-
PDF
- Split View
-
Views
-
Cite
Cite
Carlos D Messina, Jose Rotundo, Graeme L Hammer, Carla Gho, Andres Reyes, Yinan Fang, Erik van Oosterom, Lucas Borras, Mark Cooper, Radiation use efficiency increased over a century of maize (Zea mays L.) breeding in the US corn belt, Journal of Experimental Botany, Volume 73, Issue 16, 12 September 2022, Pages 5503–5513, https://doi.org/10.1093/jxb/erac212
- Share Icon Share
Abstract
In the absence of stress, crop growth depends on the amount of light intercepted by the canopy and the conversion efficiency [radiation use efficiency (RUE)]. This study tested the hypothesis that long-term genetic gain for grain yield was partly due to improved RUE. The hypothesis was tested using 30 elite maize hybrids commercialized in the US corn belt between 1930 and 2017. Crops grown under irrigation showed that pre-flowering crop growth increased at a rate of 0.11 g m–2 year–1, while light interception remained constant. Therefore, RUE increased at a rate of 0.0049 g MJ–1 year–1, translating into an average of 3 g m–2 year–1 of grain yield over 100 years of maize breeding. Considering that the harvest index has not changed for crops grown at optimal density for the hybrid, the cumulative RUE increase over the history of commercial maize breeding in the USA can account for ~32% of the documented yield trend for maize grown in the central US corn belt. The remaining RUE gap between this study and theoretical maximum values suggests that a yield improvement of a similar magnitude could be achieved by further increasing RUE.
Introduction
In the absence of abiotic and biotic stress, crop growth depends on the amount of light intercepted and absorbed by the canopy and the conversion efficiency, also known as radiation use efficiency (RUE). RUE is quantified as the mole ratio between reduced carbon and absorbed photons. In field studies, RUE is approximated by the ratio between grams of dry matter per MJ (g MJ–1) (Monteith and Moss, 1977; Sinclair and Muchow, 1999). RUE for maize was estimated between 1.5 g MJ–1 and 1.86 g MJ–1 of intercepted solar radiation (Sinclair and Muchow, 1999). Using modern germplasm with erect leaves, Lindquist et al. (2005) reported an RUE a value of 1.85 g MJ–1, which is at the upper bound of the values previously reviewed by Sinclair and Muchow (1999). During reproductive stages, RUE often decreases due to a reduction in leaf nitrogen (N) concentration (Sinclair and Horie, 1989; Sinclair and Muchow, 1995). RUE can also vary with changes in (i) atmospheric transmission, partitioning of radiation between direct and diffuse radiation (Sinclair et al., 1992), and N uptake and its vertical distribution within the canopy (Hammer and Wright, 1994); (ii) leaf erectness and leaf area index (LAI; Duncan, 1971); (iii) atmospheric vapor pressure deficit (VPD; Stockle and Kiniry, 1990; Kiniry et al., 1998) and air temperature (Andrade et al., 1992, 1993; Stirling et al., 1993); and (iv) efficiency of light interception due to leaf area distribution (Perez et al., 2019).
Leaf erectness in temperate maize increased concurrently with year of commercialization (Duvick et al., 2004; Fig. 1) or in response to selection (Edwards, 2011). Leaf angle scores increased ~1 point per decade (Duvick et al., 2004) and accelerated concurrently with the introduction of single-cross maize breeding in the 1960s (Duvick et al., 2004). Yields measured in experiments conducted in the absence of water limitations increased at a rate of 8.6 ± 0.4 g m–2 year-1, which is similar to the improvement recorded in experiments conducted in the central US corn belt (Cooper et al., 2014). Agronomic management increased over the year, notably the increase in plant density. These concurrent changes, along with the effect of plant density on light interception and of leaf erectness on RUE (Duncan, 1971; Hammer and Wright, 1994; Long et al., 2006; Yin et al., 2015), suggest a role for leaf erectness in the genetic gain in yield.
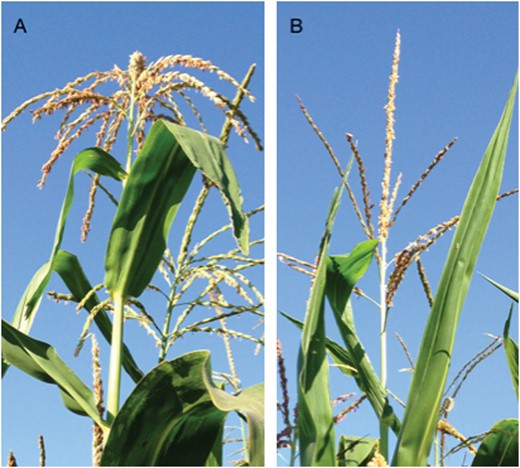
Canopy architecture examples for hybrids commercialized in 1930 (A) and 2010 (B).
Experimental work provides evidence to support a positive relationship between leaf erectness and yield (Pendleton et al., 1968; Pepper et al., 1977; Lambert and Johnson, 1978). A positive correlation between leaf erectness interacting with plant density and yield in maize was demonstrated in simulation studies (Hammer et al., 2009). Simulation of breeding programs suggests that leaf erectness can both increase and decrease with cycles of selection when positive selection is applied to yield, depending on the intensity of drought stress conditions (Messina et al., 2009, 2011). Because both drought and well-watered environments are components of the target population of environments (TPE) of maize (Messina et al., 2015; Cooper et al., 2020), a causal relationship between changes in leaf angle and yield would depend on the frequencies of environment types that occurred during maize breeding for all breeding programs contributing germplasm to the development of maize hybrids.
