-
PDF
- Split View
-
Views
-
Cite
Cite
J. Dorval, C. M. Boily, E. Moraux, O. Roos, Wide- and contact-binary formation in substructured young stellar clusters, Monthly Notices of the Royal Astronomical Society, Volume 465, Issue 2, February 2017, Pages 2198–2211, https://doi.org/10.1093/mnras/stw2880
- Share Icon Share
Abstract
We explore with collisional gravitational N-body models the evolution of binary stars in initially fragmented and globally subvirial clusters of stars. Binaries are inserted in the (initially) clumpy configurations so as to match the observed distributions of the field-binary-stars’ semimajor axes a and binary fraction versus primary mass. The dissolution rate of wide binaries is very high at the start of the simulations, and is much reduced once the clumps are eroded by the global infall. The transition between the two regimes is sharper as the number of stars N is increased, from N = 1.5 k up to 80 k. The fraction of dissolved binary stars increases only mildly with N, from ≈15 per cent to ≈25 per cent for the same range in N. We repeated the calculation for two initial system mean number densities of 6 per pc3 (low) and 400 per pc3 (high). We found that the longer free-fall time of the low-density runs allows for prolonged binary–binary interactions inside clumps and the formation of very tight (a ≈ 0.01 au) binaries by exchange collisions. This is an indication that the statistics of such compact binaries bear a direct link to their environment at birth. We also explore the formation of wide (a ≳ 5 × 104 au) binaries and find a low (≈0.01 per cent) fraction mildly bound to the central star cluster. The high-precision astrometric mission Gaia could identify them as outflowing shells or streams.
1 INTRODUCTION
The properties of stellar populations at birth hold a key to their long-term evolution as clusters or associations. Whether a stellar system will remain bound or undergo rapid dissolution hinges on the global star formation efficiency, the phase-space statistics of the stars, and the demographics of the type of stars involved (single or multiple; see e.g. Portegies Zwart, McMillan & Gieles 2010 for a synthetic review). The properties of binary stars in dense stellar associations in particular may shed light on the discovery of multiple star-formation episodes in rich stellar clusters (Anderson et al. 2009). For instance, binary stars enhance strong dynamical interactions which in turn may speed-up evolution off the main sequence and thus boost enrichment of the interstellar medium through winds (e.g. Tailo et al. 2015). Tight binaries of short-lived massive stars may evolve to produce exotic stellar remnants including black hole progenitors (Bacon, Sigurdsson & Davies 1996; Davies et al. 2009).
That gravitational dynamics should play a role early-on in the life of a star cluster is often illustrated by comparison of a low-density environment of irregular geometry such as the Taurus Aurigae region, to the more symmetrically shaped and denser Orion Nebula Cluster (ONC, e.g. Da Rio, Tan & Jaehnig 2014). Another example comes from APO Galactic Evolution Experiment spectroscopic observations of NGC1333 showing that main-sequence stars have much larger velocity vectors than candidate stellar cores, a strong indication that the velocity field develops on the same Myr time-scale as star formation (Foster et al. 2015). Thus the assembly of a cluster as a whole may proceed over time in a hierarchical fashion, and undergo a phase of dynamical relaxation to virial equilibrium. The rapid, global merging of substructures would bring stars together at a different stage of their formation (as in NGC1333), while inducing a shift from a clumpy Taurus-like profile to a more regular one. A simple but important question is how the internal dynamics of such complex configurations may affect the characteristics of a population of binary stars.
Many authors have explored this question through optimized initial conditions (Kroupa & Burkert 2001; Marks & Kroupa 2012) or fractal configurations evolved with N-body integrators (Goodwin & Whitworth 2004; Allison et al. 2009; Parker, Goodwin & Allison 2011; Parker & Meyer 2014). A recurring feature of all these studies is that the binary fraction drops over time regardless of their components (masses), due, e.g., to close star–star encounters or heating from the external galactic tidal field (though in specific cases, such as supervirial substructured systems, the binary fraction may be constant, see e.g. Moeckel & Clarke 2011). Another common result is that large-separation, weakly bound binaries are preferentially destroyed during dynamical evolution. Vesperini & Chernoff (1996) explored the fate of binaries in violently relaxing uniform systems. Comparing the internal velocity dispersion of the pairs and that of the cluster, they showed their soft binaries were destroyed, though it had no significant impact on the global structure of the cluster.
Parker & Meyer (2014) pointed out that the distribution of semimajor axes a of the field population is a strong function of the primary's mass: at fixed a, low-mass binaries carry less binding energy so the distribution cuts off at shorter separation (∼20 au) than for binaries with a more massive primary (∼300 au). Their study of fractal initial conditions shows that gravitational dynamics enhances the dissolution of low-mass systems. This then provides a clue to account for the larger relative fraction of heavy stars in binaries, such as seen in a compilation by Raghavan et al. (2010).
We note that hydrodynamical calculations of star formation have found young heavy stars to be preferentially hosted in dense clumps (Maschberger et al. 2010). Furthermore, it is not clear yet whether binary populations should be tailored according to the system total mass because of the limited range of M ∼ 102 to ∼103 M⊙ of these studies (Kroupa & Burkert 2001; Parker et al. 2011; Parker & Meyer 2014). Recall that the intensity of the tidal field is a prime agent of binary heating. A trend with mass may be expected on the ground that the drive to equilibrium of more massive systems leads to deeper potential wells (e.g. Aarseth, Lin & Papaloizou 1988; Boily, Athanassoula & Kroupa 2002). A steep potential will give rise to strong tidal fields which may disrupt bound subsystems (Boily et al. 2004; Renaud, Gieles & Boily 2011). A definitive assessment of this effect is difficult to reach because the results are a strong function of the system's initial-mass distribution and kinetic-energy content (Boily et al. 2002; Caputo, de Vries & Portegies Zwart 2014).
In this contribution, we revisit this issue but through initial conditions built from the self-consistent Hubble–Lemaître (HL) fragmentation of a star cluster (Dorval et al. 2016). The clumpy mass profile has both a self-consistent velocity field and built-in mass segregation (more massive stars on average in more massive clumps). We ask how the characteristics of the binaries evolved from such models compared with those of the well-sampled field-binary population (Duquennoy & Mayor 1991; Raghavan et al. 2010). A basic expectation is that evolution of massive binaries in dense clumps will enhance mass segregation while boosting the dissolution of the wider binaries on a very short time-scale (e.g. Bacon et al. 1996; Aarseth 2003; Marks, Kroupa & Oh 2011).
In Sections 2 and 3, we recall the basic procedure to obtain initial conditions for stellar dynamics and how the binary population was created. We present our data sets and main results in Section 4. In Section 5, we discuss the formation of very tight binaries (separation a of the order of 10−2 au) on a few Myr time-scale. We sum up our findings and address open questions in Section 6.
