-
PDF
- Split View
-
Views
-
Cite
Cite
Jacquelin Peck, Michael J Wishon, Harrison Wittels, Frederick Hasty, Stephanie Hendricks, Stephen J Lee, S Howard Wittels, COVID-19-Induced Changes in Photoplethysmography, Military Medicine, Volume 188, Issue 7-8, July/August 2023, Pages e2661–e2669, https://doi.org/10.1093/milmed/usad025
- Share Icon Share
ABSTRACT
Photoplethysmography (PPG) is the science behind many commonly used medical devices such as the pulse oximeter. PPG changes, herein as “PPG dropouts,” have been described in existing in vitro studies following artificially induced clot activation. Because COVID-19 causes increased arterial, venous, and microvascular clot formation, our hypothesis is that PPG dropouts identified in vitro can also be found in vivo in patients with COVID-19. The aim of this study is to evaluate PPG recordings and D-dimer levels for patients hospitalized with COVID-19 and compare them with the PPG tracings from non-COVID controls.
PPG recordings were obtained for 197 ICU patients with COVID-19 and 300 non-COVID controls. PPG tracings were obtained using a TigerTech CovidPlus monitor, which received U.S. FDA emergency use authorization in March 2020 for monitoring the biometrics of patients with COVID-19 and featured unfiltered red and infrared spectrum PPG monitoring. D-dimer lab results were also recorded whenever available.
The results demonstrated significant differences in the prevalence rate of PPG dropout among patients with COVID-19 vs. non-COVID controls. The median PPG dropout rate was 0.58 for COVID-19 patients (median 0.58, IQR 0.42-0.72, P < .05) as opposed to a median 0.0 for non-COVID patients (median 0.0, IQR 0.0-0.0, P < .05). Furthermore, at least one incidence of PPG dropout was detected in 100% of COVID-19 patients, as opposed to 2.3% of non-COVID controls (P < .05). PPG dropout also correlated closely with the normalized serum D-dimer levels taken on the same day. The change in the normalized D-dimer levels was plotted against the change in PPG dropout, and a line of best fit was created. Linear regression resulted in R2 = 0.743 (P < .05), indicating that changes in the PPG dropout rate correlate with hemorheological changes in COVID-19 patients.
PPG dropout, like D-dimer, may not be specific for COVID-19. However, the inflammatory nature of the disease and the prevalence of prolonged ICU created a large sample size and allowed the authors to observe PPG changes in vivo in a statistically meaningful way. Further confirmatory studies are needed to confirm the potential application of PPG dropout as a measure of inflammation in other disease processes.
INTRODUCTION
Hemorheology is the study of hematologic flow properties. Alterations in hemorheology or blood flow mechanics can be seen in many disease processes, especially relating to infection, inflammation, sepsis, atherosclerosis, and cardiovascular disease.1 COVID-19 (or SARS-CoV-2) is a global pandemic responsible for more than 6.4 million deaths globally since it was first reported in 2019.2 COVID-19 was once thought to be a primarily respiratory disease but is now better understood as a systemic inflammatory disease with well-established hemorheological changes. These changes include inflammation-induced hyperviscosity, hypercoagulability, hyperfibrinogenemia, and thrombosis that may be refractory to therapeutic anticoagulation.3,4 One recent evaluation of COVID-19-induced-hyperviscosity assessed capillary viscometry and reported significantly increased plasma viscosity ranging from 1.9 to 4.2 centipoise (reference range 1.4-1.8) as well as increased fibrinogen concentrations ranging from 459 to 1188 mg/dL (median value 708 mg/dL; normal reference range 200-393 mg/dL).3 Hypercoagulability and thrombosis increase COVID-19 morbidity and have led to complications including deep vein thrombosis, pulmonary embolism, and stroke.5
Real-time monitoring of hemorheological changes may help inform clinical decision-making. However, modern assessments of hemorheology have many limitations. Advanced hemorheological testing such as lumi aggregometry, light transmission, platelet aggregation, and flow cytometry is primarily reserved for advanced specialty centers and research labs.6 Serum D-dimer is a well-established marker for COVID-19 disease severity and prognosis.7,8 However, serum D-dimer and other commonly used laboratory (lab) measurements of platelet adhesion, clotting and degradation, blood viscosity, and rigidity index are invasive and nursing labor intensive and may take several hours to provide results. Furthermore, frequent lab draws contribute to iatrogenic anemia and increase costs. Finally, existing point-of-care testing options such as the thromboelastogram (TEG) are expensive and require a high level of training and expertise to perform and interpret results. Moreover, the thromboelastogram is time-consuming to perform and is a static measure of hemorheology at the time of blood draw—meaning that the lab results may be obsolete by the time they become available or that repeated measures may be necessary for rapidly evolving clinical scenarios.