Leaf carbon exchange, self-organization, and lower plant to plant variation were reported to change with selection in an Argentinian semi-flint panel and the Ontario panel (Cagnola et al., 2021; Gonzales et al., 2021), and a change in light interception efficiency (Perez et al., 2019) was reported for a European maize panel. However, direct evidence for changes in RUE with cycles of selection is limited to non-temperate maize germplasm. RUE increased during grain fill but not during vegetative stages in northern North American hybrids (Tollenaar and Aguilera, 1992), marginally increased in tropical germplasm (Bolaños and Edmeades, 1993), and significantly changed in Argentinian semi-flint hybrids (Luque et al., 2006). Studies in wheat in Australia (Sadras and Lawson, 2011) reported an increase in RUE over 50 years of varietal releases. The objective of this study was to test the hypothesis that long-term genetic gain for grain yield in the longest running commercial breeding program for maize was due, at least in part, to increased RUE.
Materials and methods
Field experiments
Field experiments were conducted over five growing seasons in Chile and the USA (Table 1). At both locations, weather variables were monitored using an automated weather station (Onset Corp, St. Louis, MO, USA with a silicon pyranometer Onset S-Lib-M003) and data are summarized in Fig. 2. The experiments were planted in six-row plots in Chile and four-row plots in the USA. All plots were 4.5 m long with 0.76 m spacing between rows. Each row was irrigated through a dedicated drip tape buried 12 cm below the soil surface to ensure good stand establishment, incorporation of fertilizer, and satisfied crop water demands. Plant stands were thinned to nine plants per square meter in the USA and 11 plants per square meter in Chile. Fertilizer applications were 26.1 g m–2 and 18.7 g m–2 of N in each growing season in the Chile and US experiments, respectively. Experiments were maintained free of pests, diseases, and weeds.
Agronomic management and sampling details for experiments conducted at Corteva research centers
Year . | Location . | Planting date . | Latitude/longitude . | Irrigation (mm) . | SWHC (cm3 cm–3) . | Initial biomass at sampling (g m–2) . | Sampling period (d) . |
---|---|---|---|---|---|---|---|
2012 | USA | 7 May | 38.7/–121.8 | 529 | 0.13 | 714.1 | 42 |
2017 | USA | 20 May | 38.7/–121.8 | 679 | 0.13 | 194.0 | 30 |
2011 | Chile | 24 November | –33.8/–70.8 | 612 | 0.16 | 671.3 | 42 |
2017 | Chile | 23 October | –33.8/–70.8 | 673 | 0.16 | 632.7 | 29 |
2019 | Chile | 28 October | –33.8/–70.8 | 841 | 0.16 | 216.6 | 36 |
Year . | Location . | Planting date . | Latitude/longitude . | Irrigation (mm) . | SWHC (cm3 cm–3) . | Initial biomass at sampling (g m–2) . | Sampling period (d) . |
---|---|---|---|---|---|---|---|
2012 | USA | 7 May | 38.7/–121.8 | 529 | 0.13 | 714.1 | 42 |
2017 | USA | 20 May | 38.7/–121.8 | 679 | 0.13 | 194.0 | 30 |
2011 | Chile | 24 November | –33.8/–70.8 | 612 | 0.16 | 671.3 | 42 |
2017 | Chile | 23 October | –33.8/–70.8 | 673 | 0.16 | 632.7 | 29 |
2019 | Chile | 28 October | –33.8/–70.8 | 841 | 0.16 | 216.6 | 36 |
SWHC: soil water holding capacity. Initial biomass at sampling is the biomass at first sampling for crop growth rate determination. Sampling period is the number of days between the first and last biomass sample for crop growth rate determination.
Agronomic management and sampling details for experiments conducted at Corteva research centers
Year . | Location . | Planting date . | Latitude/longitude . | Irrigation (mm) . | SWHC (cm3 cm–3) . | Initial biomass at sampling (g m–2) . | Sampling period (d) . |
---|---|---|---|---|---|---|---|
2012 | USA | 7 May | 38.7/–121.8 | 529 | 0.13 | 714.1 | 42 |
2017 | USA | 20 May | 38.7/–121.8 | 679 | 0.13 | 194.0 | 30 |
2011 | Chile | 24 November | –33.8/–70.8 | 612 | 0.16 | 671.3 | 42 |
2017 | Chile | 23 October | –33.8/–70.8 | 673 | 0.16 | 632.7 | 29 |
2019 | Chile | 28 October | –33.8/–70.8 | 841 | 0.16 | 216.6 | 36 |
Year . | Location . | Planting date . | Latitude/longitude . | Irrigation (mm) . | SWHC (cm3 cm–3) . | Initial biomass at sampling (g m–2) . | Sampling period (d) . |
---|---|---|---|---|---|---|---|
2012 | USA | 7 May | 38.7/–121.8 | 529 | 0.13 | 714.1 | 42 |
2017 | USA | 20 May | 38.7/–121.8 | 679 | 0.13 | 194.0 | 30 |
2011 | Chile | 24 November | –33.8/–70.8 | 612 | 0.16 | 671.3 | 42 |
2017 | Chile | 23 October | –33.8/–70.8 | 673 | 0.16 | 632.7 | 29 |
2019 | Chile | 28 October | –33.8/–70.8 | 841 | 0.16 | 216.6 | 36 |
SWHC: soil water holding capacity. Initial biomass at sampling is the biomass at first sampling for crop growth rate determination. Sampling period is the number of days between the first and last biomass sample for crop growth rate determination.