2 INITIAL CONDITIONS FROM HUBBLE–LEMAîTRE FRAGMENTATION
2.1 Initial setup
Dorval et al. (2016) pointed out that fragmentation modes of self-gravitation developed in expanding coordinates lead to substructured mass distributions that have characteristics similar to star-forming regions (i.e. fragments mass function, stellar contents). This ‘cosmological’ HL expansion phase is interpreted as global pressure support mimicking the action of turbulence and magnetic fields, which both delay the large-scale collapse of the self-bound system (e.g. Mac Low & Klessen 2004), yet allow for small-scale density enhancements to grow. A self-consistent fragmented state is reached when the expansion stops (Dorval et al. 2016; see Fig. 1). That configuration is then used as initial condition to explore the stellar dynamical evolution. The initial HL expansion parameter |${\cal H}_{\rm o} > 0,$| so the vector field is simply |$\boldsymbol {v} = {\cal H}_{\rm o} \boldsymbol {x}$|, with the positions |$\boldsymbol {x}$| drawn from a uniform sphere. For the current work, we performed a large set of runs of HL fragmentation, listed in Table 1.
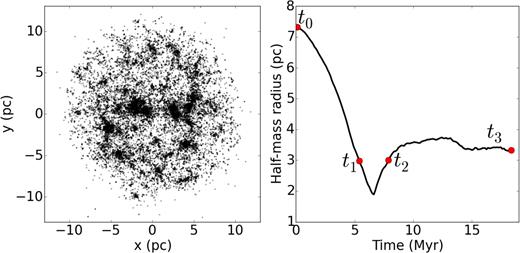
Left-hand panel: spatial distribution of a Hubble–Lemaître fragmented model. Right-hand panel: evolution of the half-mass radius over time. The times labelled as t0–t3 are the system configurations used in Section 4 for analysis. The model presented here is scaled for the lowest of the two initial stellar densities, 6 stars per pc3, has an initial half-mass radius of 7 pc, and is evolved up to 18 Myr. The denser model, starting with 400 stars per pc3, has the same aspect and evolution, with an initial half-mass radius of 1.8 pc and is evolved up to 2.3 Myr.
Summary of simulations. Starting from an HL fragmented configuration, a binary population was injected to complete the spontaneous binaries, reaching an overall binary fraction of 0.42. Densities within half-mass radius are shown at t = 0 and time of deepest collapse.
Name . | N . | Sampling . | ρh,0 (pc−3) . | ρh,max (pc−3) . |
---|---|---|---|---|
R1.5h | 1500 | 40 | 400 | 1.7 × 103 |
R5h | 5000 | 10 | 400 | 4.0 × 103 |
R20h | 20 000 | 10 | 400 | 1.4 × 104 |
R80h | 80 000 | 1 | 400 | 7.1 × 104 |
R1.5l | 1500 | 40 | 6 | 13 |
R5l | 5000 | 10 | 6 | 79 |
R20l | 20 000 | 10 | 6 | 192 |
R80l | 80 000 | 1 | 6 | 103 |
Name . | N . | Sampling . | ρh,0 (pc−3) . | ρh,max (pc−3) . |
---|---|---|---|---|
R1.5h | 1500 | 40 | 400 | 1.7 × 103 |
R5h | 5000 | 10 | 400 | 4.0 × 103 |
R20h | 20 000 | 10 | 400 | 1.4 × 104 |
R80h | 80 000 | 1 | 400 | 7.1 × 104 |
R1.5l | 1500 | 40 | 6 | 13 |
R5l | 5000 | 10 | 6 | 79 |
R20l | 20 000 | 10 | 6 | 192 |
R80l | 80 000 | 1 | 6 | 103 |
Summary of simulations. Starting from an HL fragmented configuration, a binary population was injected to complete the spontaneous binaries, reaching an overall binary fraction of 0.42. Densities within half-mass radius are shown at t = 0 and time of deepest collapse.
Name . | N . | Sampling . | ρh,0 (pc−3) . | ρh,max (pc−3) . |
---|---|---|---|---|
R1.5h | 1500 | 40 | 400 | 1.7 × 103 |
R5h | 5000 | 10 | 400 | 4.0 × 103 |
R20h | 20 000 | 10 | 400 | 1.4 × 104 |
R80h | 80 000 | 1 | 400 | 7.1 × 104 |
R1.5l | 1500 | 40 | 6 | 13 |
R5l | 5000 | 10 | 6 | 79 |
R20l | 20 000 | 10 | 6 | 192 |
R80l | 80 000 | 1 | 6 | 103 |
Name . | N . | Sampling . | ρh,0 (pc−3) . | ρh,max (pc−3) . |
---|---|---|---|---|
R1.5h | 1500 | 40 | 400 | 1.7 × 103 |
R5h | 5000 | 10 | 400 | 4.0 × 103 |
R20h | 20 000 | 10 | 400 | 1.4 × 104 |
R80h | 80 000 | 1 | 400 | 7.1 × 104 |
R1.5l | 1500 | 40 | 6 | 13 |
R5l | 5000 | 10 | 6 | 79 |
R20l | 20 000 | 10 | 6 | 192 |
R80l | 80 000 | 1 | 6 | 103 |
2.2 Simulations
Each model was set up with stars drawn from the L3 single star initial mass function (IMF) of Maschberger (2013). The L3 IMF matches the better-known Kroupa (2001) and Chabrier (2003) functional forms but with fewer free parameters. We set a lower truncation mass of 0.1 M⊙ and a maximum of 30 M⊙, for an average mass of 0.5 M⊙. This choice of truncation values allows us to take into account the impact of massive stars on the dynamics, while keeping the vast majority of stars on the main sequence for up to 18 Myr, the maximum simulation runtime. Simulations were therefore performed without stellar evolution. All phase-space coordinates were scaled to standard Hénon N-body computational units (Heggie 1975; Heggie & Mathieu 1986). In those units, G is set to unity, the total system energy E = −1/4, system mass M = 1, and global 1D velocity dispersion |$\sigma = 1/\sqrt{3}$| (exact for single-mass models). The initial velocity field of the HL fragmentation was set to |$\tilde{H}_{\rm o} = 1.2$| (dimensionless computational units). All simulations were run for t = 30 units of time.
All integrations were done with the stellar dynamics code nbody6 (Aarseth 2003; Renaud et al. 2011; Nitadori & Aarseth 2012) for the efficient treatment of tight binaries. We analyse in the next section the population of binary stars in HL configurations before turning to their time evolution.
3 THE BINARY POPULATION
In this section, we first introduce our binary-detection algorithm. We then address the origin of spontaneous binaries, before adding ‘by hand’ a second population, with the aim to create a global population that recovers important characteristics of binaries (separations, binary fractions) observed in the galactic field.
3.1 Detecting the binaries
Stars can be found to be part of several binaries at once, which happens more often for massive stars as they clear the density threshold more easily. When this happens, the algorithm selects from such connected systems only the pairs exhibiting the lowest (most negative) binding energy.
Star–star interactions which take place during the HL expansion phase speed up the internal evolution of small substructures (or clumps). The global expansion, on the other hand, brings about correlations in phase-space coordinates due to the predominantly radial motion of the stars. As a result, stars may pair up in the time-dependent potential if their mutual binding energy becomes negative (see Appendix A and Kouwenhoven et al. 2010; Moeckel & Clarke 2011 who discuss this in the context of dissolving clusters). We refer to this population as spontaneous binaries. There is a trade-off between the creation of spontaneous binaries during expansion, and their destruction/heating when they sit near or inside a clump. Wide binaries may survive for several internal periods if they form in low-density regions where the tidal field is weaker. Inspection of several simulations suggests that roughly 10 per cent of stars end up in wide binaries. Their properties as a subpopulation will be addressed statistically through numerical experiments.