In a 2017 Scientific Reports publication, Njoum et al. evaluated the accuracy of photoplethysmography (PPG) as a noninvasive, diagnostic measure of hemorheology in an in vitro model mimicking human arterial circulation.6 The study used PPG, an intra-arterial pressure transducer, and an ultrasonic Doppler flow meter to track changes in flow mechanics following alterations in the shear rate and clot formation.6 PPGAC and PPGDC both achieved statistically significant changes during periods of increased shear rate and immediately following thromboplastin activation. The authors also described a change in the PPGAC morphology and amplitude following clot formation. The authors concluded that PPG could be used as an effective, noninvasive method for mapping hemorheology and identifying patterns in blood flow mechanics, fibrin cross-linking, and clot disaggregation in vitro.6
The following is a proof-of-concept study applying PPG findings reported by Njoum et al. to living patients for the first time. In this observational study, we evaluate PPG tracings from 197 patients with COVID-19 and compare them to PPG tracings from 300 non-COVID controls. We also compare PPG trends within the COVID-19-positive population to trends in serum D-dimer, an established clinical marker for COVID-19 and a frequently used marker for non-COVID inflammation.7,8 COVID PPG changes may offer a dynamic, noninvasive, and affordable alternative to existing COVID-19 measures.
AIM
The aim of this study is to evaluate the PPG tracings from patients with COVID-19 and compare them to PPG tracings from patients without COVID-19 to determine whether the same PPG changes described in vitro by Njoum et al. can be seen in vivo. Patients with COVID-19 were chosen because this disease has well-documented inflammatory and hypercoagulable properties and because of the high prevalence of COVID-19, which would allow for a larger sample size over a shorter time period.
METHODS
Study Design and Participants
This is a partially prospective, observational, single-institution study. A total of 497 patients were enrolled (300 COVID-19-negative and 197 COVID-19-positive). The inclusion criteria for the prospectively collected COVID-19 cohort included COVID-19-positive status with confirmatory SARS-CoV-2 RT-PCR and ICU admission within the Mount Sinai Medical Center ICU (Miami Beach, FL) from April 8, 2020, to June 29, 2020. The exclusion criteria included age less than 18 years or ICU admission without a SARS-CoV-2 RT-PCR positive test result.
A control group of 300 COVID-19-negative patients was retrospectively selected from an institutional database consisting of 6000 patients with PPG recordings and was assumed to be COVID-19-negative because the data were collected before the first reported case of COVID-19 within the United States.9 This institutional database was created before the development of COVID-19 under an institutional review board titled, “Collect and Build a De-identified Single Limb Biometric Database” (FWA00000176 and protocol number 18-05-H-02). All patients provided written informed consent for the ongoing use of the database for purposes including improved disease diagnosis, prediction, and prevention using biomarkers. Control patient selection was completed using a random number generator in MATLAB. The exclusion criteria included an age less than 18 years. No exclusion criteria based on disease status or comorbidity were applied. The non-COVID cohort was not matched to the COVID-19-positive cohort.
All PPG recordings were assessed for PPG peaks, pulse rates, PPG dropouts, and dropout rates.
Data Collection
Included patients’ electronic medical records were reviewed by trained, IRB-approved physicians. COVID-19 status, demographic information, and lab results were recorded. PPG waveforms from 197 COVID-19-positive ICU patients were prospectively recorded by a Collaborative Institutional Training Initiative -trained research coordinator. Recordings were time-stamped and saved for analysis. D-dimer levels and SARS-CoV-2 RT-PCR results were prospectively obtained by study physicians from the patients’ electronic medical records. A total of 300 non-COVID patients were randomly selected from an institutional database for comparison, and corresponding PPG recordings and demographic information were retrospectively collected. D-dimer levels were captured by individual chart review. D-dimer values were not available for the non-COVID cohort due to the observational and retrospective nature of this study because D-dimer is not typically collected unless clinically indicated.
PPG recordings were obtained using a proprietary wearable technology that received U.S. FDA authorization for COVID-19 screening and biophysical monitoring (Tiger Tech Warfighter Monitor and COVID Plus Monitor, Tiger Tech Solutions, Inc., Miami, FL).10 The device is worn as a bicep armband and features several monitors including PPG, temperature, single limb ECG, and SpO2. It was used in the present study to provide unfiltered PPG recordings in the red and infrared spectra.