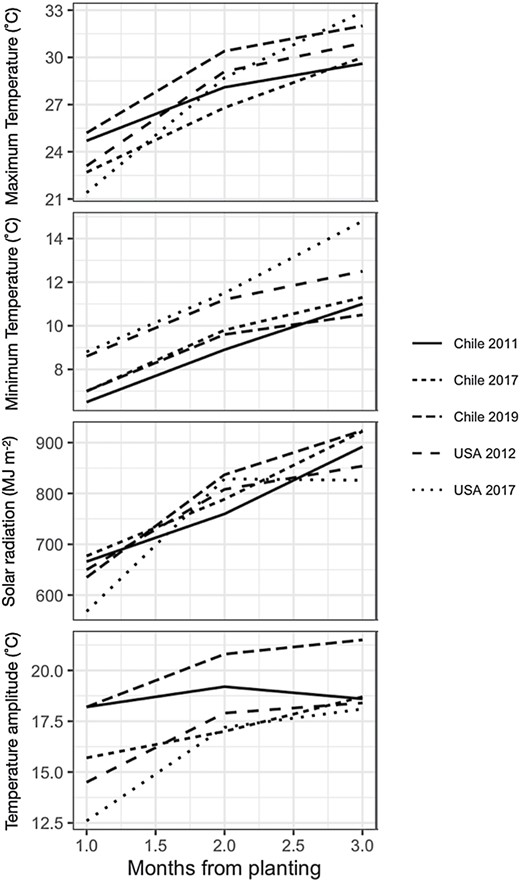
Temporal dynamics of monthly solar radiation (MJ m–2), minimum temperature (°C), maximum temperature (°C), and temperature amplitude (°C) in months after planting.
Hybrids
Thirty hybrids that were commercialized between 1934 and 2017 were planted in experiments in five growing seasons (Table 2). These represented a sample of double-cross and single-cross hybrids that have previously been included in long-term genetic gain experiments for maize in the US corn belt (Duvick et al., 2004; Cooper et al., 2014). These so-called ERA hybrids represent a historical sequence of hybrids that were widely grown in the US corn belt after their commercial release. Field experimentation in managed drought environments and in the US corn belt demonstrated yield improvement under various environmental conditions with a concurrent change in leaf angle (Duvick et al., 2004; Cooper et al., 2006).
Single-cross maize hybrids included in experiments conducted in the USA and Chile and their year of commercial release
Hybrid . | Year . | USA 2012 . | USA 2017 . | Chile 2011 . | Chile 2017 . | Chile 2019 . |
---|---|---|---|---|---|---|
351 | 1934 | x | ||||
322 | 1936 | x | x | |||
340 | 1941 | x | ||||
352 | 1946 | x | ||||
347 | 1950 | x | ||||
301B | 1952 | x | ||||
3306 | 1963 | x | x | |||
3376 | 1965 | x | x | x | x | |
3334 | 1969 | x | ||||
3366 | 1972 | x | x | x | ||
3301A | 1974 | x | x | |||
3541 | 1975 | x | x | x | x | |
3382 | 1976 | x | x | x | ||
3377 | 1982 | x | x | x | x | x |
3475 | 1984 | x | x | x | ||
3379 | 1988 | x | x | x | x | x |
3394 | 1991 | x | x | x | x | x |
3489 | 1994 | x | x | x | ||
34G81 | 1997 | x | x | x | ||
33P67 | 1999 | x | x | x | x | x |
34H31 | 2002 | x | x | x | x | |
34A16 | 2005 | x | ||||
33D14 | 2007 | nT | x | nT | ||
33D49 | 2007 | x, nT | x | x | ||
35F40 | 2007 | x | x | |||
33W84 | 2008 | x | x | |||
P1162XR | 2009 | x | ||||
P1151YHR | 2011 | x | x | x | ||
P1197YHR | 2014 | x | x | x | ||
P0574YHR | 2017 | X |
Hybrid . | Year . | USA 2012 . | USA 2017 . | Chile 2011 . | Chile 2017 . | Chile 2019 . |
---|---|---|---|---|---|---|
351 | 1934 | x | ||||
322 | 1936 | x | x | |||
340 | 1941 | x | ||||
352 | 1946 | x | ||||
347 | 1950 | x | ||||
301B | 1952 | x | ||||
3306 | 1963 | x | x | |||
3376 | 1965 | x | x | x | x | |
3334 | 1969 | x | ||||
3366 | 1972 | x | x | x | ||
3301A | 1974 | x | x | |||
3541 | 1975 | x | x | x | x | |
3382 | 1976 | x | x | x | ||
3377 | 1982 | x | x | x | x | x |
3475 | 1984 | x | x | x | ||
3379 | 1988 | x | x | x | x | x |
3394 | 1991 | x | x | x | x | x |
3489 | 1994 | x | x | x | ||
34G81 | 1997 | x | x | x | ||
33P67 | 1999 | x | x | x | x | x |
34H31 | 2002 | x | x | x | x | |
34A16 | 2005 | x | ||||
33D14 | 2007 | nT | x | nT | ||
33D49 | 2007 | x, nT | x | x | ||
35F40 | 2007 | x | x | |||
33W84 | 2008 | x | x | |||
P1162XR | 2009 | x | ||||
P1151YHR | 2011 | x | x | x | ||
P1197YHR | 2014 | x | x | x | ||
P0574YHR | 2017 | X |
35A52 was included in the Chile 2917 experiment. nT indicates the non-transgenic version of the hybrid.