3.2 Spontaneous binary fraction versus primary mass
Several studies have found a strong correlation between the binary fraction fm and the primary mass m of a binary system (for compilations, see e.g. fig. 17 of Bate 2012 and fig. 12 of Raghavan et al. 2010).2 Since heavy stars tend to drive the formation of clumps in HL models, by attracting stars to themselves, it is natural to expect the HL procedure to give rise to a correlation of that nature.
The spontaneous binary fractions found in HL models for logarithmic primary-mass bins are plotted as light grey lines on Fig. 2. The shaded area shows the 1σ dispersion for these distributions. The fraction increases rapidly with primary mass, and is in close agreement with the data for primaries of mass higher than 2 M⊙, when fm exceeds 50 per cent. However, the HL models show a significant deficit of low-mass primary binaries in comparison to observational data.
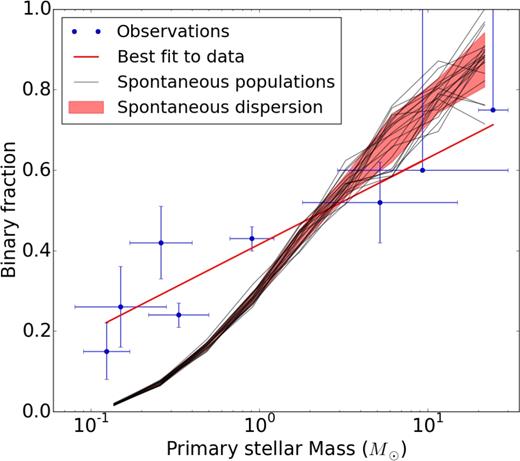
Observational data of binary fractions (dots with uncertainties) as a function of primary mass. The data are taken in increasing primary mass from the following: Close et al. (2003); Basri & Reiners (2006); Fischer & Marcy (1992); Ward-Duong et al. (2015); Raghavan et al. (2010); Patience et al. (2002); Preibisch et al. (1999); Mason et al. (1998). The red line is a best-fitting linear relation. The thin curves and 1σ dispersion shaded area are the results for a population of spontaneous binaries obtained from HL models. The bins have a logarithmic width of about log(2).
The high binary fraction for heavy primaries can be explained, at least in part, by considering the mass segregation occurring in the clumps during their formation. Dorval et al. (2016) have shown that massive stars tend to sink to the centre of clumps. These high-mass stars are more likely to capture another star to form a binary through a three-body interaction as they sit in denser environments (Spitzer 1987). A heavy star also creates a deeper potential well wherein to trap a fly-by star at the onset of HL fragmentation. There is indirect evidence for the three-body binary formation process to draw from mass-segregated clumps, because these binaries have a mean mass ratio q = m2/m1 that is significantly larger than expected from random pairing. The mean value of q for binaries with a primary star in the range 15–30 M⊙ is indeed 0.21 ± 0.11, whereas random pairing yields a mass ratio of 0.02 ± 0.02 for that mass range. Due to mass segregation inside clumps, massive stars are more likely to pair up with moderately heavy companions rather than light ones.
3.3 Spontaneous semimajor axis distribution
The distributions of semimajor axes a and orbital periods are the main parameters used to characterize binary populations. Up to this point, the distances were given in computational N-body units. To convert the models to physical scales, we matched their stellar number density within the half-mass radius to that of observed clusters. King et al. (2012) compiled data for several young clusters and gave their stellar densities within half-mass radii, with high values reaching 400 stars per pc3, typical of the ONC, and low densities of ∼6 stars per pc3, more akin to the Taurus region. These are the two reference values used to build up our data set of numerical models. The time conversion gives a total duration of the simulations of 2.3 Myr for the high-density clusters and 18 Myr for the low-density ones.
In practice, the spontaneous binaries develop a bell-shaped distribution of separation centred on ∼2000 au for a high-density HL model, and ∼7000 au for a low-density one (see the broken curve on Fig. 4). This is much wider than the averaged value of ∼50 au for the Galactic field population (Duquennoy & Mayor 1991; Raghavan et al. 2010), where separations of ∼1 au or lower are not uncommon. It is none the less shorter than the 104–105 au peak found in N-body simulations of dissolving clusters (Kouwenhoven et al. 2010; Moeckel & Clarke 2011; see also the discussion in Appendix A).
Hydrodynamical calculations by Bate (2012) show that orbital energy dissipated in the early stages of formation may cause binaries with an ∼10 au separation to shrink to a ∼ 0.5 au in the course of t ∼ 1 Myr. Analytical arguments by Stahler (2010) and Korntreff, Kaczmarek & Pfalzner (2012) would have external drag forces from residual gas drive a tight binary to merge completely. Kroupa & Burkert (2001) have shown that stellar collisions alone cannot bring a narrow distribution of semimajor axes to the full width of observed values. Other authors, such as Parker & Meyer (2014) investigated the evolution of a binary population identical to the field but embedded in clumpy, fractal clusters (Goodwin & Whitworth 2004) to test the robustness of the field population, while Vesperini & Chernoff (1996) introduced binaries with a binding energy inverse power-law, favouring weakly bound pairs.
A full spectrum of separations is desirable for comparison with data and theoretical models but is not a natural outcome of the HL fragmentation.
A trick was used to add binaries to the fragmented HL initial conditions. We follow Parker & Meyer (2014) to ease comparison with their setup, by supplementing the population of spontaneous binaries with one that matches the field galactic populations at small a. In doing so, we should also constrain the statistical weights with mass so as to redress the deficit of small-mass primaries (Fig. 2).
3.4 Complete separation distribution: procedure
The addition of new binaries to the HL distribution is not straightforward, as the phase space coordinates of stars in an HL fragmented system are the consequence of the numerical evolution during the expansion (no known functional distribution function). A practical and coherent method builds the extra binary population before the HL expansion phase.
3.4.1 Binary parameter distributions
When introducing binaries in the system, primary masses are picked to account for the observed decrease in binary fraction with decreasing primary mass (see Fig. 2). Secondary masses are then randomly chosen in the remainder of the population. The resulting mass-ratio distribution is generally flat, slightly peaking at high mass ratios, mostly from low-mass stars paired with other low-mass stars. This has no bearing on our study, as the mass-ratio distribution has a negligible impact on the processing of a binary population (see Parker & Reggiani 2013).
Eccentricities are drawn from a flat distribution, which is consistent with observed populations; see section 5.1.4 of Duchêne & Kraus (2013). This distribution is not expected to be substantially affected over a few Myr of dynamical evolution (Kroupa 1995b) and evolves to a thermalized profile on a longer time-scale (e.g. Kroupa 2008).
Finally, binary separations and periods are drawn from Raghavan et al. (2010). To account for the excess of (spontaneous) binaries at large separations, a reduced statistical weight is used to lower their impact on the final distribution. For simplicity and to limit the number of parameters in this work, we chose to apply the same distribution of separations to all binaries. In future work, one would like to factor in the fact that observations show that the distribution changes with the primary mass (see Duchêne & Kraus 2013; Parker & Meyer 2014).
A binary is generated by a Monte Carlo method, then replaced by a point mass equal to the sum of its components, and the HL expansion carried out with a subset of such fused binaries. Once the system has fragmented, the fused binaries are split and their two components recovered along with their set internal parameters. The barycenter of the new binary follows the orbit of the original point mass.