PPG Monitoring
The PPG data acquired by the Warfighter Monitor measure the reflectance of blood tissue on the outer left bicep, as is typical among wearable PPG monitors.11,12 Measuring PPG reflectance results in inverse PPG signals (i.e., temporary changes of tissue-reflected intensity), which are represented in this article. This method for obtaining PPG contrasts absorption-based PPG monitoring, which is commonly used in a clinical setting, on thinner tissues such as a finger or earlobe that allow LED light from a light-emitting diode to pass through and reach a photodetector on the opposite side. This should be noted when adapting these findings in the clinical space.
Acquired data were analyzed using a proprietary algorithm to detect PPG peaks, PPG valleys, and PPG dropouts.9 PPG peaks and valleys are defined as the point of maximal and minimal amplitude for each pulse wave from the continuous PPG recording. Utilizing PPG peaks, the pulse rate is calculated as the number of pulse peaks that occurred in 1 minute for a pulse rate of pulses per minute.
PPG Dropout
The PPG signal is composed of both pulsatile (AC) and non-pulsatile (DC) components. The AC component of the signal is the light reflected relative to volume changes generated by cardiac systole and diastole. The DC signal component is the light reflected by the skin, subcutaneous tissue, and arterial and venous blood volume. The DC component is often described as the bias of the non-pulse-related signal reflection and provides a signal average.
Changes in the PPG morphology have previously been described in hypercoagulable states in in vitro studies.6 To quantitatively define this, we termed the change in morphology “PPG dropout” and defined it as an excursion of the AC signal minimum more than 1 SD below the 60-second moving DC average signal.
More specifically, PPG dropouts are defined as a binary metric where during any pulse wave, the PPGAC signal voltage value decreased such that it is below the PPG dropout threshold. The PPG dropout threshold was defined as the 60-second moving average of the PPG, which characterizes the DC signal minus the 60-second moving SD of the PPG. For every pulse wave, there is either a dropout or not depending on whether the condition is met or not. Using PPG dropouts and pulse rate, a ratio of PPG dropouts per pulse wave or the dropout rate was derived as the ratio of the number of dropouts per minute divided by the pulse rate in beats per minute (the number of PPG dropouts per minute/pulse waves per minute). This gives a ratio of dropouts/pulse waves as a number between 0 and 1 (with 0 corresponding to a dropout in 0% of pulse waves and with 1 corresponding to a dropout in 100% of pulse waves).
Statistical Analysis
Statistical significance was set at P < .05. Two sample t-tests were performed. Analysis was performed using linear regression analysis and comparing the above statistical metrics between COVID and non-COVID patients utilizing scatter and line plots.
RESULTS
Demographics
In this section, PPG tracings from 497 patients are analyzed and presented (197 COVID-19-positive ICU patients and 300 non-COVID controls). Relevant medical information was recorded for the duration of their hospitalizations. Patient characteristics are presented in Table I. The randomly selected control group was representative of the COVID-19 dataset in terms of age, sex, ethnicity, and comorbidities.
COVID-19 status . | COVID-19 positive (n = 197) . | COVID-19 negative (n = 300) . |
---|---|---|
Patient characteristics | ||
Heart rate (beats per minute): median (IQR) | 75 (62-88) | 83 (74-92) |
Age (years): median (IQR) | 67 (54.75-79.25) | 59 (35.5-82.5) |
Male | 116 (59%) | 141 (47%) |
Hispanic | 81 (41%) | 81 (27%) |
Caucasian | 77 (39%) | 141 (47%) |
African American | 37 (19%) | 54 (18%) |
Obesity | 97 (49%) | 114 (38%) |
Diabetes | 41 (21%) | 36 (12%) |