Single-cross maize hybrids included in experiments conducted in the USA and Chile and their year of commercial release
Hybrid . | Year . | USA 2012 . | USA 2017 . | Chile 2011 . | Chile 2017 . | Chile 2019 . |
---|---|---|---|---|---|---|
351 | 1934 | x | ||||
322 | 1936 | x | x | |||
340 | 1941 | x | ||||
352 | 1946 | x | ||||
347 | 1950 | x | ||||
301B | 1952 | x | ||||
3306 | 1963 | x | x | |||
3376 | 1965 | x | x | x | x | |
3334 | 1969 | x | ||||
3366 | 1972 | x | x | x | ||
3301A | 1974 | x | x | |||
3541 | 1975 | x | x | x | x | |
3382 | 1976 | x | x | x | ||
3377 | 1982 | x | x | x | x | x |
3475 | 1984 | x | x | x | ||
3379 | 1988 | x | x | x | x | x |
3394 | 1991 | x | x | x | x | x |
3489 | 1994 | x | x | x | ||
34G81 | 1997 | x | x | x | ||
33P67 | 1999 | x | x | x | x | x |
34H31 | 2002 | x | x | x | x | |
34A16 | 2005 | x | ||||
33D14 | 2007 | nT | x | nT | ||
33D49 | 2007 | x, nT | x | x | ||
35F40 | 2007 | x | x | |||
33W84 | 2008 | x | x | |||
P1162XR | 2009 | x | ||||
P1151YHR | 2011 | x | x | x | ||
P1197YHR | 2014 | x | x | x | ||
P0574YHR | 2017 | X |
Hybrid . | Year . | USA 2012 . | USA 2017 . | Chile 2011 . | Chile 2017 . | Chile 2019 . |
---|---|---|---|---|---|---|
351 | 1934 | x | ||||
322 | 1936 | x | x | |||
340 | 1941 | x | ||||
352 | 1946 | x | ||||
347 | 1950 | x | ||||
301B | 1952 | x | ||||
3306 | 1963 | x | x | |||
3376 | 1965 | x | x | x | x | |
3334 | 1969 | x | ||||
3366 | 1972 | x | x | x | ||
3301A | 1974 | x | x | |||
3541 | 1975 | x | x | x | x | |
3382 | 1976 | x | x | x | ||
3377 | 1982 | x | x | x | x | x |
3475 | 1984 | x | x | x | ||
3379 | 1988 | x | x | x | x | x |
3394 | 1991 | x | x | x | x | x |
3489 | 1994 | x | x | x | ||
34G81 | 1997 | x | x | x | ||
33P67 | 1999 | x | x | x | x | x |
34H31 | 2002 | x | x | x | x | |
34A16 | 2005 | x | ||||
33D14 | 2007 | nT | x | nT | ||
33D49 | 2007 | x, nT | x | x | ||
35F40 | 2007 | x | x | |||
33W84 | 2008 | x | x | |||
P1162XR | 2009 | x | ||||
P1151YHR | 2011 | x | x | x | ||
P1197YHR | 2014 | x | x | x | ||
P0574YHR | 2017 | X |
35A52 was included in the Chile 2917 experiment. nT indicates the non-transgenic version of the hybrid.
Light interception
Cumulative light interception was estimated as the sum of the product of the daily solar radiation times the daily relative light interception. Relative light interception was calculated as the ratio between the solar radiation measured above the uppermost senesced leaf within the canopy, and the incident solar radiation under non-shaded conditions at the top of the canopy. Data were collected within 1 h of solar noon for every plot in all replications using a linear quantum sensor AccuPAR (Decagon Devices, Inc., Pullman, WA, USA). Data collection dates were 45, 51, 60, and 80 days after planting (dap) in the USA in 2012; 33, 51, and 62 dap in the USA in 2017; 49, 56, 61, 68, and 83 dap in Chile in 2011; 50, 59, 66, and 78 dap in Chile in 2017; and 38, 46, 56, and 73 dap in Chile in 2019. Linear interpolation was used to estimate values between data collection dates. Four measurements per plot were recorded by placing the sensor at an angle such that the ends of the sensor touched two planting rows. The average of these four measurements was utilized as the value for the plot and day for statistical analyses. For 2011, solar radiation data reported by a weather station located <300 m from the experimental site in Chile were used to estimate incident solar radiation for relative light interception calculations.
Mass measurements, crop growth rate, and RUE calculations
Crop mass was measured between three and five times during the growing season concurrently with measurements of light interception. Plants within 1 m2 were harvested from two bordered planting rows at each sampling time. Plants were cut at ground level, and whole-plant samples were dried to constant weight at 70 °C. Dry weight was expressed in g m–2. Crop growth rate for the sampling period was calculated as the slope of the regression between cumulative biomass and time. The biomass (g m–2) of the first sample for crop growth rate determination and the sampled period (days) for the five experiments are reported in Table 1. RUE was calculated as the slope of the regression between the cumulative mass and intercepted total solar radiation (Sinclair and Muchow, 1999).
A conversion factor was calculated to report RUE in mole of CO2 per mole of photons. To estimate the moles of CO2 required to produce a gram of dry matter, we made the following assumptions: (i) glucose requirements (g GLU g per product) were equal to 1.21 for carbohydrates, 2.48 for protein, 2.71 for lipids, and 1.92 for lignin (Loomis and Connor, 1992); and (ii) dry matter composition was 85% carbohydrate, 11% protein, 3% lipid, and 2% lignin. Based on these assumptions, we calculated that 1.4 g of GLU is required to produce 1 g of dry matter. Dividing the GLU equivalents by the molecular weight of GLU, multiplying by 6, which is the number of moles of CO2 required to produce a mole of GLU, and multiplying by 0.21 MJ per mole of photons (Nobel, 2009), we propose a conversion factor of 9.8 to convert grams of dry matter per MJ into mmoles of CO2 per mole of photons.