There are drawbacks to the implementation of the method; the main ones are as follows: (1) The HL fragmentation develops with fewer stars than in the final system, after the splitting. Therefore, fewer spontaneous pairs may form than might have otherwise. (2) Some spontaneous pairs form at the end of the HL phase with one fused binary as a component. Many of these spontaneous binaries (we found ≈50 per cent) are no longer classified as bound pairs once the massive point mass is split into the binary. Thus, the value of a and the amplitude of the peak of the statistics for spontaneous binaries after the split is hard to fix or predict. These two caveats make the insertion of binaries a complex statistical process, which leaves some freedom in the final distribution at the characteristic scale of the spontaneous population (few 103 au). Nevertheless, the final distribution comes close to the one sought, with differences (i.e. a relative excess of wide binaries) we may attribute to the formation history of the system.
3.4.2 Results for N = 20 k models
The procedure outlined in the previous subsection was tested on a set of 20 identical low-density models (≈6 stars per pc3; see Section 3.3) of 20 k stars. The resulting binary fraction is plotted on Fig. 3 as a function of the primary mass. The deficit of low-mass primaries was bridged, while the fraction for high-mass primaries was slightly reduced (from 0.85 down to 0.75 for a primary mass of ≈20 M⊙; note the increased scatter). This is the effect mentioned in Section 3.4 of splitting fused spontaneous binary components with a heavy primary. (Several of these heavy spontaneous binaries with large separation no longer satisfy equation 3). A few massive binaries were introduced to compensate for this effect, which remains relatively small. The values derived for this range of primary masses remain in complete agreement with observational data. Extensive numerical exploration of binary tidal heating has been performed by one of us (Roos 2013). Binaries were put on highly eccentric orbits in cuspy Dehnen (1993) potentials, thus experiencing large variations in the tidal force. It was found that the binary binding energy varied by ≈50 per cent or more only when the semimajor axis a > 100 au. No binary–single stars or binary–binary interactions were included. The conclusion from this study is that binaries with axis a shorter than 100 au should rarely unbind due to tidal heating. Further tests with nbody6, and the results of the next section, largely confirm this. With that in mind, and in view of the computational costs, we truncated the binary population at a = 1 au. This choice allows us to recover the range found in the SPH calculations of Bate (2012), so a closer parallel can be made with his setup.
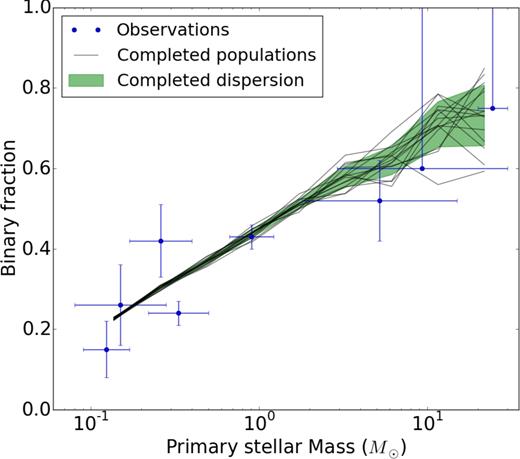
Binary fraction as a function of primary mass. The results displayed are the average of 20 realizations for 20 k particles models; the shade indicates 1σ deviations. Short semimajor axis binaries were injected to complete the spontaneous population (compare with Fig. 2).The bins have a logarithmic width of about log(2).
We show on Fig. 4 in short-dashed red the full spontaneous binary population (with a peak value at a ≈ 7000 au), prior to the procedure to split fused binaries. The distribution of separations for the fused binaries is shown as the long-dashed curve, with a dip around a ≈ 10 000 au. Finally, the splitting procedure is carried out, and the result shown as the solid curve. The grey shade is the expectation value for the parametrized Gaussian distribution of Raghavan et al. (2010). Discrepancies with this distribution are only significant for binaries with a > 4000 au. The peak of the spontaneous population, still visible after the splitting procedure, introduces an excess of binaries for (roughly) 4000 < a < 20 000 au. Note how the excess of binaries in that range has been halved by the splitting procedure, dropping from a maximum of ≈370 to ≈170. For separations larger than a > 106 au, the binaries are not identified by the density threshold algorithm and are dropped from consideration. Since none of them are likely to survive for a long period of time given the density of the system, this will have no bearing on our conclusions.
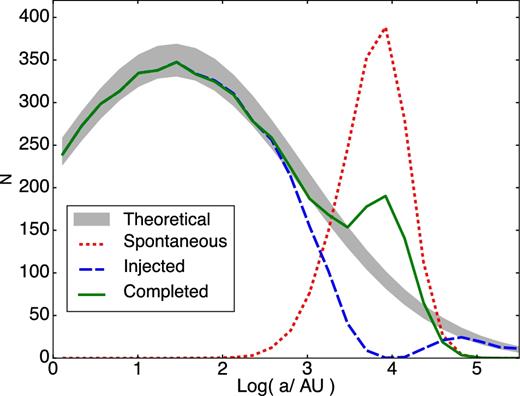
Distribution of binary separations for the completion of a population in a low-density model. Spontaneous binaries before splitting are shown in short-dashed red, the population injected in the system in long-dashed blue, and the resulting measured distribution in the completed system in green. The observational separation distribution from Raghavan et al. (2010) is shown as a grey area, taking into account Poisson dispersion.
4 EVOLUTION OF BINARY POPULATIONS
A system bound gravitationally will resist external tidal forces if it sits within its Roche radius (Binney & Tremaine 2008, section 8; Renaud et al. 2011). For binary stars, the condition for boundedness is given by equation (3) with D = 3 and setting the mean density ρbin over the full Jacobi volume. Thus, the question of how much the mean background density rises and compares to the mean density of the binary has important consequences for the further evolution of the binary. The situation is made more complicated if the host's potential changes rapidly, on the dynamical time-scale tcr of equation (1).
Let us first recall the results of the collapse of a homogeneous sphere of N identical stars. In this case, the sphere collapses by a factor C before it rebounds and evolves towards equilibrium (Aarseth et al. 1988; Boily et al. 2002). The ratio of minimum radius achieved during collapse, to the initial system radius, is a 1/3 power-law of N, so C ∝ N−1/3. If the total mass is conserved during the collapse, the mean background density scales as ρ ∝ C−3 and so reaches a peak value max (ρ) ∝ N. Based on this analysis, one would expect a strong relation between the system total mass |$M = N \overline{m}$| and the rate of destruction of binary stars, especially those of large semimajor axis a, if and when a cluster undergoes a phase of collapse.
Various simulations were performed: their parameters are summarized in Table 1. Runs with N ranging from 1500 to 80 000 were sampled in such a way that ensemble-averaging gave roughly the same Poissonian standard deviations in each case.
4.1 Results
We show on Fig. 5 the evolution of the binary fraction in HL fragmented systems as a function of time. The time when the systems rebound from the global infall, t ≈ 10 units, is marked with a vertical dotted line on each frame. The binary fraction decays in each case during the course of evolution, regardless of their membership N or initial density. All systems display two different regimes of binary destruction, before and after the bounce from global collapse. Before the bounce, binaries are destroyed at a higher average rate, more so for the more massive systems (large N). After the bounce (t > 10), the slopes all flatten out and binaries are continuously destroyed, but at a lower rate. For example, the high-density N = 80 k simulation removes 2.5 per cent of its binaries per time unit before the collapse; this rate goes down to 0.25 per cent afterwards. Similarly, the low-density N = 80 k run has 1.7 per cent before the collapse and 0.16 per cent thereafter.