Heart disease | 18 (9%) | 21 (7%) |
Cardiac arrhythmia | 28 (14%) | 36 (12%) |
Sepsis | 14 (7%) | 9 (3%) |
HIV/AIDS | 2 (1%) | 3 (1%) |
Cancer | 12 (6%) | 12 (4%) |
COPD | 14 (7%) | 15 (5%) |
Mental disorder | 24 (12%) | 54 (18%) |
Anti-inflammatory drugs | 122 (62%) | 111 (37%) |
Acetaminophen | 75 (38%) | 21 (7%) |
NSAIDs | 61 (31%) | 96 (32%) |
Decongestants | 33 (17%) | 12 (4%) |
Antihistamines | 16 (8%) | 9 (3%) |
Photoplethysmography findings | ||
Dropout rates: median (IQR) | 0.58 (0.42-0.72) | 0.00 (0.00-0.00) |
Number of dropouts (%) | 197/197 (100.0%) | 7/300 (2.3%) |
Maximum dropout rate | 1.00 | 0.01 |
Minimum dropout rate | 0.11 | 0.00 |
COVID-19 status . | COVID-19 positive (n = 197) . | COVID-19 negative (n = 300) . |
---|---|---|
Patient characteristics | ||
Heart rate (beats per minute): median (IQR) | 75 (62-88) | 83 (74-92) |
Age (years): median (IQR) | 67 (54.75-79.25) | 59 (35.5-82.5) |
Male | 116 (59%) | 141 (47%) |
Hispanic | 81 (41%) | 81 (27%) |
Caucasian | 77 (39%) | 141 (47%) |
African American | 37 (19%) | 54 (18%) |
Obesity | 97 (49%) | 114 (38%) |
Diabetes | 41 (21%) | 36 (12%) |
Heart disease | 18 (9%) | 21 (7%) |
Cardiac arrhythmia | 28 (14%) | 36 (12%) |
Sepsis | 14 (7%) | 9 (3%) |
HIV/AIDS | 2 (1%) | 3 (1%) |
Cancer | 12 (6%) | 12 (4%) |
COPD | 14 (7%) | 15 (5%) |
Mental disorder | 24 (12%) | 54 (18%) |
Anti-inflammatory drugs | 122 (62%) | 111 (37%) |
Acetaminophen | 75 (38%) | 21 (7%) |
NSAIDs | 61 (31%) | 96 (32%) |
Decongestants | 33 (17%) | 12 (4%) |
Antihistamines | 16 (8%) | 9 (3%) |
Photoplethysmography findings | ||
Dropout rates: median (IQR) | 0.58 (0.42-0.72) | 0.00 (0.00-0.00) |
Number of dropouts (%) | 197/197 (100.0%) | 7/300 (2.3%) |
Maximum dropout rate | 1.00 | 0.01 |
Minimum dropout rate | 0.11 | 0.00 |
COVID-19 status . | COVID-19 positive (n = 197) . | COVID-19 negative (n = 300) . |
---|---|---|
Patient characteristics | ||
Heart rate (beats per minute): median (IQR) | 75 (62-88) | 83 (74-92) |
Age (years): median (IQR) | 67 (54.75-79.25) | 59 (35.5-82.5) |
Male | 116 (59%) | 141 (47%) |
Hispanic | 81 (41%) | 81 (27%) |
Caucasian | 77 (39%) | 141 (47%) |
African American | 37 (19%) | 54 (18%) |
Obesity | 97 (49%) | 114 (38%) |
Diabetes | 41 (21%) | 36 (12%) |
Heart disease | 18 (9%) | 21 (7%) |
Cardiac arrhythmia | 28 (14%) | 36 (12%) |
Sepsis | 14 (7%) | 9 (3%) |
HIV/AIDS | 2 (1%) | 3 (1%) |
Cancer | 12 (6%) | 12 (4%) |
COPD | 14 (7%) | 15 (5%) |
Mental disorder | 24 (12%) | 54 (18%) |
Anti-inflammatory drugs | 122 (62%) | 111 (37%) |
Acetaminophen | 75 (38%) | 21 (7%) |
NSAIDs | 61 (31%) | 96 (32%) |
Decongestants | 33 (17%) | 12 (4%) |
Antihistamines | 16 (8%) | 9 (3%) |
Photoplethysmography findings | ||
Dropout rates: median (IQR) | 0.58 (0.42-0.72) | 0.00 (0.00-0.00) |
Number of dropouts (%) | 197/197 (100.0%) | 7/300 (2.3%) |
Maximum dropout rate | 1.00 | 0.01 |
Minimum dropout rate | 0.11 | 0.00 |
COVID-19 status . | COVID-19 positive (n = 197) . | COVID-19 negative (n = 300) . |
---|---|---|
Patient characteristics | ||
Heart rate (beats per minute): median (IQR) | 75 (62-88) | 83 (74-92) |
Age (years): median (IQR) | 67 (54.75-79.25) | 59 (35.5-82.5) |
Male | 116 (59%) | 141 (47%) |
Hispanic | 81 (41%) | 81 (27%) |
Caucasian | 77 (39%) | 141 (47%) |
African American | 37 (19%) | 54 (18%) |
Obesity | 97 (49%) | 114 (38%) |
Diabetes | 41 (21%) | 36 (12%) |
Heart disease | 18 (9%) | 21 (7%) |
Cardiac arrhythmia | 28 (14%) | 36 (12%) |
Sepsis | 14 (7%) | 9 (3%) |
HIV/AIDS | 2 (1%) | 3 (1%) |
Cancer | 12 (6%) | 12 (4%) |
COPD | 14 (7%) | 15 (5%) |
Mental disorder | 24 (12%) | 54 (18%) |
Anti-inflammatory drugs | 122 (62%) | 111 (37%) |
Acetaminophen | 75 (38%) | 21 (7%) |
NSAIDs | 61 (31%) | 96 (32%) |
Decongestants | 33 (17%) | 12 (4%) |
Antihistamines | 16 (8%) | 9 (3%) |
Photoplethysmography findings | ||
Dropout rates: median (IQR) | 0.58 (0.42-0.72) | 0.00 (0.00-0.00) |