Statistical analysis
All experiments had a randomized complete block design with replications blocked using prior information on soil spatial variability patterns. Hybrids were randomized within replications. Five replications were included in each experiment in 2017, while three replications were included in all other experiments. For each plot, the first recorded observation was used as the base or time zero. The cumulative biomass for each day was calculated by taking the difference between observed biomass at time t and time zero. Cumulative solar radiation for each day was calculated in a similar manner.
A two-stage analysis was used to estimate crop growth, light interception, and RUE trend over hybrid year of release. The stage one model for RUE models the cumulative dry matter as a function of cumulative solar radiation using a linear model. Formally, for intercept β0=0, the slope β1 is the best unbiased linear estimator (BLUE) for RUE,
where yijklm, xijklm, and ijklm are vectors of cumulative dry matter and solar radiation for each plot, and residuals (each element is an observation over time). RUE (β1) was modeled as a function of location Ei, hybrid Hj, the interaction (E×H)ij, block bk within location (b/E)ki, and row rl and column cm within location (r/E)li and (c/E)mi, respectively. Overlines for model terms indicate random effects,
For each environment, residuals ijklm were modeled using a first-order autoregressive model (AR1) with variance σ2i and correlation matrix Σi,
The stage two model used BLUEs computed for RUE for each location and hybrid as,
Average genetic gain (α1i) for a location was modeled as a function of hybrid and year of commercialization for the hybrid (tj) as,
By subtracting 1930 from tj, α0 is an estimator of RUE at the designed onset of 1930 for the sequence of hybrids for the breeding program, where α0 and α1 are in turn BLUEs for the intercept and slope after subtracting their corresponding random error term for the location i.
Results
Environments
Monthly maximum, minimum, and amplitude of temperature, and solar radiation are shown in Fig. 2. Monthly mean temperature during the measurement periods (18.7–21.1 °C), but not minimum temperatures (Fig. 2), was optimal for leaf photosynthesis (Rotundo et al., 2019). VPD varied between 2.5 kPa and 3.2 kPa, which was above the threshold at which RUE has been found to decrease with increasing VPD (0.9 kPa; Kiniry et al., 1998). Differences in temperature amplitude among locations are most evident (Fig. 2).
Light interception is a virtual constant in modern plant populations
Cumulative light interception increased with the progression of the crop towards maturity, with rates that varied among hybrids (P<0.001), environments (P<0.01), and for hybrids within environment (P<0.001). BLUEs were calculated using all three sources of variation in order to estimate changes in light interception with respect to year of commercialization, which decreased at a rate of –0.0097 MJ m–2 year–1 (Table 3). Figure 3 shows BLUEs for intercepted radiation for Chile and the USA by hybrid and location. The slight negative trend in light interception, while consistent with increased leaf angle, is small in magnitude. The size of the random component relative to the standard error of the estimate for the environment effect on cumulative light interception for the 1930 hybrid suggests that the effect is significant (4.6 ± 3.3).
Estimates and standard errors for parameters of a linear model of radiation use efficiency, crop growth rate, and cumulative intercepted radiation in relation to year of commercialization
Source . | Radiation use efficiency . | Crop growth rate . | Daily intercepted radiation . |
---|---|---|---|
g MJ–1 year–1 | g m–2 d–1 year–1 | MJ m–2 d–1 year–1 | |
Year | 0.0049 ± 0.0012 | 0.11 ± 0.03 | –0.0097 ± 0.005 |
g MJ–1 | g m–2 d–1 | MJ m–2 d–1 | |
Intercept | 1.54 ± 0.17 | 39.4 ± 3.2 | 25.9 ± 1.0 |
Source . | Radiation use efficiency . | Crop growth rate . | Daily intercepted radiation . |
---|---|---|---|
g MJ–1 year–1 | g m–2 d–1 year–1 | MJ m–2 d–1 year–1 | |
Year | 0.0049 ± 0.0012 | 0.11 ± 0.03 | –0.0097 ± 0.005 |
g MJ–1 | g m–2 d–1 | MJ m–2 d–1 | |
Intercept | 1.54 ± 0.17 | 39.4 ± 3.2 | 25.9 ± 1.0 |
The intercept of the model estimates the radiation use efficiency, crop growth rate, and daily intercepted radiation at year of release 1930.
Estimates and standard errors for parameters of a linear model of radiation use efficiency, crop growth rate, and cumulative intercepted radiation in relation to year of commercialization
Source . | Radiation use efficiency . | Crop growth rate . | Daily intercepted radiation . |
---|---|---|---|
g MJ–1 year–1 | g m–2 d–1 year–1 | MJ m–2 d–1 year–1 | |
Year | 0.0049 ± 0.0012 | 0.11 ± 0.03 | –0.0097 ± 0.005 |
g MJ–1 | g m–2 d–1 | MJ m–2 d–1 | |
Intercept | 1.54 ± 0.17 | 39.4 ± 3.2 | 25.9 ± 1.0 |
Source . | Radiation use efficiency . | Crop growth rate . | Daily intercepted radiation . |
---|---|---|---|
g MJ–1 year–1 | g m–2 d–1 year–1 | MJ m–2 d–1 year–1 | |
Year | 0.0049 ± 0.0012 | 0.11 ± 0.03 | –0.0097 ± 0.005 |
g MJ–1 | g m–2 d–1 | MJ m–2 d–1 | |
Intercept | 1.54 ± 0.17 | 39.4 ± 3.2 | 25.9 ± 1.0 |
The intercept of the model estimates the radiation use efficiency, crop growth rate, and daily intercepted radiation at year of release 1930.