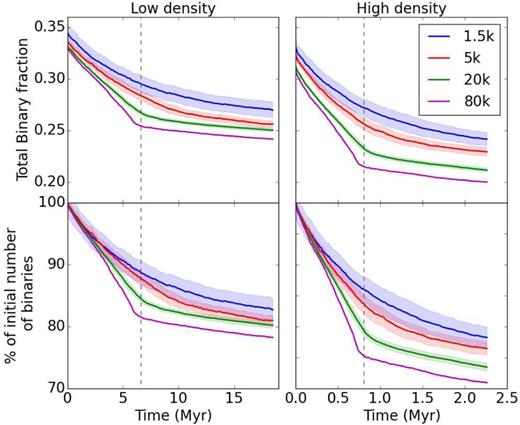
Top panels: total binary fraction over time for different cluster memberships. Lower panels: total number of binary over time compared to initial number in each system, in percentage. The vertical dashed line indicates the time of deepest collapse.
We interpret these findings as follows. In the first stages of evolution, the rate of binary destruction is driven by the two-body relaxation in the small clumps of the HL configuration. To see this, Fig. 5, bottom row, graphs the relative fraction of surviving binaries for each model. The linear slope is virtually identical up to t ≈ 5 units. The internal dynamics of clumps is independent of the larger system in which they are embedded. As collapse proceeds, larger N systems develop a deeper global potential well: this is easier to see for t → 10 as the curves fan out. The range, of about 10 per cent, accrued at t = 10 between runs of different N, is almost unchanged at the end of the simulations, at t = 30 units. The rate of binary destruction post-bounce is practically the same for all N, though note that it remains higher for the high-density calculations (the final count of binaries drops from ≃80 per cent at low density to ≃70 per cent at high density).
There is a clear tendency for the pre- and post-collapse transition to be sharper as N increases. We interpret this in the light of equations (1) and (2). We note that the N = 1.5 k models are dominated by two-body interactions, when the mass-segregation time-scale drops to ∼1 time units, and not by the overall collapse motion that drives density upwards, destroying binaries. At the other end of the spectrum, the N = 80 k models have a global mass-segregation time-scale >30 time units. These models, like all the others, are initially dominated by two-body interactions in their clumpy substructures. However, the later evolution sees the overall collapse motion take over. It is the imprint of that global infall which allows the density to peak at higher values (cf. Table 1) and laminate binaries more efficiently around that time. Since the rebound is of short duration, the strong tidal field drops quickly as we shift in the post-bounce phase.
To determine whether the evolution affects binaries differently according to their mass, we show on Figs 6 and 7 the binary fraction in relation to the primary mass. Results are shown at four different times, and for all runs of N. The top row of each figure is the binary fraction fm and the bottom row the percentage of binaries with respect to the initial distribution.
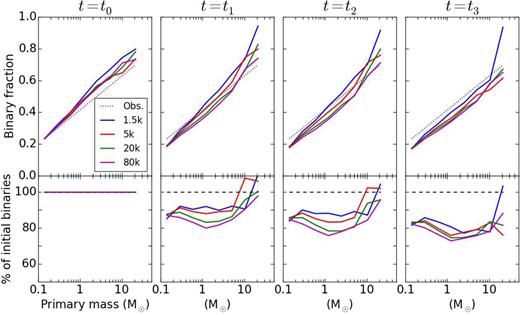
Top panels: the binary fraction in logarithmic bins for the primary masses. The dotted line is the linear fit to observations shown on Fig. 2. Bottom panels: the number of binaries in each primary-mass bin is shown as a percentage of the initial number (t = 0). The horizontal dashed line is 100 per cent. The data are from low-density models.
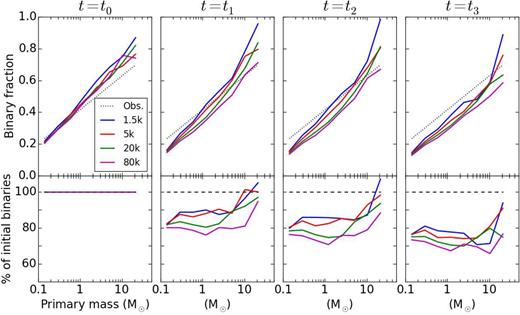
This representation highlights which binaries are the most processed in the system. The panels for t = t1 and t2 are for times immediately before and after the bounce (cf. Fig. 1). The dynamical evolution within the clumps and during the collapse impacts preferentially light-primary binaries; binaries with a more massive primary (say, >5 M⊙) survive better. We stress that the trends are indicative and at the ∼20 per cent level in all cases. Bearing this caveat in mind, inspection of the different cases does suggest that the results for low-N runs are more the result of the internal dynamics of clumps, where star–star interactions would favour the ejection/destruction of low-mass binaries. Note how the low-N models in fact form additional high-mass binaries during infall, since the binary fraction exceeds 100 per cent at t = t1 and t2. This is not so for the N = 20 k and 80 k models, which we interpret as being due to the stronger tidal fields in these models which stops new binaries from forming. It is interesting that despite the deeper infall achieved by these large-N models, the trend of increased survival with primary mass is not eradicated: this would have been the case had the external (global) tidal field clearly dominated the binary destruction. Instead, we find that the strong fields do not erase memory of the early evolution phase of the clumpy distribution.
The results for the later time t = t3 displayed coincides with the end-time of the simulations. At that point, all models have reached equilibrium and the binaries have been processed dynamically in such a way that the binary fraction decreased monotonically for all primary masses. It is worth noting that the low-density models (Fig. 6) have evolved for a physical time t ≈ 18 Myr, while the high-density ones (Fig. 7) up to t ≈ 3 Myr only. This may explain the greater scatter among the different runs for these models (they have more intense tidal fields but have less time to act on the binaries). We also note that the peak at high primary mass, clearly visible at t = t2, is still apparent at t = t3, except for the case N = 80 k, which is the model with the highest density and the strongest tidal field. We interpret this as indicating that the wide binaries have had time to be split, while this process is yet incomplete in the other models. This view is backed up from inspection of the low-density runs at t = t3 on Fig. 6, where all the peaks seen at t = t2 have been flattened, save the runs with N = 1.5 k. We believe that the more stochastic low-N runs may have produced more wide-binary escapers due to their shallower potential well. These would therefore not be processed collisionally in the final cluster and survive in isolation.
4.2 Semimajor axis distributions
The evolution of the distribution of semimajor axes a in a binary population hinges on its dynamical environment. Several analytical and numerical studies (Heggie 1975; Kroupa 1995a,c; Vesperini & Chernoff 1996; Heggie, Trenti & Hut 2006; Parker et al. 2009, 2011) have shown that wide, weakly bound binaries are preferentially disrupted, thus sculpting the distribution towards tighter, more bound binaries. This evolution is shown on Fig. 8, which graphs the distribution histograms as a function of semimajor axis a. The distributions are plotted for four various times, membership N and both the values of initial density. The averaged distribution computed from the models with N = 1.5 k is shown as a dotted line and serves as reference. To ease the comparison between models with different N, all histograms were normalized to the reference initial profile at t = 0 (e.g. the area under the curve is the same for all the models).