Number of dropouts (%) | 197/197 (100.0%) | 7/300 (2.3%) |
Maximum dropout rate | 1.00 | 0.01 |
Minimum dropout rate | 0.11 | 0.00 |
No adjustments were made for age, sex, or coexisting morbidity. However, the COVID-19-positive cohort and the control cohort had similar ages (median 67 years [IQR: 54.75-79.25] vs. 59 years [IQR: 35.5-82.5]), gender distribution (59% male vs. 47% male), race (41% vs. 27% Hispanic, 39% vs. 47% Caucasian, and 19% vs. 18% African American), obesity (49% vs. 38%), diabetes (21% vs. 12%), and heart rate (75 [62-88] vs. 83 [IQR: 74-92]).
Patients in both cohorts also had similar comorbidities, although COVID-19-positive patients had slightly higher rates of obesity and were more likely to have taken over-the-counter medications including NSAIDs, acetaminophen, and nasal decongestants on the day of PPG recording than non-COVID patients.
PPG Dropout
PPG recordings were obtained for an average of 3.4 minutes (IQR 3.1-4.2), but all samples were cut to 3 minutes in duration for analysis. To quantify the PPG dropout, the 60-second moving mean and the 60-second moving SD based on infrared PPG data were calculated for each subject in the study. The dropout threshold was calculated as the moving mean minus the moving SD of the signal. A dropout was then defined as any excursion, during a single pulse wave, of the PPG signal below the dropout threshold. To regularize the data, the bias was removed, and the PPG data were normalized by dividing them by 1.65.13
Specifically, the equation for the kth value of the PPG moving average (PPGMAk) is as follows:
where n is the number of points necessary to give a 60-second moving average depending on the sampling rate of the PPG. The equation for the kth value of the PPG moving SD (PPGMSDk) is as follows:
The threshold that defines whether or not a dropout occurs (PPGThreshk) is as follows:
The binary condition for a PPG dropout is given by whether the PPG voltage value drops below PPGThreshk during a single heartbeat or not and is given by the following equation:
Finally, the PPG dropout rate is as follows:
where pulse rate is the number of pulse peaks resulting from cardiac activity that occurs during a single minute.
Figure 1 provides a side-by-side comparison of sample PPG recordings from one non-COVID patient and one COVID-19-positive patient. Both samples were taken from patients included in this study. In these images, the moving average is denoted by a solid blue line, and the dropout threshold is denoted by a solid red line. In the top row of Figure 1’s PPG recordings, the change in morphology can clearly be seen with a sharp downward PPG dropout falling below the dropout threshold (dashed line) for each pulse wave within the COVID-19-positive patient’s sample and for zero pulse waves within the non-COVID patient’s sample. The second row of comparative images condenses the full 2.5-minute recording for each patient and again shows a significant difference in the PPG dropout.
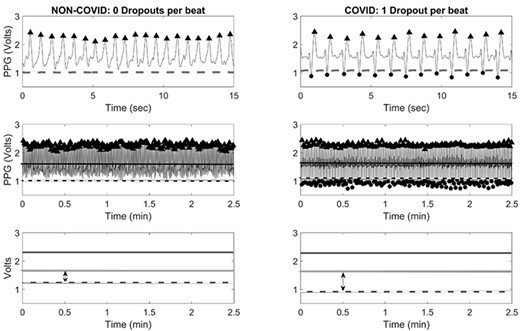
PPG Dropout Rate
The rate of PPG dropout events was calculated for each patient as a ratio of PPG dropouts per pulse wave to the total number of pulse waves per PPG recording. As an example, a PPG dropout rate of 1.00 indicates that 100% of pulse waves within a given PPG recording resulted in a PPG dropout, and a PPG dropout rate of 0.00 indicates that 0% of pulse waves within a given PPG tracing resulted in a PPG dropout. The PPG dropout rate was calculated for each patient and trends in dropout rates for COVID-19-positive patients and for non-COVID patients are presented in Table I. Non-COVID control patients exhibited a median PPG dropout rate of 0.00 (median PPG dropout rate: 0, IQR 0.00-0.00, P < .05). Patients with COVID-19 exhibited a mean PPG dropout rate of 0.58 (median PPG dropout rate: 0.58, IQR 0.42-0.72, P < .05).