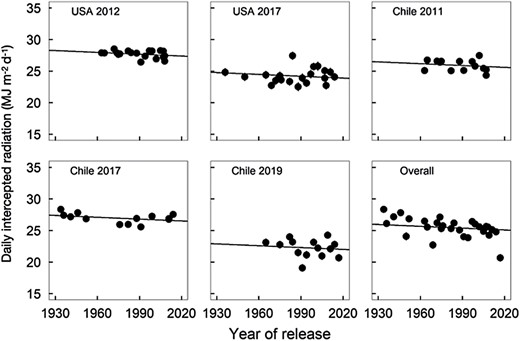
Best linear unbiased estimates and standard errors for daily light interception (LI; MJ m–2 d–1) as a function of year of commercialization and growing environment. Fitted lines have a common slope equal to –0.0097 MJ m–2 d–1 year–1 (Table 3). LI for 1930 for USA 2012, USA 2017, Chile 2011, Chile 2017, and Chile 2019 is 28.2, 24.8, 26.5, 27.4, and 22.9 g MJ–1, respectively.
Dry matter accumulation increased with year of commercialization
Crop growth rates varied among hybrids (P<0.001), environments (P<0.01), and for hybrids within environment (P<0.001). Therefore, BLUEs for the trait were calculated using all three factors. Modern hybrids grow faster than older hybrids. The rate of change over year of commercialization was 0.11 g m–2 d–1 year–1 (Table 3). The estimate for the random effect of the environment on the intercept relative to the standard error of the estimate (35.1 ± 25.9) indicates that observed differences were not significant (Fig. 4).
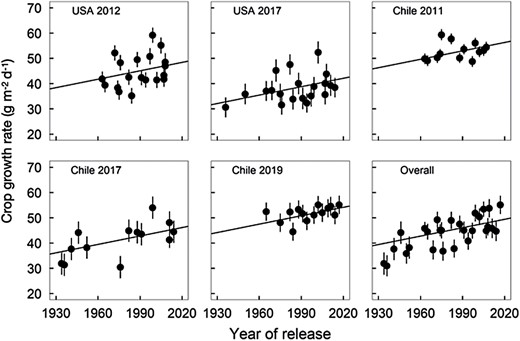
Best linear unbiased estimates and standard errors for crop growth rate (CGR; g m–2 d–1) as a function of year of commercialization and growing environment. Fitted lines have a common slope equal to 0.11 g m–2 d–1 year–1 (Table 3). CGRs for 1930 for USA 2012, USA 2017, Chile 2011, Chile 2017, and Chile 2019 are 38.4, 32.1, 46.3, 36.1, and 44.2 g MJ–1, respectively.
Radiation use efficiency increased in response to long-term selection for yield in maize
Significant hybrid (P<0.001), environmental (P<0.01), and hybrid×environment interactions (P<0.001) were detected for RUE. Therefore, BLUEs were calculated for each hybrid and location. RUE for 1930 was estimated at 1.54 g MJ–1, with a rate of improvement between 1930 and 2017 of 0.0049 g MJ–1 year–1 (Table 3). The cumulative increase in RUE over a century of breeding was 0.49 g MJ–1, which corresponds to an RUE for 2020 of 2.03 g MJ–1. The estimated random effect for the environment effect on RUE in 1930 suggests that RUE varied among the locations (0.11 ± 0.08; P<0.1; Fig. 5). RUE in 1930 at location i was correlated with amplitude of monthly temperature during the first 4 months after planting (y= –3.6 ± 0.7 + 0.28 ± 0.39×x; r2=0.94; df=3; Fig. 6) but neither with mean temperature nor with VPD (Fig. 6).

Best linear unbiased estimates and standard errors for radiation use efficiency (RUE; g MJ–2) as a function of year of commercialization and growing environment. Fitted lines have a common slope equal to 0.0049 g MJ–2 year–1 (Table 3). RUEs for 1930 for USA 2012, USA 2017, Chile 2011, Chile 2017, and Chile 2019 are 1.33, 1.31, 1.71, 1.31, and 2.05 g MJ–1, respectively.
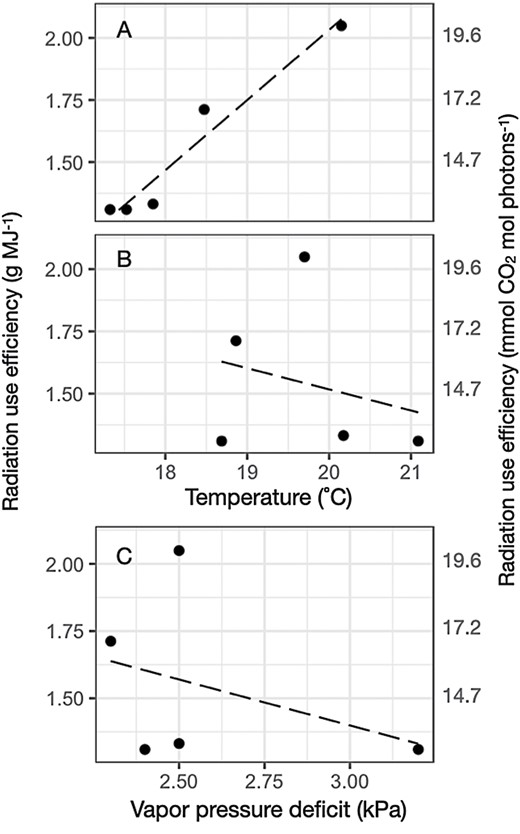
Correlation between the predicted radiation use efficiency (RUE; g MJ–2) for (A) the location and temperature amplitude (°C; r2=0.94; P<0.05), (B) mean temperature (°C; r2=0.06; P=0.68), and (C) vapor pressure deficit (kPa; r2=0.13; P=0.54).