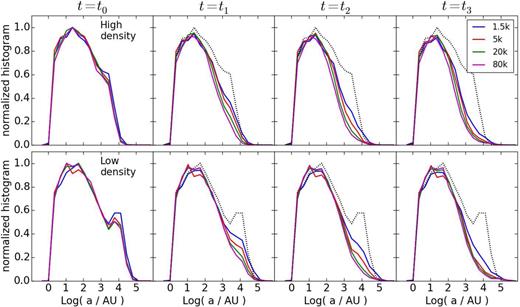
Histograms of semimajor axes for the binary population at four different times and for four different cluster membership. The dotted black line shows the distribution of N = 1.5 k models at t = 0 as a reference for the initial distributions. High-density models are in the top row, while low-density models are in the bottom row. The reference times are taken from Fig. 1.
We first distinguish between high- and low-density models. The overall behaviour of the models is the same, with a rapid dissolution of large semimajor axis binaries, a ∼ 103 au or more. This takes place prior to the bounce, when t < t1, but is a continued trend from the start of the computations to the end. As anticipated, high-density models (top row on Fig. 8) process the binary population more efficiently, reaching deeper in the short-axis range, down to a ∼ 20 au, compared to a ∼ 100 au for the low-density models. This can be gauged qualitatively by the gap that opens up between the dotted line and the histograms.
The system mass (or membership N) has little influence on the evolution of the histograms; however, as the collapse factor C increases with N, the larger N models process significantly wider a ∼ 103 au binaries; this is true regardless of the initial density (high or low). Note that, here too, the N = 1.5 k models stand out, in the sense that their wide binary population is less efficiently processed.
Having identified the mean initial density as the main driver for binary population evolution, we wish to quantify the rate of survival of binaries. We define the erosion factor as the ratio, in each semimajor axis bin, of the current number of binaries to the initial population. This allows us to asses easily the processing power of the system at all separations. The idea was borrowed from Marks et al. (2011) and Marks & Kroupa (2012) who introduced an analytic operator acting on a binary population to mimic its evolution in a host star cluster. The erosion factor is bound to the interval [0, 1], and equals 1 when all binaries of a given semimajor axis a are retained (zero when they are all destroyed).
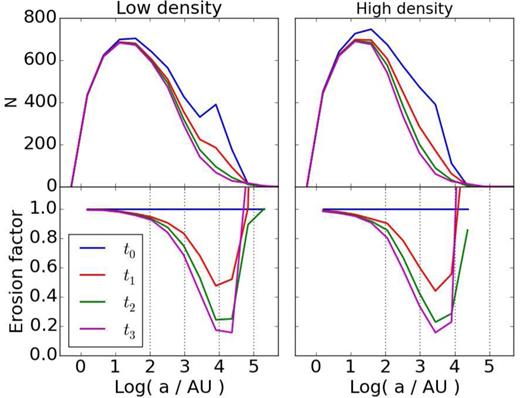
Top panels: histogram of semimajor axis at four different times for the N = 20 k models. Bottom panels show the erosion factor, the ratio between the sizes of the initial binary population and the one at a given time, in each primary-mass bin.
This fact alone implies that the evolution of fragmented mass profiles through a relaxation phase is driven mostly by stellar encounters. The same conclusion applies for the evolution of the fractal models of Parker et al. (2011).
4.3 Tidal shocks
We noted how larger N simulations tend to iron out a larger fraction of binaries (see Fig. 8). The weak trend with increasing N implies that both star–star interactions (including multiple stars) and global tidal forces both boost the heating up and unbinding of binaries. The shift seen on Fig. 7 is small but systematic: from 15 per cent for N = 1.5 k to 25 per cent for N = 80 k of all binary stars are destroyed at the bounce (t ≈ 10 units). To get a better appreciation for the trend (or lack thereof) with N, let us compute the energy transferred to a binary star by the tidal field. At the end of the collapse, the stars move on mostly radial orbits at high velocities. Since they cross a dense region in a short time, we make use of the tidal shock approximation developed by Spitzer (1958, see also Boily et al. 2004; Binney & Tremaine 2008, section 8).
An increase in membership N implies more significant heating of the binary star. If we set ΔEs/Es = 1, then an increase of N → 10 × N should have the same relative effect on a binary of semimajor axis a → a/101/3 ≈ a/2.15. By implication, the peak of the distributions seen on Fig. 8 should shift from a ≈ 40 au to a ≈ 40/3.76 = 10.64 au, as we work up from N = 1.5 k to 80 k calculations. This shift is not seen on the figure. What we see, on the other hand, is that large-N systems tend to deplete more efficiently the wide binaries, so that at the same stage of evolution, the richer systems have a binary distribution that falls off more quickly at large separations. We attribute the weak dependence on N to the approximation of a static background mass distribution.3 In reality, the whole structure moves on the same short dynamical time-scale of equation (1) and hence the effective surface density Σ is much reduced if the system as a whole begins to re-expand. We suspected that the choice of fixed initial density may be the reason for the undetectable shift of the peak on Fig. 8. Our choice of initial conditions implies that the system size Ro ∝ M1/3; had we chose instead to use an empirical relation such as Ro ∝ M1/5 for stellar clusters (Larsen 2004), we would have found a scaling of ΔEs/Es ∝ a3N7/5. The same rise in N as before would have produced a shift from ≈40 to 6.5 au in the peak of the distribution, and this is still too large to go undetected.
5 EXTREME TIGHT AND WIDE BINARIES
As mentioned in Section 3.2, Bate (2012) has found several examples of binaries reducing their separation over time through stellar encounters, from ∼10 au down to ≲0.5 au. Tighter systems were hindered by numerical resolution issues. The regularized treatment of close encounters (which allows us to integrate up to machine precision) of the code nbody6 means that the same collisional process will be at play in the calculations that we have performed. Since no binaries with semimajor axis a shorter than 1 au were inserted in the initial conditions, we focus first on the statistics of binaries that evolved to a < 1 au. In the second part of this section, we will explore the formation and the evolution of very wide (a > 104 au) binaries, many of which end up loosely bound to the stellar cluster as a whole.
5.1 Tight binaries
Several binaries with a < 1 au were detected at the end of the low-density simulations. By comparison, almost none developed in the high-density runs. Their properties are summarized in the left-hand panel of Fig. 10. The data are taken from N = 20 k runs but similar statistics were obtained for the other setups. The top row shows the binary's mass as a function of semimajor axis, while the bottom row on that figure graphs the distance of their barycentre to the centre of the cluster. Open circles denote binaries that already existed at t = 0, and became tighter over time, while filled circles are new binaries that formed in the course of the simulation. Inspection of these new, tight binaries shows that both of their components were part of binaries originally inserted in the system. Thus all new tight binaries shown on Fig. 10 are the results of strong binary–binary interactions leading to splitting or exchange. (None of the original binaries that survived to the end of the simulation had a < 1/2 au.)