The highest and lowest PPG dropout rates are also provided in Table I. Of the 300 non-COVID patients, seven (2.3%) experienced a PPG dropout in at least one pulse wave. Among non-COVID patients, the lowest recorded PPG dropout rate was 0.00, and the highest recorded PPG dropout rate was 0.01. This indicates that the highest PPG dropout rate demonstrated by a non-COVID patient was one PPG dropout per one-hundred pulse waves. Conversely, of the 197 COVID-19-positive patients, 197 (100%) experienced PPG dropout in one or more pulse waves. Among patients with COVID-19, the lowest recorded PPG dropout rate was 0.11 and the highest recorded PPG dropout rate was 1.00. These results reached statistical significance.
PPG and D-dimer
D-dimer is an established biophysical marker for COVID-19-positive disease progression and severity.7,8 We therefore trended PPG dropout rates and compared PPG dropout rates to trends in serum D-dimer levels for all included COVID-19-positive patients. Of the 197 COVID-19-positive patients, 21 patients had seven or more consecutive days of D-dimer lab values available and, therefore, had adequate data for D-dimer trend analysis. The remaining patients were discharged or expired within 7 days of admission.
Figure 2 displays a sample trend of the normalized serum D-dimer protein level and PPG dropout rate plotted over time. This figure, which is represnetative of the overall dataset, shows a strong temporal correlation between the PPG dropout rate and the serum D-dimer levels. The PPG data in the figures were normalized by 1.65 and the DC bias was removed. The D-dimer levels were normalized by dividing all the levels by the maximum D-dimer level acquired for that patient during their stay.

Figure 3 is a scatter plot comparing trends in the D-dimer change to trends in the PPG dropout rate change. For each patient, the normalized percent change in D-dimer during the patient’s hospitalization (patient’s maximum D-dimer value minus minimum D-dimer value divided by maximum D-dimer during hospitalization) was plotted against the corresponding change in the PPG dropout rate for PPG recordings taken on the dates of the maximum and minimum D-dimer levels (the PPG dropout rate from the date of the maximum D-dimer level minus the PPG dropout rate from the date of the minimum D-dimer level) for all 197 COVID-19 patients with at least two D-dimer and PPG data points. The data were then analyzed using linear regression which resulted in an R2 = 0.743 (P = 1.71E−105), demonstrating a strong correlation between changes in D-dimer levels and changes in PPG dropout rates in COVID-19 patients.
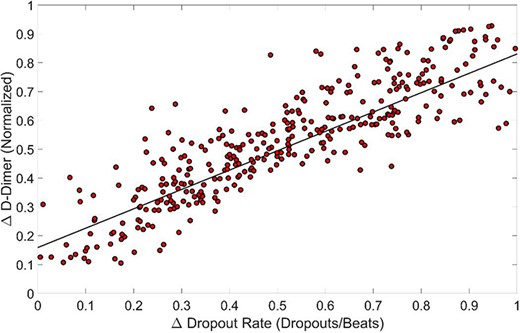
DISCUSSION
A growing body of research points to endotheliitis as a cause of hemorheological changes and a key component of COVID-19 pathophysiology.14–18 Under normal conditions, endothelial cells help prevent coagulation within the intravascular space by producing heparan sulfate proteoglycans within the glycocalyx; by expressing thrombomodulin, which binds to thrombin and limits its affinity for fibrinogen and other coagulation factors; by expressing endothelial protein C receptors that facilitate factor C activation; and by releasing tissue-type plasminogen activator.15 However, COVID-19 disrupts these processes and damages endothelial cells via direct viral toxicity and immune-mediated inflammatory changes.16–19 In a 2020 Lancet study, Varga et al. provided histological evidence showing the presence of viral bodies within endothelial cells as well as the presence of accumulated inflammatory cells with evidence of endothelial and inflammatory cell death.19 The authors concluded that COVID-19 infection resulted in endotheliitis in multiple organs throughout the body. This finding is supported by other studies reporting severe endothelial damage in pathology specimens from both fatal and nonfatal cases of COVID-19.16 COVID-19 has also been directly shown to infect engineered human blood vessel endothelial cells in vitro.20