Discussion
The objective of this study was to answer a long-standing question about the plausible role of leaf erectness and RUE in the widely reported yield improvement in the ERA maize hybrids (Duvick et al., 2004; Cooper et al., 2006, 2014). This study focused on the longest running commercial maize breeding program in the world and included hybrids commercialized in all decades from 1930 to 2020. During this period of breeding, both yield and leaf erectness changed at a rapid rate (Cooper et al., 2006). Five experiments were conducted in environments with high dry matter accumulation and yield potential, and were managed intensively to minimize biotic and abiotic stress. Measurements were taken during a period of maximum light interception and prior to the onset of N remobilization (Sinclair and Muchow, 1995; DeBruin et al., 2013) to minimize the effect of factors that may affect the estimation of RUE. Maximum observed RUE values were among the highest reported in the literature (Sinclair and Muchow, 1999; Lindquist et al., 2005) but lower than theoretical potentials (Loomis and Amthor, 1999; Long et al, 2006), demonstrating the effectiveness of the experimental approach. Results were consistent but higher than theoretical calculations for whole-season RUE (Yin and Struik, 2015), probably due to differences in light interception during incomplete canopy cover. This study demonstrates that (i) RUE increased as a consequence of long-term selection for yield (Table 3; Fig. 5); (ii) the expression of this change varied among locations, possibly associated with differences among the locations in seasonal temperature amplitude (Figs 2, 5, 6); (iii) the magnitude of the change in RUE is sufficient to explain ~32% of the observed change in yield during a century of breeding as estimated by Cooper et al. (2014); and (iv) there is a significant RUE gap that warrants further studies to unravel the physiological underpinnings of the change and to explore opportunities to accelerate genetic gain by engineering and/or augmenting selection through direct selection of photosynthesis/respiration (Long et al., 2006; Yin and Struik, 2015; Bailey-Serres et al., 2019; Hammer et al., 2019; Reynolds et al., 2021). This result conforms with the observation that long-term selection for yield increased yield potential and decreased the yield gap (Cooper et al., 2020) for a wide range of evapotranspiration conditions but not extreme water deficits.
Amplitude temperature may underpin variation in RUE between environments
Across-environment hybrid variation for RUE conformed well to previous reports (Kiniry et al., 1989; Sinclair and Muchow, 1999; Lindquist et al., 2005). However, mean RUE varied between locations. Although RUE decreases with decreasing temperature (Andrade et al., 1992, 1993) and with increasing VPD (Kiniry et al., 1998), RUE was correlated neither with mean temperatures (r2=0.06; P=0.68) nor with VPD (r2=0.13; P=0.54) across locations. In contrast, RUE was highly correlated (r2=0.94; P<0.05) with mean amplitude temperature (Fig. 6). Millet et al. (2019) reported a negative correlation between kernel number and night temperature that is consistent with the positive correlation between RUE and temperature amplitude observed in this study. Kernel set depends in part on growth, and thus RUE, around flowering time (Andrade et al., 1999; Borrás and Vitantonio-Mazzini, 2018). A reduction in RUE due to high night temperatures would be conducive to kernel abortion (Messina et al., 2019); however, it is not possible to rule out effects of night temperature on hastening kernel development (Cantarero et al., 1999). Because minimum temperatures were far below optimal temperatures for photosynthesis (Fig. 2; Rotundo et al., 2019), the evidence suggests that cold nights may have decreased respiration, and thus increased RUE (Di Mateo et al., 2018; Reynolds et al., 2021). Loomis and Amthor (1999) estimated that respiration can decrease RUE from 2.7 g MJ–1 to 1.5 g MJ–1 due to variation in maintenance respiration, which exceeds the range observed in this study. While the number of environments is arguably small, the estimated average RUE for each location resulted from the evaluation of several hybrids, making this estimate robust (Table 2; Fig. 5), and thus strengthening the case for investigating the processes undermining the reported association (Fig. 6).
RUE increased over a century of maize breeding
This study provides evidence that RUE increased as a consequence of selection for increased yield over almost a century of maize breeding (Fig. 5). Previous studies focused on characterizing changes in RUE in northern North American, tropical, and semi-flint Argentinian germplasm (Tollenaar and Aguilera, 1992; Bolaños and Edmeades, 1993; Luque et al., 2006). Despite the magnitudes and moment during the growth cycle when differences in RUE became apparent, there is agreement among studies that RUE, or other estimates of efficiency (Crosbie et al., 1978), underpin yield improvement in maize. The results are also in agreement with outcomes from simulation studies that predict yield improvement under well-watered conditions in response to increases in leaf erectness and RUE (Hammer et al., 2009; Messina et al., 2009, 2011). These predictions were based on a theoretical framework that links canopy architecture, light penetration, and leaf photosynthetic efficiency to predict canopy photosynthesis and RUE (Duncan, 1971; Hammer and Wrigh, 1994). The agreement between theoretical analyses and empirical results from experimentation presented in this study provides strong support for a significant contribution of RUE to genetic improvement for yield in maize. Two hypotheses are proposed for future evaluation.
