy of Sciences. This work is supported by the National Natural Science Foundation of China under grants 12133007, U1838201, and U1938201.
DATA AVAILABILITY
The data used in this paper can be found in the Insight-HXMT website (http://hxmtweb.ihep.ac.cn/).
Footnotes
The fractional rms is calculated in the same way as for Bu et al. (2015), but ignoring the background correction, since the correction coefficients are the same for both models and our aim is only to compare their differences. Neglecting the background correction leads to a lower fractional rms for the energy band with a lower signal-to-noise ratio (in this paper it is the higher energy band), but it does not change our conclusion.