Njoum et al. evaluated the sensitivity of PPGAC and PPGDC components to changes in hemorheology following changes in endovascular shear rates and induced clotting in vitro.6 The authors reported that the PPGAC amplitude started to decrease within a few seconds of administering the clotting agent and continued to drop until the blood achieved the maximum clotting after approximately 20 minutes. The PPGAC waveform at that moment displayed significantly lower amplitudes and disrupted the PPGAC morphology.6 These findings can be explained by the presence of a fibrinous matrix, which alters the absorption and scattering properties of the sample. Similar PPG changes have also been evaluated in the context of sympathetic blockade,21–23 thermal stress,24 sleep,25,26 and altered venous oxygenation.27 Recent investigations have reported that the PPG amplitude drops during sleep is an independent marker for cardiometabolic outcomes26 and hypertension.27
Given the current body of evidence surrounding inflammation-induced hemorheological changes caused by COVID-19, it is unsurprising that patients with COVID-19 experienced increased rates of PPG dropout. Of the 197 enrolled ICU patients with COVID-19, every patient exhibited at least one PPG dropout event during PPG recording (n = 197, P < .05). Furthermore, within COVID-19-positive PPG tracings, the median PPG dropout rate was 0.58 (median 0.58, IQR 0.42-0.72, P < .05). This indicates that roughly 58% of individual pulse waves within the cumulative PPG tracings of all 197 patients translated into a PPG dropout event. PPG dropout was not only displayed by every included COVID-19 patient but also displayed to a considerable extent.
In contrast, non-COVID patients had low rates of PPG dropout with a median PPG dropout rate of 0.0 (median 0.0, IQR 0.0-0.0, P < .05). Because non-COVID control patients underwent PPG recording before the arrival of COVID-19 to the United States, these patients were assumed to be without COVID-19-related inflammatory or hypercoagulable processes. It was therefore expected that these patients would produce PPG tracings without PPG dropouts. However, because these control patients were retrospectively selected using computer randomization, it was impossible to control for all confounding factors. Among non-COVID control patients, seven patients or 2.3% of controls displayed one or more PPG dropout events. The events observed in the control population may be attributable to artifacts or may be the result of underlying disease processes such as liver disease, diabetes, or sepsis.
The lowest PPG dropout rate recorded among patients with COVID-19 was 0.11, and the highest PPG dropout rate recorded among patients without COVID-19 was 0.01. Even the PPG dropout rate for the lowest outlier among patients with COVID-19 is higher than the highest reported PPG dropout rate displayed among patients without COVID-19 by more than a factor of 10. This is unsurprising given that COVID-19 is strongly inflammatory and patients within the ICU have severe cases. However, PPG dropout may not be specific to COVID-19, and evaluations of possible PPG changes in the context of sepsis, cardiovascular disease, and similar inflammatory processes are also warranted.
The PPG dropout rate also correlated closely with normalized D-dimer trends. COVID-19 results in increased arterial, venous, and microvascular thrombus formation.5,28,29 Furthermore, despite the frequent use of prophylactic and therapeutic anticoagulation, patients with COVID-19 display similar thrombin generation to healthy controls.7 D-dimer is a well-described biophysical marker used for following COVID-19 disease severity and prognosis.7,8 Xiaokang et al. evaluated the value of D-dimer as a tool for COVID-19 prognosis in 1,114 patients with COVID-19 from Wuhan, China.8 The authors reported that D-dimer levels were closely related to COVID-19 prognosis and that D-dimer levels were more likely to be elevated among severely ill patients than patients with mild disease.8 Xiaokang et al. also reported that D-dimer levels were significantly higher among patients who died from COVID-19 than those who survived and that the optimal probability cutoff value for D-dimer was 2.025 mg/L.8 Strong correlation between D-dimer levels and PPG dropout rates following linear regression (R2 = 0.743 [P < .05]) therefore supports the potential clinical value of monitoring for PPG dropout.