Hypothesis 1: RUE improvement is associated with N uptake and canopy maintenance
Although the contribution of improved RUE to yield is hard to dispute, it is feasible to propose a companion hypothesis that involves the inseparable link between RUE, N, and yield. While changes in leaf erectness may have improved light penetration and consequently RUE (Pendleton et al., 1968; Hammer and Wright, 1994), they may have also stimulated the retention of N stored in the lower leaves within the canopy (DeBruin et al., 2013), the N uptake pre-flowering (van Oosterom et al., 2010a; Sinclair and Sheehy, 1999), the maintenance of leaf N during grain fill and the consequent delay of leaf senescence (Tollenaar and Aguilera, 1992; van Oosterom et al., 2010b; Mueller et al., 2019), and finally yield. Additional illumination of the ear leaf could have increased plant growth during the critical window for kernel set determination, and thus contributed to yield improvement (Perez et al., 2019), Following Sinclair and Muchow (1999) and Sinclair and Sheehy (1999), and assuming that (i) specific leaf N is 1.8 g m–2; (ii) 65% of N in leaves is remobilized to kernels; and (iii) kernels contain 0.8 g kg–1 of N (DeBruin et al., 2013), a leaf area index of 7.4 is required to produce 1600 g m–2 of grain yield. In the absence of improved light penetration within the canopy, it is unlikely that a maize crop has the ability to maintain pre-flowering specific leaf N above the critical value of 1.8 g m–2 (DeBruin et al., 2013) in order to support kernel growth. In conclusion, the changes in RUE are interpreted as both a cause of yield improvement and also the manifestation of high N uptake pre-flowering that contributes to the stay green phenotype (Duvick et al., 2004), which is required to support high growth rates and yield.
Hypothesis 2: RUE improvement is due to stress tolerance
Genetic gain for yield in maize has been associated with the improvements of maize tolerance to stresses that often occur in the TPE and with the improvement of resource use efficiencies (Duvick et al., 2004; Messina et al., 2020, Preprint). The results presented in this study suggest that the tolerance to stress occurs for RUE, in addition to reproductive resilience (Messina et al., 2021). Indeed, this study shows that RUE increased throughout a century of breeding to close the gap between current and potential RUE but not to increase the RUE potential (1.5–2.03 g MJ–1 versus 2.7 g MJ–1, Fig. 4; Loomis and Amthor, 1999). Whigham (1971) reported that maize leaves tied in an upright position showed delayed symptoms of wilting and cooler leaf temperature than leaves laying in a flat position on high demand days. Barker et al. (2005) reported a negative correlation between year of commercial release and canopy temperature in a sequence of 18 ERA hybrids, and attributed this result to the lower radiation intensity on the more upright leaves of modern hybrids. Erect leaves reduce the irradiance per unit leaf area (Duncan, 1971; Hammer and Wright, 1994) and can decrease photo-damage and high metabolic costs of repairing proteins such as D1 in the core of PSII (Loomis and Amthor, 1999). It is unknown whether changes in the xanthophyll photo-protective system occurred, which could have improved non-photochemical quenching at PSII and reduced photo-damage to the canopy. Leaf orientation and an enhanced xanthophyll cycle were implicated in the photo-protection of young soybean leaves (Jiang et al., 2006). Further research is warranted to test the hypothesis that genetic improvement for RUE is due in part to improved canopy architecture effects on radiation load, leaf temperature, leaf water status, and increased photo-protection. Outcomes from this research in combination with high-throughput field phenotyping of photosynthesis (e.g. Furbank et al., 2019; Meacham-Hensold et al., 2020) can lead to new opportunities to accelerate genetic gain for RUE and yield in maize (Long et al., 2006; Murchie and Niyogi, 2011; Bailey-Serres et al., 2019; Hammer et al., 2019).
Perspectives for genetic improvement
Yields measured in experiments conducted in the central US corn belt increased at a rate of 8.6 g m–2 year–1 (Cooper et al., 2014). Under those experimental conditions, it is feasible to grow a crop yielding 1600 g m–2, which is higher than the 10 year average for the state of Iowa of 1137 g m–2. Provided that the harvest index remained constant at the optimal plant population for the hybrid (Duvick et al., 2004), for maize to reach this yield it must intercept 675 MJ m–2 of solar radiation during the growth cycle. Considering this value for light interception, a trend in RUE equal to 0.0049 g MJ–1 year–1 translates to a trend of 3 g m–2 year–1 in yield, which is ~32% of the observed trend in yield for experiments conducted in the US corn belt (Cooper et al., 2014). This calculation suggests that RUE was a major physiological process contributing directly to genetic improvement for maize yield in the US corn belt. Because growth and tolerance to crowding are related (Messina et al., 2019), an indirect contribution of RUE to crop improvement would make this contribution greater than 32%.
The magnitude of this result motivates inquiring about future opportunities to continue improving RUE. Considering that the potential for RUE is ~2.3–2.7 g MJ–1 (Loomis and Amthor, 1999; Long et al., 2006), the results presented here suggest an existing RUE gap between 0.3 g MJ–1 and 0.7 g MJ–1. Closing this gap would be equivalent to the genetic gain for yield due to RUE improvement over the last century of maize breeding in the central US corn belt with the additional contribution to combat climate change through carbon sequestration (Burney et al., 2010). This hypothesis could be tested within active breeding programs. The use of predictive frameworks that can accommodate the incorporation of physiological knowledge and multiple traits (Messina et al., 2018, 2022) to inform decisions in combination with prior knowledge on the genetic architecture of canopy traits (e.g. Tian et al., 2011; Li, et al., 2015) will be necessary to accelerate genetic gain while increasing RUE.
Acknowledgements
The authors thank Andrea Salinas, Logan Anderson, and Karen Thompson for their assistance with field experimentation.
Author contributions
CDM, GLH, and MC: conceptualization and writing; CDM, CG, AR, and MC: conducting the field experiments; JR, YF, EVO, and LB: calculations and statistical analyses. All authors contributed to the manuscript.
Conflict of interest
JR, AR, YF, and LB are employed by Corteva Agriscience.
Data availability
Hybrids described in this publication may be made available to the academic community and other not-for-profit institutions solely for non-commercial research purposes depending on seed availability and upon request through Corteva openInnovation portal. https://www.openinnovation.corteva.com
Comments