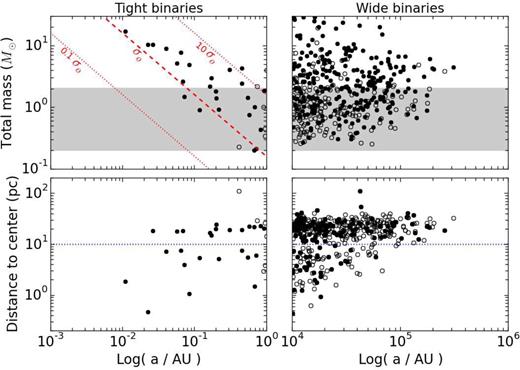
Left-hand panels: total binary mass (top) and distance to centre of the cluster (bottom) versus the semimajor axis for binaries tighter than 1 au at the end of the simulation. Right-hand panels: same layout for binaries wider than 104 au. The greyed area in the top panels shows the 90 per cent probability range of a binary total mass from random pairing given the present IMF. The red dashed line in the top-left panel shows the relation for constant |$v^2_\infty \sigma _{\rm {coll}} \equiv \sigma _0$|; it is bounded by two dotted curves that have 10σ0 (above) and 0.1σ0 (below). The horizontal dotted line in the bottom panel indicates the boundary between the ejecta (distance to centre >10 pc) and the central system. Open circles are binaries which already existed at t = 0. The data are from 10 simulations of N = 20 k stars with initial mean density of 6 per pc3.
These statistics are in good agreement with the identification in the 10 N = 20 k runs of a total of 24 binaries with a < 0.6 au, for an average of 2.4/5000 ≈ 0.05 per cent of all binary stars (fb ≈ 1/3 at t = 0). The very tight binaries each have a combined mass exceeding 2 M⊙ with a low mass ratio q, ranging from 0.01 up to 0.2. It is important to mention that main-sequence binary stars with a separation a ≲ 0.02 au would be contact binaries when both members are solar-type or more massive stars. Clearly the evolution of such objects (and in fact their formation process) calls for hydrodynamical effects that were not included in our study. It is therefore remarkable that contact binaries should form strictly through gravitational scattering on such short time-scales.
These results apply to runs with initial mean number density of 6 per pc3. The same analysis carried out for the higher density models give a different outcome. For these models, the initial density is larger by a factor ∼60 and the velocity dispersion by a factor ∼2, for all stars. From equation (11), we find a collisional rate ∼30 times higher than previously. If direct collisions were the main channel for the formation of tight binaries, the number of events should increase in the same proportions. However, inspection of the simulations yielded only four tight binaries, each with a semimajor axis a ≳ 0.1 au. Thus the rate of formation of tight binaries drops to 0.4 per 5000 binaries, or 0.008 per cent, a six-fold decrease. We argue that two factors hinder the formation of these objects in high-density environment. First, Geller & Leigh (2015) pointed out that exchange encounters between single stars and binaries are not instantaneous (see also Hut & Bahcall 1983). The process can be perturbed by other stars, so modifying the outcome of the collisions. This is also true for binary–binary collisions: casual inspection of the time series of (tight) binary stars showed that their mean separation keeps evolving throughout the evolution of the model, which suggests that the binaries are part of a small-N hierarchical system. It is reasonable to expect that more hierarchical systems will develop in a higher density environment (involving more stars) and so the exchange process may never have time to take place.
As the encounter velocity increases, it becomes harder and harder to perform a successful exchange. The two initial mean densities that we picked may therefore cover the transition from low- to high-velocity regimes, and reduce the number of tight binaries created to just a handful.
Going back to Fig. 10, we plot the relation |$v^2_\infty\, \sigma _{\rm {coll}} \equiv \sigma _0$| of equation (11) as straight red lines on the top-left panel. Two dashed curves bracket a curve in full type, each with a value of σcoll differing by a multiplicative factor of 10 (increasing from the lower curve, up). The large separations between the curves and the clustering of data points along the dashed curve indicate how a single value of σ0 effectively cuts through the diagram in two well-delimited regions. Thus, the trend of binary mass increasing as M ∝ 1/a for the most tight binaries is consistent with a constant product of |$\sigma _0 = v^2_\infty \sigma _{\rm {coll}}$| at the time of formation.
5.2 Wide binaries
The formation of wide ‘spontaneous’ binaries during the HL fragmentation process naturally leads one to expect that more wide binaries will form in the post-collapse evolution of the system, when expanding streams of stars emerge from the compact bounce to form a tenuous halo. We already noted how the erosion factor on Fig. 9 shoots up for large semimajor axes, exceeding unity at the later stages of evolution in both high- and low-density calculations. This implies either that a subset of wide binaries got softer over time, or that new binaries of separation >104 au formed during the virialization phase. If we compare the numbers for axes a > 5.104 au ≃ 0.25 pc and for all 10 R20l models, then there were 120 binaries more in this range at the end of the simulations, right after the bounce, at time t = t2. Of those 120, some 40 new binaries formed in the expanding volume, while 80 are soft binaries that became softer as a result of collisional evolution, the well-known Heggie–Hills law (Heggie 1975; Hills 1975). While these numbers of very wide binaries are very low indeed, a ratio of 120 per 10 × 20 k stars (0.06 per cent), they are still significant because they would be associated with neighbouring star-forming regions, and may yet register as correlations in phase-space coordinates.
The right-hand panels of Fig. 10 graph the basic properties of this subset of extreme wide binaries. We selected all binaries with separations exceeding 104 au, the largest semimajor axes that were found in the initial conditions. Hence, all data points shown here are the result of some evolutionary mechanism. The horizontal dotted line on Fig. 10 marks a distance of 10 pc as the separation between the central equilibrium cluster and the volume where weakly bound ejecta orbit. Close to ≈60 per cent of wide binaries are escaping the main clusters or are very weakly bound to it. Therefore, most of them would be lost to the tidal field in a real cluster environment.
We stress that the semimajor axis a of an individual binary star is not a monotonous function of time. Fig. 11 graphs the evolution of six perturbed binaries, three tight and three wide binaries. Tight binaries mostly suffer strong interactions early on, before the collapse, inside a clump. This would take place most likely at the centre of the clump, where the density is higher. What happens next is hard to predict, as the pair can then be ejected from the system by this interaction (e.g. the first binary, top panel); or sink at the centre of the cluster and remain there (second binary, middle frame); or follow the bulk of the ejecta, falling once through the core and then leaving the system without further strong interactions (third binary, bottom row). Most wide binaries experience several strong interactions. Many of these take place around the time of the collapse, as they orbit through the dense core of the cluster. These repeated strong interactions will lead to heating and result in binary splitting. That is also why no (spontaneous) wide binary was found in the dense clump cores of the initial HL configuration; instead, they form in the low-density interclump space. Practically all wide binaries had a first strong interaction during the densest phase of the infall (t1 < t < t2).
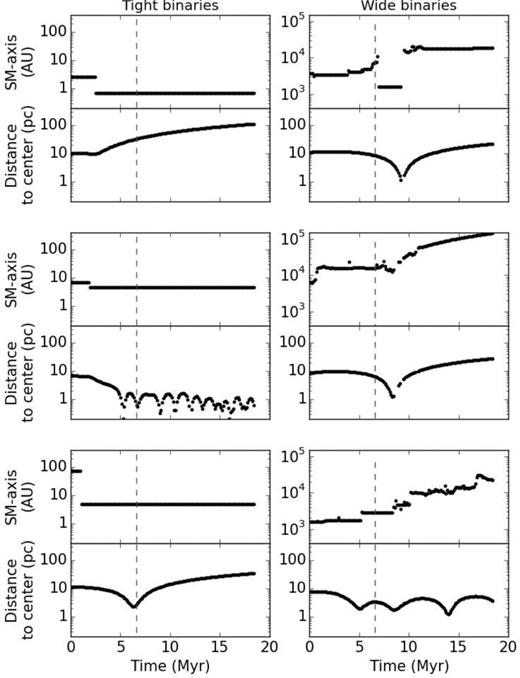
Evolution of individual binaries semimajor axis and distance to centre over time in the low-density 20 k particles models. The vertical dashed line marks the moment of deepest collapse t ≃ (t1 + t2)/2.