Limitations
This study has several limitations including the sample size and the use of retrospectively collected control patients. Recruitment was limited by the ability of a single clinical research coordinator to obtain armband PPG recordings for all included patients and for physician investigators to review relevant electronic medical records. The sample size was generally adequate, but this limitation became apparent during the comparison of PPG dropout and D-dimer level trends. A minimum of seven sequential daily D-dimer levels was required to establish a serial D-dimer and PPG dropout trend. Therefore, all patients who were discharged or deceased within 7 days were excluded from that portion of the analysis, leaving only 21 included patients. However, we chose to include all available data from all 197 patients with COVID-19, irrespective of the duration of stay to complete a scatterplot comparison of serum D-dimer values with the PPG dropout rate, and linear regression demonstrated a strong correlation.
Because the control population was randomly and retrospectively collected, we did not select a matched population for comparison. However, the goal was to identify a population that reflected the general population of patients treated within our institution, and the two cohorts were similar in terms of age, sex, ethnicity, and comorbidities.
CONCLUSION
Changes in PPG were previously described in vitro following artificially induced clotting activation. The present study analyzed PPG waveforms from 197 patients with COVID-19 (a population with well-described hypercoagulability and pro-inflammatory processes) vs. 300 non-COVID controls to determine whether similar changes could be seen in vivo. PPG dropout rates were successfully identified, and the rate of these changes was significantly higher among patients with COVID-19 than patients without COVID-19, closely mirroring trends in serum D-dimer elevation.
PPG dropout rates may be a useful addition to options for biophysical monitoring. PPG has the benefits of being noninvasive, painless, and dynamic (offering results in real time). PPG can be placed on a patient continuously for monitoring of evolving clinical states and can also be used remotely, limiting disease exposure. However, inflammatory changes and hypercoagulability are not unique to COVID-19, and further investigations should evaluate the presence of PPG dropout during other inflammatory disease processes.
CLINICAL SIGNIFICANCE
The present study is not a proof of concept advocating for the use of PPG for COVID-19 detection over existing measures. However, the results from this study establish that PPG tracings undergo a morphological change in the context of a pro-inflammatory and hypercoagulable state and that these changes mirror changes in serum D-dimer levels, which already have established clinical use. The clinical significance of these results is to establish that these changes can be seen in vivo, which can provide a foundation for future studies to investigate the potential use of PPG for disease diagnostic or prognostic measures.
FUNDING
Armband PPG recordings were obtained using the Tiger Tech Warfighter Monitor. The PPG device used in this study (Tiger Tech Warfighter Monitor) was provided by Tiger Tech Solutions, Inc. The Tiger Tech Warfighter Monitor was developed partially under a Small Business Innovation Research contract (W81XWH19C0059) from the Defense Health Agency. However, no funding was provided for the present post-development study using the device.
CONFLICT OF INTEREST STATEMENT
J.P., MD, S.J.L., PhD, and F.H., MD, have no conflicts of interest to declare. The PPG device used in this study (Tiger Tech Warfighter Monitor) was provided by Tiger Tech Solutions, Inc. S.H., BA, is a research coordinator employed by Tiger Tech Solutions, Inc. M.J.W., PhD, is the Head of Data Sciences and Analytics for Tiger Tech Solutions, Inc. S. H.W., MD is the Chief Medical Officer for Tiger Tech Solutions, Inc. H.W., Esq., is the Chief Executive Officer for Tiger Tech Solutions, Inc.
DATA AVAILABILITY
The data underlying this article will be shared on reasonable request to the corresponding author.
CLINICAL TRIAL REGISTRATION
None declared.
INSTITUTIONAL REVIEW BOARD (HUMAN SUBJECTS)
This study was approved by the Mount Sinai Medical Center Institutional Review Board (Federalwide Assurance Number: FWA00000176).
INSTITUTIONAL ANIMAL CARE AND USE COMMITTEE (IACUC)
Not applicable.
INSTITUTIONAL CLEARANCE
Institutional clearance approved.
INDIVIDUAL AUTHOR CONTRIBUTION STATEMENT
J.P. was involved in the research design/concept, study implementation, and writing of the initial draft and subsequent revisions. M.J.W. was involved in the research design/concept, study implementation, data analysis, and manuscript revisions. H.W. was involved in the research design/concept, study implementation, data collection, and manuscript revision. F.H. was involved in the study implementation, data collection, and manuscript revision. S.H. was involved in the data collection and manuscript revision. S.J.L. was involved in the research design/concept, study implementation, study supervision/mentorship, and manuscript revision. S.H.W. was involved in the research design/concept, study implementation, study supervision/mentorship, and manuscript revision.
REFERENCES
Author notes
Preliminary results were presented at the International Anesthesia Research Society virtual conference, which was held in May 2021.
The views expressed in this material are those of the authors and do not reflect the official policy or position of the U.S. Government, the DoD, or the Department of the Army.