6 DISCUSSION AND CONCLUSIONS
6.1 Discussion
In this paper, we have shown with numerical experiments that the dissolution of binary stars proceeds at a much higher rate in initially clumpy configurations. The two-body relaxation time of clumps of typical membership Nc = 50 is about six times shorter than the global free-fall time of the subvirial star-forming volume. As a result, binaries are destroyed at a rate ≈10 times higher in clumps than in the later stages of evolution, when clumps have merged and a cluster of stars achieves dynamical equilibrium. We have also highlighted that the transition between clumpy and equilibrium states is much sharper when N ≳ 104: in such rich star-forming regions, the binary dissolution rate is well approximated with a linear relation in both the regimes. When N ≲ 104, we find a more gradual transition (Fig. 5). These findings compare well with those from e.g. Parker et al. (2011) based on fractal models, where the mass segregation by collisional evolution was found also to be very significant early-on.
The Gaia spacecraft's resolution reaches down to ≈26 μas at magnitude 15 (V band), with a five-year timeline and could detect such outflows out to a distance of ∼600 pc. Well-known star-forming regions such as the Orion Nebula or ρOphiucus are possible targets for such outflows. Brighter, young stars should allow a more precise determination of vp and possibly set new constraints on the formation history of rich open clusters.
We also noted that very tight binaries (a ≲ 0.01 au) formed as a result of binary–binary exchange collisions, when a new binary is formed as a result of the collision between two existing ones. The process begins in the clumps at the start, and carries on throughout the duration of the runs. Statistically we found that ≈0.05 per cent of all binaries end up with a semimajor axis a < 0.6 au. While these are low-number statistics, these events are important because they involve the most massive stars of the models, and their rapid formation (on a time-scale of ∼1 Myr) has direct implication for the formation by merger of very massive stars/contact binaries. The shortest axis system that we found had a ≃ 0.01 au and a total mass of 18 M⊙ (see Fig. 10). We observed similar trends in all the simulations, but noted that virtually no such tight binaries form in high-density runs. One important factor contributing to this situation is the increased collision velocities and (therefore) reduced effective interaction cross-sections in denser regions (the Safronov number Θ drops by a factor of ≈4). Another possibility comes from inspection of the time series of events from a subset of the runs, when we observe that the semimajor axis of very tight (a < 0.1 au) binaries continues to evolve right until the end of the simulations. This is a strong indication that these binaries are members of a compact hierarchical system. The very formation of the tight binaries may involve not just a pair of binaries, but a more complex situation involving a small number of stars (see e.g. Leigh & Geller 2013; Geller & Leigh 2015). We feel unable to disentangle the web of possible formation channels with confidence given the limited statistics of our sample of simulations. All calculations reported here where performed with a stellar IMF truncated at 30 M⊙ and without stellar evolution. This brings up issues for the later stages of evolution when the system age exceeds 10 Myr. It will be important in future investigations of that formation scenario to include a full stretch of the stellar IMF and perform the time evolution of the stars simultaneously with the dynamics.
6.2 Conclusions
The main findings of this paper are as follows.
The HL fragmented systems host a population of ‘spontaneous’ wide binaries that form from phase-space correlations. The distribution of separations a of that population is bell-shaped with a peak shifting from a ≃ 2000 to 8000 au as the system mean density is decreased. The multiplicity fraction of that population increases with the mass of the primary, and matches the trend observed in the field for primaries of mass >2 M⊙.
We presented a method allowing us to add to the spontaneous population a subpopulation of binaries in such a way that the final, total distribution of semimajor axis a and multiplicity fraction fb matches targeted trends. We have implemented a version recovering the field distribution of Raghavan et al. (2010).
The clumps process binaries up to 10 times faster than a relaxed spherical configuration, while the deeper potential of large-N runs reached during the merging and relaxation phase means that about twice as many binaries are dissolved in rich open clusters as there are in small N ≲ 5000 systems.
Very tight and massive binaries, with semimajor axis a down to 0.01 au, and total mass up to 15 M⊙, are formed first inside the clumps through exchange binary–binary interactions (cf. Fig. 10). Such tight binaries continue to form and evolve as the clumps merge and the star cluster relaxes to equilibrium. These binaries would form contact systems on a time-scale ∼1 Myr with an occurrence frequency of 0.2 binaries per 10 k stars per Myr.
Very wide binaries, of separation a up to 2.105 au, are formed in the low-density volumes of ejected stars during the relaxation phase of the runs. The mean velocities of ≈2–6 km s−1 of these stars mean that they are ideal targets for proper-motion studies by the Gaia programme.
Acknowledgments
We thank the anonymous referee for a constructive report which led to significant improvements of the paper. We also thank B. Goldman. M. Giersz, and E. Vesperini for discussions. We also gratefully acknowledge financial support from Institut National des Sciences de l'Univers through grant AO2011-684316.
Varying the number of neighbours in this range has no strong effect on the detection rate.
It should be noted that selection effects may at least in part explain such correlations; see e.g. De Rosa et al. (2014).
The original treatment by Spitzer fixed a thin (vertically mixed) disc crossed by a stellar cluster at high speed.
REFERENCES
APPENDIX A: PHASE-SPACE CORRELATIONS
The calculation presented above predicts that virtually all stars should end up in binaries of separation ≈l = R(τ)/N1/3 (t = τ being the time when |$\mathcal {H} = 0$|). This is not so in practice because the velocity field around t = τ is not the Hubble flow of equation (A1), but is approximately a Gaussian field due to the formation of clumps (cf. Dorval et al. 2016). Because of density enhancements, where the tidal field would be stronger, only a subfraction of stars eventually form wide binaries; we found about 10 per cent in a typical simulations.
This situation is different from the one addressed by Kouwenhoven et al. (2010) and Moeckel & Clarke (2011). These authors found very wide binaries to form in the tenuous haloes of dissolving supervirial star clusters. Kouwenhoven et al. (2010) found a formation rate of a few per cent for these wide binaries and a separation distribution peaking at about 105 au. We also found a peaked distribution but with a peak value of about 104 au or less (see Section 3.3). Inspection of HL fragmented configurations showed that the interclump distance is typically of the order of 105 au or more, which may explain why binary stars of such wide separations do not form (they are disrupted by the tidal field of clumps).
In integral form, this formalism would allow us to perform Monte Carlo draws from any functional form for the distribution function f. Binney & Tremaine (2008), section 7.5.8, give a numerical example for the case when |$f = \overline{\rho }(\boldsymbol {x},t) / \overline{m} \, \tilde{f}(\boldsymbol {v},t)$|, with |$\overline{\rho }(\boldsymbol {x},t) / \overline{m} =$| constant and the velocity d.f. |$\tilde{f}(\boldsymbol {v},t)$| is a Maxwellian.